1/2022 RESEARCH LETTERS FMI’S CLIMATE BULLETIN
FMI’S CLIMATE BULLETIN 2 | FMI’S CLIMATE BULLETIN: RESEARCH LETTERS 1/2022
Seasonal preparedness pilot – sub-seasonal and seasonal predic tion service for tyre companies — 5 Development of the weather and climate service “CRITERION” for the touristic sector in e-shape — 8
CONTENTS
REVIEW BOARD ECRA community DESIGN Marko Myllyaho Please mention the source when citing the content. A DOI is available for each research letter article. © FMI 1/2022CRAESERHSRETTEL
Editorial — 4
EDITOR IN CHIEF Hilppa Gregow
Urban resilience to extreme weather - sub-seasonal and seasonal forecasts for winter maintenance activities in Helsinki — 11 Harvester Seasons – a forestry service supporting climate smart operations — 14 Seasonal climate predictions for German cities to strengthen urban resilience to climate variability — 17
The Kemijoki Hydrological Forecast System – a service supporting sydropower production in Northern Finland — 21 Future climate projection of heat indices for Austrian major cities: strengthening urban resilience and meeting user needs — 24
FMI’S CLIMATE BULLETIN: RESEARCH LETTERS Volume 4 Issue 1 ISSN: 2341-6408 DOI: 6408-IK-2022-01-RL10.35614/ISSN-2341PUBLISHER Finnish ilmastokatsaus@fmi.fiwww.ilmastokatsaus.fiFI-00101P.O.InstituteMeteorological(FMI)BOX503HELSINKI
RESEARC LETTERS
H
EDITORIAL COMMITTEE Juha A. Karhu Anna Luomaranta Tiina Ervasti
MIKKO STRAHLENDORFF space advisor, FMI’s Principal Investi gator of the EU -projecte-shape
Climate adaptation is local, and it happens differently depending on the location and differently around a year, so climate services have to be able to address this. Downscaling global gridded information in time and space means concretely to have seasonal averages turning into daily timescales and information updates to be available monthly. Space-wise 100 km global grids need to be turned into limited area neighborhood scales with under 100 m grids. The task is challenging – the auxilliary in formation like orography, land cover/vegetation would be needed in the higher resolutions both in time and in space. Now we have openly availa ble Earth Observation satellite data sets for many years that can provide this information. We can use machine learning to find the relationships between coarse and high resolution information.
EDITORIAL
Monthly Updated Climate Services
Seasonal forecasts are the tool to enable the transition to more fre quent climate services. They are produced monthly, looking forward half a year ahead and looking a full year ahead every 3 months. Their resolu tion is closer to climate predictions than to state of the art weather fore casts. The need to downscale and adjust biases is a prerequisite for a use ful service, but after this realizes, daily ensemble information is available to provide probability distributions to the end users and this information can be used for very specific questions. Currently most users are transi tioning from 10 day weather forecasts to using more statistical products. Subseasonal (~42 day) forecasts twice a week are helping to bridge the gap for these users.
The e-shape pilot climate services have developed many applications from sub/seasonal data and the letters in this issue describe the use cas es and the data value and production chains developed. Enjoy the read and get excited of what can be done already now – and imagine what could be done in a thousand more applications.
4 | FMI’S CLIMATE BULLETIN: RESEARCH LETTERS 1/2022
End users will need to transition to using climate services more often to adapt well in time, if infrastructure needs to be adapted.
As the delegate of Finland to the Group on Earth Observation (GEO) and the EU Space program Copernicus committee, it has been a truly ex cellent experience to combine the high political programming with con crete and ambitious service development for specific end users. Climate change is clearly a first priority in the EU Space program, and in GEO, one of four priorities. EU policy impact assessments on Earth Observation have, since 2007, always given climate change adaptation the biggest economical impact. We are still in the beginning of the development of adaptation services, as end users, like municipalities and trade organ izations, are only used to reading research reports now and then. For now, there is both a time and space limitation for service development. Reports are produced years apart, and the information is regional at best.
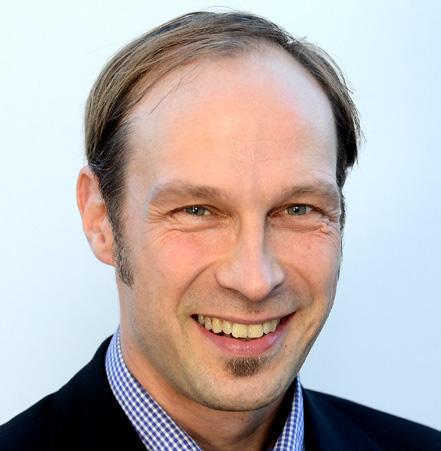
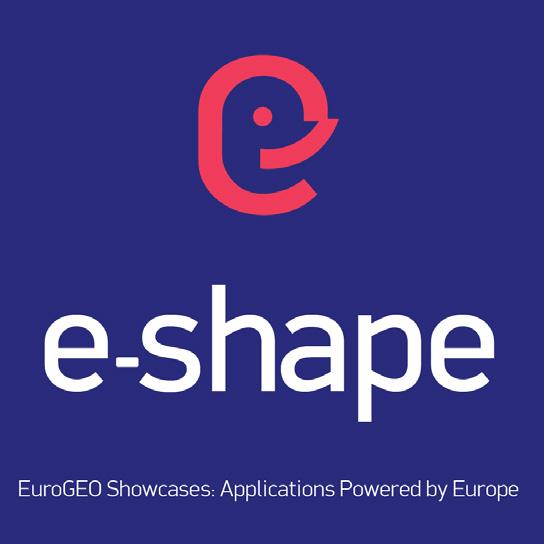
ANDREA VAJDA, OTTO HYVÄRINEN, MIKA RANTANEN, ANDREAS TACK, MARKUS SILVENNOINEN Finnish Meteorological Institute According to the legislation, winter tyres must be used in Finland from 1 November to 31 March if the weather and road surface conditions require (Traficom 2020). Thus, the time of win ter tyre installation and distribution to the customers varies from year to year in conformity with the weather condi tions. In this paper, we present a new user-oriented climate service provid ing tailored sub-seasonal to seasonal predictions for tyre companies, devel oped within the H2020 e-shape pro ject, Seasonal preparedness pilot. The service was co-created with the tyre and car service chain, Vianor Oy. The predictions provided via the sub-sea sonal and seasonal climate outlooks for the upcoming six weeks and three months, respectively, allow tyre com panies to plan the distribution of right tyre types, the seasonal management, and to provide relevant information for their customers about the time of tyre change. The climate service is currently in pilot phase, running in a fully automated operational mode. Development of the sub-season al and seasonal climate outlooks. An iterative strategy was followed in the creation of climate outlooks. The end-user, Vianor, was engaged through dialog from the beginning of the design and development process to harmonize their needs in terms of forecast products, visualization and delivery method. Information about the skill of forecasts and uncertainties were also discussed. A set of sub-sea sonal and seasonal forecast products (Table 1) relevant for winter driving safety were selected and designed with the user. For the onset and off set of winter tyre season outlook the thresholds for slippery conditions, hazarding driving safety, were deter mined based on literature survey and on analysis of road accident data from Finland (Finnish Crash Data Institute, OTI) against weather variables for the periodData2005–2018.fromthestate-of-the-art pre diction systems from the European Centre for Medium-Range Weather Forecasts (ECMWF), i.e., sub-season al forecasts from the ensemble pre diction system (EPS) (ECMWF 2016) and seasonal forecasts from SEAS5 system (Johnson et al. 2019) were used in the development and oper ational runs of the forecast products.
FMI’S CLIMATE BULLETIN: RESEARCH LETTERS 1/2022 | 5
In winter, driving safety is strongly weather dependent. Timely installation of winter tyres is essential when temperature falls to near 0 °C and the surface of the roads becomes slippery due to ice and snow. A new user-oriented climate service providing tailored sub-seasonal and seasonal climate outlooks for winter tyre season and safety driving conditions was developed and piloted by the Finnish Meteorological Institute and Vianor Oy.
25 Jan. 2022, first online 03 Feb. 2022, published 02 Sep. 2022
All the variables were bias adjusted by the first pilot season (winter 2020–2021) except the sub-seasonal snow depth, which was adjusted by the start of the pilot season 2021–2022. After the post-processing of the in put variables, the outlooks were com puted. The winter tyre season, prob ability of snow cover and freezing temperature outlooks describe the likelihood of expected climate con ditions through begins after the new
The skill of variables used in the de velopment of outlooks was assessed and systematic errors from the raw data were reduced through bias ad justment to improve the quality of the forecasts. The variables were eval uated and calibrated using re-fore cast data for the period 2000–2019 and ERA5 reanalysis (Hersbach et al. 2020) as reference for the same pe riod. The quality of the sub-seasonal variables was successfully improved by applying the mean bias removal method for 2m temperature and the quantile mapping method for snow depth (Fig. 1). In case of seasonal fore casts, the biases of 2m temperature and snow depth variables were re duced by ensemble model output sta tistics (EMOS) (Gneiting et al. 2005).
The EPS data is produced for 46 days ahead twice a week, on Mondays and Thursdays, with 0.4° spatial resolution and is accessed through the ECMWF dissemination. SEAS5 system data is accessed from the Copernicus C3S Climate Data Store, where it is avail able with a horizontal resolution of 1° and updated on 13th of each month.
Seasonal preparedness pilot – sub-seasonal and seasonal prediction service for tyreReceivedcompanies29Nov.2021,accepted
DOI: 10.35614/ISSN-2341-6408-IK-2022-02-RL
TIME-SCALE CLIMATE OUTLOOKS WEATHER CONDITIONS DESCRIBED VARIABLES USED IN THE PRODUCT DEVELOPMENT METHODOLOGY AND THRESHOLD APPLIED
2 m air temperature Tmin > 0 °C for 7 con secutive days
Probability of snow cover Monthly probability of snow cover on the ground SEAS5 snow depth, snow density Snow depth ≥ 1 cm
Monthly probability of freezing temperature SEAS5 2 m air tempe rature Tmean < 0 °C CLIMATE BULLETIN: RESEARCH LETTERS 1/2022 probability forecast, while the snow depth outlook predicts the absolute value of snow accumulation. Production of sub-seasonal out looks. The newly developed sub-sea sonal outlooks are produced in oper ational runs on the FMI servers using the improved version of the opera tional service prototype developed in the CLIPS project (Ervasti et al. 2018) produce sub-seasonal climate out looks (clips.fmi.fi). The production run
Weekly probability of slippery road conditions during the fo recast week in autumn
outlookSub-seasonal
Weekly probability of freezing temperature 2 m air temperature Tmean < 0 °C
FIG 1: Root mean square error calculated for sub-seasonal raw model, bias adjusted snow depth and observed snow climatology (2000–2019) for two locations.
Probability of freezing temperatures
Weekly probability of non-slip pery road conditions during the forecast week in spring
Probability of freezing temperatures
Seasonal climate outlook
Probability of snow cover Weekly probability of snow cover on the ground Snow depth, snow density Snow depth ≥ 1 cm Snow depth Weekly average snow depth Snow depth, snow density Weekly average snow depth
TABLE 1: The climate outlooks co-designed and developed including the definitions and variables used.
Winter tyre season
2 m air temperature Tmin < 0 °C for 2 con secutive nights
6 | FMI’S
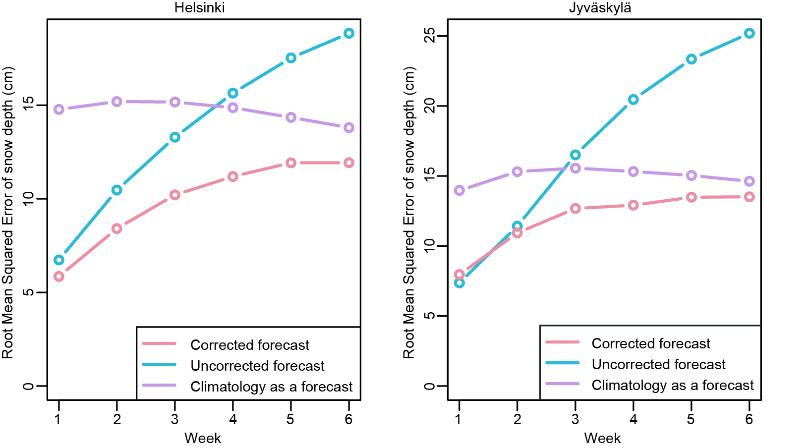
Acknowledgements: The study contributes to the H2020 e-shape pro ject (grant 820852) funded by the Eu ropean Commission. The first version of the operational service prototype used in the production of sub-sea sonal outlooks was developed in the SA CLIPS project (clips.fmi.fi) funded by the Academy of Finland (grant no. 303951). We thank the Finnish Crash Data Institute (OTI) for the road acci dent data provided.
The first pilot season was run dur ing winter 2021–2022. After the first test season a preliminary qualitative evaluation of the forecast products was performed. Since the service wasn’t included yet to the workflow of the user during the first pilot season, their feedback on the forecast prod ucts will be received after the sec ond pilot season. Based on the user’s feedback and the results of qualitative evaluation of the forecast products, the outlooks will be further improved to assure their usability.
This new climate service showcases the applicability of sub-seasonal and seasonal predictions tailored to sup port decision taking in transportation sector, allowing end-users to increase their preparedness and resilience to inter-annual climate variability.
Finnish Transport and Communication Agency (TRAFICOM), 2020.: Winter tyres. Accessed 6 September 2020, https://www.traficom.fi/en/tran Gneiting,sport/road/winter-tyresT.,A.E.Raftery, A. H. Westveld III, and T. Goldman, 2005: Calibrated Probabilistic Forecasting Using Ensemble Model Output Statistics and Minimum CRPS Estimation. Mon. Wea. Rev., 133, 1098–1118, https://doi.org/10.1175/MWR2904.1 Hersbach, H., and Coauthors, 2020: The ERA5 Global Reanalysis. Q. J. R. Meteorol. Soc., 146, 1999–2049, https://doi.org/10.1002/qj.3803
2018: Mapping users’ expectations regarding extended-range forecasts. Adv. Sci. Res., 15, 99–106, https://doi.org/10.5194/asr-15-99-2018
Johnson, S. J., and Coauthors, 2019: SEAS5: The new ECMWF seasonal forecast system. Geosci. Model Dev., 12, 1087–1117, https://doi.org/10.5194/
FMI’S CLIMATE BULLETIN: RESEARCH LETTERS 1/2022 | 7 ECMWF, 2016: IFS documentation, CY43R2, Part V: Ensemble Prediction System. 23 pp, https://www.ecmwf.int/sites/default/files/elibra Ervasti,ry/2016/17118-part-v-ensemble-prediction-system.pdfT.,H.Gregow,A.Vajda,T.K.Laurila,andA.Mäkelä,
FIG 2: An example of the winter tyre season outlook animation issued on 19.11.2020.
forecastgmd-12-1087-2019isdisseminated to FMI. The variables extracted from the dissemi nation stream are post-processed and the climate outlooks are generated. The output data is visualized through weekly maps presenting the predicted weather conditions for each forecast product and disseminated to the us ers through an online user interface, the Ilmanet service platform (ilmanet. fi). The users can access the service using credentials. A user-friendly, eas ily understandable and interpretable format and easily accessible system were the main aspects when imple menting the outlooks and the ser vice. Each outlook consists of (1) an informational page including a short description of the forecast product and its definitions, information on the computation of the product and ad vice on how to interpret the forecast; (2) six static weekly maps presenting the predicted weather conditions ei ther through probabilities or absolute values, and (3) animated version of the weekly maps (Fig. 2). The sub-season al outlooks are updated twice a week, on Tuesday and Friday. In September 2020, the first version of the service was set up and the winter tyre season outlook was released. The other out looks were added in December 2020. Production of seasonal climate outlooks. The seasonal climate out looks are produced on WEkEO, a Co pernicus DIAS services as soon as the updated seasonal forecast products from C3S CDS are shared on WEkEO. The forecast products are visualized through maps that are disseminated to the users through the seasonal.fmi.fi web-portal. The outlooks are updated on the 13th of each month. The pro duction of seasonal climate outlooks was deployed in January 2021.
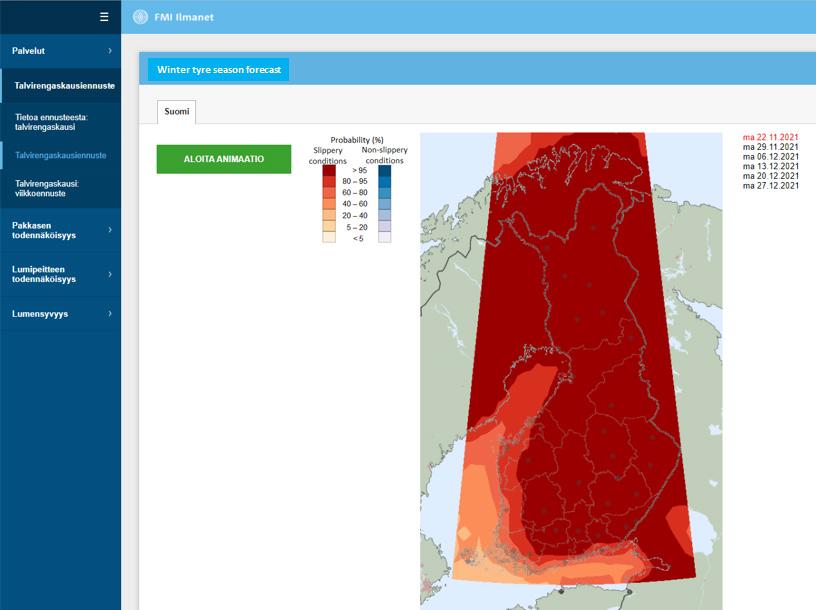
STAVROS SOLOMOS¹, LIDA DIMITRIADOU¹, JOHN KAPSOMENAKIS¹, IOANNIS BINIETOGLOU², PANAGIOTIS NASTOS1,3, CHRIS TOS ZEREFOS1,4,5,6
8 | FMI’S CLIMATE BULLETIN: RESEARCH LETTERS 1/2022
1Research Centre for Atmospheric Physics and Climatology, Academy of Athens, Athens, Greece ²IAASARS, National Observatory of Athens, Athens, Greece ³Laboratory of Climatology and Atmospheric Environment, Department of Geology and Geoenvironment, National and Kapo distrian University of Athens, Athens,Greece ⁴Biomedical Research Foundation, Academy of Athens, Athens, Greece ⁵Navarino Environmental Observatory (N.E.O.), Messinia, Greece
⁶Mariolopoulos-Kanaginis Foundation for the Environmental Sciences, Athens, Greece
17 Feb. 2022, first online 18 Feb. 2022, published 02 Sep. 2022
“CRITERION” for the touristic sector in
Development of the weather and climate service
We present the methodological development steps as well as first results of the e-shape service CRITERION. The service is based on Copernicus ERA5 data for climatological information, the Copernicus C3S 50-member ensemble for the seasonal forecasting and on WRF local scale weather forecasts at 4×4 km over Greece. Detailed information is provided at 20 UNESCO cultural heritage sites in Greece in the form of simple symbols and charts. The service has been developed by the Research Center for Atmospheric Physics and Climatology at the Academy of Athens and is operational since April 2021 at https://unesco-weather.gr/.
DOI: 10.35614/ISSN-2341-6408-IK-2022-03-RL
Receivede-shape9Nov.2021,accepted
Introduction. Weather and climate information is necessary for deci sion-making for a range of tourism stakeholders. The decision to make a trip is greatly influenced by the phys ical and financial capabilities of the individual as well as by two important parameters: the individual’s desires that are formed based on the climat ic conditions prevailing in the place where she/he resides and the char acteristics of the desired destination, such as the natural landscape, cultural features and climate that make it at tractive. A typical example is the pref erence of the British for destinations abroad with milder climates (Amelung et al. 2007). Furthermore, the generic climatic conditions which would at tract tourists may differ substantially from the actual prevailing conditions during their visit. Greece offers many options on which places to visit dur ing a specific time schedule. Even the same site can offer a completely dif ferent experience when visited at dif ferent seasons. For these reasons we decided to develop a tailored weath er and climate forecasting tool in the frame of e-shape, focusing on 20 sites in Greece that have been declared by UNESCO as World Cultural Heritage sites (Fig. 1a). Service Description and co-design. The service is named “CRITERI ON” since its purpose is to provide an additional climate-relevant criterion for stakeholders in the touristic sec tor as well as to individual visitors for planning their vacations and trips. It is developed by the Research Center for Atmospheric Physics and Climatology at the Academy of Athens and co-de signed with the Institute of Greek tourism confederation for the final format of the web page and user op tions. Co-design was mainly focused towards three directions: i) Identify the locations for the pilot service; ii) Define the requested timescales for the forecasts and iii) Design the web page. An iterative process was estab lished with the end-user and through a series of discussions we came up with the idea to start from the em blematic UNESCO cultural heritage sites that are known worldwide and could provide a testbed for our de velopments. The requested forecast ing window was three months for the
FIG 1: a) UNESCO cultural heritage sites in Greece, b) CRITERION service methodological outline. emy of Athens and is composed of three individual pillars as seen in Fig. 1b. First the local monthly climatology for each site is derived from the ERA5 dataset (Hersbach et al. 2020) for the period 2005–2019, including temper ature, relative humidity, daily precipi tation, and wind speed. These values are used to provide the prevailing cli matological conditions at each site. This information is accompanied with a short description of each monument and can be used as a first step for de ciding the visiting locations. The sea sonal forecast is derived from the 51 ECMWF System 5 ensemble members (Stockdale et al. 2018) at single at mospheric levels, interpolated at each site. The ensemble forecasts are used without bias correction. The seasonal
FIG 2: a) Example of local climatological values and seasonal forecast for the Acropolis of Athens, b) example of local weather forecast for the Temple of Apollo Epicurius in Peloponnese. Daily
seasonal forecast and four days for the short-range forecasts to facilitate both long-term and daily vacation planning. The final web page struc ture was also co-designed to include a simple front page with minimal in formation and atmospheric symbols along with more advanced timeseries for the interested user. The service is hosted at the Acad
precipitationMonth(mm)Precipitation FMI’S CLIMATE BULLETIN: RESEARCH LETTERS 1/2022 | 9
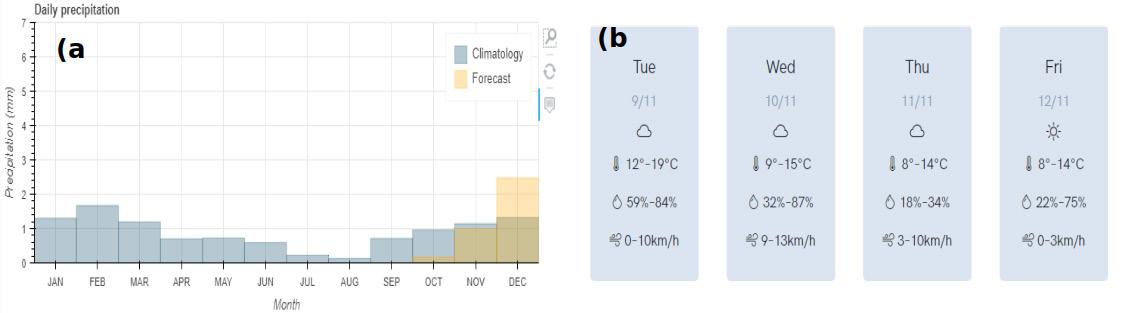
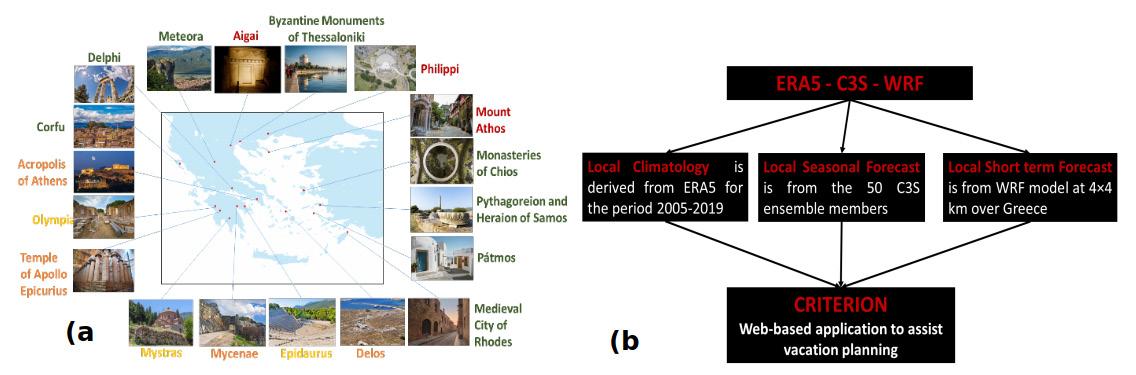
forecast is provided for three months ahead and is given along with the climatological values of each site, to provide a measure of possible devia tion of certain atmospheric variables from their average values (Fig. 2a). Finally, the 4-day forecast is provided at each one of the CRITERION sites, based on WRF-ARW v4.1.3 model (Skamarock et al. 2019) at 4 × 4 km grid space over Greece. Such high resolution is required for the com plex mountainous terrain of Greece in order to resolve local scale flows (e.g., downslopes, breezes, convec tive storms) and site-specific weather conditions (Solomos et al. 2021). The entire CRITERION process is fully au tomatic and is operational at the su percomputing cluster at the Academy of Athens. The development is mainly done in Bash shell scripting, Python scripting and Fortran environments. Potential impact of the service. CRITERION provides a simple inter face that can be easily adopted by tourism operators such as touristic agents, hotels, Airbnb apartments etc. The service is available at https://un esco-weather.gr/ and the web page provides easy navigation and both simple and detailed information types for the interested visitor (Fig. 2a, b).
Acknowledgements. The study contributes to e-shape project that is funded from the European Union’s Horizon 2020 research and innovation programme under grant agreement 820852.
Amelung, B., S. Nicholls and D. Viner, 2007: Implications of Global Climate Change for Tourism Flows and Seasonality. J. Travel Res., 45, 285–296, Hersbach,https://doi.org/10.1177%2F0047287506295937H.andCoauthors,2020:TheERA5global reanalysis. Quart. J. Roy. Meteor. Soc., 146, 1999–2049, https://doi.org/10.1002/qj.3803 Skamarock, W.C. and Coauthors, 2019: A Description of the Advanced Research WRF Model Version 4. NCAR Tech. Note NCAR/TN-475+STR, 145 pp., Solomos,http://dx.doi.org/10.5065/1dfh-6p97S.,P.T.Nastos,D.Emmanouloudis, A. Koutsouraki and C. Zerefos, 2021: A Modeling Study on the Downslope Wind of “Katevatos” in Gree ce and Implications for the Battle of Arachova in 1826. Atmosphere, 12, 993, https://doi.org/10.3390/atmos12080993 Stockdale T., S. Johnson, L. Ferranti, M. Balmaseda and S. Briceag, 2018: ECMWF’s new long-range forecasting system SEAS5. ECMWF Newsletter, No. 154 – Winter 2017/18, 15-20, http://dx.doi.org/10.21957/tsb6n1
The developed modules can be easi ly expanded upon request to include also more sites in Greece. Application to other countries is also feasible and can be co-designed with potential end users to fulfil their specific needs.
10 | FMI’S CLIMATE BULLETIN: RESEARCH LETTERS 1/2022
Urban resilience to extreme weather - subseasonal and seasonal forecasts for winter maintenance activities in Helsinki
FMI’S 1/2022 DOI: 10.35614/ISSN-2341-6408-IK-2022-04-RL
• Longer periods of warm weather during winter (sub-seasonal scale)
• Snow accumulation (sub-season al and seasonal scale)
CLIMATE BULLETIN: RESEARCH LETTERS
| 11
• Slippery conditions for pedestri ans (sub-seasonal scale)
Received 29 Nov. 2021, accepted 23 Mar. 2022, first online 23 Mar. 2022, published 02 Sep. 2022
• Conditions for spring street clean ing (sub-seasonal scale). Some potential products were exclud ed due to the low skill of the variables, for example, forecasts of snow melt. The forecast data used in the de velopment of the sub-seasonal and seasonal climate outlooks are the ex tended-range forecast dataset pro vided by the ensemble prediction system of ECMWF (ECMWF 2016) and the SEAS5 long-range forecast ing system of ECMWF (Johnson et al. 2019) available from Copernicus CDS. Sub-seasonal products are processed
Sub-seasonal and seasonal climate outlooks for winter street maintenance were developed and piloted by the Finnish Meteorological Institute in co-operation with the City of Helsinki.
OTTO HYVÄRINEN, ANDREA VAJDA, MIKA RANTANEN, ANDREAS TACK, MARKUS SILVENNOINEN Finnish Meteorological Institute In Helsinki, there are on average 95 days per year with snow cover (based on the observation period 2000–2019). However, winter conditions vary considerably from year to year. The City of Helsinki is responsible for the maintenance of streets in Helsinki. Winter street maintenance includes snow ploughing, the prevention of slipperiness by sanding, and street cleaning. Street cleaning includes sweeping up the sand and other grit accumulated over the winter months on the roadways, sidewalks and at public transportation stops, and is especially important in spring, after the snow season. Planning of these maintenance activities are currently done based on the short-term weath er forecasts provided by the Finnish Meteorological Institute and from experience gained from the previous years. Within the H2020 e-shape pro ject, under “S7-P2-Urban resilience to extreme weather”, a climate service providing sub-seasonal and seasonal climate outlooks tailored for winter street maintenance was developed in co-operation between the Finnish Meteorological Institute (FMI) and the City of Helsinki. Providing user-ori ented sub-seasonal and seasonal forecast products tailored for win ter maintenance activities serves city authorities in planning activities, im proving winter safety, and optimizing maintenance costs. The co-design and technical implementation. From the beginning, the City of Helsinki was engaged in the co-design and development process. Face-to-face and web-meetings were organized with the representatives of the Urban Environment Division of City of Helsinki. During the joint sessions, the potential offered by the sub-seasonal and seasonal forecasts for city management were discussed, forecast skill and uncertainties related to variables used in the development of outlooks were presented, and the most relevant sub-seasonal and sea sonal forecast products were select ed. Finally, the visualization and de livery modalities for the six-week and monthly outlooks were agreed. Initially, the plan was to solely use seasonal-range forecasts. However, it became clear that the sub-seasonal forecast time scale is more relevant for maintenance work planning as most of the activities are scheduled on timescales of several weeks. In formation on snow depth is needed also on the seasonal time scale for the preparation of snow dump sites and haulage. In the end, the following tai lored sub-seasonal and seasonal fore cast products were selected in the co-design development process for testing. These outlooks (see Table 1 for detailed definitions) were defined and designed in collaboration with the users, based on the current mainte nance practices and additional infor mation from literature:
• Longer periods of freezing weath er during winter (sub-seasonal scale)
2 m temperature, snow depth Tmin > 0 °C and snow < 1 cm at FMI and disseminated through the Ilmatie/Ilmanet platform developed within FMI. The seasonal product, snow accumulation outlook, is pro cessed in WEkEO, a Copernicus DIAS service and disseminated through the
outlook(six-week)onalSub-seas
2 m temperature Tmean < 0 °C during > 90% of a 7-day period
2 m temperature, total precipitation, snow depth Tmin = -2…2°C and Prec >0 mm OR Snow = 1 cm
FIG 1: Examples of winter preparedness outlooks for the City of Helsinki: (a) weekly snow accumulation issued on 15 Nov 2021 on the Ilmatie portal, (b) monthly snow accumulation outlook published on the seasonal.fmi.fi portal on 17 Nov 2021. 12
VARIABLES USED IN THE PRO DUCT DEVELOPMENT METHODOLOGY AND THRESHOLD APPLIED
2 m temperature Tmean > 0 °C during > 90% of a 7-day period
Snow accumulation
Probability of weekly ave rage snow depth Snow depth, snow density Weekly average snow depth
Long periods of freezing and mild weather
| FMI’S CLIMATE BULLETIN: RESEARCH LETTERS 1/2022
Slippery conditions for pedestrians Weather conditions fa vorable for development of slippery conditions on sidewalks
Conditions for spring street cleaning Non-slippery weather conditions when grit is not needed anymore on the streets
TIME-SCALE OUTLOOKS WEATHER DESCRIBEDCONDITIONS
Mild weather: 7 consecutive days with warmer weather conditions
Freezing weather: 7 con secutive days with freezing weather conditions
TABLE 1: The winter preparedness outlooks developed for and with the City of Helsinki. seasonal.fmi.fi web portal. Examples of weekly and monthly snow depth outlooks are presented in Fig. 1. The service was deployed, and the first weekly outlooks published in Decem ber 2020. The project timeline is divid ed into two Sprints, and Sprint 1 has been completed at the time of writing. During Sprint 1, sub-seasonal temper atures and seasonal snow accumu lation have been bias-adjusted. The bias adjustment of sub-seasonal snow
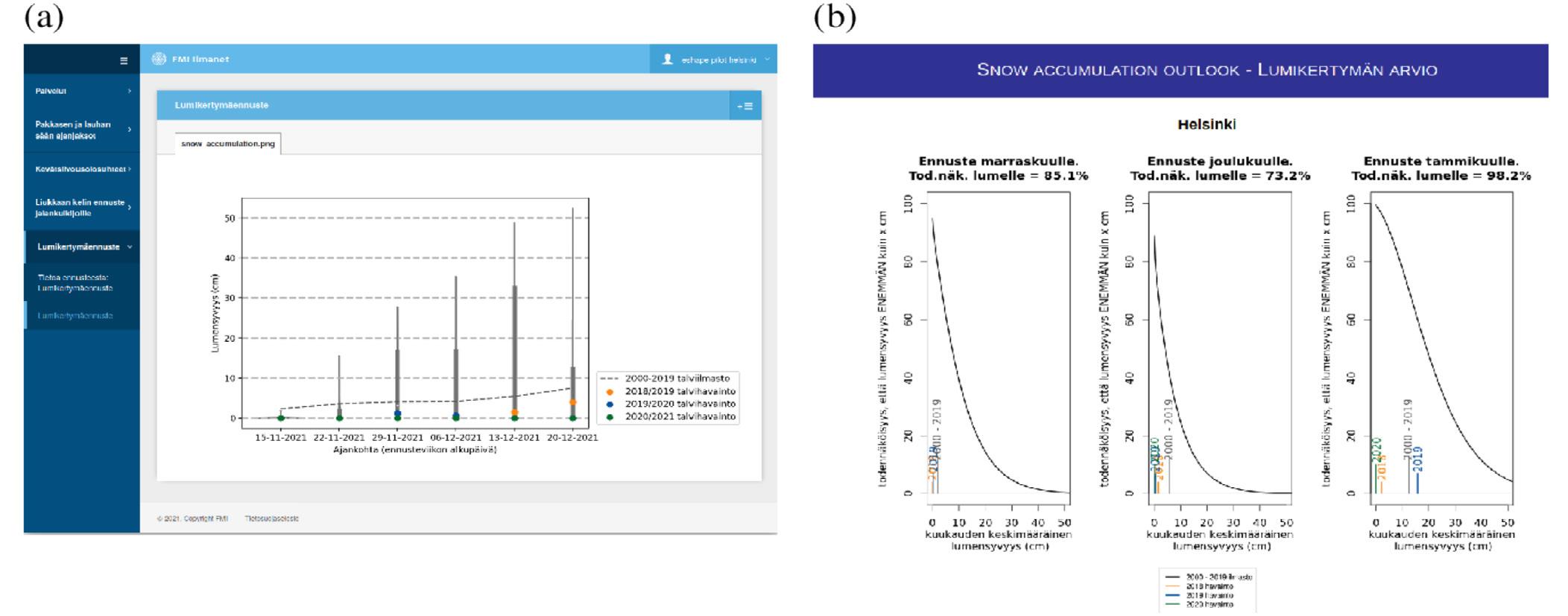
ECMWF, 2016: IFS documentation CY43R2 - Part V: Ensemble Prediction System. 23 pp, https://www.ecmwf.int/node/17118
Johnson, S. J., and Coauthors, 2019: SEAS5: The new ECMWF seasonal forecast system. Geosci. Model Dev., 12, 1087–1117, https://doi.org/10.5194/ Rantanen,gmd-12-1087-2019M.,M.Kämäräinen, O. Hyvärinen, and A. Vajda, 2021: Skill assessment of sub-seasonal 2-m temperature forecasts for Helsinki, Finland. EMS Annual Meeting 2021, online, 6–10 Sep 2021, EMS2021-138, https://doi.org/10.5194/ems2021-138 Vajda, A., O. Hyvärinen, M. Rantanen, A. Tack, and M. Silvennoinen, 2021: Seasonal preparedness pilot – sub-seasonal and seasonal service for tyre companies. FMI’s Clim. Bull. Res. Lett., 4(1), 5-7, https://doi.org/10.35614/ISSN-2341-6408-IK-2022-02-RL and precipitation will be explored in Sprint 2. Thus, the products are con tinuously improved in its usability. The feedback on the outlooks. The feedback from the City of Helsinki was gathered after the first pilot sea son 2020–2021. Outlooks for snow ac cumulation, and for longer periods of freezing or mild weather during win ter were found the most useful. The outlook of conditions for spring street cleaning was consulted, but often the sand was removed from the street before the product indicated suitable conditions. The forecast of periods of mild weather was more useful for this task. Moreover, the snow condition threshold in the spring street clean ing product (less than 1 cm of snow) proved to be too strict, as the sand can be removed even if some snow is present. For the user it is more impor tant to know if new snow is expected in the near future. The outlooks for slippery condi tions were used as background infor mation. However, as decisions to sand or not to sand are made on a daily basis, its benefit is still unclear. More over, it is also unclear if slippery con ditions can be forecasted using the simple rule-based methodology used here or if more advanced methods should be explored in the future. The outlooks have been updated consid ering the feedback received from the user and will be tested during winter 2021–2022. The seasonal outlook of snow accumulation became available late in the season, so it will be tested during Sprint 2. The City of Helsinki also expressed a wish for the quantitative validation of outlooks. This is planned for the near future. Some early results for sub-sea sonal snow depth and 2 m tempera ture validation have been presented in Rantanen et al. (2021) and Vajda et al. (2021). A quantitative evaluation of the sub-seasonal forecast products will be performed following the sec ond testing period, when a larger data pool will be available. Acknowledgements. The study contributes to the H2020 e-shape project (grant 820852) funded by the European Commission.
FMI’S CLIMATE BULLETIN: RESEARCH LETTERS 1/2022 | 13
Background. The Finnish forestry sec tor traditionally plays a significant role for the national economy. More than 75 % of Finland is covered with for ests, which leads to the wood produc tion being responsible. More than 75 % of Finland is covered with forests, which leads to the wood production being responsible for 1/5 of Finland’s export market. Approximately 2.7 bil lion euros from the forestry sector is an important part of Finland’s annual tax revenue (Finnish Forest Industries 2021). Commercial timber harvesting in Finland takes place mechanically with wheel-based forest machines. The machines used by forest machine contractors and operators need good load-bearing conditions for their op erations due to their heavy weight. A typical harvester chain consists of a harvester and a forwarder, of which the forwarder can weigh almost 40 tons when fully loaded (Fig. 1). According to the Finnish Forest Act on forestry, wild species, and eco systems, the “damage to the terrain which may weaken the growing con ditions for the tree stand must … be avoided” (Ministry of Agriculture and Forestry 2014). Especially the num ber of deep ruts greater than 10 cm caused by heavy machinery has to be kept minimal. On surfaces with low soil bearing capacity, heavy harvest ing machines can cause damage and prevent adequate regeneration of the ground.Finnish forest can be classified into areas of good harvestability, depend ing on annual season, weather condi tion and soil condition. Based on ALS Airborne Laser Scanning via LIDAR measurements and a digital elevation model, Metsäkeskus (Finnish Forest Centre) is providing static trafficabil ity mosaics for Finland (Metsäkeskus, 2021). Those maps are differentiating between mineral soil and peatlands and classifying whether those sur faces have sufficient soil bearing ca pacity. Classes are color-coded from green to red, thus representing the trafficability on different soil condi tions (Fig. 2, right) and seasons. In Finland, the share of peatlands from which wood can be harvested only in winter is relatively high. Within the re cent years good winters for harvest ing have decreased. Warmer winter
MIRIAM KOSMALE1, JAAKKO IKONEN1, MIKKO MOISANDER1, TUOMO SMOLANDER1, HEIKKI OVASKAINEN2, ASKO POIKELA2, MIKKO STRAHLENDORFF1
Harvester Seasons – a forestry service supporting climate smart operations
14 | FMI’S CLIMATE BULLETIN: RESEARCH LETTERS 1/2022 DOI: The10.35614/ISSN-2341-6408-IK-2022-05-RLFinnishMeteorologicalInstitute
2Metsäteho Oy
FIG 1: Heavy and middle-sized forwarder operating on peatland. Received 14 Dec. 2021, accepted 12 Apr. 2022, first online 11 May 2022, published 02 Sep. 2022
has developed Harvester Seasons, a web-based service for the forestry sector aiming to pave the way for climate smart operations. This service is supporting the forestry sector by offering a dedicated trafficability and soil information service based on weather and seasonal forecast models. This service leverages the European efforts on climate monitoring by linking Copernicus DIAS platforms, the Copernicus Climate Data Store, and Copernicus seasonal forecasts with climate data records.
1Finnish Meteorological Institute
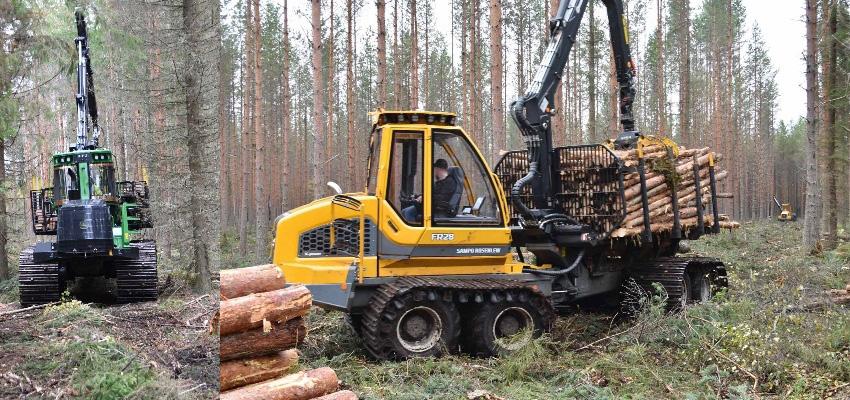
Harvester Seasons (https://har vesterseasons.com) is a web applica tion developed by the Finnish Mete orological Institute that supports the Finnish forestry sector by offering dedicated trafficability and soil infor mation system.
FMI’S CLIMATE BULLETIN: RESEARCH LETTERS 1/2022 | 15 like in 2019/2020 (Lehtonen 2021) made it necessary to adapt harvesting operation planning. The challenge in timber harvesting is to optimize the operation planning according to actu al carrying capacity of the ground to avoid significant shortfalls in produc tion. Smart operation planning does not only have monetary effect but also help protecting our forest envi ronment and ecosystem.
6 month forecast on trafficability starting from selected date is given as time series. Shortterm forecast (red in time series) is based on FMI 10-day weather forecast information. produces monthly updates of dai ly seasonal weather forecasts for 6 months ahead (Copernicus 2021a) as well as grid-based reanalysis data by ERA5-Land (Muñoz Sabater 2019). By bias-adjusting this seasonal weather forecast with higher resolved climate statistics of ERA5-Land, it is possible to ingest 51 highly resolved ensemble forecasts into the Harvester Seasons service backend. Trafficability infor mation is then derived by combining statistically model forecasts of soil pa rameters and seasonal forecast data with the lidar based static surface classification datasets. For the shortterm forecast FMI’s 10-day weather forecast information is used for ser vice prediction of trafficability. In col laboration with the experts from the forestry sector and based on works of Sirén et al. (2019) and Bergqvist et al. (2018), thresholds for hydrolog ical and meteorological conditions were set in order to derive a dynam ic classification (Fig. 2, right). Even though long-term seasonal forecasts up to half a year are still challenging (Hyvärinen et al. 2020), the approach of dynamic trafficability indices helps the forestry sector in climate smart operationWEkEOplanning.isoneof the 5 EU Coper nicus DIAS reference services for en vironmental data, virtual processing environments and skilled user support (Copernicus 2021b). WEkEO has di rect access to the Climate Data Store, which leverage C3S data for Harvest er Seasons. Backend and frontend of the Harvester Seasons service is completely implemented within the WEkEO virtual machines. Co-design and user feedback. Within the funding frameworks of Copernicus Programme’s Climate Change Service (C3S) as well as the e-shape project, the primary focus of this service is on direct user interac tion. As co-design partners FMI had Metsäteho and with its customers and clients, direct access to feedback from the forestry sector. Metsäteho’s share holders are all large Finnish forest companies (Metsä Group, Stora Enso, UPM) and state forests (Metsähallitus),
Copernicus, the backbone for Harvester Seasons. Copernicus, Europe’s eyes on Earth, offers access to global and local data from satellites, groundbased measurements, or model data. Harvester Seasons input data stream is built upon this backbone of Coper nicusCopernicusdata. Climate Change Ser vice (C3S), implemented by ECMWF, FIG 2: Screenshot of trafficability provided by Harvester Seasons web application from location near Lappeenranta (61.25331N,27.97171E).
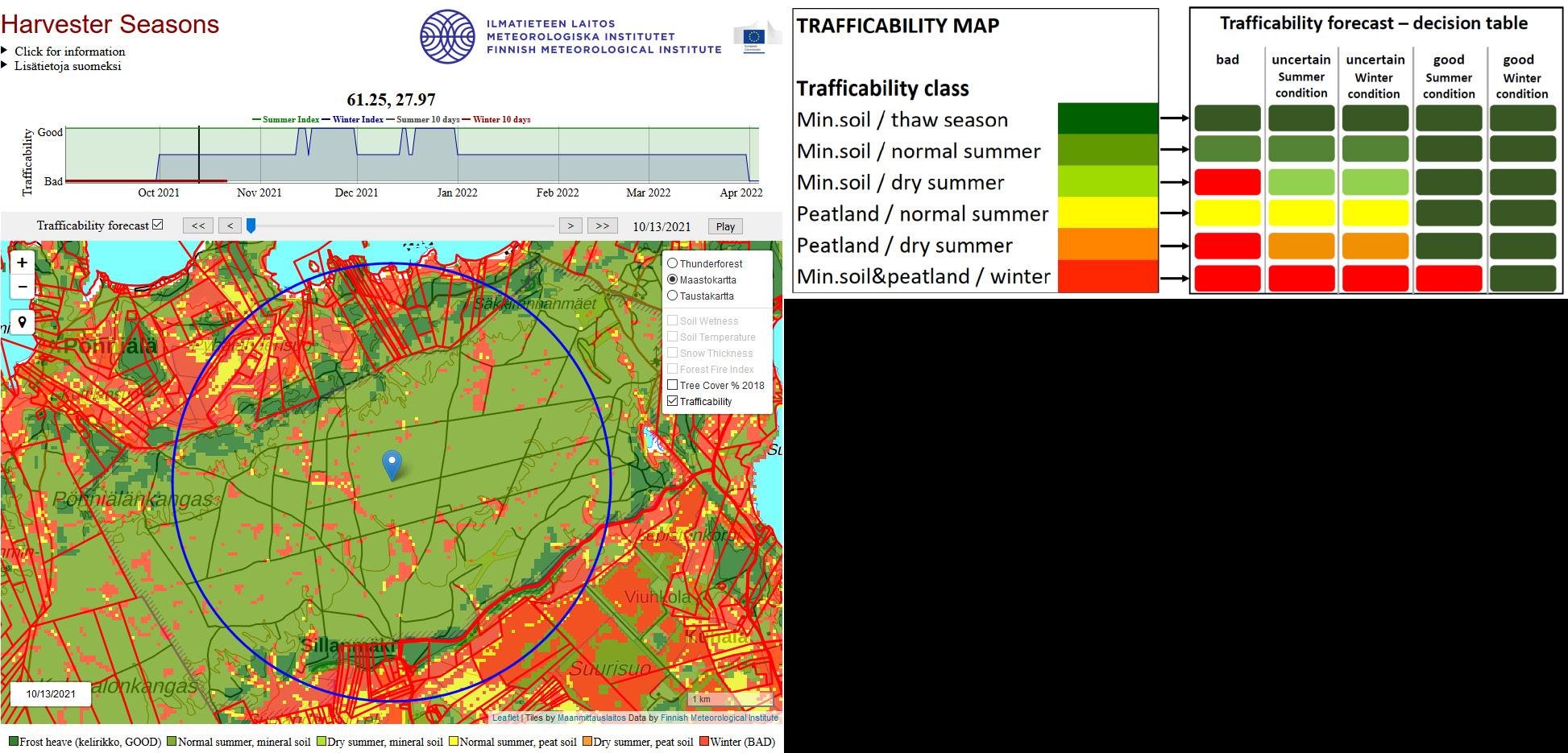
Accessed 14 December 2021, https://www.metsakeskus.fi/fi/avoin-metsa-ja-luontotieto/metsatietoaineistot/ MinistrykorjuukelpoisuusofAgriculture and Forestry, 2014: Forest Act (1093/1996; amendments up to 567/2014 included), 16 pp, Muñozhttps://www.finlex.fi/en/laki/kaannokset/1996/en19961093_20140567.pdfSabater,J.,2019:ERA5-Landhourlydatafrom1981topresent.Copernicus Climate Change Service (C3S) Climate Data Store (CDS). Acces sed 14 Dec 2021, http://doi.org/10.24381/cds.e2161bac Sirén, M., A. Salmivaara, J. Ala-Ilomäki, S. Launiainen, H. Lindeman, J. Uusitalo, R. Sutinen, and P. Hänninen, 2019: Predicting forwarder rut formation on fine-grained mineral soils. Scandinavian J. Forest Res., 34, 145-154, https://doi.org/10.1080/02827581.2018.1562567 Vajda, A., A. Venäläinen, I. Suomi, P. Junnila, and H. Mäkelä, 2014: Assessment of forest fire danger in a boreal forest environment: description and evaluation of the operational system applied in Finland. Met. Apps., 21: 879-887. https://doi.org/10.1002/met.1425 for which private harvesting contrac tors offer harvesting services. Service developers implemented user requirements iteratively. These requirements were, e.g., an easily un derstandable map layer, index-based trafficability information (good vs bad) and the necessity of direct API access for user interfaces. Color-cod ing for the dynamic weather depen dent trafficability has been estab lished in a similar way to the already existing static trafficability maps. This helps users to easily grasp the new service information by its well-estab lished look (Fig. 2). Foremen of har vesting machines as well as operation planners require condensed informa tion for it to have a real added value for their daily work. As a special request by stakehold ers, selected land cover characteris tics (Copernicus 2021c) like tree cov er density and FMI’s forest fire index are included in the service. Harvesting operations with machines equipped with steel tracks increases the risk for light fires within forests. In Finland authorities give warnings during high forest fire risk and harvesting opera tion activities should be suspended. The forest fire warning system is de signed to inform the general public and the fire authorities about forest fire risk caused by dry terrain (Vajda et al. 2014).
16 | FMI’S CLIMATE BULLETIN: RESEARCH LETTERS 1/2022
Bergqvist, I., E. Willén, J. Peuhkurinen, K. Väätäinen, H. Lindeman, A. Laurén, and A. Poikela, 2017: Forest trafficability maps – data sources and methods. EFFORTE Deliverable 3.3, 15 Hyvärinenfinnish-forest-industryFinnishCopernicus,Copernicus,Copernicus,https://projects.luke.fi/efforte/wp-content/uploads/sites/14/2017/09/EFFORTE-D3.3-Forest-trafficability-maps-data-sources-and-methods.pdfpp,2021a:Seasonalforecasts.https://climate.copernicus.eu/seasonal-forecasts2021b:WekEO–CopernicusandSentineldataatyourfingertips-DataandInformationAccessServices(DIAS),https://wekeo.eu2021c:LandMonitoringservice:Landcovercharacteristics;https://land.copernicus.euForestIndustries,2021:Futuregrowthandsustainablewelfare.Accessed14December2021,https://www.metsateollisuus.fi/en/facts-about-O.,A.Venäläinen,andA.Vajda,2020:Bias-adjustedseasonalforecastsofsoilmoistureforforestryapplicationsinFinland. Adv. Sci. Res., 17, 23-27, Lehtonen,https://doi.org/10.5194/asr-17-23-2020I.,2021:Recordmildwinterof2019/2020
Summary. The Harvester Seasons service has been launched for a great er Finland domain in May 2020. The web application is focusing on traf ficability to support planning of har vesting operation and fostering Earth Observation and model prediction data in business operations. Having direct access to Copernicus climate data records and having them trans lated to a tailored information content bridges the gap between science and community. Service Info. The service is acces sible at https://harvesterseasons.com Find updated info on LinkedIn. A video on how to use the service is available on Youtube Acknowledgements. This service was developed as a use case by the Copernicus Programme’s Climate Change Service (C3S) contract un der framework agreement tions-for-harvesting-timbervice-to-help-with-estimating-condiharvester-seasons-new-climate-sere-shape.eu/index.php/news-events/dersearchEuropeanprojectlyvester-seasonshttps://climate.copernicus.eu/harCOPERNICUS/2019/C3S_428g_FMI:ECMWF/.Theservicewaspartfundedbye-shape.Thee-shapehasreceivedfundingfromtheUnion’sHorizon2020reandinnovationprogrammeungrantagreement820852:https://
in most of Finland. FMI’s Clim. Bull. Res. Lett., 3(1), 4–7, https://doi.org/10.35614/ISSN-2341Metsäkeskus,6408-IK-2021-02-RL2021:Korjuukelpoisuus.
The Deutscher Wetterdienst (German Meteorological Service, DWD) provides operational seasonal climate predictions that are statistically downscaled for selected German cities. The new climate service, based on the German Climate Forecast System, was developed within the European Union Horizon 2020 project e-shape and is part of the DWD climate prediction website at www.dwd.de/climatepredictions.
DOI: 10.35614/ISSN-2341-6408-IK-2022-06-RL
Receivedvariability26Nov.2021,accepted
For a robust statistical estimate of the quality and reliability of the pre dictions, a large number of historical forecasts starting in the past (also called re-forecasts or hindcasts) are calculated for each forecast and com pared to observations. Each forecast starts with slightly varying initial con ditions of the climate system. The re sulting variety of solutions, also called ensemble, is used to evaluate the un certainties caused by uncertainties in the assimilated observations and the non-linearity of the climate system. In GCFS2.1 the ensemble is gener ated by different methods in atmos phere and ocean. For the hindcasts, GCFS2.1 starts 30 ensemble members for each calendar month in the years between 1990-2020. Real-time fore casts are performed with 50 ensem ble members per forecast to achieve even more robust forecasts than the 30 members in the past (for which 50 members would not be feasible due to the high computational demand). For Germany, the spatial resolution of the global climate predictions is increased using the empirical-statis tical downscaling method EPISODES (Kreienkamp et al. 2019). EPISODES consists of two modules. In the first step, the statistical relationship be tween large-scale processes (e.g., large-scale relative humidity fields), prescribed by reanalysis data or the global climate forecast model and high-resolution precipitation and tem perature observations, are defined by the combination of the selection of analogue days with a follow-up re gression. In the second step, multi variate time series are created with a weather generator on the high-reso lution target grid for various output variables. The HYRAS gridded data (Razafimaharo et al. 2020, Rauthe et al. 2013) are used as near-surface ob servational data for the downscaling.
Seasonal climate predictions for cities to strengthen urban resilience to climate
| 17
SASKIA BUCHHOLZ, ANDREAS PAXIAN, BIRGIT MANNIG, AMELIE HOFF Deutscher Wetterdienst Potential impact of the service. The DWD recently launched a new climate service for selected German cities based on seasonal climate predictions for the two variables, near-surface (2 m) temperature and precipitation. The new service aims at decreasing the vulnerability of urban population to hazardous weather events and risks caused by climate variability. Climate predictions for urban areas can pro vide the scientific basis for midterm planning decisions. The new product is tailored for city authorities helping to plan and prepare, e.g., for periods of anomalous high temperatures, so that preventive measures can be taken in the occupational health and safe ty sectors. With regard to long-last ing dry periods, the decision makers can plan the distribution of water re sources among different sectors (e.g., agriculture, industry, and urban green irrigation) more effectively. Description of the pilot. Seasonal predictions give a prognosis on the development of temperature and pre cipitation for the coming 1-6 months. The German Climate Forecast System Version 2.1 (refined version based on GCFS2.0) (Fröhlich et al. 2021) pro vides the operational seasonal pre dictions for the new climate service. It is based on the global coupled earth system model of the Max Planck In stitute for Meteorology (MPI-ESMHR) (Müller et al. 2018, Mauritsen et al. 2018). Exact initial conditions of a forecast are crucial for the forecast skill. For the climate forecast to be as close as possible to the real state of the atmosphere, observations are as similated into the MPI-ESM-HR prior to the start of the predictions. The cur rent GCFS2.1 uses continuous nudging to bring the state of the model climate close to the observation-based state of the climate (Baehr et al. 2015, Fröh lich et al. 2021).
FMI’S CLIMATE BULLETIN: RESEARCH LETTERS 1/2022
31 May 2022, first online 02 Jun. 2022, published 02 Sep. 2022
German
The EPISODES output has a horizon-
FIG 1: Map of the seasonal ensemble mean prediction of temperature for the e-shape pilot city Aschaffenburg. (Figure source: DWD) 18 | FMI’S CLIMATE BULLETIN: RESEARCH LETTERS 1/2022
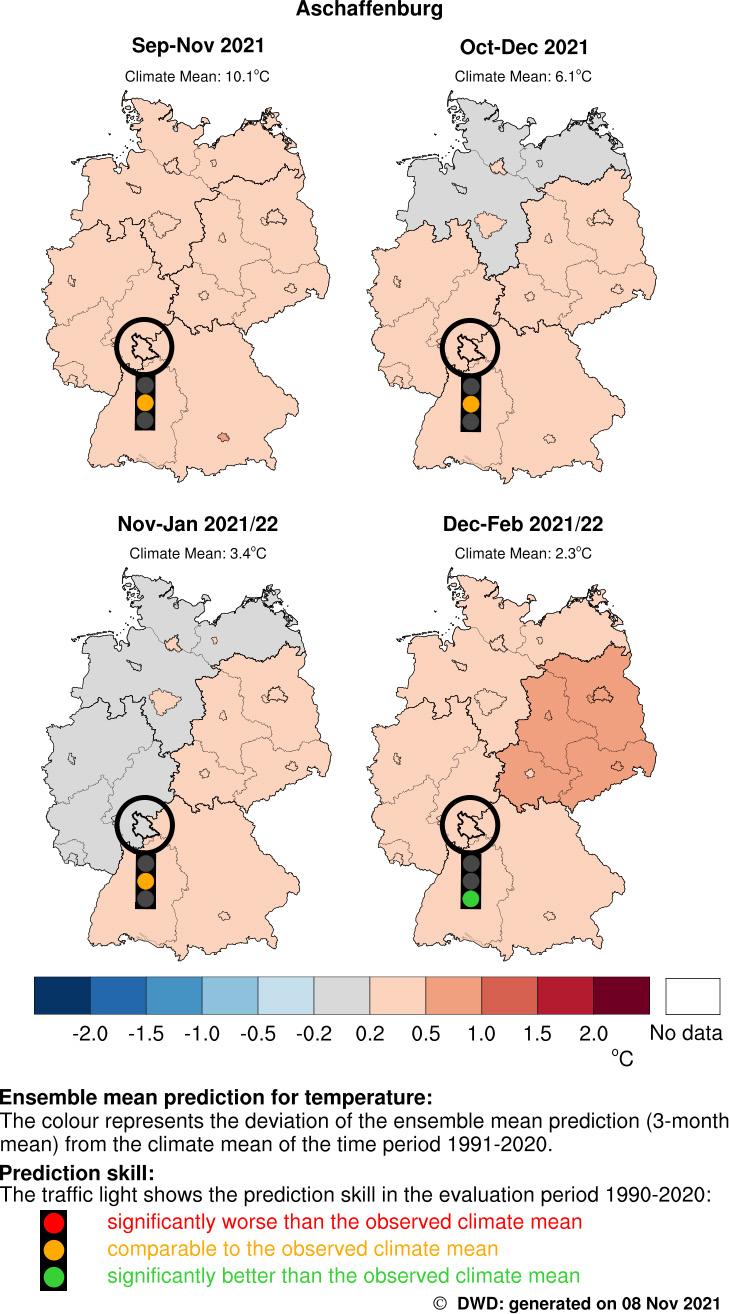
FMI’S CLIMATE BULLETIN: RESEARCH LETTERS 1/2022 | 19
tal grid point distance of about 5 km. The seasonal prediction for each city is an average of all grid boxes within the city boundary. In the case the city area is small, fewer than the usual min imum number of 9 grid boxes are used for aggregation. This is still feasible as the EPISODES output is no dynamical model output (which needs to be ag gregated for larger areas) but is based on real observations. The new service includes maps (Fig. 1), time series (Fig. 2) and tables of the ensemble mean and probabilistic predictions in combination with the prediction skill of temperature and precipitation for the 16 German state capital cities and Aschaffenburg (e-shape partner for the service development). The quality (i.e., skill) of the climate prediction is evaluated against the skill FIG 2: Time series of the seasonal probabilistic prediction of precipitation for the German capital city Berlin. (Figure source: DWD)
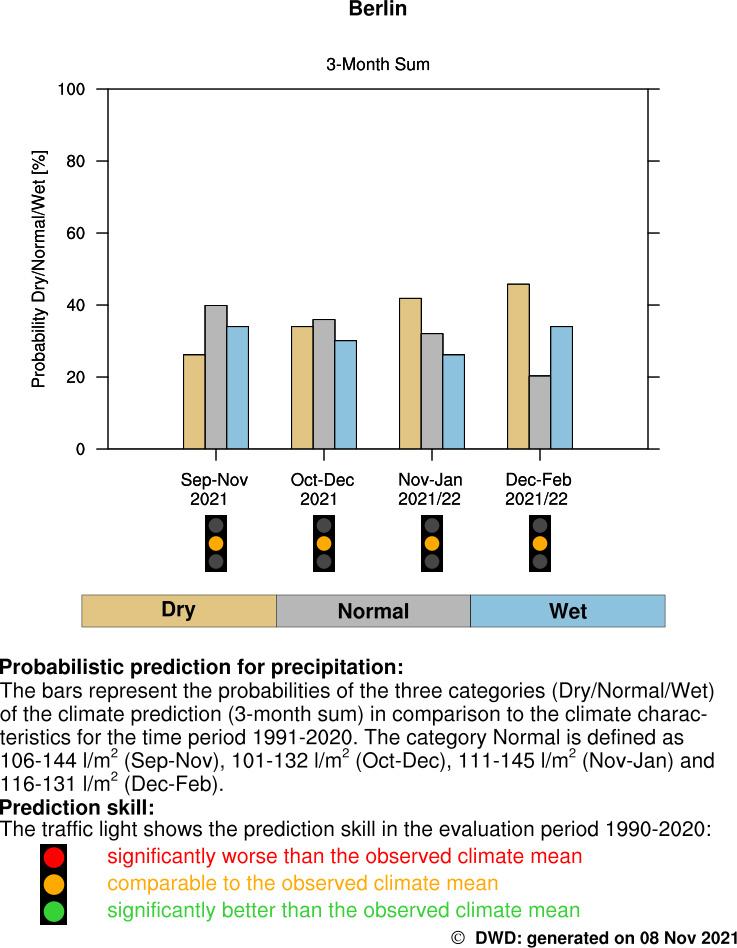
Müller, W. A., and Coauthors, 2018: A Higher-resolution Version of the Max Planck Institute Earth System Model (MPI-ESM1.2-HR). J. Adv. Model. Earth Syst., 10(7), 1383-1413, https://doi.org/10.1029/2017MS001217
Rauthe, M., H. Steiner, U. Riediger, A. Mazurkiewicz, and A. Gratzki, 2013: A Central European precipitation climatology – Part I: Generation and validation of a high-resolution gridded daily data set (HYRAS). Meteorologische Zeitschrift, 22(3), 235-256, https://doi.org/10.1127/0941Razafimaharo,2948/2013/0436C., S. Krähenmann, S. Höpp, M. Rauthe, and T. Deutschländer, 2020: New high-resolution gridded dataset of daily mean, minimum, and maximum temperature and relative humidity for Central Europe (HYRAS). Theor. Appl. Climatol., 142, 1531-1553, https://doi.org/10.1007/ prediction climate mean (climatology) observed over the evaluation period, which is commonly used by climate data users if climate predictions are not available. For the evaluation we use all available hind casts, which encompass the initialisa tion years 1990–2020. The skill score of the ensemble mean prediction is determined using the skill score of the mean-squared error between the en semble mean of the hindcasts and the actual observation (MSESS). The pre diction skill score of the probabilistic predictions is determined using the ranked probability skill score (RPSS), which examines to which extent the predicted probabilities of occurrence of the categories (e.g., ‘below normal’, ‘normal’, ‘above normal’, thresholds are the 33rd and 66th terciles of the reference period 1991–2020) agree with the category actually observed. The reference prediction for the skill score is the observed climatology. A bootstrapping method is then applied to verify whether the skill comparison between hindcasts and a reference prediction is subject to accidental variations due to small sample sizes (significanceEPISODEStest).conserves the skill of the downscaled hindcasts for the two variables, near-surface (2 m) tem perature and precipitation. Thus, the seasonal information is available at a higher spatial resolution without los ing skill. Furthermore, the output of the statistical downscaling is nearly bias-free, which is, beside the higher spatial resolution, an added value for the climate service (Ostermöller et al. 2021). Co-design. The design of the DWD climate prediction website has been developed in close cooperation with users from various sectors. At the be ginning of the service development for German cities, a small survey was carried out among eleven cities for their requirements on the climate prediction products. During two indi vidual meetings with representatives from the municipality of Aschaffen burg (departments of urban planning and environmental protection), the city’s individual needs for the ser vice (e.g., graphs and description of temperature extremes and threshold exceedances, information on hot and dry episodes and heavy precipita tion) could be discussed. During the last decade, city authorities in Ger many have gained knowledge to deal with climate projections, impacts and uncertainties. Despite the achieved goals in the interpretation of climate projections, there are still knowledge gaps for the vast majority of cities when it comes to climate predictions and their added value. The website content is presented in two sections, “basic” and “expert”, to cater for the specific needs of differ ent users. The “basic climate predic tions” place a particular emphasis on comprehensibility, while the “expert climate predictions” offer more de tailed information, including predic tion skill maps. City authorities attach great importance to data quality and uncertainties. To offer a low-threshold access to this information, a user-ori ented traffic light shows at first glance the qualitative information about the prediction skill (“basic climate predic tions”). It indicates whether regional mean climate predictions are signifi cantly better (green), not significant ly different (yellow) or significantly worse (red) than the reference predic tion. A feedback formula on the web site offers the possibility to express suggestions, praise, and criticism. Fur thermore, the DWD holds an annual workshop for end-users and informs twice per year about new innovations and changes via a newsletter.
Baehr J., and Coauthors, 2015: The prediction of surface temperature in the new seasonal prediction system based on the MPI-ESM coupled clima te model. Clim. Dyn., 44, 2723–2735, https://doi.org/10.1007/s00382-014-2399-7
ofs00704-020-03388-wthereference
Mauritsen, T., and Coauthors, 2018: Developments in the MPI-M earth system model version 1:2 (MPI-ESM1.2) and its response to increasing CO2. J. Adv. Model. Earth Syst., 11(4), 998-1038, https://doi.org/10.1029/2018MS001400
Acknowledgements: We thank Kristina Fröhlich, Sascha Brand and Katharina Isensee for their support with GCFS2.1, as well as Philip Lorenz and Frank Kreienkamp for their sup port and further adjustments of the EPISODES model. Besides, we would like to thank Klaus Pankatz for his help with the evaluation, Miriam Tivig for her support with the climate forecast website and Barbara Früh for her con ceptual guidance. The e-shape project is funded under the European Union’s Horizon 2020 research and innovation programme under grant agreement 820852.
20 | FMI’S CLIMATE BULLETIN: RESEARCH LETTERS 1/2022
Ostermöller, J., P. Lorenz, K. Fröhlich, F. Kreienkamp, and B. Früh, 2021: Downscaling and Evaluation of Seasonal Climate Data for the European Power Sector. Atmosphere, 12(3), 304, https://doi.org/10.3390/atmos12030304
Fröhlich, K., and Coauthors, 2021: The German Climate Forecast System: GCFS. J. Adv. Model. Earth Syst., 13(2), Kreienkamp,https://doi.org/10.1029/2020MS002101F.,A.Paxian,B.Früh,P.Lorenz, and C. Matulla, 2019: Evaluation of the empirical-statistical downscaling method EPISODES. Clim. Dyn., 52, 991-1026. https://doi.org/10.1007/s00382-018-4276-2
FMI’S CLIMATE BULLETIN: RESEARCH LETTERS 1/2022 | 21 DOI:
The
Hydrological Forecast System –a service supporting hydropower production in Northern Finland
1Finnish Meteorological Institute
JAAKKO IKONEN1, CEMAL TANIS1, MATTI KÄMÄRÄINEN1, MIRIAM KOSMALE1, HEIKKI POIKELA2, MIKKO STRAHLENDORFF1
published
The Finnish Meteorological Institute has recently developed a web-service that provides detailed hydrometeorological information to support more efficient hydropower production operations in Northern Finland. This service aims to reduce uncertainties in freshet forecasts through assimilation of satellite data-based cryosphere products to an in-house developed hydrological modelling system (HOPS) and an in-house developed machine learning streamflow forecasting algorithm. The service leverages common European efforts in climate monitoring by linking data from the Copernicus Climate Data Store with Copernicus seasonal forecasts data records as well as Copernicus Earth Observations.
Received 17 Dec. 2021, accepted 31 May 2022, first online 03 Jun. 2022, 02 Sep. 2022 10.35614/ISSN-2341-6408-IK-2022-07-RL
2Kemijoki Oy Background. Kemijoki Oy is the most important hydropower and adjustable power producer in Finland. Kemijoki Oy operates 16 hydropower plants and 4 regulated reservoirs in the Kemijoki watercourse area. These simultane ously act as the most important flood regulation structures in Northern Fin land. Currently, hydropower produc tion accounts for approximately 19% of total energy production in Finland. Freshet inflows to hydropower res ervoirs, typically from mid-April/ear ly-May to the end of June, accounts for 55 to 70% of the total annual in flows to reservoirs in the Kemijoki watershed. It is therefore essential to have careful planning and reservoir management schemes in place well before the onset of the peak inflow volumes. Reservoir management is important as the energy demand is out of phase with the natural availa bility of the water resources; typically, demand is higher during the colder months when the inflows are lower and vice versa. Hydropower produc ers need to redistribute the availabili ty of these resources from the spring inflow periods to other times of the year when electricity demand is high er, i.e., during the six months of colder weather while maintaining the bal ance between a sufficiently large vol ume of water for optimal production and enough remaining capacity for safe flood risk management. Traditional hydrological forecast ing in the Kemijoki watershed. FMI’s Hydrological Operations and Predic tions System (HOPS) model is used operationally by FMI to provide daily hydrological nowcasts, 10-day deter ministic forecasts and 90-day ensem ble forecasts for Kemijoki Oy over the entire Kemijoki watershed (FMI 2021). The HOPS modelling system consists of a distributed and modified ver sion of the Sacramento Soil Moisture Accounting Model (SAC-SMA) (Bur nash 1995) coupled with a modified version of the SNOW-17 temperature index snow accumulation and abla tion model, a soil temperature model based on Rankinen et al. (2004) and an in-house developed distributed routing model accounting for over land, and channel flow retention and attenuation, based on hydrographic properties. The parameters for the SAC-SMA model are derived from a semi-physical a-priori parametriza tion scheme introduced by Koren et al. (2000). Satisfactory simulation re sults have been achieved (with an av erage Nash-Sutcliffe coefficient (NS) of efficiency of 0.79 and an average Pearson correlation of 0.94 between observations and hindcast simulations across selected validation points, see e.g., Fig. 1.) by running the modelling system without any calibration apart from manually adjusting parameters governing snowmelt rates. Machine learning for a novel approach for hydrological forecasts. Machine learning algorithms have also been tested and implemented in the Kemijoki
Kemijoki watershed with very promis ing results. The machine learning model used is based on gradient boosting. The machine learning pro cess consists of constructing a care fully designed predictor set to which an optimized gradient boosting re gression model between the predictor data and the streamflow observations in each measurement station using historical data between 2007–2020 is fitted. As predictors, the model uses the ECMWF HRES deterministic tem perature and precipitation forecasts over the watersheds of measurement stations, the analytical representa tions of annual and daily cycles, and the observed streamflow of each sta tion in an autoregressive manner. To be able to simulate the cumulative ef fect of, e.g., precipitation and melting processes, the weather model output is lagged in time multiple times; for example, when predicting the stream flow at t=0, precipitation values of the catchment grid cells from t=0, t=-1, t=2, ... t=-10 time steps are considered. The model was proven to be able to produce good forecasts despite a relatively small and restricted set of predictors, and with a more complete FIG 1: (a) Location of the Kemijoki HOPS modelling domain (red dots: regulated flow points, blue dots: natural flow observation points) and (b) Streamflow simulation (HOPS) timeseries at the Marraskoski observation point and comparison with Finnish Environment Institute’s observations (OBS). predictor data set, including, e.g., satellite observations and predict ed snow depths, even better perfor mance is expected.
Example results of SWE assimilation can be seen in Fig 2. and Fig 3. As similation of EO-based SWE data to HOPS model simulation runs in, for example the Kemihaara sub-basin, ge nerally improves overall peak stream flow forecasting results. However, this is not always the case. In 2018, for example, the peak streamflow error to observed streamflow peak is in creased from 141 m3/s to 365 m3/s. The NS coefficient and Pearson cor relation between observed and simu lated streamflow for the spring peak runoff period (April 1st to June 30th) is however not significantly affect ed. From 2019 onwards, assimilation of EO-based SWE observations im proves spring streamflow forecast re sults with varying success. However, in general, improvements of both Pear son correlation and NS are minimal apart from the spring of 2021, where NS is increased from 0.49 to 0.89. The more significant improvements in sim ulation accuracies manifest improve ments in peak streamflow error while
22 | FMI’S CLIMATE BULLETIN: RESEARCH LETTERS 1/2022
Assimilation of satellite-based cryosphere products. Assimilation of Earth Observation (EO) based snow observations can significantly reduce uncertainties in hydrological mod el snowpack simulation. Currently Copernicus EO-based Snow Water Equivalent GlobLand SWE (Coper nicus 2021) data is operationally as similated into the HOPS modelling procedure. The use of EO-based snow observations offer two main types of advantages: 1) EO-based snow obser vations are independent from point scale observations (usually used to drive hydrological models), and 2) they provide basin-wide information and data on the state of the snow pack as opposed to point scale obser vations.Snowfall is a dominant control vari able in SWE accumulation. We mod ify snowfall forcing according to EObased SWE observations to obtain the required model SWE accumulation constraint. In our application, the cor rections are determined by comparing EO-based SWE observations with an initial model simulation and then ap plying the corrections retrospectively as part of a second model simulation.
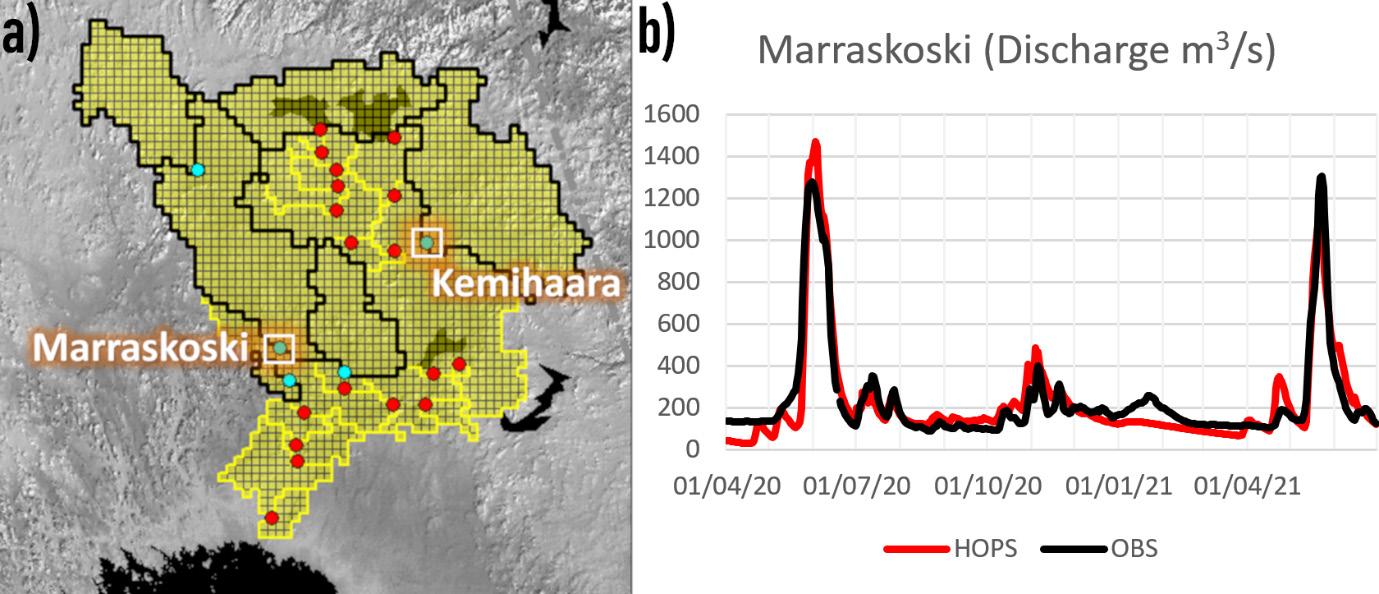
FIG 2: Kemihaara streamflow observations (see Fig. 1a) and HOPS simulation runs with (Qsim SAT) and without (Qsim GD) SWE assimilation (left, 2018 and right, 2019).
FIG 3: Kemihaara streamflow observations (see Fig. 1a) and HOPS simulation runs with (Qsim SAT) and without (Qsim GD) SWE assimilation (left, 2020 and right, 2021). LETTERS 1/2022
FMI’S CLIMATE BULLETIN: RESEARCH
Burnash, R.J.C., 1995: The NWS river forecast system—catchment modeling. Computer Models of Watershed Hydrology, V.P. Singh, Ed., Water Resources Publications, 311–366, https://www.wrpllc.com/books/cmwhn.html
Copernicus, 2021: Snow Water Equivalent. Copernicus Global Land Service, Accessed 17 December 2021, e-shape,https://land.copernicus.eu/global/products/swe2021:Pilot7.4|Hydropowerinsnowreservoir – climate service. Accessed 17 December 2021, https://e-shape.eu/index.php/showcases/ Finnishpilot7-4-hydropower-in-snow-reservoir-climate-serviceMeteorologicalInstitute(FMI),2021:KemijokiHydrological Forecasting System. Finnish Meteorological Institute, Accessed 17 December 2021, Koren,https://hops.fmi.fi/V.I.,M.Smith,D.Wang, and Z. Zhang, 2000: Use of Soil Property Data in the Derivation of Conceptual Rainfall-Runoff Model Parameters. Proc. 15th Conf. Hydrol. 2000, Long Beach, CA, Amer. Meteor. Soc., 103–106. Rankinen, K., T. Karvonen, and D. Butterfield, 2004: A simple model for predicting soil temperature in snow-covered and seasonally frozen soil: model description and testing, Hydrol. Earth Syst. Sci., 8, 706–716, https://doi.org/10.5194/hess-8-706-2004 peak flow timing errors remain largely unchanged. In spring 2019, the peak streamflow error is reduced from 141 m3/s to 86 m3/s and in 2020 from 247 m3/s to 105 m3/s as well as in 2021 from 451 m3/s to only 46 m3/s. In the spring of 2021, however, the peak flow timing error is increased from -1 days to -5 days. Acknowledgement. The service is funded by the e-shape project. The e-shape project has received fund ing from the European Union’s Ho rizon 2020 research and innovation programme under grant agreement 820852 (e-shape 2021).
| 23
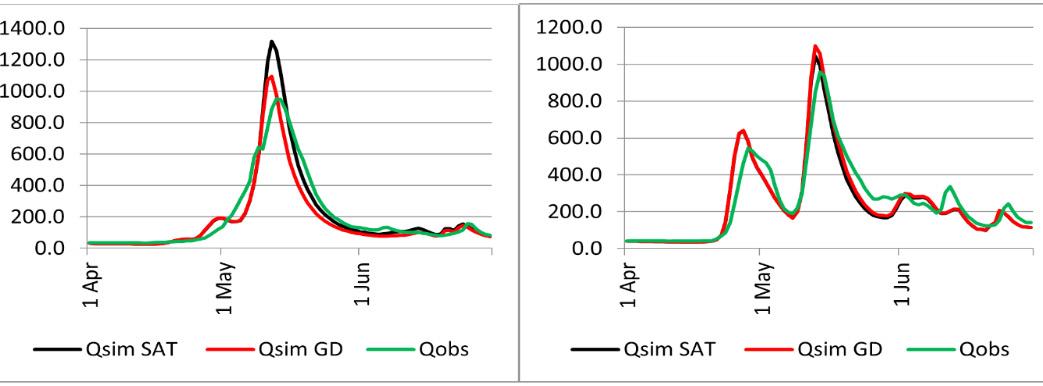
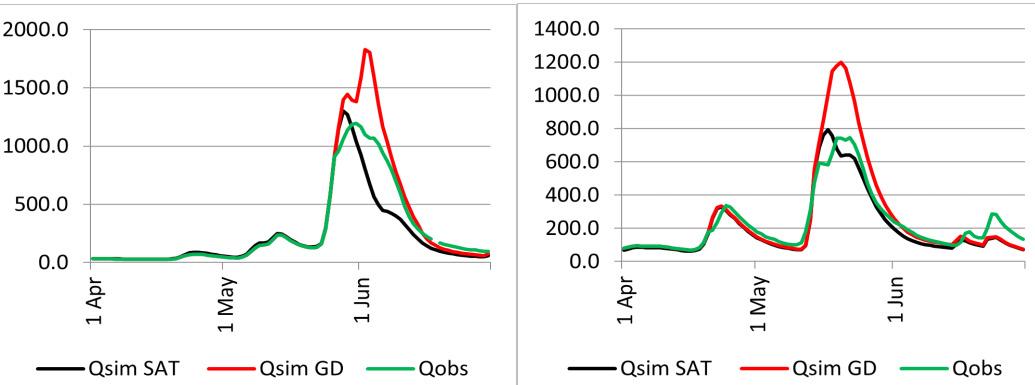
In order to improve the user uptake of the EO data the H2020 project e-shape (EuroGEO Showcases: Appli cations Powered by Europe) aims to gather these EO resources in Europe and enhance the capabilities of exist ing European services in addressing different societal challenges. Further more, e-shape supports the coordi nated exploitation of EO data and services through 27 cloud-based pilot applications in seven thematic areas, as well as the generation of informa tion for these applications together with the users.
SANDRO OSWALD1, MAJA ZUVELA-ALOISE1, MICHAEL AVIAN1, CHRIS SCHUBERT2
As part of e-shape, the showcase “Urban resilience to extreme weather” consists of three pilots with the goal to strengthen resilience and adaptive capacity to extreme weather in sum mer season on various timescales (e.g. from seasonal forecasts to climate projections). The pilot “Climate pro jection products for Austrian cities”, carried out by Austrian National Me teorological and Geophysical service (ZAMG), demonstrates the develop ment of climate services for urban re gions by creating climate projections for various Austrian cities. This is done by providing high spatial resolution climate information based on the EO data and modelling, focusing on heat indices. Data are made freely avail able on a national climate data plat form (Climate Change Centre Austria (CCCA) data) to support urban plan ning, risk management and environ mental protection. Thereby, the pilot follows the standardization of the method for urban climate modelling and its output to ensure a comparable modelling basis for other European cities.
DOI: 10.35614/ISSN-2341-6408-IK-2022-08-RL
24 | FMI’S CLIMATE BULLETIN: RESEARCH LETTERS 1/2022
Future climate projection of heat indices for
1Zentralanstalt für Meteorologie und Geodynamik (ZAMG), Vienna, Austria 2Vienna University of Technology (TU Wien) Urban heat in changing climate. The local surface energy balance is al tered in cities due to (i) high fraction of sealing and (high) building density as well as (ii) comparably low fraction of vegetation land cover. As a result, urban areas have higher ambient tem peratures than rural ones, also known as the Urban Heat Island (UHI) effect (Landsberg 1981; Oke 1982). Due to the rising urbanization and growing fraction of sealed surfaces in total land use, temperature is increasing in urban areas. Hence, more and more people suffer during extreme heat waves as, e.g., in the summers of 2003 (García-Herrera et al. 2010) or 2015 (Urban et al. 2017). In order to coun teract the negative effects of heat waves, which are expected to inten sify even further in the future (IPCC 2013), and develop urban planning strategies for the mitigation of the UHI, detailed information on thermal conditions within a city is required, in cluding possible development of heat extremes in the future.
Based on Earth Observation and modelling data, climate indicators for urban regions can support sustainable urban planning, risk management and environmental protection. The current study demonstrates the development of climate services for major cities in Austria by providing climate projections on an urban scale. This approach focuses on heat indices and improves the data uptake by end users through standardizing modelling methodology, as well as formats, and facilitating data access. This enables quick visualization using data services of the Austrian national Climate Change Data Centre.
Urban resilience to extreme weather. The European Commission imple mented several climate services, such as the Copernicus Climate Change Service (C3S), providing climate in dicators based on Earth Observation (EO) information and modelling data in order to support different types of end users and decision makers tack ling the problems of climate change.
Received 29 Nov. 2021, accepted 31 May 2021, first online 03 Jun. 2022, published 02 Sep. 2019
Austrian major cities: strengthening urban resilience and meeting user needs
Urban climate model simulations
FMI’S CLIMATE BULLETIN: RESEARCH LETTERS 1/2022 | 25
Within the cooperation with Germa ny’s National Meteorological Service (DWD), the ZAMG carried out urban climate model simulations with the MUKLIMO_3 model (Sievers 2016), which allows utilization of various satellite products of Copernicus ser vices (e.g. Urban Atlas, tree cover
FIG 1: Schematic workflow of deriving climate indices based on EO data, urban climate model simulations and regional climate projections for the major cities in Austria. Top and mid left: Modelling system including integration of the EO data and below the cuboid method is illustrated. Lower left: Number of summer days for the city of Vienna as a 30-year average for the scenarios RCP4.5 and RCP8.5 as well as the periods of 2021-2050 and 2071-2100. Lower right corner: Number of summer days for Vienna (spatial information, time series) visualised on the Climate Change Centre Austria (CCCA) data server. Download here for full-size image. (Source: ZAMG) density etc.) as input data to describe land surface distribution and proper ties. With the simulation of radiation, soil and atmospheric temperature, relative humidity, and wind flow in ur ban areas on a 3D grid (100 m spatial resolution, vertical resolution 10–100 m), the model provides the basis for evaluation of the (surface) tempera ture distribution within urban areas. In combination with meteorological measurements and regional climate model scenarios (representative for the background climate by applying the cuboid method (Früh et al. 2011, Žuvela-Aloise et al. 2018, Geletič et al. 2019)), the model can be used to derive several climate indices related
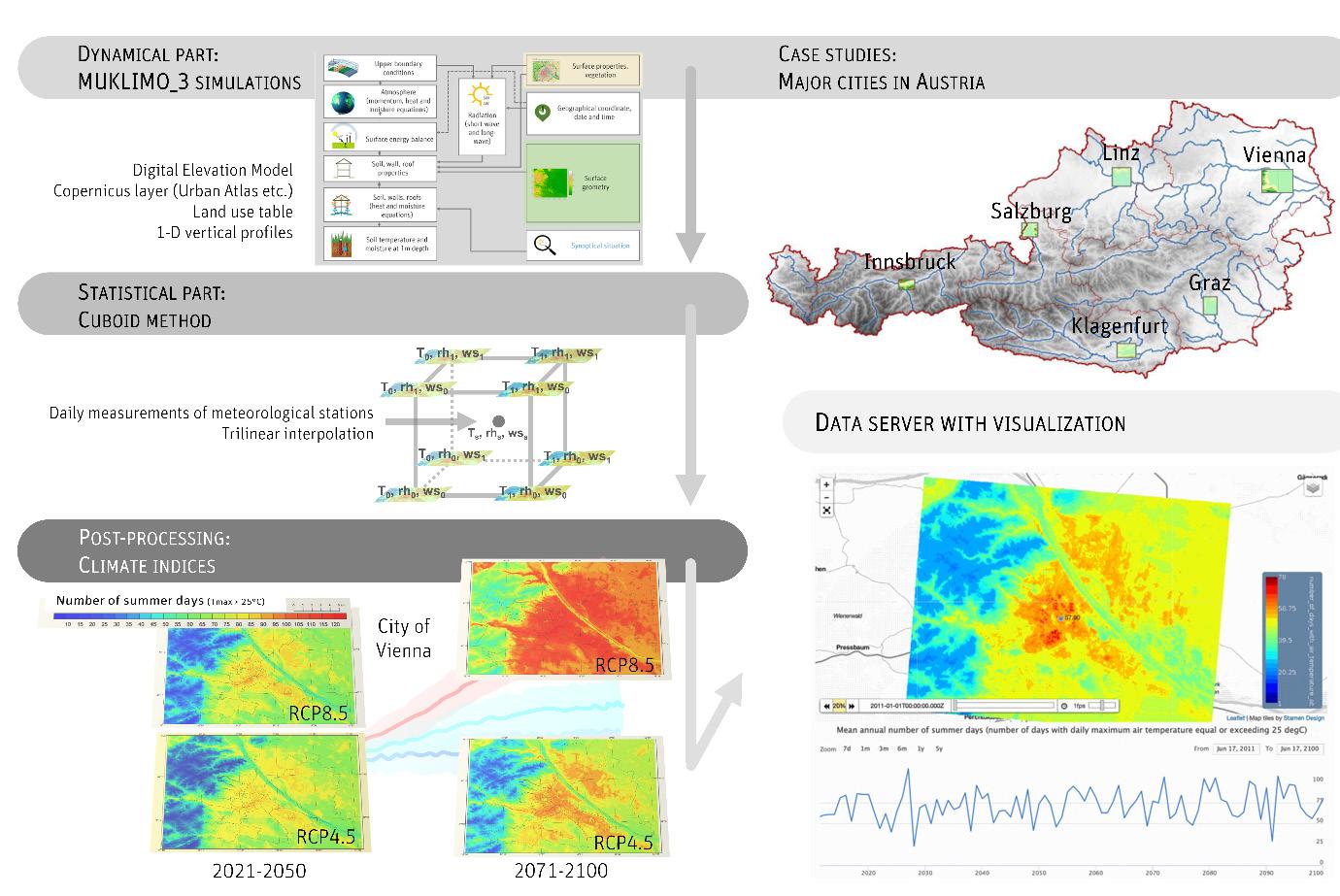
2011: Estimation of climate-change impacts on the urban heat load using an urban climate model and regional climate projections. J. Appl. Meteor. Climatol., 50(1), 167–184, https://doi.org/10.1175/2010JAMC2377.1 García-Herrera, R., J. Díaz, R. M. Trigo, J. Luterbacher, and E. M. Fischer, 2010: A review of the European summer heat wave of 2003. Crit. Rev. Envi ron. Sci. Technol., 40(4), 267-306, https://doi.org/10.1080/10643380802238137
Geletic, J., M. Lehnert, P. Dobrovolny, and M. Zuvela-Aloise, 2019: Spatial modelling of summer climate indices based on local climate zones: expe cted changes in the future climate of Brno, Czech Republic. Climatic Change, 152, 487–502, https://doi.org/10.1007/s10584-018-2353-5
Landsberg, H. E. (editor), 1981: The Urban Climate. Academic Press, 275 pp, ISBN: 9780080924199. Oke, T. R., 1982: The energetic basis of the urban heat island. Quart. J. R. Met. Soc., 108, 1-24, https://doi.org/10.1002/qj.49710845502
| FMI’S CLIMATE BULLETIN: RESEARCH LETTERS 1/2022
Schubert C., G. Seyerl, and K. Sack, 2019: Dynamic Data Citation Service—Subset Tool for Operational Data Management. Data, 4, 115, Sievers,https://doi.org/10.3390/data4030115U.,2016:DaskleinskaligeStrömungsmodell MUKLIMO 3 Teil 2: Thermodynamische Erweiterungen. Berichte des Deutschen Wetterdienstes, 248, 151 pp, ISBN 978-3-88148-490-9, http://nbn-resolving.de/urn:nbn:de:101:1-201606173510 (pdf).
IPCC, 2013: Climate Change 2013: The Physical Science Basis. Contribution of Working Group I to the Fifth Assessment Report of the Intergovern mental Panel on Climate Change, Cambridge University Press, Cambridge, United Kingdom and New York, NY, USA, 1535 pp, Jacob,https://doi.org/10.1017/CBO9781107415324D.,andCoauthors,2014:EURO-CORDEX: new high-resolution climate change projections for European impact research. Reg. Environ. Change, 14, 563–578, https://doi.org/10.1007/s10113-013-0499-2
26
Urban, A., H. Hanzlíková, J. Kyselý, and E. Plavcová, 2017: Impacts of the 2015 heat waves on mortality in the Czech Republic—A comparison with previous heat waves. Int. J. Environ. Res. Public Health, 14, 1562, https://doi.org/10.3390/ijerph14121562
CCCA, 2011: Climate Change Centre Austria. Accessed 09 May 2022, https://data.ccca.ac.at CF-MC, 2010: Climate and Forecast (CF) Metadata Conventions. Accessed 09 May 2022, https://cfconventions.org e-shape 2022: e-shape Project-Urban resilience to extreme weather. Accessed 09 May 2022, https://data.ccca.ac.at/group/e-shape-urban-resilien Früh,ce-to-extreme-weatherB.,andCoauthors,
Wilkinson, M., and Coauthors, 2016: The FAIR Guiding Principles for scientific data management and stewardship. Sci. Data, 3, 160018, dehttps://doi.org/10.1038/sdata.2016.18Wit,R.,andCoauthors,2020:Supporting climate proof planning with CLARITY’s climate service and modelling of climate adaptation strate gies–the Linz use-case. Urban Climate, 34, 100675, https://doi.org/10.1016/j.uclim.2020.100675
Žuvela-Aloise, M., K. Andre, H. Schwaiger, D. N. Bird, and H. Gallaun, 2018: Modelling reduction of urban heat load in Vienna by modifying surface properties of roofs. Theor. Appl. Climatol., 131, 1005-1018, https://doi.org/10.1007/s00704-016-2024-2
to heat conditions such as the annu al average number of summer days (days with maximum air temperature ≥ 25 °C). The regional climate model simulations from the EURO-CORDEX project (Jacob et al. 2014) provide information on meteorological vari ables until 2100 under various climate change scenarios, e.g., RCP4.5 and RCP8.5 (IPCC 2013). An ensemble of model output for different RCP sce narios is used to project future heat conditions in urban areas. In the pi lot, eight different combinations of bias corrected regional/global climate model output were used to calculate climate indices for each year between 2011 and 2100 (Fig. 1). Co-design of climate service and data uptake. In order to support ur ban planning and decision-making processes, urban climate projections and representation of climate infor mation have been gaining importance among the researchers and stake holders in recent years (e.g. de Wit et al. 2020). In case of urban resilience in Austria, continuous interaction be tween climate scientists, city admin istrations and urban planning experts have pointed out several key issues, which could improve the uptake of research results: (i) data accessibil ity, (ii) standardization of data for mats, (iii) improved data quality with description of uncertainties, and (iv) easy data presentation. To tackle at least aspects i, ii, iv and provide data as a service regarding FAIR Guiding Principles (Wilkinson et al. 2016), the Climate projection products are cu rated by the CCCA data repository (CCCA 2015) as part of the Austrian research data infrastructure. The urban climate projections data are distributed in the NetCDF format and follow the standards of NetCDF Climate and Forecast (CF) Metadata Conventions (CF-MC 2010) and Open Geospatial Consortium (OGC 2022) web services. The data collection for this pilot is available under e-shape (2022). The CCCA Data Centre en ables an on-the-fly interactive data visualisation of NetCDF data with its server architecture, e.g. THREDDS Data Server (Fig. 1, bottom right). The user (registration required) can adapt and modify style parameters (e.g. col our scheme, portrayal) and the map design. More relevant is the on-de mand processing. A time slider func tion allows to dive into different time scenarios and automatically creates a graph of the time series from 2011 until 2100 for a single spatial point. In addition, an integrated CCCA sub set service (Schubert et al. 2019) al lows for the creation and persistently republishing of any data fragments, filtered by an “Area of Interest” or a specific time frame. Source code of these functionalities is provided as open source on Github (Github 2008).
Acknowledgements: We thank the European Commission H2020 project e-shape (grant agreement No. 82085) for their support. The German Meteor ological Service (DWD) for providing the urban climate model MUKLIMO_3. The Climate Change Centre Austria for access to data services. We gratefully thank the cities of Vienna, Linz, Salz burg, Innsbruck, Graz, and Klagenfurt for providing diverse geoinformation.
Github, 2008: CCCA-DC at GitHub Inc. Accessed 09 May 2022, https://github.com/ccca-dc/ckanext-thredds
FMI’S CLIMATE BULLETIN: RESEARCH LETTERS 1/2022 | 27
Ilmatieteen www.ilmastokatsaus.fiilmastokatsaus@fmi.filaitos