EXECUTIVE
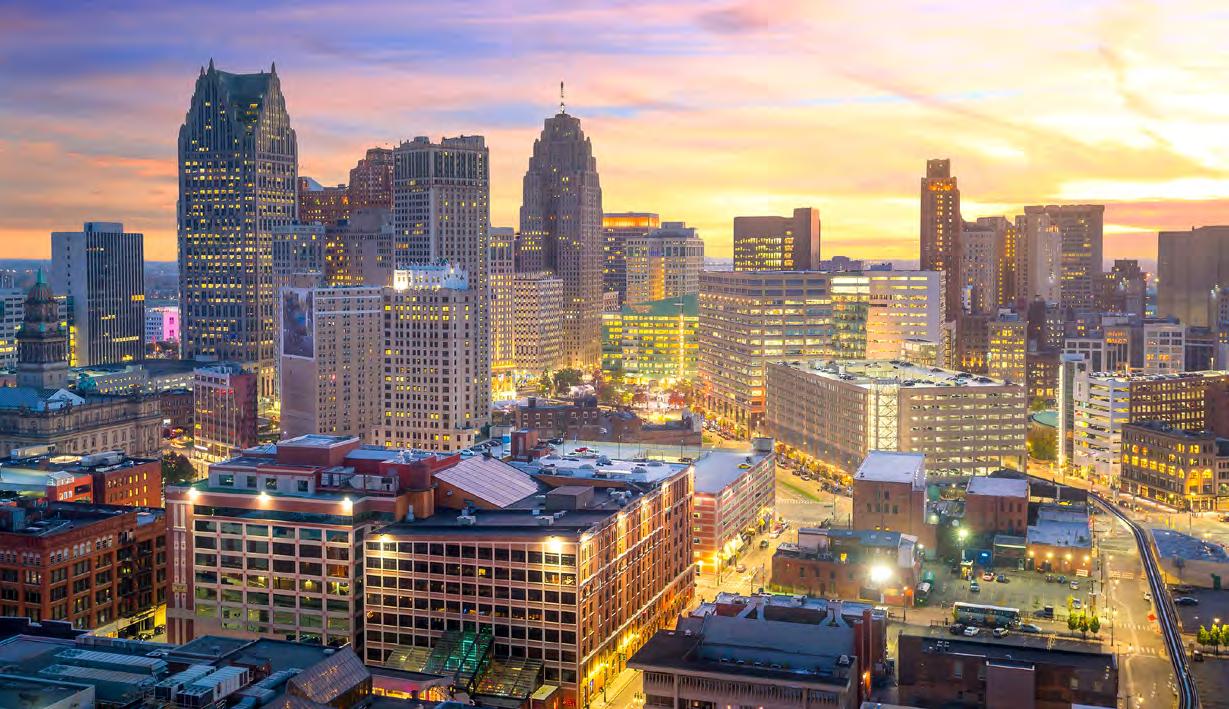
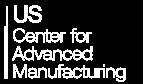
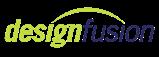
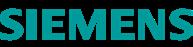
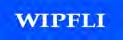

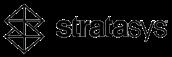
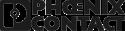
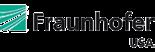
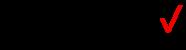


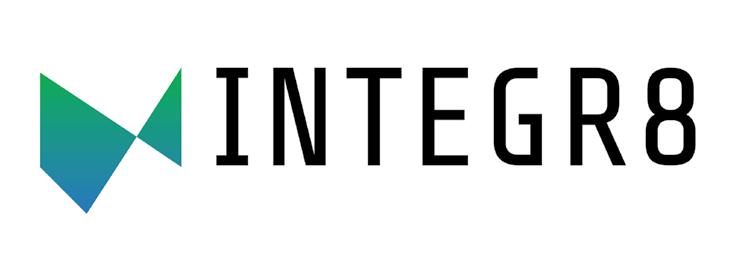
The Integr8 Roundtable Summit brought together leaders from manufacturing, technology, and government to discuss critical topics driving Industry 4.0 adoption and digital transformation. Attendees engaged in discussions on cutting-edge advancements, including IoT, AI, cybersecurity, additive manufacturing, and the shift to electric vehicles, exploring their implications for global trends, workforce development, and sustainability. This executive summary provides a comprehensive look at these insights, featuring perspectives from our key company sponsors and actionable takeaways from each roundtable, designed to guide industry decision-makers in navigating the future of manufacturing innovation. Plus, it offers an exclusive look at our 2025 topics—save the date to join us for next year’s series!
Thank you to our greatest supporter:
Additive manufacturing presents a transformative opportunity for production, enabling rapid innovation, customization, and supply chain flexibility. However, companies must address challenges related to awareness, workforce training, material understanding, and i ntegration with existing processes. Collaboration across industries and a culture of innovation are key to overcoming these hurdles and realizing the full potential of additive manufacturing.
“
The greatest opportunity for Industry 4.0 over the next decade is to sever the dependency on complex supply chains. Companies will leverage AI design and additive manufacturing to decentralize and localize their production. Reducing cost and lead time while improving quality and advancing sustainability.
Fadi Abro Global Automotive Director
Middle Ground between Prototyping and Production: Additive manufacturing plays a crucial role in fixturing and tooling, bridging the gap between prototyping and full-scale production, making it a valuable "additive first" solution.
Advanced Simulation Tools and Additive Cost Models: These tools enable companies to predict the performance and cost-effectiveness of additive manufacturing, improving decision-making in design and production.
Workforce Readiness: Today’s workforce is more open to adopting new technologies, making it easier to integrate additive manufacturing into existing processes.
Comprehensive Financial Analysis: Beyond traditional ROI calculations, companies are now considering risks and unexpected failures in their financial evaluations of additive manufacturing investments.
Reverse Engineering and Mass Customization:
Additive manufacturing facilitates reverse engineering, allowing for faster product iterations and repairs. Mass customization is possible but must comply with industry regulations and standards.
Faster Time-to-Market: Additive manufacturing enables rapid prototyping and product adaptation, leading to shorter development cycles and quicker market entry.
On-Demand Printing and Flexibility: The ability to print parts on demand and adapt to changes in design or part requirements enhances production flexibility and reduces inventory costs.
AI and Software Integration: AI-driven additive processes and advanced software are enhancing precision, optimizing material use, and improving overall production efficiency.
Supply Chain Diversification and Sustainability:
Additive manufacturing can diversify the supply base, allowing for more frequent product revisions, reduction in waste, and reuse of materials, contributing to a more sustainable production cycle.
Complex Geometries: Additive manufacturing allows the creation of complex geometries that traditional manufacturing cannot easily achieve, broadening design possibilities.
Awareness and Tooling Gaps for Additive-First Manufacturing: Significant gaps exist in industry awareness and the availability of necessary tools to convert traditional processes to additive manufacturing.
Process-Application Fit: Not all processes are suitable for additive manufacturing, and understanding which applications benefit most from it remains a challenge.
Integration with Other Manufacturing Processes:
Additive manufacturing does not operate in isolation; it requires integration with other traditional manufacturing processes for maximum impact.
Workforce Training Needs: Developing a skilled workforce capable of utilizing additive technologies remains a critical challenge.
Recycling and Sustainability: While additive manufacturing reduces waste, recycling solutions for additive materials are still underdeveloped, presenting sustainability challenges.
Material Understanding and Standardization: A broader understanding of materials and the establishment of industry-wide standards and certifications are essential to scale additive manufacturing effectively.
Cultural Shift and Innovation: A significant cultural shift is needed in organizations to embrace the innovative potential of additive manufacturing fully. This shift can unlock new problem-solving approaches and business models.
International Trade and Geopolitical Risks: Trade issues, particularly with countries like China, pose risks to the global supply chain for additive manufacturing, especially in sectors like defense and aerospace.
Leadership from Defense and Aerospace: These industries are leading in additive manufacturing adoption, offering a model for other sectors, including automotive, which faces unique challenges in implementation.
Technological Evolution: The fast pace of change in additive technologies requires continuous adaptation, making it difficult for companies to keep up with the latest advancements.
Artificial intelligence (AI) is transforming cybersecurity by enh ancing threat detection, response times, and overall resilience against cyber threats. AI algorithms can analyze vast amounts of data to identify patterns and anomalies that signal potential breaches, enabling proactive defense strategies. However, the integration of AI into cybersecurity also presents significant challenges. Organizations must address concerns related to data privacy, bias in AI models, and the potential for adversarial attacks that exploit AI systems. Additionally, there is a pressing need for skilled professionals who can interpret AI-driven insights and adapt security protocols accordingly.
““I expect to see a move to ‘Trust Architectures’ using distributed ledger technology, such as Blockchain, to become more efficient with identity management, audit compliance, and incorporation AI to aid with threat analysis.”
“Scott Beasley Senior Cybersecurity Sales Engineer
Become I4.0- or AI-ready. Data-Infrastructure readiness is crucial to proficiently collect, and clean data and make data accessible towards a unified architecture and ensure interoperability between OT and IT. Upgrade IoT Connectivity for manufacturing equipment and your data-infrastructure to enable Near-Edge AI applications. Practice Organizational (Culture) readiness —Artificial Intelligence (AI) in manufacturing is not a plug-and-play tool but requires a team to implement it and continuously improve it.
Sebastian Wicklein Director, Business Development Coordination
AI as a Consultant: AI can effectively recognize anomalies within vast data sets, helping detect threats before they escalate. It should be used to support human decision-making without making direct changes to systems.
Threat Prediction and Fire Drills: AI can simulate cyberattacks on internal systems, exposing vulnerabilities and preparing organizations for potential threats through predictive modeling and cybersecurity fire drills.
Proactive Security: AI allows for early identification of phishing attempts and other cyber threats, enabling organizations to address risks before they become major issues.
Return on Investment (ROI) in Security: AI can provide valuable ROI by preventing catastrophic incidents, balancing risk with workability, and justifying investment in cybersecurity infrastructure.
Workforce Literacy: A workforce knowledgeable in AI and cybersecurity strengthens security posture. Ongoing training ensures that employees are prepared to detect and manage cybersecurity risks.
Layered Security with AI: Integrating AI with existing security layers offers enhanced protection, allowing manufacturers to combat evolving cyber threats more effectively.
SaaS Vendor Vetting: Manufacturers should carefully vet SaaS vendors for cybersecurity before integrating them into the supply chain, ensuring they meet regulatory compliance and security standards.
Diversification of Security Tools: Relying on diverse AI and cybersecurity solutions, rather than monopolistic tools, helps mitigate risks and ensures the right tools are applied to the right problems.
Emergency AI Controls: Implementing manual cut-off mechanisms ensures that AI systems can be disabled if they begin to behave in unexpected ways, maintaining control over cyber operations.
Workforce as a Vulnerability: Each employee represents a potential entry point for cyberattacks, making comprehensive and consistent training essential across all levels of the organization.
Development Silos: AI systems are often developed in silos, leading to gaps in collaboration and increasing the risk of uncoordinated cybersecurity responses across departments.
Ethical Frameworks and Privacy Concerns: Global governments must define the ethical frameworks for AI, determining how AI can be used without violating privacy rights, and establishing clear rules for responsible AI usage.
Small Business Cybersecurity: SMEs often lack the resources or expertise to adequately address cybersecurity risks, making them more vulnerable to attacks and in need of proactive support from larger vendors and service providers.
Rapid AI Development: AI technology is advancing at a pace that outstrips the ability of cybersecurity measures to keep up, creating gaps in security as systems struggle to manage emerging threats.
False Positives: AI’s detection systems can flag false positives, complicating threat detection and increasing the burden on security teams to identify real risks.
Integration with Legacy Systems: AI cannot function in isolation; it must be integrated with legacy systems and other traditional cybersecurity processes to be fully effective.
Overconfidence in Old Systems: Relying on outdated cybersecurity measures and not adapting to new technologies can leave companies vulnerable to modern, sophisticated cyber threats.
Accountability in Vendor Relationships: Manufacturers must hold third-party vendors accountable for cybersecurity compliance, ensuring that the entire supply chain is secure.
Power and Data Requirements: AI requires significant data and energy resources, and manufacturers must secure these in ways that do not expose vulnerabilities or overextend their capabilities.
AI Monopolies: The rise of AI monopolies introduces the risk of overreliance on single-vendor solutions, potentially leading to vulnerabilities if those systems are compromised.
AI Hallucinations: The risk of AI producing inaccurate or false information (“hallucinations”) can create significant cybersecurity challenges, necessitating human oversight at all times.
Cultural Shift in Cybersecurity: To fully benefit from AI in cybersecurity, organizations must undergo a cultural shift, recognizing AI as a support tool rather than a replacement for human oversight and decision-making.
Smart factory technology is transforming manufacturing through the integration of automation, IoT, and data analytics, optimizing production and enhancing efficienc y. However, this transition brings challenges such as cybersecurity risks, the need for workforce training, and ensuring interoperability among diverse systems. To navigate these hurdles, collaboration between manufacturers, technology providers, and educational institutions is crucial. By addressi ng these issues, businesses can unlock the full potential of smart factories, paving the way for a more ag ile and resilient manufacturing ecosystem.
“To thrive in the next decade, manufacturers must embrace the fusion of AI, edge computing, and real-time data to create self-optimizing, autonomous production ecosystems. The boldest companies will move beyond incremental improvements and reimagine their entire value chains, unlocking levels of agility and efficiency that will define global leadership.
Luv Aggarwal Portfolio Development Executive - Digital Manufacturing
In the next decade, competitive advantages will go to those who use real-time data analytics and advanced visualization tools to create adaptive, self-optimizing production lines. As technology advances, we'll shift from today's data-rich yet insight-poor landscape. Companies adopting data-driven, autonomous manufacturing systems will significantly boost efficiency, innovation, and agility. Moreover, forward-thinking organizations will employ these optimized technologies to meet sustainability goals in manufacturing and will promote a more harmonious employee experience.
Jeff Hall Vice President - Global Strategic Sales
“We are heading towards a future where manufacturing will see a seamless integration of humans and machines, where augmented reality and AI can facilitate workers with real-time data and insights, fostering unprecedented levels of efficiency and innovation. Companies that embrace immersive technologies will empower their teams to innovate in virtual spaces, allowing them to create, simulate, and test new products and processes at a pace that has the potential to redefine industry standards. As we blur the boundaries between physical and digital, AR and VR will create new opportunities for product design, marketing, and customer experiences, that will transform the way companies engage with their target audience.
Dr. Khalid Mirza Founding Director, Augmented Reality Center
Intergenerational Knowledge Transfer: Younger employees can introduce technology, while older workers contribute operational expertise. This combination creates a powerful force for knowledge transfer and innovation within smart manufacturing.
Early Wins for Technology Implementation: Prioritize small projects that offer early success to demonstrate the value of new technologies and encourage broader adoption throughout the organization.
Collaborating with Universities: Partnering with academic institutions to integrate new technology into manufacturing helps produce a workforce ready to apply cutting-edge solutions immediately.
Pilot Projects for Buy-In: Start with small, focused pilot projects to gain buy-in from older, more experienced workers. This approach helps build trust and eases the transition into new technologies.
Digital Twin and Data Contextualization: Implementing digital twins complemented by real-time data capture improves product design and operational efficiency. Traceability across operations and supply chains becomes essential in refining processes.
AI for Explaining, Not Just Implementing: Using AI to explain and contextualize data, rather than simply implementing solutions, enhances understanding and confidence among the workforce, especially as AI adoption increases .
Sandbox for Predictive Analysis: Create a parallel "sandbox" system to safely explore predictive analytics and test new technologies without affecting core operations, helping small businesses start smart manufacturing with manageable risks.
As-a-Service Mindset: Leasing equipment and technology, rather than owning them outright, offers flexibility and allows manufacturers to stay updated with minimal investment in rapidly evolving technologies.
Sharing Success Stories and Data: Benchmarking success stories and sharing resources across the supply chain fosters collaboration and helps smaller businesses adopt smart manufacturing solutions efficiently.
Generational Change in Technology Adoption: Younger generations are accelerating the adoption of technology in manufacturing, pushing the industry toward faster implementation of smart solutions.
Securing Buy-In from Small Manufacturers: Small manufacturers, representing 95% of the sector in many regions, often struggle to adopt smart manufacturing due to limited resources and lack of internal buy-in beyond leadership roles.
Siloed Organizations: Larger companies are often siloed, with internal competition making it difficult to get on the same page regarding technology adoption. This lack of visibility hampers collaboration.
Dirty Data and Trust Issues: Poor data quality leads to bad decisions, making it crucial to build trust in data accuracy before relying on it for decision-making and new initiatives.
Aging Workforce and Knowledge Retention: As the workforce ages, retaining systemic knowledge becomes a challenge. Organizations must find ways to ensure this expertise is transferred to younger employees to prevent knowledge loss.
Balancing Security and Scalability: Implementing smart manufacturing requires balancing security with scalability and transparency. Cybersecurity and data hygiene must be understood by the entire workforce to protect the company’s digital assets.
Complexity of Digital Twin Implementation: Many companies lack the necessary technology or understanding to effectively build and maintain digital twins. Getting data in one place and ensuring accuracy in digital simulations is a major hurdle.
Cybersecurity Maturity in Smaller Companies: Smaller manufacturers often have less mature cybersecurity systems, making them more vulnerable to digital threats. They need tailored solutions that balance security with their operational needs.
Fear of Obsolescence: Rapid technology changes create fear around software updates and obsolescence, particularly among companies with limited IT resources. This fear hinders adoption of new systems.
Changing Mindset Toward Transparency: Traditionally, manufacturers keep data and processes close to the vest. However, increased collaboration and data sharing within the supply chain is necessary for true smart manufacturing.
People Shortages and Technology Complexity: Labor shortages and the small pool of people capable of understanding and managing smart technologies create obstacles for widespread adoption and effective implementation.
Electrification and mobility are transforming the transportation landscape by promoting cleaner, more efficient alternatives to traditional fossil fuel vehicles. This shift not only reduces greenhouse gas emissions but also enhances energy efficiency and urban air q uality. However, challenges such as charging infrastructure development, battery technology, and regulatory frameworks need to be addressed. Collaboration among manufacturers, governments, and tech innovators is crucial to create a seamless transition to electric mobility. By tackling these obstacles, we can pave the way for a more sustainable and connected transportation future.
“Industry 4.0 and the exponential use of AI will require industry to adapt to address management of workforce, data and IP ownership, data privacy, cybersecurity of systems and machines, and more. In general, industry must embrace the concept of rapid change.
“Mitch Zajac Shareholder
• There’s potential in remanufacturing and recycling supply chain components to support the transition to EV production.
• Fleets of EVs are economically viable and can drive adoption without requiring external incentives.
• Public-private collaboration, such as government and utility partnerships, can foster innovation and infrastructure development for EVs.
Infrastructure Advancements for Widespread EV Adoption:
• Public-private partnerships are crucial to advancing infrastructure, including charging stations, which are key for EV adoption.
• Innovations like bi-directional charging and micro-grids could help manage the growing demand on the electrical grid while creating new business models for EV charging.
• Curbside and home charging infrastructure must evolve to accommodate different use cases and make EVs more accessible.
• Advancements in materials, battery technology, and renewable energy sources like solar power will play a major role in improving EV sustainability.
• Government funding through programs like SBIR and STTR is supporting technical innovators, offering opportunities to increase efficiency and validate new technologies for EV batteries.
• ESG (Environmental, Social, Governance) initiatives provide a framework for companies to ensure the sustainability of their supply chains, offering a competitive advantage.
• The integration of autonomous vehicles in dedicated lanes or geo-fenced areas, such as on campuses or in cities like Phoenix, provides a controlled environment to refine and scale the technology.
• Emerging use cases in industries like agriculture and mining demonstrate the value of autonomous technologies beyond consumer applications.
• Autonomous driving solutions could address generational needs, especially for older populations or individuals who are no longer able to drive, offering improved mental health and independence.
• Managing supply chain uncertainties and the long lead times for new designs, particularly in EV battery production, could take a decade to resolve.
• The U.S. risks falling behind globally, especially compared to regions like China, where EV technology and infrastructure are advancing faster.
• There is a significant talent shortage, especially in key areas like EV battery engineering, electricians, and repair technicians, hindering growth and innovation.
• The lack of standardized charging cables and inconsistent infrastructure across regions creates barriers to widespread EV adoption.
• Cybersecurity concerns related to grid management and EV charging infrastructure pose significant risks, especially with the increasing complexity of bidirectional charging and micro-grid systems.
• There is a shortage of transformers and other critical infrastructure components, which could delay the expansion of EV charging networks.
• The uncertainty around future battery technologies, such as hydrogen fuel cells, remains a challenge as current solutions struggle to meet the growing demand for EVs.
• Questions about where electricity for EVs will come from and how it will be stored highlight ongoing concerns about the sustainability of battery production and energy sourcing.
• The slow pace of autonomous technology integration into everyday mobility landscapes, combined with cost barriers and infrastructure challenges, has tempered expectations for widespread adoption by 2025.
• Climate and geographic challenges, as well as the need for cybersecurity measures, pose significant risks to autonomous driving systems.
• The technology still faces a “testing phase” perception, which delays full consumer trust and acceptance, particularly for long-distance travel and in unpredictable environments.
The global trends in manufacturing are increasingly intertwined with sustainability efforts. While significant opportunities exist to optimize supply chains, adopt new technologies, and improve sustainability metrics, challenges related to cultural shifts, funding, and geopolitical risks remain. By fostering collaboration, leveraging data, and staying adaptable to change, manufacturers can lead the charge toward a more sustainable and resilient future.
“In the U.S. we will eventually adopt something very similar to the European Corporate Sustainability Reporting Directive. This will be used to tax companies for their carbon footprint. Investors will compare companies and invest in those with a strong commitment to sustainability; companies with a proven sustainability program will be the ones that thrive.
““Gill Chan Vertical Market Manager - Factory Automation
Industry 4.0 has the potential to transform manufacturing by optimizing efficiency, improving productivity and quality control, and enhancing supply chain management. By leveraging Artificial Intelligence (AI) coupled with IoT data capture, manufacturers have the opportunity to realize ‘near-zero downtime and near-zero waste’ operations by minimizing scrap and maximizing uptime and energy savings. Industrial AI and Generative AI tools will transform the manufacturing supply chain across all functions beyond the shop floor to sales/marketing, product design, field service, and customer/supplier relationships.
“Mo Abuali Director
Change Management and Organizational
Transparency: Companies must offer full visibility into Industry 4.0 projects across the organization to navigate change effectively. Transparency fosters trust and smoother transitions.
Sustainability Plans are Essential: Developing and implementing a sustainability plan is critical but not as difficult as perceived. A clear policy outlining the reasons behind sustainability efforts helps organizations see the benefits more clearly.
Supply Chain Optimization and Nearshoring: Optimizing supply chains through nearshoring—bringing the supply base closer to home—can reduce risks and ensure more resilient operations.
Designating a Sustainability Champion: Assigning a dedicated person to drive sustainability efforts within an organization ensures focused progress and accountability.
Leveraging Federal Investments: Companies should take advantage of federal government investments in sustainability initiatives, including energy audits and grants, to accelerate their sustainability transformations.
Industry 4.0 Tools and Data Collection: The availability of new technology tools and the importance of robust data collection for Industry 4.0 adoption are paramount for tracking progress and making informed decisions.
Sustainability Scores and Reporting: Sustainability reporting is gaining traction, with scores becoming a key metric for companies to communicate their environmental performance, particularly with investors and stakeholders.
Collaboration with Universities and Unions: Leveraging university expertise for navigating grants and involving unions in discussions about automation can help overcome resistance and cultural barriers related to technology adoption.
Geopolitical Awareness and Resource Management: Companies must consider geopolitical risks, including reliance on rare earth materials from unstable regions, and should evaluate alternatives for sourcing and resource management.
Lightweighting and Remanufacturing: Adopting new materials and remanufacturing processes can reduce cycle times, improve efficiency, and contribute to sustainability goals, such as reducing waste.
Net Zero Cloud and Big Data Platforms: Large corporations are increasingly adopting platforms like Net Zero Cloud, allowing companies to upload and manage sustainability data more efficiently, creating industry-wide benchmarks.
Collaboration Between Suppliers and OEMs: A partnership approach between suppliers and Original Equipment Manufacturers (OEMs) can help solve common sustainability problems and enhance overall operational performance.
Cultural and Generational Shifts: Cultural resistance, particularly generational barriers, remains a challenge for companies embracing new technologies like Industry 4.0. Concerns over job security, particularly among unionized workers, need to be addressed early on.
Funding Justification and Policy Support: Justifying the funding for sustainability initiatives can be difficult, and navigating the complexity of available grants requires both expertise and strategic partnerships. Long-term policies are needed to enable long-term planning.
Energy Audits and State Reporting: Companies should utilize energy audits, often funded by government programs, to assess efficiency. Michigan, for example, is working on offering a toolkit for energy reporting.
Sourcing Rare Earth Materials: The sourcing of rare earth materials is a pressing challenge due to their limited availability and geopolitical risks. Manufacturers must find alternative materials or recycling solutions to mitigate supply disruptions.
Waste Management and Recycling: Handling waste, especially hazardous materials, and finding sustainable recycling solutions remain a significant hurdle for manufacturers striving to improve sustainability.
Transition from Internal Combustion Engines (ICE) to Electric Vehicles (EVs): The shift from ICE to EV production has proven difficult for many manufacturers, requiring new skills, supply chains, and operational models.
Metrics and Measurement Tools: Companies need effective measurement systems, including sensors and data analytics, to track progress on sustainability goals and ensure continuous improvement.
Private vs. Publicly Traded Sustainability Push: Publicly traded companies are often under more pressure to adopt sustainability initiatives due to investor demands, whereas private companies may lag in their commitment.
Geopolitical and Trade Concerns: Geopolitical risks and trade disruptions can jeopardize global supply chains, emphasizing the need for diversified and regional supply networks to mitigate vulnerabilities.
The integration of robotics into the workplace is reshaping job roles and requirements across various industries, enhancing productivity and efficiency. While automation can streamline operations, it also presents challenges related to workforce displacement and the need for new skills. To thrive in this evolving landscape, employees must adapt by acquiring technical competencies and embracing continuous learning. Collaboration between businesses, educatio nal institutions, and policymakers is essential to develop training programs that prepare the workfor ce for these changes.
“I believe that embracing agility and the ability to pivot quickly is critical to keep up with the demands of the changing workforce over the next decade.
“Peter Jones Managing Partner, Wireless Business Sales
“As AI becomes more integrated into the workplace, companies must prioritize human workforce strategies to help employees thrive. This involves fostering a human-centric culture, rethinking work processes and roles, and investing in upskilling and reskilling.
Key roles will emerge, such as AI Trainers and Ethical Evaluators, who will ensure AI systems are unbiased; AI-Enhanced Designers, who will refine AI-generated ideas; and AI-Assisted Decision Makers, who will combine data analysis with human intuition. Additionally, AI-Supported Customer Experience Specialists will manage routine inquiries with AI, while complex issues remain with humans. AI-Integrated Researchers will analyze data and apply human expertise for interpretation.
By identifying skill gaps and offering targeted training, companies can equip employees to collaborate effectively with AI. Rethinking job roles involves recognizing tasks for automation and creating new positions that enhance human-AI collaboration. Ensuring a smooth transition to these roles is essential.
Finally, prioritizing ethical considerations, such as guidelines, bias mitigation, and privacy protection, is crucial for responsible AI use in the workplace.
“Noel Pineda Account Executive
Technological Advancement and Upskilling:
• Companies that invest in upskilling their workforce can retain talent and ensure mission success.
• Real transformation requires not just technology upgrades but also a mindset shift to embrace continuous learning.
• Apprenticeship programs, co-op opportunities, and flexible schedules provide real-world experience, fostering talent development.
Data-Driven Talent and Process Optimization:
• Data is crucial for enhancing both workforce management and robotic systems performance. Creating dashboards and prioritizing performance improvements based on data can drive profitability.
• Collecting and benchmarking data to assess skills and equipment usage over time helps businesses continuously optimize their processes.
Fostering Innovation:
• Using mentorship programs and real-world projects can help foster continuous learning and talent growth.
• Innovative approaches, like simulation for running full systems before implementation, ensure that robotics and other technologies are smoothly integrated.
Adoption of New Technologies:
• Micro-credentialing and hands-on site training with cobots allow staff to adapt to technological advancements.
• Building a culture of learning and embracing creativity can help overcome the challenges of integrating new technologies.
Public and Private Sector Collaboration:
• Initiatives like “shark-tank” style symposiums funded by corporations for universities can drive innovative talent development and workforce preparedness for Industry 4.0.
Workforce Resistance to Change:
• Fear of job loss due to automation is a major barrier to technological integration. Providing situational awareness and clear benefits to employees helps alleviate this fear.
• There's a shortage of skilled trades, programmers, and budget to support ongoing technological transformation.
• Many companies struggle to utilize the data they collect due to a lack of data science expertise, limiting their ability to improve processes or profitability (Dan).
• Smaller businesses may lack the resources or knowledge to collect and leverage data effectively (Noel).
Barriers to Technology Integration:
• Workforce reduction fears persist, even though studies show that robots increase efficiency rather than replacing jobs.
• Cybersecurity remains a concern as businesses integrate more robotics and connected technologies.
Supply Chain and Equipment Challenges:
• Supply chain disruptions and availability of machines can hinder the timely integration of new technologies.
• Providing shop floor workers with the time to train and work with new technologies is a persistent challenge.
Skills Gap and Workforce Shortage:
• The shortage of workers with the necessary skills to operate advanced technologies presents a significant barrier to workforce development and robotics integration.
• Contract staffing and external experts may serve as short-term solutions but don’t address the long-term need for skilled talent.
Automation Alley is a nonprofit technology business association and Digital Transformation Insight Center focused on driving the growth and success of businesses in Michigan and beyond through innovation and automation. With a global outlook and a regional focus, we foster a vibrant community of innovators, entrepreneurs, and business leaders through opportunities for collaboration and learning. Our programs and services help businesses develop the skills and expertise needed to effectively jumpstart or accelerate digital transformation. By bringing together industry, academia, and government, we aim to create a dynamic ecosystem that drives innovation and growth across Michigan.
At Automation Alley, our mission is to help businesses thrive in the rapidly changing digital economy. We equip them with the knowledge, insights, and tools to develop a software-first mindset that leverages the power of automation, AI, and other cognitive technologies. We believe that by working together, we can build a stronger, more innovative, and more competitive economy for the future.
Wealth, prosperity and equality through technology.
January
February
May
May
March
April
August
August
August
September
Made possible in part through ongoing support from the