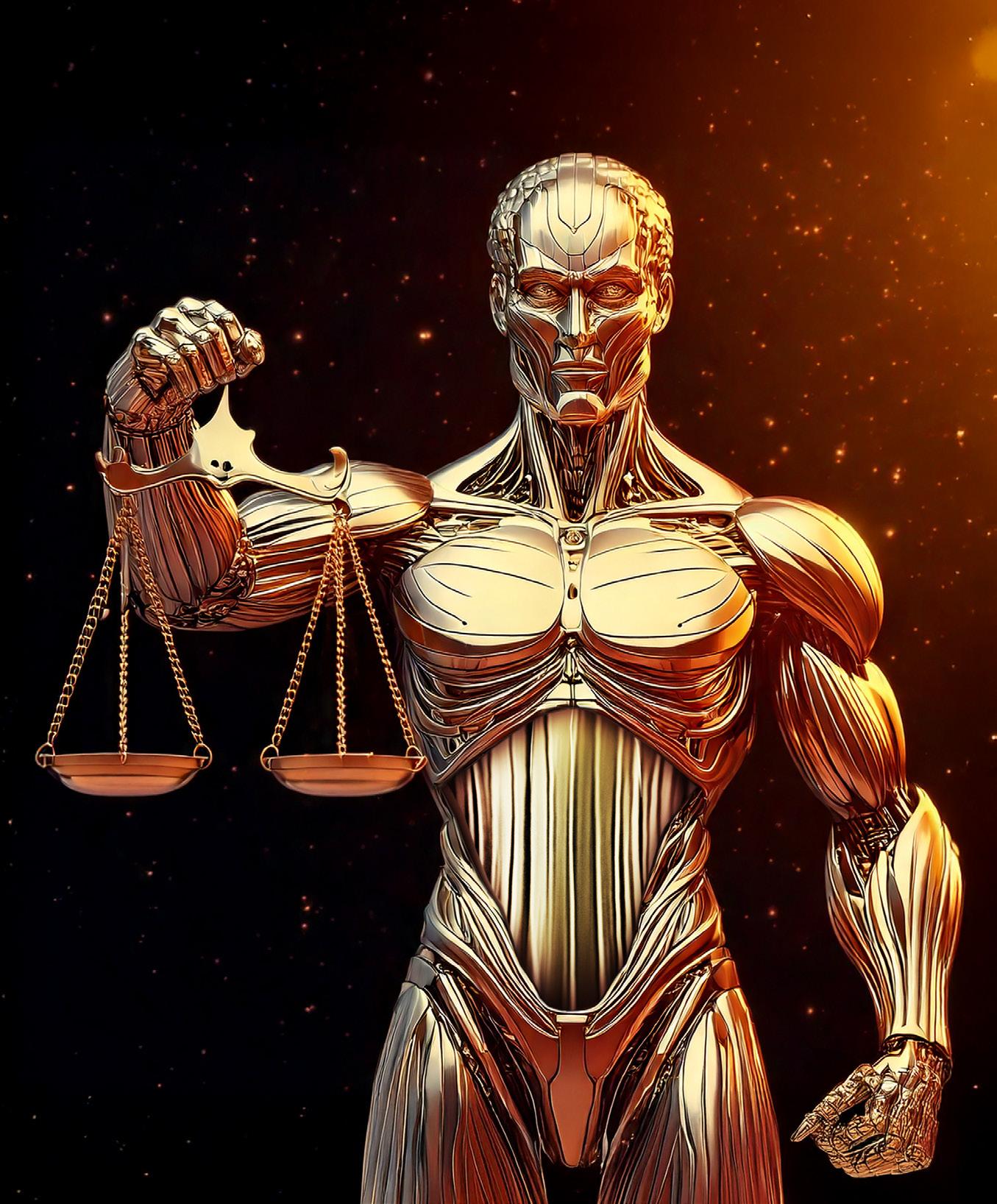
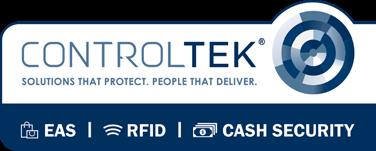
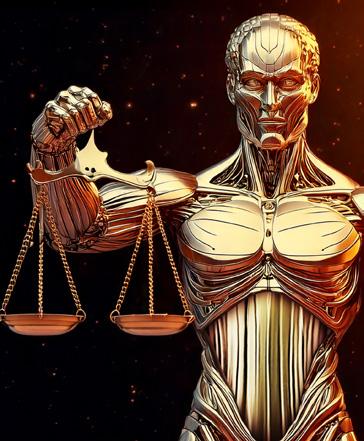
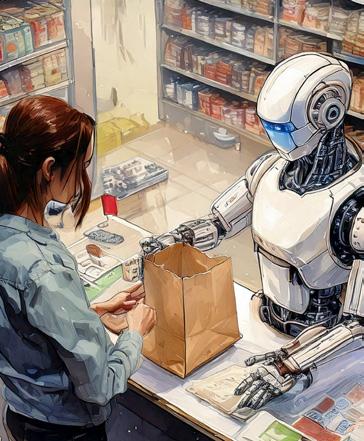
By Tom Meehan, CFI
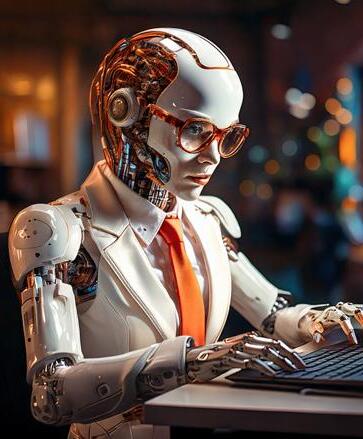
By Courtney Wolfe
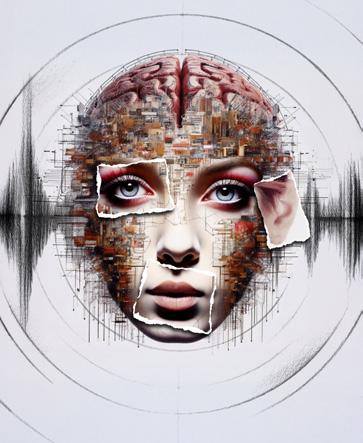
By Caleb Bowyer
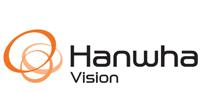
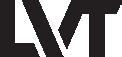
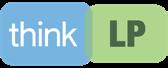
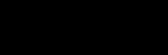
By Sam Boykin
By Tom Meehan, CFI
By Courtney Wolfe
By Caleb Bowyer
By Sam Boykin
LVT provides a rapidly deployable enterprise-grade safety and security ecosystem that can eliminate blind spots and protect any physical environment.
THE LVT DIFFERENCE
RAPIDLY DEPLOYABLE Our solar-powered, cellular-connected solution can be up and running in a matter of minutes.
SECURE WITH LVT Your data never hits a public network. All customer data is protected with AES-256 encryption at rest and TLS 1.2 during transit.
AUTOMATED DETERRENCE Keep bad actors on their toes with industry-leading AI-generated audio deterrents that refer to an individual’s clothes and attributes without human intervention.
in shoplifiting
trespassing
in burglaries and break-ins
disorderly conduct
Andrews, CFI Vice President, Loss Prevention, VF Corporation
Rick Beardsley Senior Director of Loss Prevention and Safety, At Home Group
Michael Limauro, LPC Vice President, Global Asset Protection, Whole Foods Market
David Lund, MBA, LPC, CFI Executive Director, International Association of Interviewers
Cloud Group Senior Vice President, Loss Prevention, Ross Stores
Dan Moren Senior Manager, Starbucks
Peck, LPC Senior Vice President, Loss Prevention, The TJX Companies
Global Protection
LPM's Editorial Board is composed of some of the loss prevention and asset protection industry's top executives from a wide range of retail sectors. These senior executives provide guidance to the LPM team on article topics and industry issues that are of current concern and interest to LP professionals. To learn more about the Editorial Board, contact Stefanie Hoover, CFI, at StefanieH@LossPreventionMedia.com.
Rhett Asher SVP, Community Relations and Partnerships
Bobby Haskins SVP, Customer
James Stark Segment Manager, Retail
Stephen B. Longo VP, Strategic Initiatives
Stuart Rosenthal VP, Global Sales
Tom Meehan, CFI CEO
Chris Reene Head of Commercial
Craig Greenberg Chief Commercial Officer
Vy Hoang Chief Customer Officer
Dave Sandoval President
Alix Arguelles Director of Product
Jack Ashton VP, Strategic Development
Amit Kumar Founder/CEO
VP, Retail Sales
Scott Thomas National Director for Signature Brands
I.
Sr. Director, Vertical & Enterprise Business Development
Cita Doyle, LPQ, LPC VP, Sales and Marketing
Matt Kelley, MBA Head of Retail, Go To Market
Kevin O'Brien EVP of Business Development
McCauley Director, Sales
Robb Northrup Director, Marketing Communications
Tony Sheppard, MSM, CFI, LPC VP, Retail Risk Solutions
Scott Pethuyne Sr. Analytics Solution Consultant
LPM's Solution Partner Board is composed of the magazine's strongest solution provider supporters. These executives provide their counsel on how the magazine can better advance and serve the loss prevention and asset protection industry. To learn more contact Ben Skidmore at Ben@PartnersPR.com.
By Tom Meehan, CFI
One of the potentially most important technological developments in modern criminal justice is happening today—the emergence of artificial intelligence.
As AI systems become ever more capable and powerful, their greatest impact may be felt in the most basic criminal justice application—the investigation process itself. Used correctly, AI can help security and law enforcement solve more crimes and do so faster than ever before. Used incorrectly, it can lead to egregious privacy invasions and a multitude of ethical problems.
Today, investigators often have an overwhelming sea of digital evidence to sort through. They face a nearly ubiquitous social media presence, image and video recordings of private moments lived in public, and the public email accounts of individuals. Those emails often contain a mind-numbing volume of private business, from conversations with an ex-spouse about child custody arrangements to a person’s effort to repair his or her credit—and a myriad of other irrelevant matters. On top of all this “evidence,”
there are all the smart devices that fill our homes and businesses, which are extraordinarily geolocative and surveillance-optimized. Against this backdrop, AI offers one of the most attractive benefits for investigators—an unprecedented ability to analyze colossal amounts of data rapidly and precisely. This capability is realized in a number of different areas:
1. Enhanced Data Analysis and Pattern Recognition
AI is vastly superior to the human mind for analyzing not only what is private (and probably should stay that way), but also who is private (and probably should retain some privacy)—in addition to when, where, and why
AI is rapidly becoming a key part of the investigator’s toolkit for making sense of the huge amounts of digital evidence that the human mind alone cannot comprehend. For example, advanced AI with machine learning capabilities can be used to analyze:
● Video evidence from surveillance cameras
● Evidence found in public email accounts
● Human communication—in all its private and not-so-private forms
● Human behavior
● Human presence in public and private spaces
2. Improved Evidence Processing
AI technologies have transformed the processing and analyzing of evidence, dramatically changing the investigative landscape. Systems using computer vision, for instance, can take poor-quality surveillance footage and make it possible to identify details necessary for an investigation that would otherwise be very difficult to see. License plates, actual suspicious activities, and other distinguishing features are now much easier to find in video evidence.
One application of machine learning is natural language processing (NLP), which has emerged as a powerful tool for examining the texts and spoken words that comprise our communications. This technology’s capability is nothing short of amazing; computers can pick out keywords, discern sentiment or context, and understand the who/what/where/when of it all.
The common link between these various technologies, whether computer vision, machine vision, or even a simple microphone, is that they all require high-quality inputs to be useful. Humans need clear images to accurately interpret video footage; they need good audio to understand sounds. That requirement has not changed with the new technology. In fact, with AI and machine learning, the need is heightened, because while AI operates at a much faster pace than humans, it isn’t exercising human judgment or intelligence. A
few decades ago, law enforcement officials seeking to identify a suspect would typically have to comb through a book of Polaroid photos, inspecting each image with their eyes for a potential match. Today, AI and computer vision can perform the same task almost instantly. But even with the added speed and efficiency of these powerful new tools, the basic process of picking a person’s face out of a lineup remains unchanged.
Used correctly, AI can help security and law enforcement solve more crimes and do so faster than ever before. Used incorrectly, it can lead to egregious privacy invasions and a multitude of ethical problems.
Predictive policing algorithms have shown some potential for helping police forces allocate resources more effectively (this capability has also ignited controversy, as we’ll discuss). These systems take in a number of different types of data—historical crime data as well as demographic and environmental information. They then analyze this veritable treasure trove of data, and, using sophisticated AI, discern not just the kinds of crimes being committed and the ways and means of committing them, but also the times and places they’re most likely to occur.
In the domain of interviews and interrogations, AI provides sophisticated tools for:
● Analyzing voice stress to pinpoint possible signs of deception
● Detecting facial micro-expressions during questioning for more profound emotional insights
● Employing NLP to unpack inconsistencies or oddities in statements
● Watching for behavioral patterns during long interviews that might be clues to truth or deception
While these use cases certainly demonstrate AI’s prowess, they’re also a lively testament to the fact that behavior isn’t a good predictor of truth or deception. Indeed, several studies indicate that we’re not nearly as good at reading human behavior as we might think, and some researchers have even gone so far as to say that we’re not good at it at all. When we construct models, they are only as useful as the data they are trained on. If that data is filled with creativity, subjectivity, or biases, then the model based
Justice or Jeopardy?
on it will have the same characteristics. The operative principle here could be phrased as “good data in, good model out.”
Many studies support the use of behavioral analysis. However, many others argue against using it in models. This creates a substantial challenge for those conducting investigations on the ground: they must navigate between two scientific perspectives while accounting for bias. As I frequently underscore in my writings, people are naturally biased, and the models they create learn from data generated by people—so they too, are biased. Models can be made to learn in a way that does not result in biased predictions but requires a training set that is, as much as possible, free of bias.
AI offers one of the most attractive benefits for investigators—an unprecedented ability to rapidly and precisely analyze colossal amounts of data.
1. Privacy and Civil Liberties Concerns
When power like AI is harnessed in public sector criminal investigations, it inevitably affects citizens’ privacy. This technology’s ability to concentrate on truly vast amounts of data—both personal and non-personal—makes it potentially invaluable for solving crimes. But could it also be unconstitutional in the ways it gathers, stores, and uses people’s data? This remains an open question. In the area of surveillance, there are concerns aplenty. Here are a few of the most asked questions:
● How far can law enforcement go in amassing and keeping our data?
● Can we trust authorities not to use our data in bad ways we can’t even fathom yet?
● What is the correct balance between the many benefits of AI and our security?
● What about the chilling effect on free speech that innocent individuals may suffer?
2. Bias and Discrimination
One of the most serious dangers tied to AI in law enforcement is algorithmic bias. AI learns from historical data, which often mirrors our existing societal biases and discriminatory practices. If biases seem to come from thin air when we decide certain
groups of people are “at-risk” and certain areas of the country are “high crime,” just think how much worse it would be for those decisions to be made by an algorithm working from that flawed data. Then imagine that algorithm working in real-time, identifying certain individuals as being at-risk or certain neighborhoods as high crime. Is that fair? Is it not like using a magic eight ball to decide who deserves to be treated with dignity by our justice system? These tools, which are imbued with bias, could promote inequity, and that is unjust.
3. Reliability and Accuracy Concerns
Despite the great speed at which AI systems can process the massive amounts of data they are now fed, doubts persist about their reliability and accuracy when it comes to rendering life-and-death decisions. Here are some of the most pressing concerns:
● AI can generate false positives. For example, individuals might be incorrectly identified by facial recognition systems, which could lead to wrongful arrests and accusations.
● AI can misread context. AI lacks what humans have in abundance—an understanding of nuanced cultural and situational elements essential to human judgment. When we misinterpret the context of a situation, we’re likely to miss the important elements and get it wrong. That risk is much greater with AI.
● AI can be overly relied on. AI’s predictions might be too trusted by human investigators and not scrutinized adequately, leading to more mistakes.
4. Legal and Ethical Implications
Many have expressed concern about the intricate legal and ethical issues associated with the use of AI in criminal investigations. They worry about several issues:
● The acceptability of AI-produced evidence in court
● The potential of AI to affect inquiries and still maintain due process rights
● The difficulty most people may have in understanding AI
● The potential of AI to make interrogation more effective and thus more “unfair” to defendants
I’ve looked at some of the ways AI is changing criminal justice processes. Here are some more specifics:
1. AI in Interrogation Rooms
Using AI in interrogations offers exciting possibilities but also poses some tough
challenges. AI might help law enforcement study human behavior and figure out when people are lying. But there are also concerns regarding:
● How reliable and accurate AI-based deception detection will prove to be
● The potential for AI to be used to coerce or unduly influence a suspect
● Whether AI might run afoul of suspect rights and protections
● The mental health impact on those being interrogated when AI is used in the process
2. Digital Evidence Analysis
The practice of using AI to analyze digital evidence has grown in importance. Its uses now include:
● Automated processing of electronic devices
● The recovery of deleted or hidden data
● The analysis of online activities and digital footprints
● Recognizing patterns across multiple digital sources
However, as with other applications, these uses of AI also raise concerns about:
● Chain of custody for digital evidence
● Verification of evidence processed by AI
● The significance and usefulness of evidence produced by AI
● Preservation of individual privacy rights
1. Policy Framework
To minimize these risks while maximizing the many benefits of AI, police departments should:
● Create detailed AI governance policies
● Clear up the AI usage ambiguity in existing legal guidelines
● Develop stringent oversight structures
● Be as transparent as possible without compromising public safety
● Issue regular checks for AI deployment “health” and “safety”
● Update policies and guidelines as necessary
2. Training and Education
The best policies and practices are useless if people aren’t trained to implement them. So, it is essential to train law enforcement personnel to:
● Grasp the abilities and shortcomings of AI
● Identify potential errors and biases
● Correctly analyze and comprehend the insights produced by AI
● Uphold the human judgment necessary for decision-making
● Reconcile the use of this newfangled technology with the traditional toolbox
3. Technical Considerations
Organizations putting AI systems into action should:
● Regularly test and validate their AI systems
● Maintain proper documentation of AI processes
● Ensure security of the systems and protection of the data
When power like AI is harnessed in public sector criminal investigations, it inevitably affects citizens’ privacy.
algorithms, enhanced real-time monitoring, and more advanced data analysis tools.
● Have backup systems and contingency plans in place
● Perform regular updates and maintenance of AI systems
4. Ethical Guidelines
Here are some areas ethical guidelines should focus on and address clearly:
● Safeguarding individual rights
● Ensuring fairness and non-discrimination
● Achieving transparency and accountability
● Protecting privacy
● Ensuring human oversight and control
The full capabilities of AI are just beginning to be explored. As this technology continues to advance, we can expect to see more capable behavioral analysis, better predictive
Looking ahead to implementation, agencies must determine how to mesh AI with their existing systems. They will face demands for training and adaptation, as well as financial and human resource issues. Finally, and importantly, they will need to gain the public’s acceptance and trust.
Integrating AI into criminal investigations, interviews, and interrogations is both an opportunity and a challenge for law enforcement. The possible payoffs in efficiency, accuracy, and crime prevention are large; so, too, are the risks to privacy, civil liberties, and fairness. Finding success here will require a careful balance, much like a teeter-totter, between potential benefits and risks. On one end are the potential payoffs—the promise of making things more efficient and, in some cases, more
accurate; the potential of using it to help sort through the masses of information that law enforcement agencies generate and that we, as a society, generate in our daily lives; and, on top of that, the potential of using it for the good old-fashioned crime prevention that we tend to associate with our police forces. On the other end there are the risks. It seems clear that the risks to privacy, civil liberties, and fairness are substantial.
Harnessing and using this technology effectively but judiciously will be the challenge facing law enforcement and security professionals for the foreseeable future.
Tom Meehan, CFI, is the retail technology editor for LP Magazine as well as CEO of CONTROLTEK. Previously, Meehan was director of technology and investigations with Bloomingdale’s, where he was responsible for physical security, internal investigations, systems, and data analytics. He currently serves as the chair of the Loss Prevention Research Council’s (LPRC) Innovations Working Group. Meehan is the published author of his book titled Evolution of Retail Asset Protection: Protecting Your Profit in a Digital Age. He can be reached at TomM@LossPreventionMedia.com.
By Sam Boykin
While Whole Foods Market has had much success with its community-based approach to asset protection, the company is incorporating a complementary new strategy. To further reduce shrink and increase ROI, the Austin, Texas-based grocer recently started using artificial intelligence technologies, which are poised to fundamentally change how the loss prevention industry operates.
When Michael Limauro, LPC, took the helm as vice president of global asset protection at Whole Foods Market in 2021, he was facing a particularly volatile retail environment, as the country was reeling from the COVID-19 pandemic, political upheaval, and widespread crime.
To help address these challenges, he partnered with local organizations and individuals to reduce crime and increase profitability. But Limauro wanted to introduce multiple layers of asset protection strategies, so to help spearhead the AI initiative, he called on Michael Canu, an asset protection data and information technology expert.
At the time, Canu was working in the field, but his role shifted during the pandemic, and he started developing asset protection AI technologies, or, more specifically, machine-learning models.
Canu stresses that AI—especially with the rise of ChatGPT—has become a somewhat misleading marketing term that people associate with dystopian movies like The Terminator Machine-learning is a technique that enables computers to learn from data and improve their performance over time, while the concept behind AI is that machines mimic human intelligence.
“People think AI is taking information and coming to its own conclusions,” he said. “But ChatGPT is just a machine-learning algorithm. And that’s what 99 percent of business leaders and retail consumers interact with.”
Canu’s efforts at Whole Foods contributed to Entitlement, a machine-learning program that “essentially provides ROI on our theft events,” he said.
Implemented in January 2024, the Entitlement model bolsters
“Instead of having to sift through 40,000 different event types, the system is doing all that for you, and it pushes out the information you need. The fact that Whole Foods Market has adopted this shows its real value.”
Michael Canu
Whole Foods’ case management capabilities, feeding the system a rapid stream of data points that can be quickly interpreted. Compiled information includes whether a theft event was in-store or external, if there was an apprehension or prevention, whether it was an individual or group, and what was stolen and from what department.
All these different data points are combined, which determines the ROI and helps forecast the costs and gains involved with these different theft events. Known theft events are mainly tracked for case linking and case building. If there is an incident of theft prevention, all of that data feeds into Entitlement based on the type of event.
Much more sophisticated than past methods of determining scope or stop-loss, AI has helped streamline Whole Foods’ case building. “Once we close out a case or stop a shoplifter, the magic of the machine-learning algorithm comes in,” Canu said. “It calculates ROI in a multitude of different ways and projects what the total entitlement will be over X number of days and years.”
Canu said he and his team can splice up the data sets and compare factors like retail departments and merchandise types. The technology can also forecast theft events, including potential locations and at-risk products.
Even with all these innovations, Canu stresses the important role humans play in the process. “All the data is
eventually pushed to an AP person,” he said. “It still requires human interaction and a closed human feedback loop inside our network.”
Moreover, Entitlement helps save Canu’s team’s time, energy, and effort. He recently updated the Entitlement app, which enables employees to log theft events rapidly and easily using a handheld device.
“This gives us a lot more data, and it’s quicker than the old process of using a back-of-house computer,” Canu said. “Instead of having to sift through 40,000 different event types, the system is doing all that for you, and it pushes out the information you need. The fact that Whole Foods Market has adopted this shows its real value.”
Some companies, however, are still weighing their options when it comes to AI. Brian Friedman, director of asset protection and risk management at REI, said the company is taking baby steps when it comes to adopting new AI technologies.
For case management, Friedman said the company works with the digital security provider Auror, which uses AI to help detect emerging threats and adapt. REI is also working on a customer self-serve chat project using AI, but there hasn’t been any widespread adoption beyond that. He believes that is likely to change as technology and the marketplace evolve.
“We’re an experiential company from a merchandising perspective, and there are ways we’re going to use AI to interact with customers and provide online support,” he said. “It’s going to be a huge unlock for us to gain insight quickly and put things together for case management.”
Canu said there’s likely to be rapid AI adoption over the next five to ten years, and the technology will be necessary not just for loss prevention and security, but for retailers to stay competitive. But like all technological innovations, it will come with growing pains.
“I think AI is going to sit behind a little bit of everything at some point,”
Canu said. “There’s no getting rid of AI. It’s only how and where you lean into it. But the different systems will be siloed, and we’re going to find ourselves in a pickle when we realize none of these things are talking to each other. That’s one of the big AI problems we’re going to have to address.”
Amit Kumar is working on solving some of these problems. He founded Dragonfruit AI in 2019 and serves as the company’s CEO. The company offers AI-powered video data and other applications for LP solutions, like security alerts, retail analytics, foot traffic mapping, and intrusion detection. When it comes to the current landscape of loss prevention and AI adoption, Kumar said the most common scenario is when a retailer has an existing product or solution that is made better with AI. For example, AI-powered burglar alarms help alleviate false alarms, while AI-enabled video can
“We’re being careful to ensure that the new module has human-led, opt-in functionality. AI is still a taboo subject in some circles. While retailers are excited about the possibility of AI, they also have some concerns, especially around privacy issues and evolving legalities. In retail, you’ve got to get approval and justify why you need certain data and information.”
Tony Sheppard
do a deeper analysis of specific areas, like detecting if there’s snow or other potential hazards around a business so it can be cleaned to prevent accidents and insurance claims.
“That kind of adoption is a no-brainer,” Kumar said.
Other adoption scenarios involve new capabilities that are only possible through AI. As opposed to the laborious process of watching hours of video, AI monitoring, for example, helps prevent theft at self-checkout kiosks by using cameras, sensors, and machine-learning to analyze data and detect suspicious activity.
AI technologies are also helping create full replacement loss prevention services. With exception-based reporting that feeds into case management, you can use AI to analyze thousands of transactions quickly and efficiently and add new capabilities like voice recognition.
“None of these are examples of some crazy AI technology,” Kumar said. “Those are not getting adopted right now. If you go to a retailer and say you have an AI tool for them, they’re going to show you the door. Instead, they’re looking for cheaper, faster solutions to help solve their problems.”
Kumar said he’s seen a big change in people’s attitude toward AI over the last few years.
“People almost saw AI as black magic. Fortunately, that has changed as AI has become widespread and accessible with things like ChatGPT and Google searches. We’re seeing more pragmatic applications of AI. But retail is always careful and slow with the deployment of new capabilities.”
Like Dragonfruit AI, ThinkLP is also rolling out a new AI-powered resource for loss prevention professionals. The company, a loss and safety intelligence platform, is releasing an enhanced software module in April at the Retail Industry Leaders Association Asset Protection Conference in Washington, D.C.
Tony Sheppard is vice president of risk solutions at ThinkLP. He said AI powers some of the company’s case management and incident management offerings, as well as
its exception-based reporting and analytics software. “AI integration has been gradual, but it’s certainly accelerated over the past six months,” he said.
ThinkLP’s new enhancement improves the company’s complex investigations module. Currently, the module is geared toward organized retail crime cases and enables users to collect evidence, connect incidents, and aggregate theft information, creating a case report that can be sent to law enforcement. The new version has more robust crime-linking functionality, including some capabilities powered by AI.
“You’re trying to gather as much information about an incident in the shortest amount of time possible,” he said. “AI can take information from the reports, pull out key data, and match the information to other reports.”
Sheppard stressed that while the system may indicate a suspect link or match, a human still has to confirm or reject the assertion.
“We’re being careful to ensure that the new module has human-led, opt-in functionality,” he said. “AI is still a taboo subject in some circles. While retailers are excited about the possibility of AI, they also have some concerns, especially around privacy issues and evolving legalities. In retail, you’ve got to get approval and justify why you need certain data and information.”
Bobby Haskins is senior vice president, customer - North America, for Auror. The company bills itself as a crime intelligence platform that offers tools to help reduce theft by collecting and merging detailed information on variables like suspects, locations, and items stolen.
“We use data that we collect to create a network effect not only within a single organization but with everyone using the platform inside the industry,” said Haskins, who added that about 45,000 retail locations in the US use Auror. In the past year or so, AI has become an increasingly critical part of Auror’s platform, but there are still limitations.
“It’s gotten a lot smarter, and there are a lot of benefits, but it’s not a silver
bullet,” he said. “It’s a tool. And if you don’t have quality intelligence and structured data and inputs, then AI is just reviewing garbage. And garbage in is garbage out.”
Haskins points to some of Auror’s unique capabilities, like how the app employs language processing, so you can use your voice to report a theft event and easily add details like physical description and location.
The platform can also use physical descriptions and image matching to create profiles, helping identify suspects and instantly sharing the information with other users.
“Now a store manager in Ohio can track if a suspect that’s in his store is the same person who stole from a location in California,” Haskins said. “AI is helping connect the dots. It makes it easier to aggregate data and identify the people causing the most loss.”
However, Haskins said that AI does not automatically make these links and
connections. It presents the information so a human who’s been trained can approve or deny a possible match.
“That’s really important in this space because there’s a lot of misunderstanding that AI is going to turn into The Minority Report,” he said. “I think AI is going to have a huge impact on the loss prevention industry. But that’s with the caveat that we need strong privacy frameworks and laws around what we should or shouldn’t do with this technology, because it is very powerful.”
Originally from North Carolina, Sam Boykin is a San Antonio-based writer who has written for a number of regional and national publications, including Men’s Journal, Outside, and USA Today He previously served as editor-in-chief for the San Antonio Business Journal and managing editor for the Sacramento Business Journal
“People almost saw AI as black magic. Fortunately, that has changed as AI has become widespread and accessible with things like ChatGPT and Google searches. We’re seeing more pragmatic applications of AI. But retail is always careful and slow with the deployment of new capabilities.”
—Amit Kumar
Focusing only on theft means missing most of your losses. Retailers often overlook other critical areas impacting profitability. Transforming loss prevention into a profit powerhouse requires a comprehensive approach that automates processes and maximizes profit opportunities across the retail landscape.
Focusing solely on loss prevention is limiting. Expanding to broader profitability measures can uncover significant opportunities. For example, Walgreens used predictive analytics to identify loss anomalies. Walgreens implemented Zebra’s Actionable Intelligence, saving millions, reducing waste rates by 27 percent, and flagging missed credit opportunities. The algorithm analyzed each store’s waste-to-sales ratio, alerting managers to audit compliance with markdown policies, leading to significant waste reductions.
Siloed data can hide valuable opportunities. Using machine learning to improve loss detection reveals hidden profitability drivers. Demonstrating rising profit margins helps retailers implement Total Retail Loss (TRL) strategies and showcase the value of loss prevention. Operational execution is key to minimizing retail loss. Actionable Intelligence assigns tasks to frontline staff and notifies managers upon completion, ensuring high operational efficiency and reducing shrinkage risks.
The scalability and flexibility of Actionable Intelligence make it an ideal solution for retailers of all sizes. As businesses grow and evolve, the tool adapts to changing needs, ensuring it remains
relevant and useful. Technology acts as a force multiplier, allowing smaller teams to handle more tasks efficiently. By automating routine tasks, employees can focus on core responsibilities while technology manages broader focus areas. This enhances productivity and brings in other stakeholders, supporting collective goals and driving success.
Fostering a data-driven culture is essential. Democratizing data access enables employees to make informed decisions, leading to proactive problem-solving and better performance.
Transforming loss prevention into a profit powerhouse requires a strategic approach and thinking outside a singular job function. By automating processes, uncovering hidden opportunities, and enhancing operational execution, retailers can achieve significant cost savings and drive profitability. Embracing solutions like Actionable Intelligence optimizes every aspect of the retail enterprise for sustained success. To learn more, visit zebra.com.
By Courtney Wolfe
Stopping loss at the checkout is one of the greatest challenges retailers face today. And while self-checkout kiosks are easy targets to blame for the bulk of this loss, manned checkouts also present plenty of opportunities for theft.
“Shoppers are learning the constraints of the checkout process, and then they can exploit the process
and technical shortcomings to their advantage,” said Zebra Technologies Senior Solution Manager Andy Doorty.
“Retailers are struggling with losses at the checkout due to a mix of challenges—employees giving unauthorized discounts, customers skipping scans at the self-checkout, and fraud slipping through unnoticed,” added i3 International
Chief Customer Officer Vy Hoang.
“With fewer staff on the floor and rising organized retail crime, it’s becoming harder to catch mistakes and intentional theft before they impact the bottom line.”
Thankfully, recent advances in artificial intelligence are proving to be a game-changer in helping retailers stop loss at the checkout.
“Shoppers are learning the constraints of the checkout process, and then they can exploit the process and technical shortcomings to their advantage.” Andy Doorty
Retailers are in a sticky position—not only must they work to stop loss at the checkout, but they also have to keep the customer experience top-of-mind so consumers continue patronizing their stores.
“Checkout loss management is a balancing game between reducing loss while providing the best customer experience possible—the more friction you introduce, the unhappier your customers become,” said Dragonfruit AI Cofounder and CEO Amit Kumar. “So, any efforts to reduce loss must be balanced by the amount of friction they cause in the transaction and provide a clear ROI.”
Finding that balance is much easier said than done.
“Retailers are trying to find the right balance of convenience, speed, and cost,” said Zebra Global Retail Technology Strategist Matthew Guiste. “Self-checkout improves some costs, like lower labor, but can increase costs, like loss.”
Scan avoidance, ticket switching, and faking the payment process are all ways in which theft can occur at the self-checkout. But, with consumers growing more comfortable with the self-checkout experience, Guiste says
that it is imperative for retailers to figure out how to close down these theft opportunities.
“As retail continues to evolve, checkout losses will likely become even more complex with the rise of AI-driven fraud, more sophisticated organized retail crime, and increased reliance on self-checkouts,” Hoang added. “Retailers will need smarter solutions that go beyond traditional loss prevention methods.”
Internal theft can also occur at the checkout.
“A foundational piece of retail businesses is the employees,” said Solink Senior Leader of Asset Protection Cathy Langley, LPC. “As most of us already know, high turnover rates of store employees, including cashiers and assistant store managers, create more potential for cashier thefts, discount abuse, fraudulent transactions, and more. The core challenge for retailers here is how to retain employees or train and monitor new hires quickly.”
Langley added that retailers also face the challenge of auditing their loss and being able to provide evidence of that loss: “With separate data and video systems, or camera infrastructure that is not properly positioned to collect shopper insights in the self-checkout area, retailers will find it challenging to pinpoint where loss is happening and understand effective solutions. When pilot programs for potential solutions are conducted, retailers will also face the difficulty of proving the ROI because they won’t have the tools at hand to accurately track and monitor checkout area success.”
For years, companies have been promising artificial intelligence would help solve all our LP problems—and we’ve been
left waiting. But now, the technology has finally caught up, and it truly can make a huge impact on retailers’ bottom lines.
“Until very recently, there had really been very few solutions for helping retailers manage checkout loss,” Kumar said. “With today’s video AI technology, there are now new approaches with a much lower total cost of ownership that enable retailers to tackle these challenges. These new approaches can help retailers detect and deter loss at SCO as well as for staffed lanes and even across the entire store.”
Langley agrees, saying that AI has already started to transform how retailers look at loss.
“The retail industry has already witnessed the shift from reactionary measures like reviewing video streams or POS data to track theft post-event, to preventive measures like auditing stores in real-time to ensure that the staff are actually at the till when customers are present,” Langley said. “AI has been a huge help with driving this shift, and it will be even more useful when encouraging retailers to now take on predictive measures rather than relying on preventive measures.”
Looking at how AI can help with specific challenges at the checkout, Doorty says that AI tools can determine if an item’s barcode truly belongs to the product being purchased, stopping ticket switching. This way, a shopper’s behavior can be detected and identify suspicious behavior. Once suspicious behavior is detected, an automatic alert can be sent to the SCO attendant.
Doorty added that AI can also detect and identify suspicious behavior related to scan avoidance and other checkout scams, and using UHF RFID as a sensory input for the AI engine to sense the actual product being moved through the checkout area can strengthen your defense even further.
Though cost is of course a valid concern for retailers when it comes to investing in this technology, there are ways to make implementation more cost-effective. Hoang suggests a “try and buy” approach.
“By integrating AI-powered loss prevention into existing infrastructure, retailers won’t need to invest in expensive,
“Retailers are struggling with losses at the checkout due to a mix of challenges—employees giving unauthorized discounts, customers skipping scans at the self-checkout, and fraud slipping through unnoticed. With fewer staff on the floor and rising organized retail crime, it’s becoming harder to catch mistakes and intentional theft before they impact the bottom line.” Vy Hoang
“The retail industry has already witnessed the shift from reactionary measures like reviewing video streams or POS data to track theft post-event, to preventive measures like auditing stores in real-time to ensure that the staff are actually at the till when customers are present. AI has been a huge help with driving this shift, and it will be even more useful when encouraging retailers to now take on predictive measures rather than relying on preventive measures.” Cathy Langley, LPC
fragmented solutions,” Hoang explained. “This approach ensures that protecting profits doesn’t come at the cost of efficiency, scalability, or affordability.
AI-powered loss prevention isn’t just a theory; it’s a proven, data-driven approach that can be tested in your own store with minimal risk.”
Other advice our sources offered for retailers regarding AI included:
● Evaluate your brand promise and specific needs. AI checkout solutions are not one-size-fits-all, and different segments and brands will have different needs and goals.
● Focus on ROI. Take an expansive view of the costs at the checkout, including things like square footage and operational costs, peak seasons, etc., to figure out the right mix of staffed, self, fixed, and mobile checkouts.
● Consider customer needs. Make sure your customers are ready for digital experiences and lean into them carefully. It’s a journey.
● Work with your IT department to build the infrastructure and backend when implementing AI in-store.
● Partner with other executives so that they will support you when it comes time for budgeting.
● Understand how you can collect, aggregate, deliver, and respond to the data. AI cameras and sensors track customer interactions, self-checkout behavior, engagement levels, and transaction data, ensuring accurate insights. All this information is typically processed in a centralized platform, consolidating video analytics, transaction monitoring, and AI-driven alerts into a single, easy-to-use system. This can all help ensure employees engage at the right moments, preventing fraudulent scans, and even predicting high-risk situations before they happen.
While AI is already making a massive impact on the retail industry, it’s clear that its influence will only continue to grow.
“AI will evolve to not just react to theft at the checkout, but to predict and prevent it before it happens,” Hoang said. “Over time, as more data is collected and consumed by the AI engine on customer behavior, transaction anomalies, engagement levels, and self-checkout patterns, predictive modeling is being applied to the solution. This means retailers will be able to anticipate potential risks before they occur, for example, knowing when and where self-checkout fraud is most likely to happen based on past patterns. AI can then alert staff ahead of time, adjust security measures dynamically, or even automate responses, such as requiring manual approval for high-risk transactions. By integrating real-time AI insights with predictive analytics, retailers won’t just reduce loss—they will be able to fine-tune store operations, improve customer flow,
“AI products will get more accurate, faster, and be able to solve more and more edge cases. Fraud techniques will grow in sophistication, so the tools to mitigate them will also evolve.” Matthew Guiste
and enhance overall efficiency, making loss prevention both proactive and cost-effective.”
And as criminals become more clever in their fraud techniques, AI will also continue to improve.
“AI products will get more accurate, faster, and be able to solve more and more cases,” Guiste said. “Fraud techniques will grow in sophistication, so the tools to mitigate them will also evolve.”
Langley adds that while store managers are wary of new tools because they might seem like just another thing to manage, AI is well worth the effort. For those hesitant, she recommends starting with a pilot program and implementing AI in a few high-risk stores to assess its impact before scaling.
“AI is not just a tool for loss prevention—it’s a way to future-proof retail operations,” she explains. “As retail technology advances, AI will become a standard part of smart checkout solutions, improving security while enhancing the customer experience. An important consideration is to always look for solutions that will scale as your company grows. Look for solutions that are easy to deploy at a low cost but are high quality. These will be solutions that offer a high degree of security, flexibility for building into existing data or camera systems, low long-term maintenance costs, and strong customer support to ensure smooth deployment.”
Courtney Wolfe
Wolfe is LPM’s managing editor focusing on creating and curating editorial content for the magazine’s print publication and website. Prior to LPM, she was managing editor for SDM Magazine, a trade publication for security systems integrators. She received her bachelor’s degree in multimedia journalism from Columbia College Chicago. She can be reached at CourtneyW@LossPreventionMedia.com.
The LP Magazine website and digital channels offer loss prevention and retail professionals a myriad of thought leadership articles from a wide range of industry experts, original articles from LPM writers, webinars, podcasts, whitepapers, and much more. There is new content updated to the website daily that is featured in our e-newsletter. To ensure that you don’t miss this important information, subscribe to our digital channel at losspreventionmedia.com/email/ or scan the QR code on the left.
To ensure that you don’t miss any important loss prevention information, subscribe to our digital channel by scanning the QR code above.
Watch this webinar now on-demand for a look at the impact of AI on retail crime, investigations, and interviewing featuring Professor Michael Townsley, WZ’s David Thompson, and CONTROLTEK’s Tom Meehan. Retail crime is evolving fast, and AI is not only impacting the way we analyze and investigate theft and fraud, but it is also enabling the fraudsters, changing the game for LP professionals, and bringing new threats as well as powerful new solutions.
Retailers must address checkout challenges and the growing problem of shrink, much of which occurs during the checkout process. What if AI could turn these challenges into an opportunity, improving not just checkout, but the entire customer experience? For forward-thinking retailers, it’s doing just that and proving to be a gamechanger.
AI is much more than ChatGPT! In this episode of LPM’s Inside Scoop Podcast, we hear about applications to help LP (and other retail teams) become more efficient and solve problems quickly. Caleb Popow and Scott Pethuyne chat with Stefanie Hoover about what Zebra is working on in the AI space, challenges of adopting AI in day-to-day practice, and more.
As retailers grow more comfortable with generative AI, there are uses to explore that can specifically address shrink and the rise of ORC. Some potential uses include providing loss prevention specialists with faster analysis of retail crime cases and helping to create stronger case files. The more retailers invest in the technology, the more they will be able to innovate within their loss prevention strategies and protect their overall profits.
Imagine a retail store that can see and understand what’s happening around it—as clearly as a store associate—and respond intelligently in real-time. That is the promise of Vision-Language Models (VLMs). VLMs are “multi-modal” models because they can simultaneously analyze video and language data to summarize what is happening at a location in real-time.
VLMs are an exciting area of artificial intelligence that combines computer vision with natural language processing (NLP) to interpret images and videos in context. Computer vision is almost self-explanatory—it is when computers use video or other image data
By Caleb Bowyer
to “see” the world. Computer vision has been the talk of the LP industry for years because of the opportunities it can create in threat detection, investigations, and many other aspects of LP.
However, VLMs are exciting because they extend the utility of computer vision. In LP, computer vision models have been used primarily to detect and report the presence of objects and basic activities in video. VLMs layer in NLP to provide more in-depth information from visual data. NLP is a field of artificial intelligence that helps computers understand, interpret, and respond to human language. Therefore, when NLP is applied to the
output from computer vision models, computers can synthesize much richer descriptions of a scenario or activity, and its context.
To understand what VLMs are, one should first understand image captioning. Image captioning is the process of converting an input image into a text description of the image. This process is shown below; if you have ever created an image using generative AI, it is essentially the process in reverse.
For retailers focused on LP, VLMs represent a groundbreaking opportunity to enhance security, detect an array of suspicious behaviors from visitors, and
potentially make smarter LP decisions. However, VLMs have many other retail applications beyond LP. But, before we get too far, let’s break VLMs down further.
VLMs are AI systems that combine two key capabilities:
1. Vision Understanding: The ability to analyze images or videos, identifying objects, people, actions, or behaviors
2. Language Understanding: The ability to understand and generate text, including labels or descriptions of what the model is seeing, as in the case of image captioning systems.
Together, these models can look at an image or video and describe what’s happening—almost like a person would. For instance, a VLM might look at security footage and say, “A person wearing a hoodie is concealing an item in the electronics aisle.” A software program could then be written to flag the model’s concerning outputs, e.g., descriptions associated with various kinds of theft: cart pushout, concealment, etc.
records footage, VLMs can interpret what they see. They don’t just capture footage—they can recognize unusual behavior and alert store associates based on predefined prompts or questions. Unsurprisingly, this is called Visual Question Answering (VQA). For example, you might ask a VLM, “Is anyone walking out of the store carrying merchandise that is not in a bag?” and it can provide an answer.
2. Reducing False Alarms: By understanding the context of a situation through a sufficiently descriptive prompt or question, VLMs can reduce the number of false positives, like mistaking a big sales event that draws a crowd as a flash rob event, for example.
VLMs connect visual and language data. This creates incredibly powerful opportunities to protect people, places, and property and also adds to the overall value proposition of retailers’ camera systems and the video data they create.
This also means VLMs could be used to detect violent incidents or tools associated with them, such as guns or other blunt metal objects.
Traditionally, LP programs have relied on a variety of technologies, processes, human vigilance, and human expertise to prevent as well as investigate theft, fraud, or violence. Cameras, whether analog or IP, have long played an important role in LP. Unfortunately, as LPRC research shows, video review is one of the most time-consuming and inefficient aspects of the investigator’s job. Furthermore, humans have limited abilities to effectively analyze the amount of video that retail stores collect at any given time. VLMs operating on camera video streams take things to the next level by:
1. Providing Contextual Understanding: Unlike traditional cameras with a video management system (VMS) that simply
3. Supporting Decision-Making: VLMs don’t just detect problems; they describe them, helping LP teams decide how to respond once alerted to any detected and described event class of interest. In other words, they can play an important role in understanding a business problem before blindly throwing solutions at it.
Let’s explore some of the most exciting ways VLMs could be used to enhance other LP innovations:
1. Detecting Concealment Shoplifting Behaviors or Other Criminal Warning Indicators
VLMs can analyze live video feeds to identify actions like:
● Concealing items in bags, pockets, or under clothing.
● Tampering with price tags, products, or security devices.
● Suspicious hiding of identity through masks, hoodies, wearing sunglasses indoors, or bandanas.
Example: A VLM sees a person repeatedly looking over their shoulder while handling a product. It flags this behavior as unusual, and alerts store associates to check in.
2. Monitoring Self-Checkout Fraud
Self-checkouts are convenient but prone to fraud, such as scanning lower-priced items instead of the real product. VLMs can:
● Detect mismatches between scanned barcodes and the items being bagged, but, more importantly, they can also describe which mismatches occurred and potentially how the mismatch happened.
● Identify attempts to skip scanning altogether.
Example: A customer scans a water bottle but places an electronic gadget in the bag. A system connected to the VLM alerts an associate before the customer leaves.
3. Enhancing Crowd Monitoring
VLMs can monitor crowd behaviors to prevent flash robs or violent incidents by:
● Detecting large groups of people entering together with their faces obscured.
● Recognizing signs of agitation or escalating tensions such as crowds running.
Example: A group of individuals wearing hoodies and masks runs through the front entrance. The VLM flags this as a potential flash rob scenario and contacts store security or law enforcement.
Many different retail divisions, departments, and teams use video; many of these uses relate to some threat, risk, or liability or use video to mitigate these. However, VLMs make it possible for retailers to efficiently use video for many other purposes.
This is probably the most exciting aspect of VLMs—the data that retailers spend a lot of money to acquire is becoming much, much more valuable, and the retailers that succeed tomorrow will be the ones who quickly understand and leverage this valuable data. More important, readers—the LP leaders who will
make a name for themselves tomorrow are the ones who are thinking about how they can help their business partners achieve their goals today. Therefore, it is important to think about how technologies apply to the business generally while building their business cases for technology.
For example, VLMs could be used to identify and alert store employees when:
● An elderly customer is having trouble removing an item from a shelf—a potential safety incident.
● It could be used to alert managers that a case or shelf has not been stocked prior to opening—an opportunity to avoid missed sales.
● A person has spent time removing, inspecting, and replacing multiple items from a shelf—an opportunity to help a customer with product selection.
The point is that VLMs connect visual and language data. This creates incredibly powerful opportunities to protect people, places, and property and also adds to the overall value proposition of retailers’ camera systems and the video data they create.
VLMs are an exciting area of artificial intelligence that combines computer vision with natural language processing (NLP) to interpret images and videos in context.
It is still very early days for VLMs. As VLMs evolve, they will become indispensable tools for retail security teams, offering smarter, faster, and more proactive ways to combat theft, fraud, or violence while improving overall store operations. VLMs represent cutting-edge AI innovation in LP. By combining the ability to see with the ability to understand language, VLMs empower retailers to detect, describe, and respond to security threats with unprecedented accuracy and efficiency. As these models continue to advance, they promise to reduce losses and create safer, smarter, and more secure shopping environments.
how
best train cooperative autonomous agents from noisy and partially observed data for decision making tasks, primarily focusing on localization applications. He is expected to defend and graduate with his PhD in summer 2025. At the LPRC, he is head of the LPRC’s DETECT research initiative and facilitator of the Data Analytics Working Group (DAWG) that meets monthly. He researches, develops, and implements AI solutions related to detecting theft, fraud, or violence attempts—earlier and further away from retail stores. His goal at the LPRC is to integrate all of the sensors and AI models across the five zones of influence into a production ready system for better alerting of offenders in real-time and detecting repeat offenders on their journey to commit harm and then deflecting that harm.
SOLUTIONS SHOWCASE
Upuntil recently, retail security plans have been a game of reaction. An alarm sounded, a camera recorded, and a guard reviewed the footage—usually long after the threat had passed, when it was too late to change the outcome or achieve any form of justice.
But in recent years, security has shifted from reactive to proactive Smarter surveillance, real-time alerts, and AI-driven analytics now help security teams stay ahead of threats. These advancements have been game changers, cost cutters, and, in many cases, life savers.
Generative AI agents are poised to take that shift even further, transforming how businesses and communities safeguard their assets, people, and operations.
Agentic AI—AI that operates autonomously, making decisions and taking actions without constant human oversight—is a step beyond traditional AI, which relies on manual prompts or pre-programmed instructions. Instead,
Agentic AI can “think.” It can: Identify patterns and threats, responding with appropriate countermeasures
y Make decisions based on historical and contextual data
y Learn from interactions to improve future decision-making
Traditional security systems rely on cameras, alarms, and motion detectors to detect threats, but these solutions often fall short of preventing incidents entirely. LVT’s solutions have already changed that, using strobe lights, loudspeakers, and a commanding presence to deter crime before it happens. Agentic AI takes deterrence from reactive to strategic.
Rather than responding the same way to every potential threat, generative AI agents analyze situations, adapt, and react appropriately. This might look like:
y Triggering the right deterrents at the right time, whether that’s a verbal warning, flashing lights, or escalating to human intervention
y Reducing “alert overwhelm” by differentiating harmless movements from genuine threats
y Making deterrence feel more personal—because when an intruder hears a warning directed specifically at them, they’re more likely to back down
Yet, even as AI becomes more advanced, human oversight remains critical. As Steve Lindsey, CTO/CIO of LVT, puts it: “The human is still part of this puzzle, and we shouldn’t think that the human is being completely displaced by AI. We’re trying to make humans more efficient.”
Agentic AI isn’t about replacing security professionals—it’s about empowering them. By automating routine decisions and enhancing threat detection, these systems free up human operators to focus on what they do best: making informed, strategic security decisions that keep people and property safe.
To learn more, go to lvt.com.
Kevin Darnell Business Development Manager, Retail Hanwha Vision America
Considering all the attention and coverage given to AI in recent years, it would be fair to assume that by now, everyone is an expert. However, that’s still not the case in most industries, and retail is no exception, especially when it comes to AI surveillance.
The past few years have seen companies throughout the security and surveillance industry expand their use of AI, with some rapidly adopting the technology and others dipping their toes in the water. AI has certainly advanced from an emerging technology into a proven reality, with the ability to improve security camera imaging performance, enhance the accuracy of people and object detection, reduce false alarms, and conserve recording and network bandwidth.
Retail is evolving faster than ever, and as someone who has spent years on the retail side, leading both operations and loss prevention, I’ve seen firsthand what retailers need in a video security system.
Retail is evolving faster than ever, and as someone who has spent years on the retail side, leading both operations and loss prevention, I’ve seen firsthand what retailers need in a video security system.
Retailers now demand much more from their video systems than simple surveillance. Today’s video solutions must address life safety, operational efficiency, customer experience, and, of course, security—and AI can tie all four together.
However, that’s often easier said than done.
AI has been a top retail buzzword for several years. Retailers know AI is something they need, but many have limited capital dollars for technology investments. Plus, there is still much confusion about what AI is and what it can and cannot do.
The influx of data can be overwhelming. Every new product is labeled “AI.” I even have a vacuum cleaner that claims to use AI, but it’s really LIDAR remote control technology. Customers often ask, “Can AI identify a gun?” Some technologies can, but then the next question might be, “Can AI identify a gun that someone’s concealing?” or “Can AI tell me if somebody’s planning to steal?” There’s nothing out there like that, at least not yet.
Many retailers are adopting AI in a piecemeal fashion, often focusing on one specific use case rather than taking a more holistic approach to integrate it across different business functions effectively. Within one retail organization, the loss prevention team may adopt AI for its own needs, and customer service may use AI for an entirely separate task.
Success lies in busting those silos and getting everybody to collaborate on overarching AI deployments covering life safety, operational efficiency, customer experience, and security. The operator that uses AI most effectively is one that is using it across all four areas.
Workplace safety is a top priority for retailers. Hanwha provides AI solutions to help identify life safety issues, such as forklift safety violations and blocked fire exits or electrical panels. Additionally, Hanwha’s AI capabilities can detect and alert/report slip-and-fall incidents as soon as they happen, allowing for immediate response and documentation. By proactively addressing workplace hazards, retailers can enhance employee safety and reduce liability risks.
AI video helps optimize retail operations. Heat mapping and dwell-time analytics provide insights into customer traffic patterns, allowing stores to adjust layouts and staffing to maximize efficiency. AI can also monitor safety compliance, ensuring that emergency exits remain unblocked, employees follow protocols, and store conditions meet operational standards. Retailers often operate across multiple locations, making scalability and remote monitoring essential. Whether you have an on-prem appliance or cloud-based video, AI solutions allow centralized management of all stores, warehouses, and offices from a single platform. Managers can access real-time footage, review analytics, and respond to alerts instantly—no matter where they are. AI-driven search
capabilities make reviewing footage faster and more efficient, saving valuable time.
Retailers depend on multiple systems, from POS and inventory management to workforce scheduling. The most effective AI video solutions integrate seamlessly with these existing platforms to deliver comprehensive insights. For instance, combining AI-driven video analytics with POS data can identify fraudulent transactions, voids, and sweethearting, while integrating with inventory systems enhances the tracking of high-value merchandise.
Whether it’s operational, internal, or external, shrink will always be a top concern for retailers. AI-driven video analytics now provide proactive loss prevention by identifying suspicious behavior in real-time, alerting staff before an incident escalates. With advanced object recognition and analytics, AI video can detect patterns, unauthorized access, and internal fraud, minimizing losses before they happen.
Retail success hinges on the customer experience. AI-powered cameras can analyze checkout queues, automatically deploying more staff when lines become too long. A seamless customer experience is vital for retail success. AI-powered cameras optimize store performance by using customer counting data to determine conversion rates and key staffing areas. By analyzing foot traffic patterns, retailers can ensure the right number of staff are deployed in the most critical
Whether it’s operational, internal, or external, shrink will always be a top concern for retailers. AI-driven video analytics now provide proactive loss prevention by identifying suspicious behavior in real-time, alerting staff before an incident escalates.
areas of the store, improving service efficiency and boosting sales opportunities.
The AI-powered analytics in cameras can provide valuable insights into customer traffic patterns and shopping behaviors if they are used properly and effectively. However, retailers must always look ahead and think about new AI applications that can streamline store operations.
From maximizing your capital investments to proving measurable value, working with a trusted partner like Hanwha will demonstrate how AI video solutions reduce losses, optimize staffing, and improve inventory management—ensuring a retail system is future-proof to deliver a strong long-term return on investment.
By leveraging Hanwha’s advanced AI video technology, retailers can stay ahead of emerging threats, streamline operations, and enhance their customers’ experience, all while maintaining a secure environment.
Hanwha is collaborating with industry-leading third-party solutions, for example, through its Partnership Program, continuing to expand API integrations and ensuring that capital investments evolve into intelligent, data-driven assets.
Retail store employees already have enough to manage without having to react to every possible incident detected. To help reduce the pressure and stress levels of store employees, Hanwha Vision has combined AI with many of its IP surveillance technologies. Combining AI into video surveillance systems addresses this heightened need for more accurate AI-based detection of people, objects, and vehicles, leading to fewer false alarms and more effective and accurate forensic searches. Hanwha also offers IP-based audio systems that can link to a store’s video surveillance system to broadcast either real-time or pre-recorded messages to address suspicious or criminal activities preemptively. For example, the surveillance system can detect if a person is loitering in a restricted area and issue an audio warning to move.
Hanwha delivers technology that directly addresses the needs of today’s retailers. From distribution centers to storefronts, our solutions provide security, efficiency, and valuable business intelligence. Hanwha has dedicated teams designing and developing cutting-edge camera solutions and AI specifically for retail, making a global impact across all verticals, from distribution centers and home offices to big-box stores and specialty retailers.
Retail is changing, and AI-driven video systems are no longer just a security measure—they are a critical component of a retailer’s success. Learn more at hanwhavisionamerica.com.
Combining AI into video surveillance systems addresses this heightened need for more accurate AI-based detection of people, objects, and vehicles, leading to fewer false alarms and more effective and accurate forensic searches.
Many retailers rely on EAS today, but retail is evolving. Our systems evolve with you, seamlessly integrating cutting-edge technologies like computer vision, RFID, smart sensors, and LiDAR.
Start with EAS and seamlessly upgrade when you’re ready to unlock a smarter, data-rich environment with actionable insights.
Protect your investment, extend the life of your EAS systems, and stay ahead of retail’s evolution.