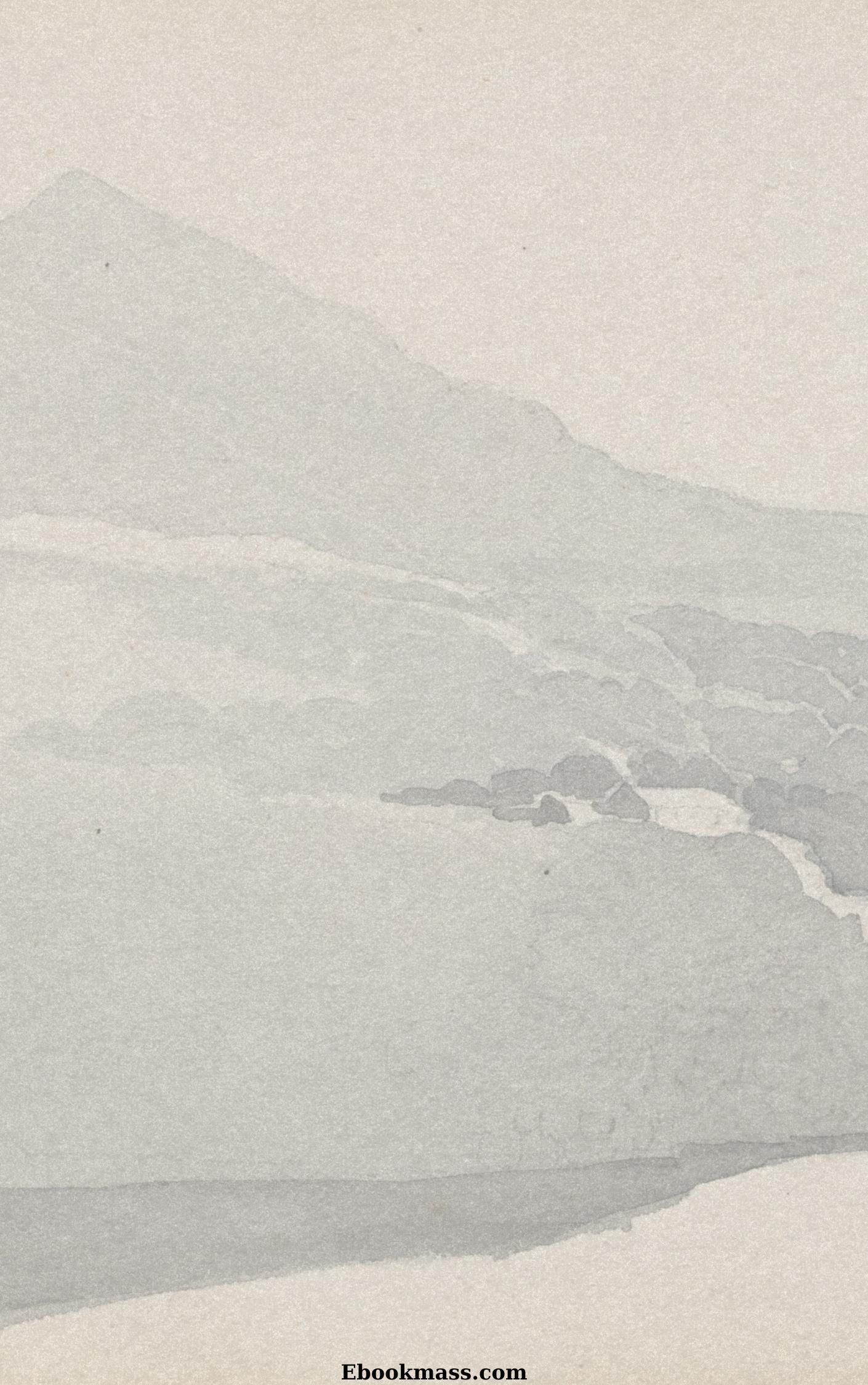
https://ebookmass.com/product/artificial-intelligence-and-
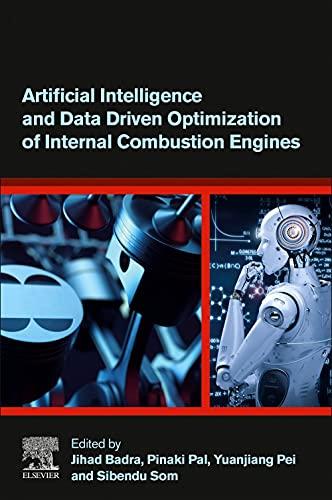
Instant digital products (PDF, ePub, MOBI) ready for you
Download now and discover formats that fit your needs...
Computational and Data-Driven Chemistry Using Artificial Intelligence: Fundamentals, Methods and Applications
Takashiro Akitsu
https://ebookmass.com/product/computational-and-data-driven-chemistryusing-artificial-intelligence-fundamentals-methods-and-applicationstakashiro-akitsu/ ebookmass.com
Artificial Intelligence and Credit Risk: The Use of Alternative Data and Methods in Internal Credit Rating
Rossella
Locatelli
https://ebookmass.com/product/artificial-intelligence-and-credit-riskthe-use-of-alternative-data-and-methods-in-internal-credit-ratingrossella-locatelli/
ebookmass.com
NOx Emission Control Technologies in Stationary and Automotive Internal Combustion Engines: Approaches Toward NOx Free Automobiles B. Ashok
https://ebookmass.com/product/nox-emission-control-technologies-instationary-and-automotive-internal-combustion-engines-approachestoward-nox-free-automobiles-b-ashok/ ebookmass.com
The Body in the Dumb River George Bellairs
https://ebookmass.com/product/the-body-in-the-dumb-river-georgebellairs/
ebookmass.com
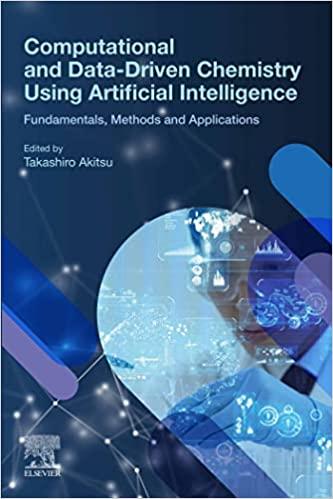
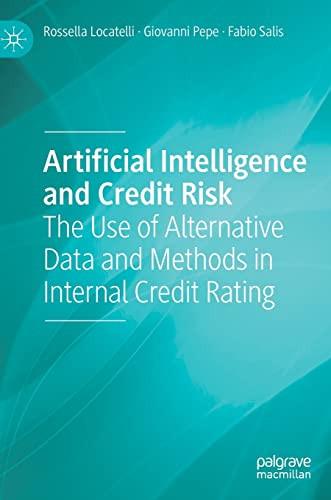
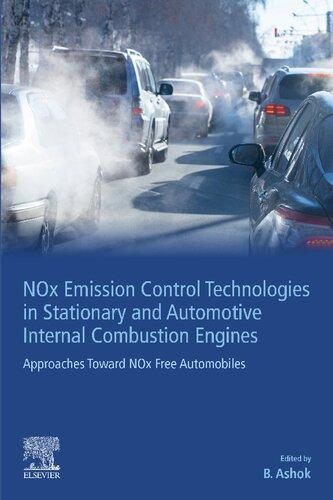
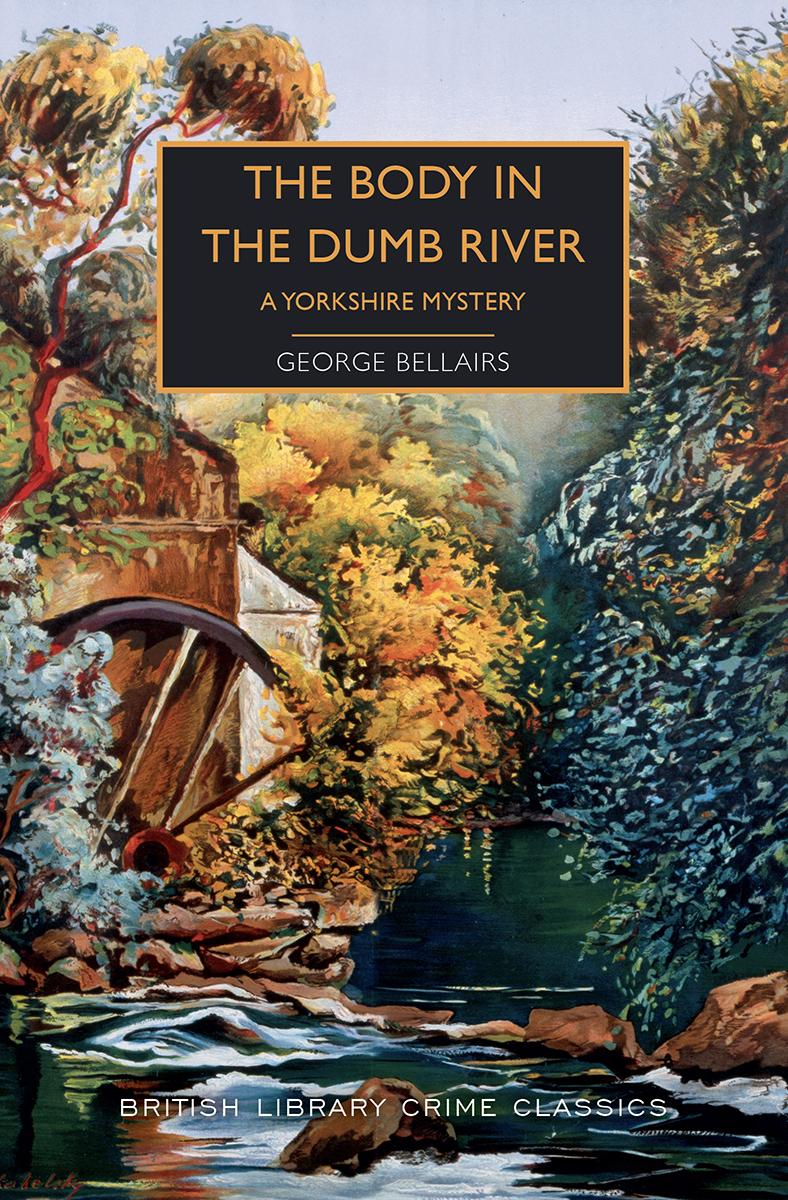
School and Society: Historical and Contemporary Perspectives 7th Edition, (Ebook PDF)
https://ebookmass.com/product/school-and-society-historical-andcontemporary-perspectives-7th-edition-ebook-pdf/
ebookmass.com
Auctioned Surrender: A Dark Mafia Romance (Sinful Duets Book 2) Mari
https://ebookmass.com/product/auctioned-surrender-a-dark-mafiaromance-sinful-duets-book-2-mari/
ebookmass.com
Saving Finley: Military Hero, Heroine Romance (Safe and Secure Book 8) Alyssa Bailey
https://ebookmass.com/product/saving-finley-military-hero-heroineromance-safe-and-secure-book-8-alyssa-bailey/
ebookmass.com
Human Evolution: Bones, Cultures, and Genes John H. Langdon
https://ebookmass.com/product/human-evolution-bones-cultures-andgenes-john-h-langdon/
ebookmass.com
Come Dance With Me: Elladine Fire Steamy Romance Series Finley Daniels
https://ebookmass.com/product/come-dance-with-me-elladine-fire-steamyromance-series-finley-daniels/
ebookmass.com
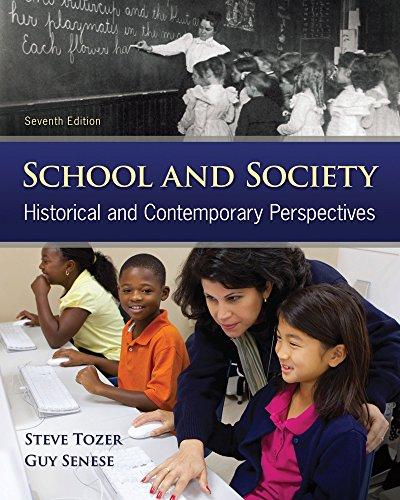
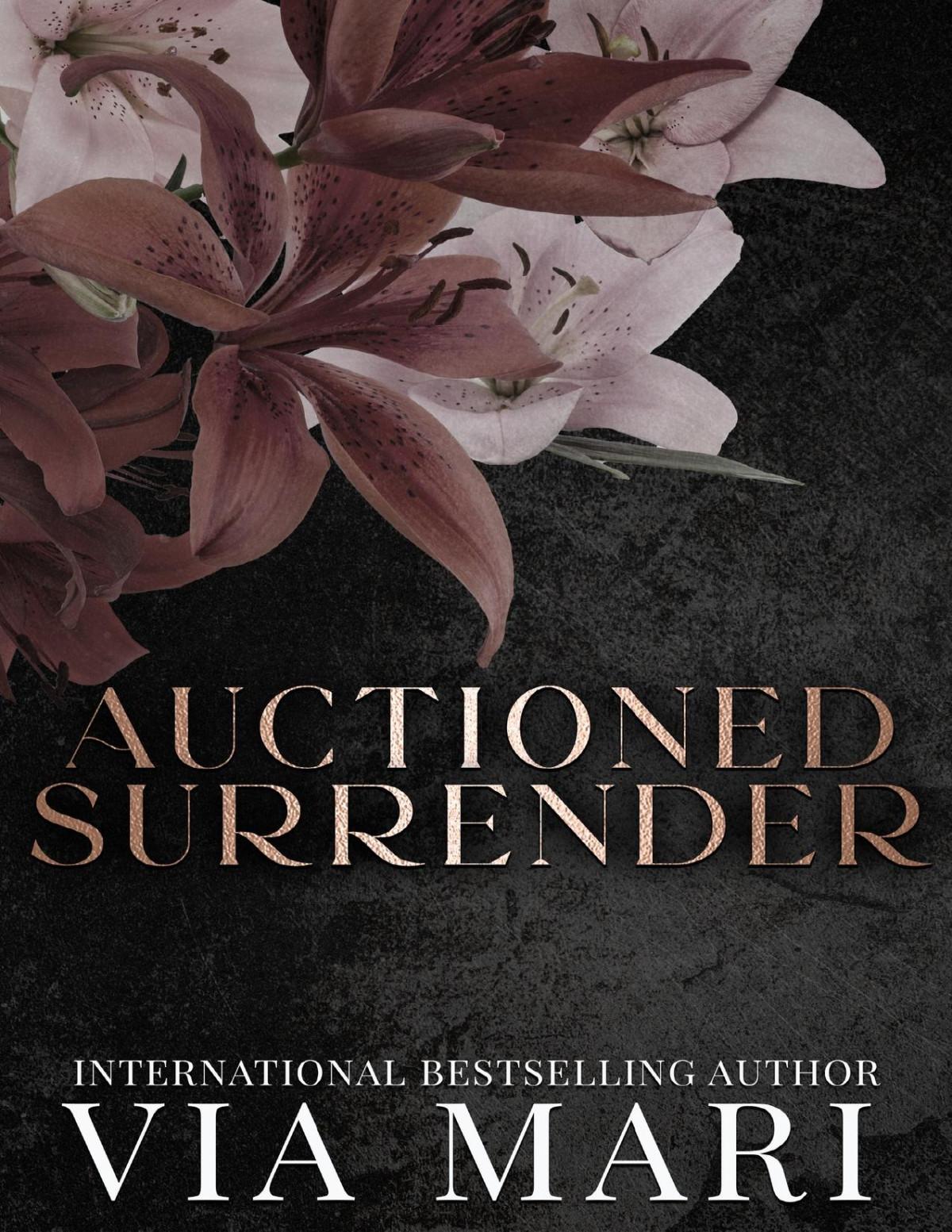
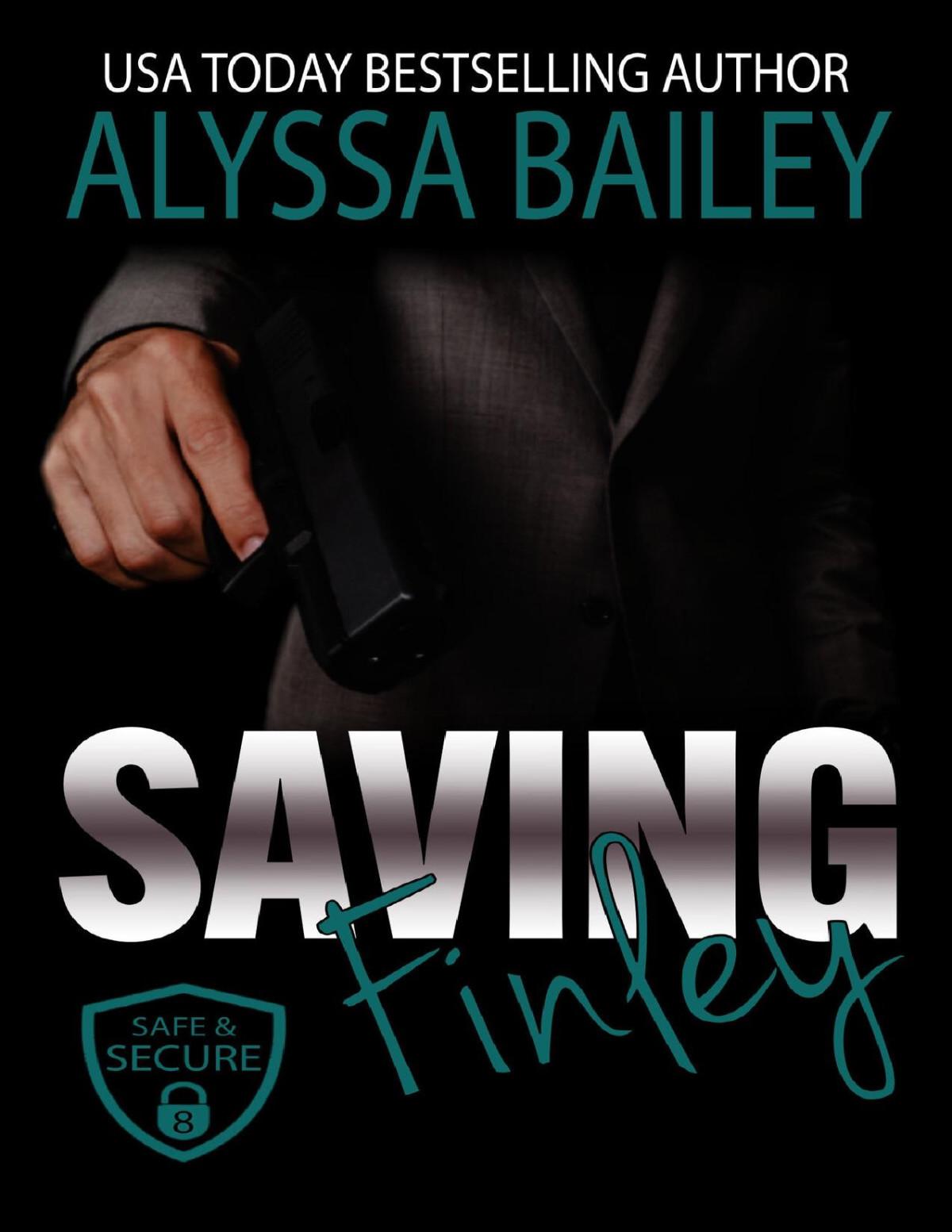
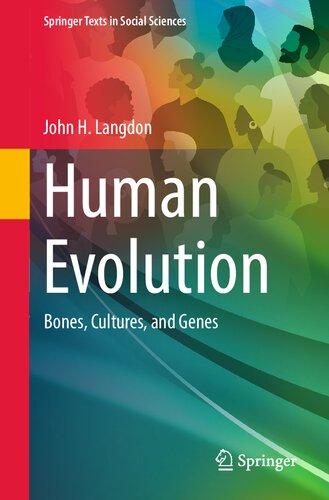
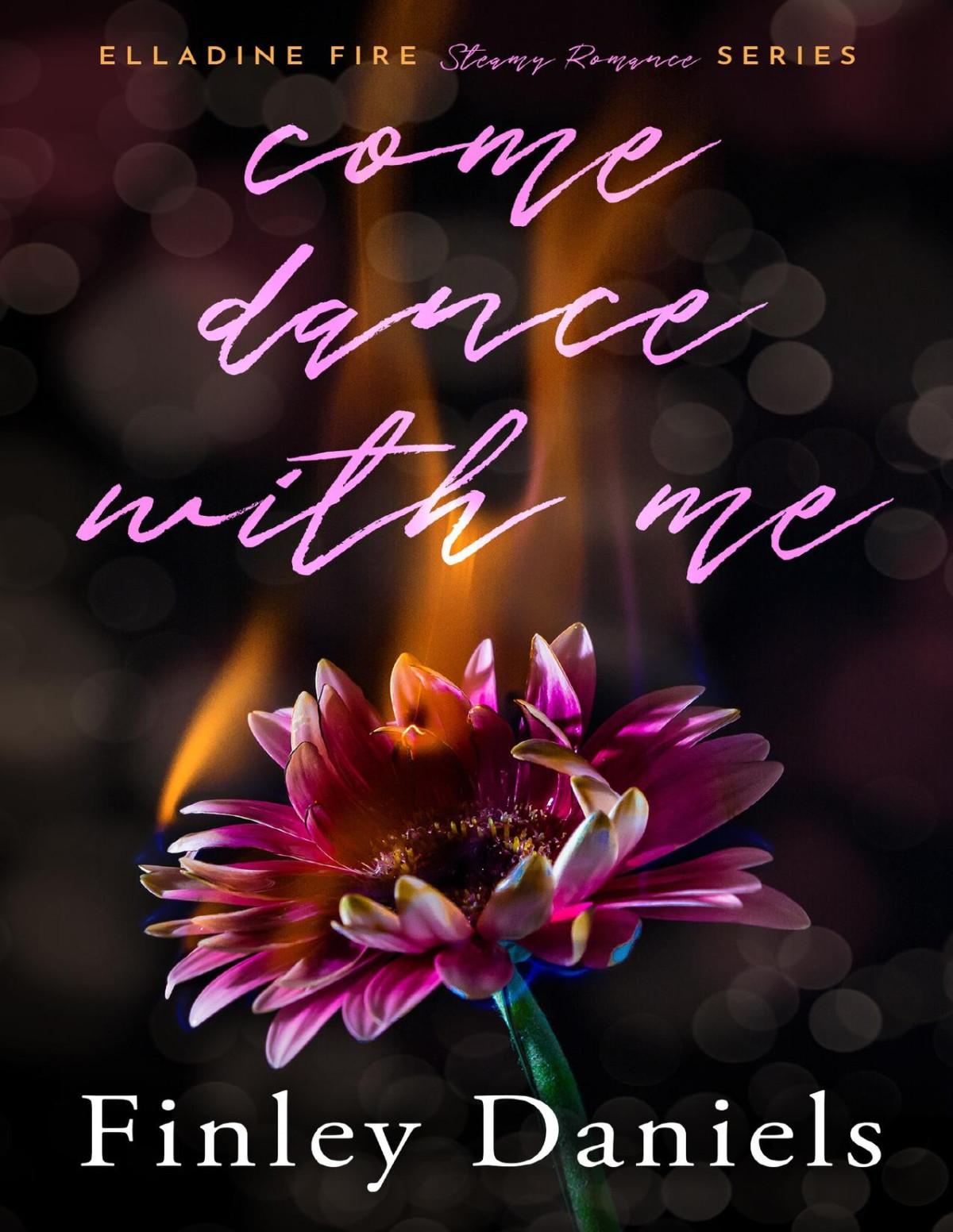
Paradigms in India Indranil De
https://ebookmass.com/product/social-norms-gender-and-collectivebehaviour-development-paradigms-in-india-indranil-de/
ebookmass.com
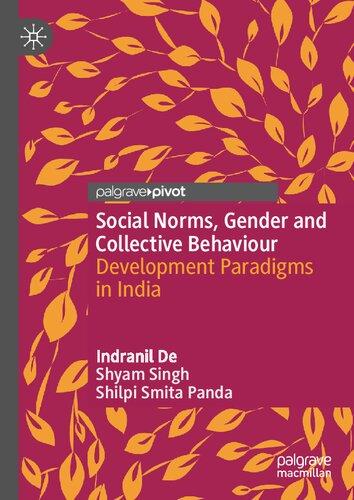
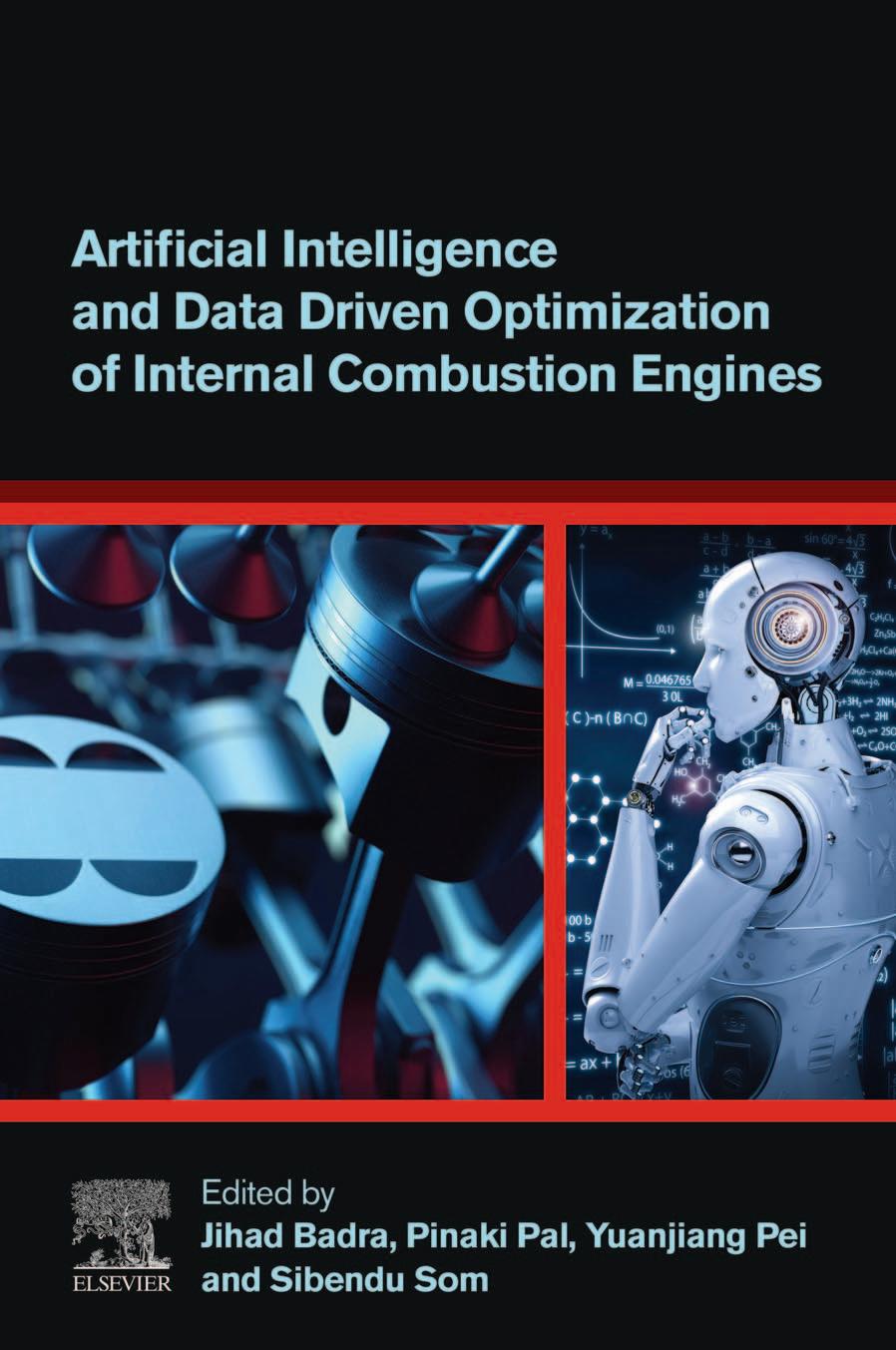
Elsevier
Radarweg29,POBox211,1000AEAmsterdam,Netherlands TheBoulevard,LangfordLane,Kidlington,OxfordOX51GB,UnitedKingdom 50HampshireStreet,5thFloor,Cambridge,MA02139,UnitedStates
Copyright © 2022ElsevierINC.Allrightsreserved.UChicagoArgonne,LLC,Contract No:DE-AC02-06CH11357.
Nopartofthispublicationmaybereproducedortransmittedinanyformorbyany means,electronicormechanical,includingphotocopying,recording,orany informationstorageandretrievalsystem,withoutpermissioninwritingfromthe publisher.Detailsonhowtoseekpermission,furtherinformationaboutthe Publisher’spermissionspoliciesandourarrangementswithorganizationssuchas theCopyrightClearanceCenterandtheCopyrightLicensingAgency,canbefoundat ourwebsite: www.elsevier.com/permissions.
Thisbookandtheindividualcontributionscontainedinitareprotectedunder copyrightbythePublisher(otherthanasmaybenotedherein).
Notices
Knowledgeandbestpracticeinthis fieldareconstantlychanging.Asnewresearch andexperiencebroadenourunderstanding,changesinresearchmethods,professional practices,ormedicaltreatmentmaybecomenecessary.
Practitionersandresearchersmustalwaysrelyontheirownexperienceandknowledge inevaluatingandusinganyinformation,methods,compounds,orexperiments describedherein.Inusingsuchinformationormethodstheyshouldbemindfulof theirownsafetyandthesafetyofothers,includingpartiesforwhomtheyhavea professionalresponsibility.
Tothefullestextentofthelaw,neitherthePublishernortheauthors,contributors,or editors,assumeanyliabilityforanyinjuryand/ordamagetopersonsorpropertyasa matterofproductsliability,negligenceorotherwise,orfromanyuseoroperationof anymethods,products,instructions,orideascontainedinthematerialherein.
LibraryofCongressCataloging-in-PublicationData
AcatalogrecordforthisbookisavailablefromtheLibraryofCongress
BritishLibraryCataloguing-in-PublicationData
AcataloguerecordforthisbookisavailablefromtheBritishLibrary
ISBN:978-0-323-88457-0
ForinformationonallElsevierpublicationsvisitourwebsite at https://www.elsevier.com/books-and-journals
Publisher: MatthewDeans
AcquisitionsEditor: CarrieBolger
EditorialProjectManager: MarianaKuhl
ProductionProjectManager: PoulouseJoseph
CoverDesigner: GregHarris
TypesetbyTNQTechnologies
Contributorsix
Forewordxi
Preface xv
1.Introduction1
BalajiMohan,PinakiPal,JihadBadra,YuanjiangPeiandSibenduSom
1. Industrialrevolution1
2. Artificialintelligence,machinelearning,anddeeplearning2
3. Machinelearningalgorithms3
4. Artificialintelligence basedfuel-engineco-optimization4
5. Summary16 References16
SECTION1ArtificialIntelligencetooptimizefuelformulation
2.Optimizationoffuelformulationusingadaptivelearningand artificialintelligence27
JulianeMueller,NamhoKim,SimonLapointe,MatthewJ.McNenly, MagnusSjöbergandRussellWhitesides
1. Introductionandmotivation27
2. Mixed-modecombustionandfuelperformancemetrics28
3. Aneuralnetworkmodeltopredictfuelresearchoctanenumbers31
4. Optimizationproblemformulationanddescriptionofsolutionapproaches32
5. Numericalexperimentsandresults37
6. Discussion40
7. Summaryandconcludingremarks42 Acknowledgments43 References43
3.Artificialintelligence enabledfueldesign47
KiranK.Yalamanchi,AndreNicolleandS.ManiSarathy
1. Transportationfuels47
2. Applicationofartificialintelligencetofuelformulation52
3. Conclusionsandperspectives58 Acknowledgments60 References60 v
SECTION2ArtificialIntelligenceandcomputational fluid dynamicstooptimizeinternalcombustion engines
4.Engineoptimizationusingcomputational fluiddynamics andgeneticalgorithms71
AlbertoBroatch,RicardoNovella,JoséM.Pastor,JosepGomez-Soriano andPeterKellySenecal
1. Introduction71
2. Modelingframeworkandaccelerationstrategies74
3. Optimizationmethods79
4. Summaryandconcludingremarks97 References98
5.Computational fluiddynamics guidedenginecombustion systemdesignoptimizationusingdesignofexperiments103
YuanjiangPei,AnqiZhang,PinakiPal,LeZhao,YuZhangand SibenduSom
1. Introduction103
2. Methodologies106
3. Arecentapplication113
4. Recommendationsforbestpractice118
5. Conclusionsandperspectives120 Acknowledgments121 References121
6.Amachinelearning-geneticalgorithmapproachfor rapidoptimizationofinternalcombustionengines125
JihadBadra,OpeoluwaOwoyele,PinakiPalandSibenduSom
1. Introduction125
2. Engineoptimizationproblemsetup127
3. Traininganddataexamination129
4. Machinelearning-geneticalgorithmapproach132
5. Automatedmachinelearning-geneticalgorithm141
6. Summary156 Acknowledgments156 References156
7.Machinelearning drivensequentialoptimizationusing dynamicexplorationandexploitation159
OpeoluwaOwoyeleandPinakiPal
1. Introduction159
2. ActiveMLoptimization(ActivO)160
3. Casestudy1:two-dimensionalcosinemixturefunction165
4. Casestudy2:computational fluiddynamics(CFD)-basedengine optimization171
5. Conclusions179 Acknowledgments180 References180
SECTION3ArtificialIntelligencetopredictabnormal enginephenomena
8.Artificial-intelligence-basedpredictionandcontrolof combustioninstabilitiesinspark-ignitionengines185
BryanMaldonado,AnnaStefanopoulouandBrianKaul
1. Introduction185
2. Casestudy:artificial-intelligence-enhancedmodelingofdilute spark-ignitioncycle-to-cyclevariability189
3. Casestudy:neuralnetworksforcombustionstabilitycontrol193
4. Casestudy:learningreferencegovernorformodel-freedilute limitidentificationandavoidance199
5. Summary204 References205
9.Usingdeeplearningtodiagnosepreignitioninturbocharged spark-ignitedengines213
EshanSingh,NursuluKuzhagaliyevaandS.ManiSarathy
1. Introduction213
2. Preignitiondetectionusingmachinelearningalgorithm215
3. Activationfunctions221
4. Experimentsanddataextraction222
5. Machinelearningmethodology224
6. Model1:Inputfromprincipalcomponentanalysis230
7. Model2:Timeseriesinput231
Contributors
JihadBadra
TransportTechnologiesDivision,ResearchandDevelopmentCenter,SaudiAramco, Dhahran,EasternProvince,SaudiArabia
AlbertoBroatch
CMT ThermalMotors,PolytechnicUniversityofValencia,CaminodeVera,Valencia, Spain
JosepGomez-Soriano
CMT ThermalMotors,PolytechnicUniversityofValencia,CaminodeVera,Valencia, Spain
BrianKaul
OakRidgeNationalLaboratory,Knoxville,TN,UnitedStates
NamhoKim
SandiaNationalLaboratories,Livermore,CA,UnitedStates
NursuluKuzhagaliyeva
CleanCombustionResearchCenter(CCRC),KingAbdullahUniversityofScienceand Technology,Thuwal,WesternProvince,SaudiArabia
SimonLapointe
LawrenceLivermoreNationalLaboratory,Livermore,CA,UnitedStates
BryanMaldonado
OakRidgeNationalLaboratory,Knoxville,TN,UnitedStates
MatthewJ.McNenly
LawrenceLivermoreNationalLaboratory,Livermore,CA,UnitedStates
BalajiMohan
TransportTechnologiesDivision,ResearchandDevelopmentCenter,SaudiAramco, Dhahran,EasternProvince,SaudiArabia
JulianeMueller
LawrenceBerkeleyNationalLaboratory,Berkeley,CA,UnitedStates
AndreNicolle
AramcoFuelResearchCenter,AramcoOverseas,Rueil-Malmaison,Paris,France
RicardoNovella
CMT ThermalMotors,PolytechnicUniversityofValencia,CaminodeVera,Valencia, Spain
OpeoluwaOwoyele
EnergySystemsDivision,ArgonneNationalLaboratory,Lemont,IL,UnitedStates
PinakiPal
EnergySystemsDivision,ArgonneNationalLaboratory,Lemont,IL,UnitedStates
JoséM.Pastor
CMT ThermalMotors,PolytechnicUniversityofValencia,CaminodeVera,Valencia, Spain
YuanjiangPei
AramcoAmericas:AramcoResearchCenter Detroit,Novi,MI,UnitedStates
S.ManiSarathy
CleanCombustionResearchCenter(CCRC),KingAbdullahUniversityofScienceand Technology,Thuwal,WesternProvince,SaudiArabia
PeterKellySenecal
ConvergentScience,Inc.,Madison,WI,UnitedStates
EshanSingh
CleanCombustionResearchCenter(CCRC),KingAbdullahUniversityofScienceand Technology,Thuwal,WesternProvince,SaudiArabia
MagnusSjöberg
SandiaNationalLaboratories,Livermore,CA,UnitedStates
SibenduSom
EnergySystemsDivision,ArgonneNationalLaboratory,Lemont,IL,UnitedStates
AnnaStefanopoulou
DepartmentofMechanicalEngineering,UniversityofMichigan,AnnArbor,MI, UnitedStates
RussellWhitesides
LawrenceLivermoreNationalLaboratory,Livermore,CA,UnitedStates
KiranK.Yalamanchi
CleanCombustionResearchCenter(CCRC),KingAbdullahUniversityofScienceand Technology,Thuwal,WesternProvince,SaudiArabia
AnqiZhang
AramcoAmericas:AramcoResearchCenter Detroit,Novi,MI,UnitedStates
YuZhang
AramcoAmericas:AramcoResearchCenter Detroit,Novi,MI,UnitedStates
LeZhao
EnergySystemsDivision,ArgonneNationalLaboratory,Lemont,IL,UnitedStates
Foreword
Theinternalcombustionengine(ICE)powersourworld.Fromcarsand long-haultruckstoagriculturalandconstructionequipments,liquid-and gaseous-fueledenginestouchpracticallyeveryaspectofourlives.ICEshave beenaroundfortwohundredyears,andthevehiclestheypowerpermeated themassesmorethanacenturyago.Thesemachineshaveimprovedour livesbyliftingpeopleoutofpovertyandgivingusthefreedomtoliveand workwhereweplease.
Today’sICElooksvastlydifferentfroman1800s-eraengine.Significant advancesinairdelivery,fueldelivery,andemissionscontrolsystemshave madethemodernengineamuchcleanerandmoreefficientmachine.Even withtheseadvances,however,thecombustionengineisnotsustainablein itscurrentform.Thereisnowaglobalracefordecarbonization,whichis leadingtothedevelopmentofnewinnovationsatanunprecedentedpace.
TheICEnolongerhastogoalone.Technologiessuchasbattery electricandhydrogenfuelcellvehiclesholdgreatpromiseforhelpingus achieveacleaner,greener,andmorediversemobilityfuture.Practically, though,thesetechnologiescanbeonly part ofthesolution.Westillneed engines alotofthem buttheengineoftomorrowmustbedifferent fromtoday’smachines;theengineoftomorrowmustbedecarbonized. Achievingafullydecarbonizedenginerequiresimprovementsinengine technologies,hybridization,andlow-orno-carbonfuels.
Howdowegetthere?Thepathforwardwillbeacombinationof improvedsimulationthroughtechniquessuchascomputational fluiddynamicsandadvancesinenginehardwareandcontrols.Virtualization throughsimulationhasbeen,andcontinuestobe,powerfulforoptimizing newenginetechnologies.Asanexample,theMazdaSKYACTIV-Xwas developedvirtuallybeforeanyphysicalprototypeswerebuilt,andthis successisjustthestartofwhatistocome.Butsimulationmethodsaloneare notenoughtogetuswhereweneedtogo,atleastnotintheshortamount oftimewehavetogetthere.
Theenginerepresentsalargeandcomplicateddesignspace,encompassingfuels,combustionchamberdesign,fuelinjection,ignitionstrategies, andmore.Thisisamassive,multi-objectiveoptimizationproblemwithnot justone,butacollectionofoptima.Makingthemostofthisparameter spacewillrequirecontinuedadvancesinenginecontroltechnologies,
sensors,andonboardcomputers.Theseadvancesarealreadyleadingto unprecedentedopportunity,butalsotoanever-expandingandunmanageabledesignspaceformodernengines.Currenttrendsareshowingan exponentialincreaseintheparameterspace,andthistrendisexpectedto continuefortheforeseeablefuture.Howcanwepossiblyevaluateand implementeverydesigncombinationto findthebestsolution?Thecurrent inabilitytorealizethepotentialofthisparameterspaceefficientlyand effectivelyisleadingtosuboptimalenginesinthemarket.
Fortunately, “bigscience” toolshaveevolvedalongsidetheengineitself. Supercomputing,advancednumericalmethods,andevenartificialintelligence(AI)arenowatourdisposal.Aswithsomanydisciplinestoday, machinelearning(ML)hasopenedanewrealmofpossibilitiesfor advancingengines.Bymarryingbigscience/datasciencewithvirtualrepresentationsoftheengine,wecan(andwill!)achievetheultimateICE design.AI/MLwillbridgeatomisticmodelingbreakthroughswithengineeringsimulations,andAI/MLcontrolswillalsoenableautonomous, intelligentsystemscontrols.Thesesystemswillhavetheabilitytolearn, adapt,andmanipulateenginecontrolstooperateattheedgeofstabilityto maximizeefficiencyandminimizeemissionsunderever-changingvehicle demands.
ThisbookdoesanexcellentjobcoveringtherelativelynewtopicofAI appliedtoenginesimulationandexperimentation.Insteadoftryingto covertheentiredesignspaceatonce,theauthorsofeachchapterfocusona singleaspect,suchasfuelformulation,enginecalibrationparameters, combustionchamberdesign,detectingabnormalenginephenomena(e.g., lowpressureignition),andmore.Furthermore,theauthorsdonotfocus solelyonthecurrentlearningalgorithms theyalsoprovideahistorical perspectiveandcoveroptimizationmethodsbasedontheprinciplesof evolution,forexample,geneticalgorithms.
Thisbookprovidesathoroughandtimelyoverviewofwhere AI-assistedengineoptimizationstandstoday,makingitavaluablereference fornewresearchersandseasonedenginedesignersalike.Theeditorshave succeededinchoosingnotonlytherighttopicstocoverbutalsotheright expertstowriteaboutthem.
WeareenteringabravenewworldfortheICE.Ourenginesmustbe cleanerandgreenerthanevertostayrelevantintoday’sdecarbonizing society.Thisbooklaysoutthemostpromisingtoolswehaveavailableto
ensuretheICEreachesitsfullpotential.Throughtherightcombinationof humanintelligenceandAI,advancedengineswillhelpusdecarbonize transportation.
Dr.KellySenecal Co-founder,ConvergentScience Madison,WI,UnitedStates
Dr.RobertWagner Director,BuildingsandTransportationScienceDivision, OakRidgeNationalLaboratory Knoxville,TN,UnitedStates
Preface
Internalcombustionenginesarenotgoingtogoawayanytimesoon. Internalcombustionengineiscurrentlytheprincipalprimemoverinthe transportationsector,anditisexpectedtomaintainitsdominancein theforeseeablefuture.Wetherefore,stronglybelievethatimprovingthe efficiencyandreducingtheemissionsfrominternalcombustionenginesare themosteffectivemeasurestohaveanimmediateandprominentimpacton theenvironment.Ouraiminthistextistoprovideanexpositionofvarious engineandfueloptimizationtechniquesandtoshowcasesomeoftheir applications.Specifically,thedesignoptimizationusingadvancedartificial intelligenceanddata-drivenmethodsisthefocusofthistext.Wehopethat thereaderswillenjoyitand,moreimportantly,learnfromit.
Thetextisprimarilyadaptedfrompreviousarchivalpublicationsmade bythevariousactiveresearchgroupsinthisspecificarea.Theauthorslistis acombinationofleading,reputable,andactiveexpertsinthe fieldofinternalcombustionenginesandartificialintelligence.Wearegratefultothe authorsfortheirinvaluablecontributionsandaretrulyhonoredtohave workedwithsuchanelitegroupofworld-renownedresearchers.
JihadBadra,TeamLeader
EngineCombustionTeam,TransportTechnologiesDivision, ResearchandDevelopmentCenter,SaudiAramco,Dhahran, EasternProvince,SaudiArabia
PinakiPal,ResearchScientist EnergySystemsDivision,ArgonneNationalLaboratory, Lemont,IL,UnitedStates
YuanjiangPei,TeamLeader
ComputationalModelingTeam,AramcoAmericas:Aramco ResearchCenter Detroit,Novi,MI,UnitesStates
SibenduSom,Manager
Multi-PhysicsComputationSection,EnergySystemsDivision, ArgonneNationalLaboratory,Lemont,IL,UnitedStates