Analysis of EEG Signal using nonextensive statistics
Pragati Patel, Ramesh Naidu AnnavarapuDepartment of Physics, School of Physical, Chemical, and Applied Sciences, Pondicherry University, Puducherry, 605014, ***
Abstract - Electroencephalogram (EEG) signal is the most effective, quick, and abundant source of information in understanding the brain related phenomenon. New avenues for EEG-based research in non-medical streams can also be seen with the growing number of qualitative and affordable wearable EEG headsets. But it is extremely hard to assess the information from EEG signal. However, information-theoretical approaches have appeared as a potentially beneficial means to gauge variations in the EEG datasets. This article discusses one such approach: the ‘measure of Tsallis entropy (TsEn)’ to explore and investigate the available natural data. This study set out to critically review the renowned research papers on Tsallis entropy-based EEG signal processing to understand the trends in EEG signal processing research. It attempts to provide practitioners and researchers with insights and future directions for applicability of Tsallis entropy for EEG signal processing and with an emphasis on the suitability of EEG research for clinical studies. It reviews about 35 published papers dividing into medical and non-medical domains and discusses the crucial role of Tsallis parameter ‘q’ in studying complex EEG systems. The result shows Tsallis's non-extensive initiatives seem to be more discriminatory than its Shannon counterpart and all other entropy variants and hence, can preferably be used to study the brain. The paper also concludes that Tsallis entropy offers a comprehensive test of any theory and it proves the efficacy of EEG research in clinical detection and therefore is highly significant in biomedical signal processing.
Key Words: Tsallis Statistics, Non-Extensive Entropy, EEG, EEG data-sets, Biomedical Signal Processing.
1.INTRODUCTION
In1803,amathematicianLazareCarnotdevelopedtheentropytheorywhenhesawthatvitalityisreducedduetofrictionand scattering[1,2].Thisentropyconceptwasmainlyusedintwobranchesofphysics;statisticalmechanicsandthermodynamics, intheearlierdaysofitsinvention.Laterin1948,thisthermodynamicentropywasintroducedasdataentropyintotheworldof dataanalysisbyShannon[3].ShannonentropyispreciselydevelopedfromtheBoltzmann-Gibbs(BG)statisticalmechanicsand standardthermodynamicsandwasprovedtobeefficientinthestudyofthecomplexityofsystems[4,5].Despitetheircolossal effectiveness, this BG concept-led entropy discusses only extensive structures with short-range interactions and fails for nonextensivesystems. In1988,Tsallisproposedanentropicexpressionwithanindexqthatresultsinnon-extensivestatistics. Tsallisentropy, ,buildsthefoundationofnon-extensivestatisticalmechanics. Numerousphenomenahavebeenstudied using non-extensive (Tsallis)statistics in a variety of fields, including physics, chemistry, biology, medicine, economics, geophysics,etc.ThisarticlefocusesonapplicationandsignificanceofTsallisentropyinEEGdataanalysis[6][7]
Thestudyofcomplexstructureshasdrawnsignificantinterestlately,andthebrainbeingthemostcomplexamongthem.There are various neuroimaging or brain scanning techniques to directly or indirectly image the brain's structure, function, or pharmacology.Owingtoits excellenttemporal resolution,electroencephalography(EEG)seemstobethemost advisable methodforstudyingthetemporalvariationofbrainactivity[8].Thebrain'selectricalactivitycharacterizedbyEEGisindeed verycomplicated.Retrievingtherightcharacteristicsfromthistimeseriesiscrucialinbrain-relatedresearch.Thereisarange of linear and non-linear approaches available to study EEG time series[9]. However, information-theoretical techniques, preciselythenonextensiveentropy-basedmethod,recentlyappearedasamostpromisingapproachforretrievingreliable information from EEG. Tsallis entropy being the most effective and robust information theoretic technique, can lay the foundationforareal-timedecision-makingaidinmanyfields,consideringitsarithmeticcodingisquick[10–12].Tsallisentropy hasprovenusefulincharacterizingsystemswithlong-rangeinteractionsinthepastthirtyyears[13–20].Thisstudyattemptsto reviewthisnonextensiveentropy'sapplicabilitytoEEGanalysisfordifferentpurposes.
1.1 Tsallis entropy in Biomedical Signal Processing

Often,biomedicalsignalsaredisruptiveandinconsistent.Theygeneratedatabasesthatarehigh-dimensionalandcomplicated. Theoutcomesofconventionalbiomedicaldataanalysistechniquesmaybeaffectedbytheexistinginterferenceorintrusionin thedata.Consideringthisproblem,nonextensiveinformationentropyhasemergedasareliableestimatorofcomplexityor uncertainty in the signal with various implementation scope. Bock et al. have formulated an early detection strategy for

AlzheimerdisorderthattakesTsallisentropyasanattributeandresultsinanaccuracyof77%[12].Zhangetal.provideda feasiblestudytodeterminetheoccurrenceofburstsandsuppressionsaftercardiopulmonaryinfarctioninratsviaTsallis entropyapplicationtoEEG.Inhisresearch,hediscoveredthatTsallisEntropyArea(TsEnA)correlateswellwithneurological outcomes,andwiththevalueofqsetto3,itcancontributetopreliminarydiagnosticestimatesabouttheretrievalofcerebral functionquicklyandefficiently[21].Asin[22],authorshaveemployedtheTsallisentropyfeaturetoestimaterhythmchangein EEGfollowingthebrainischemia.Thestudywascarriedoutusingq=1.5,3,and5withfourEEGrecordingstodiscriminate asphyxiaandearlyrecoveryperiodfromthebase-lineEEG.Resultssuggesttheentropyusedcanquantitativelydetectchanges producedbyvariationsofexperimentalsettings.Liangetal.publishedanextensivestudyonentropymeasurestomonitorthe depthofanesthesia.ThemethodemployedtwelveindicesalongwithTsallisentropy,andoutcomessuggestTsallisandRenyi permutationentropyperformedbetterthanShannon'sentropyinevaluatingtheeffectofanesthesia[23].S.Karetal.used TsallisentropyforEEGsignalanalysistoassessandquantifydriver'sfatigue,indicatingthatthedescribedapproachcanbe appliedonboardtodeterminethefatiguelevelindriversofanyfield[24].RobertRicheretal.carriedoutastudyonmental stateidentificationinreal-timeusingTsallisentropy.AuthorsfoundthatTsallishadthemaximumsensitivitywhereasRenyi hadmaximumspecificity.Asaresult,thefocusedandrelaxedstateisbestdeterminedbyTsallisandRenyibasedentropy measuresrespectively[25].

1.2 Brain Mapping With EEG
AnEEGplaysaroleindiagnosingbraindisordersandalsofortheadvancementofEEGbasedbraincomputerinterfacesystems. Electroencephalographyoffersawaytoinvestigatetheworkingsofthebrainandtomaplinksofone regionofthecentral nervoussystemtoother.EEGisutilizedinvariousresearchprotocols,medicalandnon-medical.Ithasbeenprovenhighly favorableasa diagnosticaidinpatientswithseverebraininjuries, braintumors, encephalitis,memoryproblems,stroke, cerebralinfections,sleepdisorders,epilepsy,andmultipledegenerativediseasesofthenervoussystem.Itisalsoeffectivein theevaluationofpatientswithpossiblebraindeath.Wheninadruginducedcoma,EEGisadvantageoustoestimatetheproper depthofanesthesiainpatients.EEGisconventionallyconductedinwell-controlledlaboratoryenvironments,butwithrecent technologicaldevelopments,portablemonitoringandlong-termmonitoringEEGhavealsobecomewidespread.Thepresent paperalsoexploresthesignificanceofEEGresearchinstudyingthebrain.
1.3 Mathematical Background
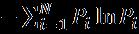
Asystem'scomplexitycanbedefinedusingentropy.Since1948,Shannon'sentropyisthefundamentalandmostwidelyused entropytoevaluatesystemcomplexity.Mathematically,Shannon'sEntropyis[3],
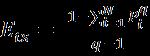







WhereNarethemicroscopicconfigurationsofthesystemand istheprobabilityofoccurrenceofthe thconfiguration.The sumoftheprobabilitiesshouldbeunity,i.e., isbasedonstandardthermodynamicsandBoltzmann-Gibbs statisticalmechanics,andtherefore,themicroscopicmemoriesandinteractionareofshortrange[26] isadditive,meaning,
ThesystemXandYareindependent.
Shannon’sentropyhashadremarkableachievementintheinterpretationoftheextensivesystems.Despitethat,itcannot properlydescribeabruptchangesandlong-rangeinteractions[27].Togetoverthisdisadvantage,anon-additivestatisticwas proposed[6,26],theTsallisentropy.Itwasdefinedas
When , reducestothedefinitionof as:
isnonextensiveandfollowsthefollowingruleofpseudoadditivity[26]
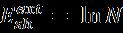

Intheaboveequations,qisaparameterthatmeasuresthedegreeofnonextensivity[28].q=1correspondstoextensivity,that is,Shannon'sentropy.Ontheotherhand,q<1correspondstosuperextensive, andq>1 correspondstosubextensive[ ]statistics.Tsallis'Entropyisextremeatequiprobability. Thisextremumisdescribedas[26]
Inthelimitq 1thisreducestotheextremumofShannon'sEntropy
TheworkofTsallisprovidesageneralizationofBoltzmann-Gibbsstatistics,whichcanappropriatelyexplainsystemswithlongrangeinteraction[26].Thoughthisgeneralizationhasbeenknownfromformercenturiesinthethermodynamiccontext,itis nowgainingbroaderapplicability.TsallisEntropyhasrecentlybeenextensivelyadoptedinbiomedicalfieldslikeECG[29]and EEG[5,30]signalprocessing.ResearchfindingshaveshownthatTsallisentropycanyieldthesystem'senhancedtechnicalities thanthetraditionalShannonentropy[5,29,31].EEGoffersinformationaboutlargescaleinteractionofbillionsofneurons.Due totheselong-rangecorrelations,nonextensivityisinstinctiveinEEG[32].Thus,usinganonextensiveapproachratherthanthe conventionalShannonentropyapproachisreasonableandessentialtoperspectivethelong-rangeconsequencesintheEEG dataset[33].Moreover,sincethereisassociativeinformationbetweenthevariousneuralpopulations,itisfairtoclassifyEEGas asubextensiveframework[5,30,34].Forinstance,considertwoneuronalclustersXandY,thenentropyrelationshipofthe twosystemsisstatedas
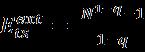

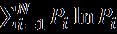

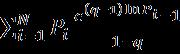
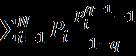
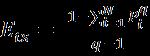



1.5 Main Contributions of The Paper
Duringourliteraturereview,weobservedthatarticlesonTsallisentropycutacrossvariouskindsofEEGprocessing.Hence, conducting an analysis of articles comprising of EEG processing using Tsallis entropy could be of great use for literature reviewstoresearchers.Inshort,contributionsofthisarticleare:
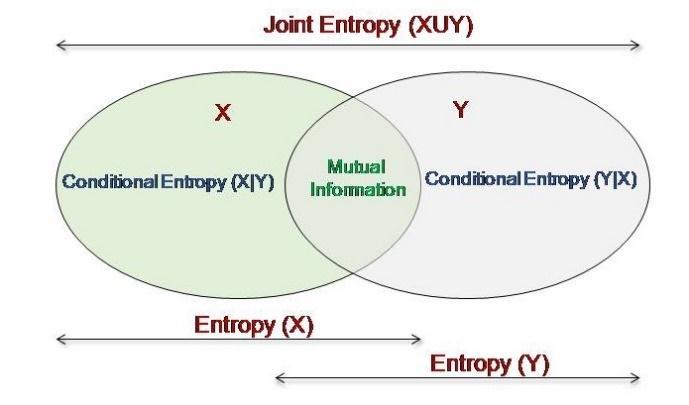
i. AllpossibleresearchworksthathaveemployedTsallisentropyfeaturetoanalyzeEEGsignalstostudyefficacyof TsallisentropyandEEGinmedicalandnon-medicaldomainhavebeentakenintoaccountinthisreview.

ii. Fromanalysis,it'sbeendiscoveredthatthenon-extensiveTsallisentropyindeedperformsbetterthanitsShannon counterpartinmanyofitsapplications.
iii. ThisanalysiswillassistresearchersastowhyTsallisentropyshouldbeprioritizedoverotherentropyfeatureswhen dealingwithanynon-extensivesystems.
iv. This will also assist learners in better comprehension of all EEG datasets available for medical and non-medical research.
v. Finally,throughthepresentreviewandanalysis,certainfindingsandopinionshavebeenlistedforfurtherstudiesin thisfield.
ThefollowingsectionsillustrateourreviewframeworkforTsallisentropybasedonallpossiblepublishedresearcharticles. Section2presentsourframeworkforreviewanalysis.ResultsanddiscussionarediscussedinSection3.Forfuturestudies, Section 4 offers an insight for EEG research as well as Tsallis entropy application. Finally, Section 5 presents the study’s conclusionbydiscussingthechallengesandtrendsinTsallisentropy-basedEEGsignalprocessing.
2. METHODOLOGY
TsallisentropyforEEGprocessinghasitsvastapplicationinbothmedicalandnon-medicalfieldsandarticlesonthesamecan befoundindifferentmultidisciplinaryjournalsnamely:PhysicaA,ClusterComputing,HealthcareEngineering,PhysicsLetters, BiomedicalEngineering,FrontiersinComputationalNeuroscience,MedicalImagingandHealthInformatics,Transportation Research,PatternRecognitionLetters,ClinicalNeurophysiology,Neurocomputing,Entropy,BiomedicalSignalProcessingand Control.Therefore,classifying,analysingandsummarizingtheresearchatoneplatformwillprovideinsightstoitsfuture aspectsinEEGsignalprocessingusingTsallisentropy.Ageneralizedblockdiagramoftheproposedmethodologytoreviewthe `Tsallisentropy-basedEEGsignalprocessing'isgiveninFigure2.
Firststepisextractingtherelevantarticlesfromvarioussources.Wesearchedforpapersinourselectedrepositoryacrossa periodoftwentyyears,from2000to2020.Secondstepisthefiltrationandselectionwhichhadstrictinclusionandexclusion criteria,asfollows
Inclusion Norms:Publicationsforgettingselectedforthereviewneedtobepartoftherelevantconferenceproceedingsormust besearchablethroughGoogleScholarsearchengine.Thefirst200articlesfeaturing`EEGdataprocessing'and`Tsallisentropy' init,areonlyselectedfromthesearchenginemethod.Alltheselectedarticlesarefromyears2000to2020.

Exclusion Norms:ArticlesthatarenotrelevanttotheTsallisentropyareruledout,asdefinedbytheprescribedsequenceof manualanalysis;Initially,publicationsthatspecificallydonotinvolveTsallisentropyrelatedresearchworkaredisqualified. Then,allconferenceproceedingsandGoogleScholarpublicationsareeliminatedonthebasisoftheirtitleand,lastly,their entirecontent.
Result:Intotal,88articleswereselectedfromGoogleScholarsearchengineandconferenceproceedingsusingthedescribed selectionnormandthenfurtherfiltereddownaccordingtoexclusionrule,resultingin35articles.Nextstepistheclassification of the article based on their application domain which makes the further analysiseasier and effective. All 35 articles are classifiedintotwodomains;medicalandnon-medical.Detailsoftheclassificationschemeisillustratedinfigure3.
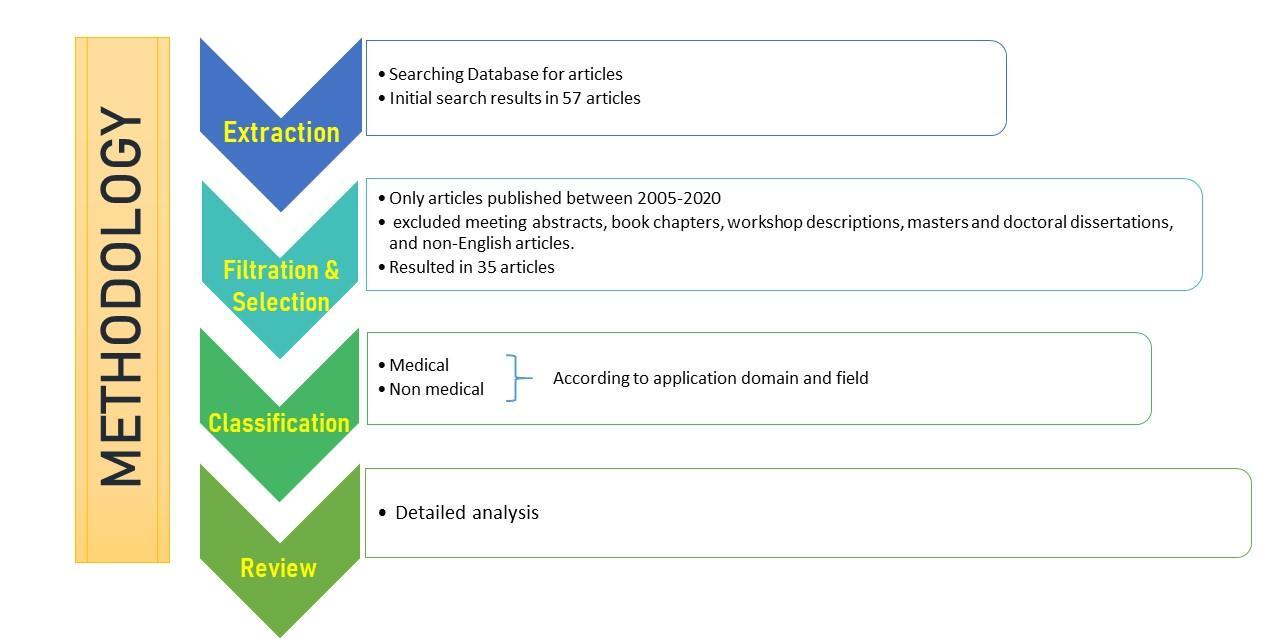
Afterclassification,finalstepistoanalyzeandreviewit,whichisdiscussedinthenextsection.
Table -1: DescriptionofalltheEEGdatasetsanalyzedusingTsallisentropyinvariousstudies.
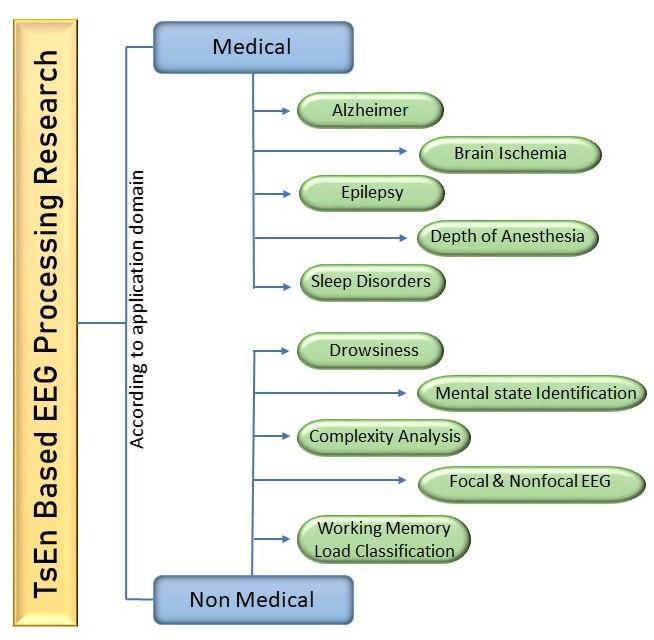
Dataset Description

BrainIschemia
Datasettoexploreneuronalactivityinthreedifferentstagesrelatedtocardiac arrest; normal,hypoxic,and asphyxic.15adultsmaleWistarrats(300-350g, mean=330g)havebeenarbitrarilygiven7minutes(10rats)or9minutes(5 rats) of asphyxial attacks, as per the experiment decorum sanctioned by the Animal Care and Use Committee of John Hopkins Medical Institutions. To guaranteeitsnomajorimpactsonEEGdataset,4.5%ofhalothanewasinjected toeachrat.BaselineEEGwasrecordedfor10minfollowedby5minwashout, finallyfollowedby7or9minsofglobalasphyxia.Subjectsweremaintainedata temperatureof36.5-37.5 .TwochannelsofEEGwererecordedintheleftand rightparietalareaswithagroundelectrodeinmidline.AdvancedCODASdata acquisition software was used to digitize the signals obtained from EEG electrodes,atasamplingrateof250hzandA/Dconversionof12bit.TheEEG datasetsrecordedwerethenfilteredwithabandpassfilterof0.5-70hzandECG artifactswereremoved.Thedatacanbecollecteddirectlyfromtheauthorsof originalarticles.
References
[22,30,35–37]
Epilepsy
BERN BARCELONA DATABASE: Datasetincludeslong-termintracranialEEG datarecordedfromfivelongstandingpharmacoresistanttemporallobeepilepsy patientsattheDepartmentofNeurology,theUniversityofBern.Multichannel EEGdevicewasusedwithanextracranialreferenceelectrodeplacedbetween 10/20 positions to record data. Data was then down sampled at sampling frequencyof512Hz.Brainareaswithseizureswerelocalizedforallfivepatients. Atlast,fourth-orderButterworthfilterwasappliedtofilterdataoffrequency 0.5-150Hz. Data available to download at:
https://www.upf.edu/web/ntsa/downloads

BONN UNIVERSITY DATABASE: Thisdatabaseincludesdatafromthreestages ofepilepsy;thenormal(SetA(eyesopen)andSetB(eyesclosed)),thepre-ictal (SetCandSetD)andtheonewithseizures(SetE),whichhave200,200and100 datapoints,respectively.Eachdatapointis23.6secondslongandisrecorded from a single EEG electrode. The complete data was recorded with a 128 electrodeEEGdeviceatasamplingfrequencyof173.61Hz,anddigitizedwitha 12bitA/Dresolution.Itwasthenfilteredwitha0.5-85Hzbandpassfilter.Data anditsdetailsavailabletodownloadat:
http://epileptologie-bonn.de/cms/upload/workgroup/lehnertz/eegdata.html

Alzheimer
DERRIFORD HOSPITAL DATABASE: Itcomprisesoftwodatasetsrecordedat Derriford Hospital (Datasets A and B). Dataset A has EEG recordings from 3 patientswithAlzheimer'sdiseaseand8healthyindividuals(over65yearsold), all of them from similar age groups. Dataset B comprises of 24 healthy individualsand17individualswithaprobabilityofhavingAlzheimer's,andare notfromthesameagegroup.Themeanageinnormalgroupsis69.4 11.5,with aminimumof40,maximumof84,and42%ofmaleinthem.Themeanageinthe Alzheimercategoryis77.6 10.0,withtheminimumof50andmaximumofis 93,ofwhich53%aremale.DatasetAwasrecordedusingthetraditional10-20 systeminaCommonReferenceMontageandconvertedtoCommonAverageand Bipolar Montages in software. Dataset B was recorded using the modified Maudsley system that is similar to the traditional 10-20 system. The EEG recordingsweresampledat128Hzinbothdatasetsandincludedifferentstates likeawake,hyperventilation,drowsyandalert,withperiodofclosedandopen eye.Datacanbeaccessedfromhospitalwithstrictprotocol.
[10,42–44]
SleepState Separation
Depthofanesthesia
DataconsistsofEEGrecordingsfrom20healthynewborninfants(10boysand 10girls)agedbetween282 9days,duringfourbehavioralstates;quietsleep (QS),activesleep(AS),quietwakefulness(QW),andactivewakefulness(AW). TheEEGwasrecordedusing19electrodesofEEGfor108.4±9.6minutes(mean ±SD)ofduration.EOGandECGwerealsorecordedforartifactreductionfrom data. The EEG signal was amplified using a REFA-72 amplifier (TMS InternationalB.V.),andrecordedat256Hzsamplingratewith69Hzbandwidth.
EEG dataset during sevoflurane-induced anesthesia: The first dataset comprisesof19patientsagedbetween18to63yearswhofastedanddidnot undergopremedicationforatleast6hoursbeforeanesthesia.Thesesubjects weretoundergoforelectivegynecologic,general,ororthopedicsurgery.Priorto have EEG recordings, an electrode-skin impedance of less than 7.5 k was maintained.AthreeelectrodeEEGdevicewasusedtorecordsignalsfromFpZ (active), Fp1 (earth), and F8 (reference). The readings for sevoflurane concentrationwastakenatthemouthat100/s[46]
EEG data set during isoflurane-induced anesthesia: The second dataset comprises29patients(9menand20women,agedbetween33and77years) undergoing elective abdominal surgery during combined isoflurane general anesthesiaandepiduralanesthesia.FiveEEGelectrodes(A1,A2,FP1,FP2,and
[23]
Fatigue/Drowsiness
FPz(ground electrode)) were placed on the patients according to the International 10-20 System prior to anesthesia induction. The EEG data was sampled at sample frequency of 512 Hz. Isoflurane concentrations were intentionallykeptatfixedlevels(1.5,1.3,1.1,0.9,and0.7%)for30minutesat each level. The EEG recordings at 0.3 and 0.5% isoflurane were recorded immediatelyaftertheoperation[47]
Thetwosetsofdatadescribedabovecanbecollecteddirectlyfromauthorsof therespectivearticles
ItconsistsofEEGdatafrommultipleparticipantsduringasetofthreeactualand simulatedscenarioexperiments,whichwererecordedusinga32-channelEEG device. Experiment 1 consists of 21 healthy male participants (professional drivers)agedbetween25-35years.Theseparticipantswereaskedtodrivefor1 hourinabusytrafficthenunderwentacomputerizedsubjectiveandpsychomotortest.TheEEGdatarecordedbeforeandaftertheexperimentarelabeled as‘Level1’\&‘Level2’respectively. Experiment 2 consistsoftwelvehealthy maleparticipantsagedbetween20–35yearsforsimulateddrivingtaskswith sleepdeprivation.Participantsunderwentphysicalexerciseonatreadmillfor2–5 min to generate physical fatigue; simulated driving for about 30 min to generatephysical,visual,andmentalfatigue;auditoryandvisualtasksfor15 min to generate mental and visual fatigue; finally, the computerized game relatedtodrivingforabout20min.Asinglephaseofexperimentlastedfora periodof3hoursandthecompleteexperimentranforapproximately36hours. Three minutes of EEG data was recorded at start and end of each phase of experiment. Experiment 3 consistsof7subjectswhoperformedactualdriving tests for validation. The data was sampled at 256 Hz with 16-bit A/D conversion.Datacanbeaccessedbyaskingtheauthors.
MentalState Recognition
CAP(Cyclic Alternatingpattern)
EEGdatawererecordedusingaMuseHeadbandhaving4activeelectrodesand acommonmodereferenceelectrode.TherecordedEEGwasthenseparatedinto fivedifferentfrequencybands.Datawasoriginallyover-sampledat12kHzand thendown-sampledat220Hz.Completedatawasrecordedinanofficespace from eleven participants aged between 28.1 4.6 years (M SD). The study procedureconsistedofmultipletaskslikeMentalArithmetic,Dictation,Where’s Waldo.Eachtaskisassociatedwithoneofthreestatesofmind:Neutral,Focus, Relax. The Neutral phases are reference measurements with no specific instructions given, except not to close their eyes. For Focus phases, the participants performed different tasks to generate high levels of mental processingandbindingdifferentsenses.DuringtheRelaxphases,participants relaxedthemselves.Datacanbeaccessedbyaskingtheauthors.
The dataset is recorded from four normal individual and four patients with sleep-disorderedbreathing.TheEEGdataissampledatasamplingfrequencyof 512Hz.ThedatasetcanbeaccessedfromonlinedatarepositoryPhysionetat https://physionet.org/content/capslpdb/1.0.0/ [49]
WorkingMemory Load
Itcontainsdatafrom12healthymalesubjectsagedbetween24-30yearswhile theydidanarithmetictaskofvaryingdifficultylevel.Thedifficultylevel was manipulated by varying the n-digit numbers used and carries required to calculatetheaddition.Inthebaseline restconditiondata takenfromrelaxed subjects with their eyes closed. To minimize any muscle movement artifact (EMG) any unnecessary physical movements were avoided with their hand placedin a fixed position. EEGsignalswere recordedusing32 EEGchannels devicewithalinkedearlobereferenceandimpedancekeptunder5k .TheEEG signalswerethenfilteredusingband-passfilterofcut-offfrequencyof0.1-100 Hzanddownsampledatafrequencyof256Hz.
[50]

Parkinson’sdisease
DataconsistsofEEGrecordingfrom62subjects;46personswithParkinson’s disorderlabelledas“PD”and16personswithoutParkinson’sdisorder,matched to patients according to sex, age, and education, and labelled as “HC”. Parkinson’s disorder was diagnosed according to the United Kingdom Parkinson’sDiseaseSocietyBrainBankcriteria.Thepatientswhohaddementia, historyofstroke,epilepsy,multiplesclerosisandsurgicalinterventionstothe brain,or/andinsufficientknowledgeofGermanlanguage,wereexcluded.
3. RESULTS AND DISCUSSIONS
WecollectedseveralarticlesonTsallisentropy-basedEEGsignalprocessingvianumerousweb-basedsources.Everyselected article was analyzed and categorized as per the classification method discussed above. Though the range of the article collectionwasnarrow,itprovidesadetailedoverviewofTsallisentropy-basedEEGsignalprocessingresearch.Allthearticles selectedafterfilteringprocesswerefurthersortedbasedontheirresearchthemes.
Articlesbasedontheirareaofimplementation,canbebroadlycategorizedanddescribedintotwocategories:MedicalandNonmedical.
Medical Context:Articleisclinicallycontextualifitisintendedtoprovideaid,improvement,tracking,evaluationanddiagnosis ofhumanmentalandneurologicaldisorders.
Non-Medical Context:Comprisesofarticlesthatareintendedtoamuse,instruct,train,track,orenhancegamingexperience ande-learningthroughTsallisentropyanalysisofEEGdatabase.
Inordertoidentifythepublicationsinthemedicalfield,thefollowingcriteriawereused:
a. Ifthearticleaddressedahealthcondition,suchasamentalorneuraldisorder;
b. Ifarticleeitherenrolledrealpatientsasthesubjectsofthestudy,orinvolvedtwogroups:onecomprisinghealthy individuals,othersofpatients;
c. Ifthestudywasperformedinaclinicalenvironment;
d. and/orthearticlewasorientedtoprovidenewmethodofaidtoanyhealthconditions.

23.51%ofallthearticlesselectedwerefoundasmedicalarticles,afterreviewingitallasperthemedicalclassificationcriteria mentionedabove.Themajority,76.5%,werenon-medical.Figure4demonstratesthenumberofmedicalandnon-medical publicationswiththeiryearofpublication.Also,allpossibledatausedhavebeendescribedundertable1.
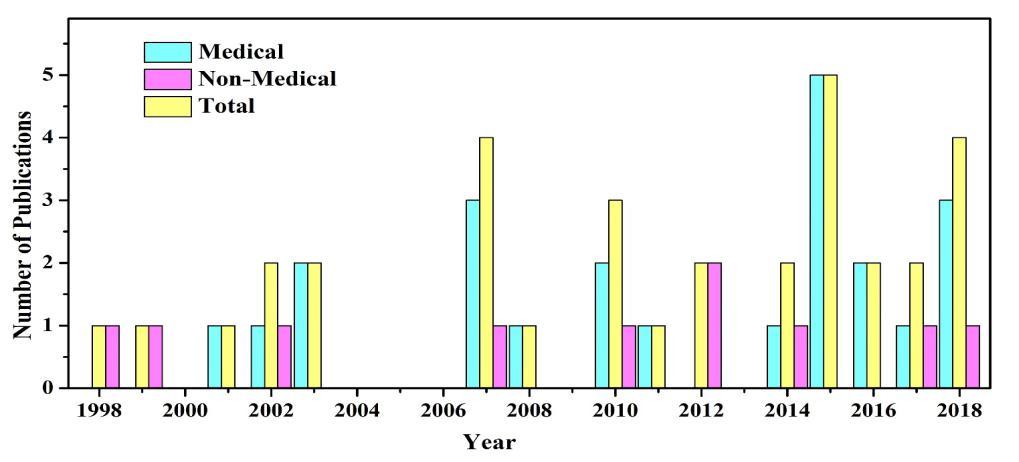
3.1 Medical context Articles
3.1.1 Brain Ischemia
Approximately 60% of all individuals who safely revived a cardiac arrest eventually die each year from traumatic brain damage.Therealtimesurveillanceofthebrainasphyxiaorhypoxiaconditionafterheartfailuresurvivalisstillacrucialhealth concern.Therearen'tanystandardscientificreal-timeobjectiveevaluationsbeingusedtotrackthesebraininjuries.

Zhangetal.[37]inhisworkusedTsallisentropytoquantifythelevelofbrainischemiaaftercardiacarrestbasedofpresenceof burstsuppression.InordertoconstructastandardizedTsEndependentmeasure,namedTsEnarea(TsEnA),Zhangcombined threediscriminatoryBurstSuppression(BS)characteristics,i.e.,burstfrequency,BSratioofamplitude(BSR),andBSlength (duration). EEG bursts and suppression are a representation of cortical neuron excitability and refractory time, respectively[52–54].Previousfindingsshowthat:1)theburstfrequencyisgreaterforparticipantswithpositiveneurological outcomes[55,56],and2)theEEGshowssuppressionphasei.e.,itisflatorsteadyfornegativeneurologicaloutcomes[37,57] Therefore,thequickerthenormalizationofcorticalneuronalactivity,theshorterthelengthofBSinEEG.WithriseinBSR,TsEn fallsmonotonicallyandsignificantlyandthisreductionofTsEnismoreprevalentinanylivingbodywithimpairedneurological performance.FindingsfromZhang'sstudyshowsthatTsEnAcorrelateswithneuronaloutcomesaroundqvalueof3andcan thereforeofferearlyprognosticinformationoncerebralfunctionalrecoveryaccuratelyandeffectively.
S.Tongetal.employedTsallisandRenyientropyapproachesinhisresearchtoanalyzebrainelectricalactivityafterhypoxicischemic(HI)injury[30].ThestudyrevealedthatTsallisentropywascapableofrecognizingthevariationsinlocalirregularity generatedbythe"spiky"burstbehaviorduringinitialasphyxicrecovery,whereasRenyientropyhasbeenthemostconciseand predictivemeasureofphysiologicalchangesinthebrainduringrecovery,andwasshowntobesensitivetoassesstherateof recovery.
Bezerianosetal.inhisstudyofEEGrhythmchangesusedShannonandTsallisentropy(q=1.5,3,5)withwindowsizew=128 datapoints,slidingsteps∆=1andamplitudepartitionL=10.
Inhiswork,fourEEGrecordingswereanalyzedandsignificantdifferencesbetweenbase-lineEEGandEEGafterasphyxiaupto theinitialphaseoflaterecoverywasobservedstatistically.ItwasfoundthatTsallisentropycandiscriminatethedifferent injury levels and different segments of recovery; the asphyxia period~silent period as well as the early recovery burst~suppressionEEGsuccessfullyfromthebase-lineEEG[22]
3.1.2 Alzheimer’s Disorder
Alzheimer'sisaneurodegenerativeconditionthataffectsthenervecellsandistheleadingcauseofdementia,whichinvolvesa varietyofsyndromessuchaslossofmemoryandtroublewiththinking,balanceorconcentration,problem-solving,orspeech andlearning.Proteinsaccumulateanddevelopplaquesortanglesinsidethebrainduringthisdisorder.Thatweakensthebond betweennervecells,andconsequentlythenervecellsdieandbraintissuesarelost.Almost44millionindividualsworldwide have Alzheimer's or associated dementia. Just 1 in 4 is diagnosed with it. While an effective therapy or medication for Alzheimerhasnotyetbeenfound,itisplausibletodecreaseitsconsequencesifidentifiedatanearlystage.Thetestingof Alzheimeriscurrentlyconductedthroughanelectroencephalography.Asbiosignaturesofdementia,informationtheoretic approacheshaveappearedasahugelypowerfulmethodofmeasuringvariationsintheEEG.Tsallisentropyleadsamongthe mostprominenttheoreticinformationmethodsforassessingvariationsintheEEG.
PZhaoetal.haveresearchedaboutEEGinterpretationinAlzheimer'sdisorderusinginformationtheoreticapproaches.He tookintoaccountthetwodatasetsAandB,thenusedLZW(Lempel-Ziv-Welch)algorithmandtheTsallisentropymodelto yield compression ratios and normalized entropies for each participant, respectively. The findings suggest that both parameters(compressionratioandnormalizedTsallisentropy)arelesserinAlzheimer'spatientthanforthehealthyindividual groupandindicatethatinformationtheoreticapproachproducesahighlybeneficialtechniquetoproduceEEGmarkersfor Alzheimer[10]
TheuseofTsallisentropyasascreeningtoolforAlzheimer'sdisorderhasalsobeenstudiedbyA.H.Al-nuaimietal.Foreach EEGelectrodeandforeveryparticipantfromareferencedatasetthatincludesdementiaandnormalindividuals,theTsallis entropywascalculated. Tohighlightthevariationsbetweentheentropyfornormalindividualanddementiapatients,the entropyvaluescalculatedwerethennormalized.ThesenormalizedTsallisentropyvalueswereusedtoconstructreference featurevectors,onefordementiapatientsandonefornormalindividuals.Andthereafter,todifferentiatebetweenAlzheimer
patientsandhealthyornormalindividuals,thefeaturevectorforthenewdatasetwascomparedwiththereferencevectors usingK-meansclustering.InordertoobtaintheAlzheimer’sbiomarkers,theentireEEGprocessingwassplitintotwophases (developmentphaseandtestphase)andTsallisentropywasestimatedinbothphases.Withthesensitivityof100%&85.7%, specificityof50%&70.9%,accuracyof84.6%&78.8%andprecisionof72.7%&77.4%fordatasetA&Brespectively,itcanbe impliedthatthemethodcanformthebasisasafirstlinedecision-makingaidfordementiaevaluation[42]
P.Lazaretal.carriedoutaworkonAlzheimer’sEEGanalysisinempiricalmodedecomposition(EMD)domainusingTsallis thresholdingi.e.EMDwasimplementedasanalternateapproachtoprocessAlzheimersignal.Theaccuracyandreliabilityof EMDdependssolelyonathresholdparameter,forwhichTsallisentropyhasbeenusedinhiswork.TheinputEEGsignalwas decomposedbyEMDintofiniteintrinsicmodefunctionsandaresiduesignal,thenwithuseofTsallisentropytheoptimal thresholdvaluewascalculated.Further,aneuralnetwork,whichisaninformationprocessingparadigm,istrained,whichgives theclassifiedoutputeitherasAD-affectedorasnormalbrain.TheresultsshowthatclassificationrateisimprovedwithTsallis entropy-basedthresholding[44].
3.3.3 Epilepsy
Epilepsyisaneurologicalconditioninwhichrepetitiveepisodesofseizuresoccurs.Whileseizurescanbeidentifiedbymeans ofanelectroencephalogram(EEG),itisquitehardtodistinguishthevariousstagesofepilepsylikenormal,preictalandictalby visualobservation,becauseofthenon-linearandnon-stationarycharacteristicsandalsothelong-termrecordingsoftheEEG signals.Inliterature,numerousstudieshavebeenperformedtoauto-detecttheseizuresforbothshort-termandlong-termEEG recordings,Tsallisentropyasoneoftheapproaches.
M.Thilagarajetal.intheirstudyusedTsallisentropyfeaturewithfivedifferentclassifiers;NaïveBayesclassifier(NBC),radial basisfunction(RBF),decisiontrees:functionaltree(DTFT),KNNandadaboost.Tenfoldcrossvalidationtechniquewasusedfor evaluatingtheclassifierswithobjectivetoclassifyabnormalandnormalstates.StudyconcludedthatTsallisentropyissimple yetthefastestmethodwiththeleastcomputationtimeof0.9msandcanbeconsideredforrealtimedetectiontasksasitoffers 92.67-100%accuraciesforbinary(two-stages)problems[41].
N.ArunkumarinhisstudyforautomaticdetectionofepilepticseizureshaveemployedTsallisentropyfeaturestogetherwith otherfeatureslikepermutationentropyandKolmogorovcomplexityalongwithfivedifferentclassifiers.Maximumaccuracyof 89.33%wasachievedwithsensitivityandspecificityof85%and84%respectively,withDTclassifier.However,themethodhas beentestedonalimitedsizeofdataandneededtogetcheckedwithotherlargedatabaseforconsistentresult[38]
KaiFuetal.usedTsallisandotherentropyfeaturesalongwithSVMclassifierwithRBHkernelintheHilbertmarginalspectrum domaininsteadofthecommonlyusedFourierspectrumdomainforautomaticseizuredetection.Theoutcomesofthestudy showthatthemarginalspectrumapproachoffershigherprecisionthanFourierspectrumanalysis.Itseemsfromthefindings thattheapproachsuggestedisnotsubstantiallybetterthantheFourieroneforparametersotherthanaccuracy.However, since the results of the classification correspond to the dataset, feature extraction and learning algorithm apart from the methodsofsignalanalysis,itisassumedthatthemethoddiscussedisapotentialtoolfordetectingseizuresinEEGsignals[40]. AlltheworksdiscussedabovehaveusedBonnuniversitydataset,detailsofwhichisgiveninTable1.
3.1.4 Depth of anesthesia
Generalanesthesiaisessentialinthesurgeryunittoensurethesafety,comfortandsuccessfulsurgeryofpatients.Anesthetic drugsmajorlytargetthecentralnervoussystem(CNS)whichcontrolmostbodilyfunctions.Theelectroencephalogram(EEG) which originates in the CNS represents the neuronal functions of the brain and has been extensively used as a reference parametertomeasuretheeffectofanesthesia.ThemeanamplitudeoftheEEGsteadilyincreaseswithdeepeninganesthesia andthenthisamplitudedecreasesintherecoverystate.Withtheemergenceofdataanalysis,differentapproacheshavebeen usedtoassesstheeffectsofanesthesia.
Zhenhu liang et al. in his work to evaluate depth of anesthesia using entropy used Tsallis wavelet entropy and Tsallis permutationentropytogetherwith12otherextensiveandnon-extensiveentropyfeatures.EEGdatasetduringsevofluraneand isoflurane-inducedanesthesiahasbeenutilizedforthisstudy,detailsofwhichisgivenintable1.Theresultsshowedthat TsallisPermutationentropy(TPE)andRenyipermutationentropy(RPE)werebetterthanShannonpermutationentropyin determiningtheeffectofanesthesia.SimilarfindingswerealsoseeninTsalliswaveletentropy,Renyiwaveletentropyand Shannon wavelet entropy respectively.NoresearchusingTPE or RPEhave ever beenconducted to monitorthe depth of Anesthesiabefore.Theexceptionalperformancerevealstheirpossibleutilityinstudyingdepthofanesthesia[23]

3.1.5 Fatigue/Drowsiness
Thetermfatigueisusedtodescribeaconditionorstateofmindthatlacksalertnessandhaveminimalmentalorphysicalwork outputs,usuallyfollowedbydrowsiness.ThisDrowsinesscangiverisetoseverefatalitiesincertainprofessionlikethatof pilots,drivers,electricpowerandsteelplantemployees.Itisthereforeofcriticalinterestandimperativeneedtoformulate methodsthatcansensedrowsiness/fatigues.Acrossarangeofneuroimagingmodalitieswhichcanbeappliedforfatigue detection,EEGisregardedasthemostprominentandaccurateone.Significantnumberofresearcharticlesarepublishedto studytheprobabilityofimplementingEEGdeviceanditsdatasettoidentifyfatiguesordrowsiness.
S.karetal.inhispaperstudiedvariousfatiguerepresentingvariablesbasedonhigherorderentropymethodsofEEGdatain thewaveletdomain.It'sbeenreportedthatwiththegrowthinfatiguerate,theentropyvaluerises.Theworkaffirmsthatthe extensiveentropies(Shannon'sEntropyandRenyiEntropy)havesignificantachievementinidentifyingthefatiguelevelsthan thenon-extensiveones(Tsallisentropy)[24].Completedetailsofthedatausedforthisworkingivenintable1.
A.Zhangetal.alsopresentedastudyonTsallisentropyapproachtodetectthedrowsiness.InhisworkEEGdatarecordedfrom 20healthyvolunteersduringtheawakeanddrowsinessstatehasbeenused.AllfourtypesofEEGrhythmsweresubsequently extracted,andthencomplexityofeachrhythmwasstudiedwithTsallisentropy.ThefindingssuggestthattheTsallisentropyof EEGthetarhythmintheawakestateissignificantlygreaterthanthestateofdrowsiness.So,theTsallisentropyofthetarhythm canbeusedtoaccuratelydetectthedrowsinessinreal-time[48]

3.1.6 Mental State Recognition
Informationregardinginnermentalstatescanbeapreciousassetinnumerouscases.Itcouldbeutilizedtoavoidpotential hazardsliketrafficfatalitiesbytrackingmentalfatiguesindrivers.EEGheadsetsalongwithotherwearabledevicescanbeused to track psychological state in patients with certain psychological disorders like anxiety or depression. Other potential application includes the measurement of various forms of reaction in response to an external stimulus. It can be used to customizethecontentorstyleandtoconductsocialanalysisifaperson'sreaction(whetheremotionalorrational)towardsa speech or advertisement is known. Devices like MRI scanners or medical grade EEG are being used in most of the latest researchonmentalstatemonitoring.Thereisstillaneedforasolutionthatcandeterminethementalstateoftheconsumerin real-timeandinaregular,everydayenvironment.
R. Richer et al. in their work incorporate and examines various methods to detect different mental state in real time by generatingscoresthatmeasurehowfocusedorrelaxedapersonis,usingasimpleEEGheadband.Thedatausedisdescribedin table1.Datawerepre-processedthennaïvescorecomputationandentropy-basedscorecomputationwereusedformental state recognition. Entropy based measures included Shannon entropy, Renyi entropy (for alpha=3), Tsallis entropy (for alpha=3) and Kullback-Leibler divergence. The findings suggest that for the Focused state, the Tsallis-based method outperformstheRenyi-basedmethod,whilefortheRelaxedstate,theRenyi-basedmethodperformedbest.Itrevealsthatthe Tsallismethodprovidesthehighestsensitivity,whiletheRenyimethodreachedthehighestprecision,anditalsoindicatesthat mentalstateidentificationcanbedoneinreal-time[25].
3.1.7 Cyclic Alternating Pattern (CAP)
Studiessuggeststhat,throughquantitativestudyofsleepdatabase,variousdisorderscanbeidentified.Identificationand evaluationofcyclicalternatingpattern(CAP)isanintegralpartofthesleepanalysis.AnditmustbeacknowledgedthatEEG analysisisthemosteffectiveapproachtotrackcognitivefunctionspecificallyduringthesleepperiod.SleepEEGdatabasenot onlyofferscyclicalternatingpatterns(CAPs)featuresbutalsootherdetailsofsleepphasessuchassleepandwakestatesof rapid and non-rapid eye movement sleep. CAPs are intermittent activities in EEG that interprets the sustained arousal instabilityoscillatingbetweenhigherandlowerarousalstagescharacterizedasphaseAandphaseBrespectively.CAPsshow variationsindisruptiveor unstablesituationscausedbyinternal(insomnia,depression,epilepsy,periodiclimbmovements, circadian constraints) and external (noise, ambient temperature) sources. The dataset used in this study is taken from Physionetdatarepository,furthersinformationisprovidedinTable1.
F.Karimzadehetal.examinedasetofentropy-basedmethodsalongwiththreeclassifiers;supportvectormachine(SVM),knearestneighbor(KNN),andlineardiscriminantanalysis(LDA)todifferentiateCAPandnon-CAPinanEEGdatabase.Inhis research,thesleepEEGof4healthyvolunteersand4patientswereexaminedbythestandardandproposedmodelstoevaluate allofit.EntropybasedfeaturesincludedTsallisentropytogetherwithShannonentropy,spectralentropy,sampleentropy, Higuchifractaldimension,KolmogoroventropyandbandfeaturewithKolmogoroventropywerecalculated.Thenbestrelevant
featureswereselectedthroughaheuristicalgorithmcalledsequentialforwardselection(SFS),thenthethreeclassifierswere used to classify the EEG signal. Findings shows that conventional features like band powers and Hjorth parameter can’t measurethemigrationofbrainstatefromCAPtonon-CAPpreciselyandentropycanbetterdetectthemigrationbetweenthese brainstates.TheresultsfurthershowthatShannonentropyoutperformedtheTsallisentropy,becauserangeofvariationof ShannonentropyishigherthanthatofTsallisentropy[49]
3.1.8 Working Memory Load
Assessingthelevelofcognitivememoryloadwhilepracticingacognitivetaskisofutmostimportanceforvariousfactors;to minimizetheerrorindecision-making,foradvancementofefficientuserinterfaces,topreventmemoryexhaustionandretain efficiencyandpotencythroughoutanycognitiveactivities,alsoinprofessionswithcritical/highmentalpressures;likepeople involvedinthemilitaryandemergency/interventionmedicinefields.Variousapproachesareavailabletodayforassessing workingmemoryload,likebehavioural/physiologicaltechniquesorperformance-based/subjectiveratingsapproach.Ofthese, EEGisrankedasthebestphysiologicalapproach,providingbetterprecisionandtolerancewhenevaluatingmemoryload.A numberoflinearandnon-linearmethodsarebeingimplementedtocalculatetheworkingmemoryloadusingEEGdata.
P.Zarjametal.intheirstudyinvestigatedtheapplicabilityofwavelet-basedcomplexityassessmentapproachofEEGsignalsto examinevariationsinworkingmemoryloadduringtheexecutionofa cognitivetaskofvariousdegreesofdifficulty/load. Wavelet-complexityassessmentsapproachrelatedwithdifferententropymethods-Shannon,Tsallis,Escort-TsallisandRenyi entropyhavebeenemployedtodifferentiatebetweensevenloadlevelsofworkingmemory.Detailsofdatasetusedforthe studyisgivenintable1.Sourcelocalizationworksuggeststhattaskloadlevelsmainlyinfluencethefrontalandoccipital regions.Foreveryentropyfunction,measuredcomplexitydatarenderedthatcomplexityvalueriseswiththeescalationintask load.ExtractedfeatureswerethenfedintoaArtificialNeuralNetwork(ANN)classifier.Tsallisentropygaveanaccuracyof 94.18%infrontalregionand88.36%inoccipitalregion,butlagsbehindtheaccuracyofShannon’sentropy[50]

3.1.9 Sleep State Separation
Avarietyofextremelycomplicatedbehaviorsariseduringsleepingstageinthebrain.Significantresearchhasrecentlybeen conductedtoanalyzethedifferentsleepstatesanditslinktoallotherpsychologicalprocesses.Yet,informationregardingsleep stagesishardlyacknowledged.Thenumberofindividualshavingsleepdisordersisveryhigh.Sleepdisordersnotonlycause varioushealthproblemstotheindividualsbutalsohardentheirday-to-dayactivitiestoagreatextent.Previousfindingsalso reportedthatsleepmayhaveasignificantroleinmemoryconsolidationwheresomememories aredevelopedwhileother lesser valuable memories are erased. Thus, providing an effective framework for sleep supervision and sleep behaviour analysisisofgreatsignificance.TheautomatedidentificationofsleepphasesfromEEGdatasetisabigchallengeandseveral methodshavebeenproposedforthesame.
N.A.Loafgrenetal.conductedastudyonsleepstageseparationviaentropyestimationusingamarkovmodelofEEG.Ifthe samplesarecorrelated,thesignalismorepredictableandprobabilitydensityfunction(PDF)ofonesamplewillusuallybe affectedbytheprevioussample.Therefore,directentropyestimationwilloverestimatetheentropywhenconsecutivesamples arecorrelated.Toapproachthisissue,theauthorsconsideredthesignalsasmarkovmodel.Thenmeasuringentropyfromthis markovmodelofEEGtransitionmatrixistakenintoconsiderationwhichcontainstheinformationregardingthetransitions amongvariousstates.Loafgrenemployedfouralgorithms;Shannonentropy,Shannonentropy ofamarkovmodel,Tsallis entropy,Tsallisentropyofmarkovmodeltostudysleepstageseparation.Theresultssuggestthatthesleepstageseparation canbeimprovedbyusingtheextrainformationgiveninthetransitionmatrixascomparedtojustusingtheprobabilitydensity function.TheseparationexpressedbytheaverageTstatisticismorethandoubledwhentheentropyisbasedonaMarkov Model of the EEG. However, the difference between the performance of Shannon entropy and Tsallis entropy is less pronounced[45]
3.1.10 Parkinson’s disease
Parkinson's disease (PD) is the second most common neurodegenerative disease resulting primarily from the death of dopaminergicneuronsinthesubstantianigra.Itisestimatedtoaffectnearly2percentofthoseoverage65.Accordingtothe National Parkinson Foundation (NPF), over the entire course of their illness, 50 to 80 percent of those suffering from Parkinson’sdiseasegraduallydevelopdementia.Theestimateddurationfromtheoccurrenceofdifficultieswithmobilityto dementiaprogressionisaroundtenyears.AccuratelydiagnosingParkinson’sDisease–especiallyinitsearlystages–requires experiencedpractitioners.Thus,providingtoolsfordetectingearlychangesinbrainactivitythatareaseasytouseastaking one’sownbloodpressureisimportant.
S.M.Kelleretal.carriedoutresearchoneffectivenessofcomputationalEEGinstudyingParkinson’sdisease.Thestudywas focusedonhowtocomplementandimproveexistingsignalpower-basedclassificationmethodsforclassifyingindividualswith Parkinson’sdiseasefromthehealthyonesby(i)introducingadditionalentropy-basedfeatures(Tsallisentropywithq=2),(ii) includingeyesopenandeyesclosedstatesintothemeasurementprocessand(iii)usingtheBergereffectasapotentialfeature indicative of abnormal brain activity. Dataset used for this work is described in table 1. Best result is achieved with a combinationofeyesclosedandeyesopenmeasurementsusingTsallisentropy(q=2)andbandpowerfeatures[51]
3.2 Role of Tsallis Parameter ‘q’
LiteratureshowedthesignificanceofTsallisentropyinextractinginformationfromcomplexnon-extensivesystems.However, theTsallisparameter‘q’iswhatrendersthepeculiaritytoTsEnestimate.TheTsallisparameter‘q’functionslikeazoomlens forthebiomedicaldatasetslikeEEGwhichcanreflectlongaswellasshort-range(burstorspikes)rhythmchangesindatawith lowandhigh‘q’valuesrespectively.Inspiteofthesignificantroleof‘q’ininformationextractionfromcomplexnon-extensive systems through Tsallis entropy, no technique of optimizing its value has been developed yet. Analysts often undertake contrastingvaluesofqtoexploreEEGoranyotherbiomedicalsignals,andrefinetherangeofqbasedoncertainprecedentand thenatureofdatabeingstudied.FormerstudiesonTsallisstudiesalsoshowsthatwiththeparameterq,thevalueofTsallis entropyreducesconstantlywhereas‘thespike-encounter-potential’increaseswithq.ItcanalsobeinferredthatTsallisentropy isassociatedtoneuronalconditionsandcanthusbeusedtoprovideearlydiagnosticinformationaccuratelyandeffectively. Table2summarizesdifferentvaluesofTsallisparameter‘q’indifferentworksbytheresearchers,whichcanbeuseful for furtherstudiesusingTsallisentropy.
4.RESEARCH DIRECTION
PresentreviewinthefieldofTsallisentropy-basedEEGanalysissuggestswaysofimprovingtheresearchintwodirections; firstconcerningthealgorithmorthemethod,i.e.,Tsallisentropy,andsecondinthefieldofEEGresearch.

ForEEGtobeperfectlysuitableforclinicalandnon-clinicaluse,thereareseveralobviousfuturedirections.Firstofallisthe optimizationofthenumberofelectrodesused.Thereare32,64,128and256electrodevariationEEGdevicesavailablefor study.Consideringdatafromalltheelectrodesmakesexperimentaltimesetupandthecomputationtimeanissue.Minimizing the use of the number of electrodes would be ideal for a more convenient application. The second direction of research addressesthesizeofthesampleordatasets.Asofnow,EEGdatasetsforalmosteveryresearchapplicationisrelativelysmaller than needed. A larger dataset is needed to examine or efficiently analyze the neurological disorders or to produce and implementanyalgorithm.ThestudyforcertaindisorderssuggeststheneedforastandardproceduretorecordEEGsignalsin botheyesopenandeyesclosedstate.FutureworksareneededinexaminingthealreadydevelopedEEGindicatorsagainst knownbiomarkersforalargerdataset.Third,investigatingthelongitudinalchangesinEEGoverrelativelyshortperiodsatthe individual level is needed to enhance the EEG based research outcomes. Fourth, data fusion should also be considered a potentialfieldofresearchforbetterdiagnosisofdisordersandadvancementinEEG-basedBCIapplications.
Possiblefuturedirectionsconcerningthemethod,i.e.,thenon-extensiveTsallisentropy,canbethemethod'svalidationina more realistic environment and its extension in other undiscovered application such as in the development of real time emotionrecognitionsystemoranyotherBCIapplicationwhereasignificantimprovementinaccuracyispossible.Also,in studiesconcerningdetectionofdiabetesinitsearlystages,autism,othermentaldisabilitieslikeADHD,dyslexia,schizophrenia. FutureworkwillalwaysbeopentofuseorintegrateotherfeatureswithTsallisentropytoexpectbetteroutcomes.
5.CONCLUSION
Thecomplexityisadistinguishingyetenigmaticcharacteristicofanyphysiologicalsystem[61].Thestableorbalancedsystems show variation in complexity related to long range interactions within the system along with other different nonlinear interactionswhereasthecomplexitydisintegrateswithinstabilityordisordersinthesystem.Thereisstillagreatnecessityof quantitativemeasureswhichcancalibratemedicaldatasetinmultipleways,therebysimplifyingthestudyofbrainwaves obtained from complex brain dynamics. For multiple issues, the Tsallis entropy approximation accurately estimated the entropyofmostEEGSignals.TheroleofTsallisentropyinbiomedicaldataanalysisdomainiscrucialfornumerouscauses, primarilybecausethetechniqueisemployedtostudyreal-worlddataasreal-worlddata.Thisoffersathoroughassessmentof anytheorythanthesimulateddata.ThebackgroundofEEGresearchconstitutesafurtherexplanationfortheimportanceof TsEninEEGfindings.EEGstudieshavenothistoricallydevelopedanytherapeuticapproacheswhicharesufficientlyreliablefor itsdirectmanifestationinmedicaldiagnosisexceptforseizuresandsleepdisorders.Manyresearchershadsurrenderedupon its effectiveness and claimed that statistical significance of EEG in medical research is unattainable. Our present review, however, indicates that such a despondency cannot be upheld. Rather EEG research in medical diagnostics only lacked techniques like Tsallis entropy for assessing the information in the EEG data. The purpose of the review conducted is to demonstratetheimportanceofi.EEGresearchintheadvancementofmedicalandBCIresearch,andii.applicationofTsallis entropy approach for the processing of existing intricate EEG signals. Study also concludes that despite of having high significance in complexity analysis of systems, optimization of Tsallis non-extensive parameter `q' remains to be studied further.Studyalsoshowsthatnon-extensiveTsallisentropyapproachgiveshighdistinctionofvariousstatesofasystemthan its conventional counterpart i.e. the Shannon entropy, which further suggests the construction of fully automated spike detectionsensorsbasedonTsallisentropyalgorithm.Amulti-diagnosissoftwarepackagecanalsobedevelopedandinstalled indifferentdiagnosticcenters.Priortotheimplementations,however,thetechniquewouldhavetobetestedextensivelyfor uniformityinprecision,specificityandsensitivitywithotherdiversedatabases.Inordertobroadlyassessthereliabilityofthis method,itisstillessentialtoacquirelargedatabases.
ACKNOWLEDGEMENT
TheauthorsacknowledgePondicherryUniversityforfinancialsupportthroughUniversityFellowship.

COMPETING INTEREST
Theauthorsdeclarethattheyhavenoknowncompetingfinancialinterestsorpersonalrelationshipthatcouldhaveappeared toinfluencetheworkreportedinthispaper.
AUTHORS' CONTRIBUTIONS
Pragati Patel:Conceptualization,Computation,Formalanalysis,Writing-originaldraft.
ContactDetails:pragatipatel.001@pondiuni.ac.in,ResearchGate:https://www.researchgate.net/profile/Pragati-Patel-2, Linkedin:https://www.linkedin.com/in/pragati-patel-8076631b8/,Orcid:0000-0003-1256-8923
Ramesh Naidu Annavarapu:Reviewing,EditingandSupervision. Allauthorsreadandapprovedthefinalmanuscript.
REFERENCES
1. MüllerI(2007)Ahistoryofthermodynamics:thedoctrineofenergyandentropy.SpringerScience\&BusinessMedia
2. NatererGF,CamberosJA(2008)Entropybaseddesignandanalysisoffluidsengineeringsystems.CRCpress
3. ShannonCE(1948)Amathematicaltheoryofcommunication.BellSystTechJ27:379–423
4. MartinMT,PlastinoAR,PlastinoA(2000)Tsallis-likeinformationmeasuresandtheanalysisofcomplexsignals.PhysA StatMechitsAppl275:262–271
5. Capurro A, Diambra L, Lorenzo D, et al (1999) Human brain dynamics: the analysis of EEG signals with Tsallis informationmeasure.PhysAStatMechitsAppl265:235–254
6. TsallisC(1988)PossiblegeneralizationofBoltzmann-Gibbsstatistics.JStatPhys52:479–487
7. TsallisC(1998)Generalizedentropy-basedcriterionforconsistenttesting.PhysRevE58:1442
8. Ishizaki R, Shinba T, Mugishima G, et al (2008) Time-series analysis of sleep wake stage of rat EEG using timedependentpatternentropy.PhysAStatMechitsAppl387:3145–3154
9. BabiloniC,BinettiG,CassettaE,etal(2006)Sourcesofcorticalrhythmschangeasafunctionofcognitiveimpairmentin pathologicalaging:amulticenterstudy.ClinNeurophysiol117:252–268
10. ZhaoP,Van-EetveltP,GohC,etal(2007)CharacterizationofEEGsinAlzheimer’sdiseaseusinginformationtheoretic methods.IEEEEngMedBiolMag1:5127

11. HamadicharefB,GuanC,IfeachorE,etal(2008)Performanceevaluationandfusionofmethodsforearlydetectionof AlzheimerDisease.In:2008InternationalConferenceonBioMedicalEngineeringandInformatics.pp347–351
12. DeBockTJ,DasS,MohsinM,etal(2010)EarlydetectionofAlzheimer’sdiseaseusingnonlinearanalysisofEEGvia Tsallisentropy.In:2010BiomedicalSciencesandEngineeringConference.pp1–4
13. PlastinoAR,PlastinoA(1994)Informationtheory,approximatetimedependentsolutionsofBoltzmann’sequationand Tsallis’entropy.PhysLettA193:251–258
14. AlemanyPA,ZanetteDH(1994)FractalrandomwalksfromavariationalformalismforTsallisentropies.PhysRevE 49:R956
15. Boghosian BM (1996) Thermodynamic description of the relaxation of two-dimensional turbulence using Tsallis statistics.PhysRevE53:4754
16. TsallisC,deAlbuquerqueMP(2000)Arecitationsofscientificpapersacaseofnonextensivity?EurPhysJB-Condensed MatterComplexSyst13:777–780
17. KoponenI(1997)Thermalizationofanelectron-phononsystemina nonequilibriumstatecharacterizedbyfractal distributionofphononexcitations.PhysRevE55:7759
18. JohalRS,RaiR(2000)Nonextensivethermodynamicformalismforchaoticdynamicalsystems.PhysAStatMechits Appl282:525–535
19. TsallisC(1995)Nonextensivethermostatisticsandfractals.Fractals3:541–547
20. PlastinoAR,PlastinoA(1993)StellarpolytropesandTsallis’entropy.PhysLettA174:384–386
21. ZhangD,JiaX,ThakorN,etal(2009)Featuresofburst-suppressionEEGafterasphyxialcardiacarrestinrats.In:2009 4thInternationalIEEE/EMBSConferenceonNeuralEngineering.pp518–521
22. BezerianosA,TongS,ThakorN(2003)Time-dependententropyestimationofEEGrhythmchangesfollowingbrain ischemia.AnnBiomedEng31:221–232
23. LiangZ,WangY,SunX,etal(2015)EEGentropymeasuresinanesthesia.FrontComputNeurosci9:16
24. KarS,BhagatM,RoutrayA(2010)EEGsignalanalysisfortheassessmentandquantificationofdriver’sfatigue.Transp RespartFtrafficPsycholBehav13:297–306
25 RicherR,ZhaoN,AmoresJ,etal(2018)Real-timeMentalStateRecognitionusingaWearableEEG.In:201840thAnnual InternationalConferenceoftheIEEEEngineeringinMedicineandBiologySociety(EMBC).pp5495–5498
26. Tsallis C (1999) Nonextensive statistics: theoretical, experimental and computational evidences and connections. BrazilianJPhys29:1–35
27. BorgesEP,RoditiI(1998)Afamilyofnonextensiveentropies.PhysLettA246:399–402
28. Gell-MannM,TsallisC(2004)Nonextensiveentropy:interdisciplinaryapplications.OxfordUniversityPressonDemand
29. TorresME,GameroLG(2000)Relativecomplexitychangesintimeseriesusinginformationmeasures.PhysAStatMech itsAppl286:457–473

30. TongS,BezerianosA,MalhotraA,etal(2003)ParameterizedentropyanalysisofEEGfollowinghypoxic ischemicbrain injury.PhysLettA314:354–361
31. GameroLG,PlastinoA,TorresME(1997)Waveletanalysisandnonlineardynamicsinanonextensivesetting.PhysA StatMechitsAppl246:487–509
32. RossoOA,MartinMT,PlastinoA(2002)Brainelectricalactivityanalysisusingwavelet-basedinformationaltools.Phys AStatMechitsAppl313:587–608
33. PatelP,NaiduRTsallisentropybasedstatisticalstudyofhumanemotionsthroughEEGSignal
34. Patel P, Balasubramanian S, Annavarapu RN (2023) Tsallis Entropy as Biomarker to Assess and Identify Human EmotionviaEEGRhythmAnalysis.NeuroQuantology21:135–149.https://doi.org/10.48047/nq.2023.21.01.NQ20009
35. TongS,BezerianosA,ZhuY,etal(2001)MonitoringbraininjurywithTsallisentropy.In:2001ConferenceProceedings ofthe23rdAnnualInternationalConferenceoftheIEEEEngineeringinMedicineandBiologySociety.pp1926–1928
36. TongS,BezerianosA,PaulJ,etal(2002)NonextensiveentropymeasureofEEGfollowingbraininjuryfromcardiac arrest.PhysAStatMechitsAppl305:619–628
37. ZhangD,JiaX,DingH,etal(2009)ApplicationofTsallisentropytoEEG:quantifyingthepresenceofburstsuppression afterasphyxialcardiacarrestinrats.IEEETransBiomedEng57:867–874
38. ArunkumarN,RamKumarK,VenkataramanV(2016)Automaticdetectionofepilepticseizuresusingpermutation entropy,TsallisentropyandKolmogorovcomplexity.JMedImagingHealInformatics6:526–531
39. ArunkumarN,RamkumarK,VenkatramanV,etal(2017)ClassificationoffocalandnonfocalEEGusingentropies. PatternRecognitLett94:112–117
40. FuK,QuJ,ChaiY,ZouT(2015)HilbertmarginalspectrumanalysisforautomaticseizuredetectioninEEGsignals. BiomedSignalProcessControl18:179–185
41. ThilagarajM,RajasekaranMP,KumarNA(2019)Tsallisentropy:asanewsinglefeaturewiththeleastcomputation timeforclassificationofepilepticseizures.ClusterComput22:15213–15221
42. Al-nuaimiAH,JammehE,SunL,IfeachorE(2015)TsallisentropyasabiomarkerfordetectionofAlzheimer’sdisease. In:201537thAnnualInternationalConferenceoftheIEEEEngineeringinMedicineandBiologySociety(EMBC).pp 4166–4169
43. Garn H, Waser M, Deistler M, et al (2015) Quantitative EEG markers relate to Alzheimer’s disease severity in the ProspectiveDementiaRegistryAustria(PRODEM).ClinNeurophysiol126:505–513
44. LazarP,JayapathyR,Torrents-BarrenaJ,etal(2018)Improvingtheperformanceofempiricalmodedecompositionvia Tsallisentropy:ApplicationtoAlzheimerEEGanalysis.BiomedMaterEng29:551–566
45. LofgrenNA,OutramN,ThordsteinM(2007)EEGentropyestimationusingaMarkovmodeloftheEEGforsleepstage separationinhumanneonates.In:20073rdInternationalIEEE/EMBSConferenceonNeuralEngineering.pp634
637
46. McKay IDH, Voss LJ, Sleigh JW, et al (2006) Pharmacokinetic-pharmacodynamic modeling the hypnotic effect of sevofluraneusingthespectralentropyoftheelectroencephalogram.Anesth\&Analg102:91–97
47. Hagihira S, Takashina M, Mori T, et al (2002) Changes of electroencephalographic bicoherence during isoflurane anesthesiacombinedwithepiduralanesthesia.JAmSocAnesthesiol97:1409–1415
48. ZhangA,BiJ,SunS(2013)AmethodfordrowsinessdetectionbasedonTsallisentropyofEEG.In:WorldCongresson MedicalPhysicsandBiomedicalEngineeringMay26-31,2012,Beijing,China.pp505–508
49. KarimzadehF,SerajE,BoostaniR,Torabi-NamiM(2015)PresentingefficientfeaturesforautomaticCAPdetectionin sleepEEGsignals.In:201538thInternationalConferenceonTelecommunicationsandSignalProcessing(TSP).pp448–452
50. ZarjamP,EppsJ,ChenF,LovellNH(2012)Classificationofworkingmemoryloadusingwaveletcomplexityfeaturesof EEGsignals.In:InternationalConferenceonNeuralInformationProcessing.pp692–699
51. Keller SM, Samarin M,MeyerA, etal (2018)Computational EEGinPersonalizedMedicine: AstudyinParkinson’s Disease.arXivPreprarXiv181206594
52. Schaul N (1998) The fundamental neural mechanisms of electroencephalography. Electroencephalogr Clin Neurophysiol106:101
107
53. SteriadeM,AmzicaF,ContrerasD(1994)Corticalandthalamiccellularcorrelatesofelectroencephalographicburstsuppression.ElectroencephalogrClinNeurophysiol90:1–16
54. Beydoun A, Yen CE, Drury I (1991) Variance of interburst intervals in burst suppression. Electroencephalogr Clin Neurophysiol79:435–439
55. GeocadinRG,ShermanDL,HansenHC,etal(2002)NeurologicalrecoverybyEEGburstingafterresuscitationfrom cardiacarrestinrats.Resuscitation55:193–200
56. JiaX,KoenigMA,VenkatramanA,etal(2008)Post-cardiacarresttemperaturemanipulationaltersearlyEEGbursting inrats.Resuscitation78:367–373

57. GeocadinRG,MuthuswamyJ,ShermanDL,etal(2000)Earlyelectrophysiologicalandhistologicchangesafterglobal cerebralischemiainrats.MovDisord15:14–21
58. CoronelC,GarnH,WaserM,etal(2017)QuantitativeEEGmarkersofentropyandautomutualinformationinrelation toMMSEscoresofprobableAlzheimer’sdiseasepatients.Entropy19:130
59. Al-QazzazNK,AliS,AhmadSA,etal(2016)Entropy-basedmarkersofEEGbackgroundactivityofstroke-relatedmild cognitive impairment and vascular dementia patients. In: Sensors and electronic instrumentation advances: proceedingsofthe2ndinternationalconferenceonsensorsandelectronicinstrumentationadvances.pp22–23
60. Lay-Ekuakille A, Vergallo P, Griffo G, et al (2013) Entropy index in quantitative EEG measurement for diagnosis accuracy.IEEETransInstrumMeas63:1440–1450
61. PatelP,AnnavarapuRN(2021)EEG-basedhumanemotionrecognitionusingentropyasafeatureextractionmeasure. BrainInformatics8:1–13