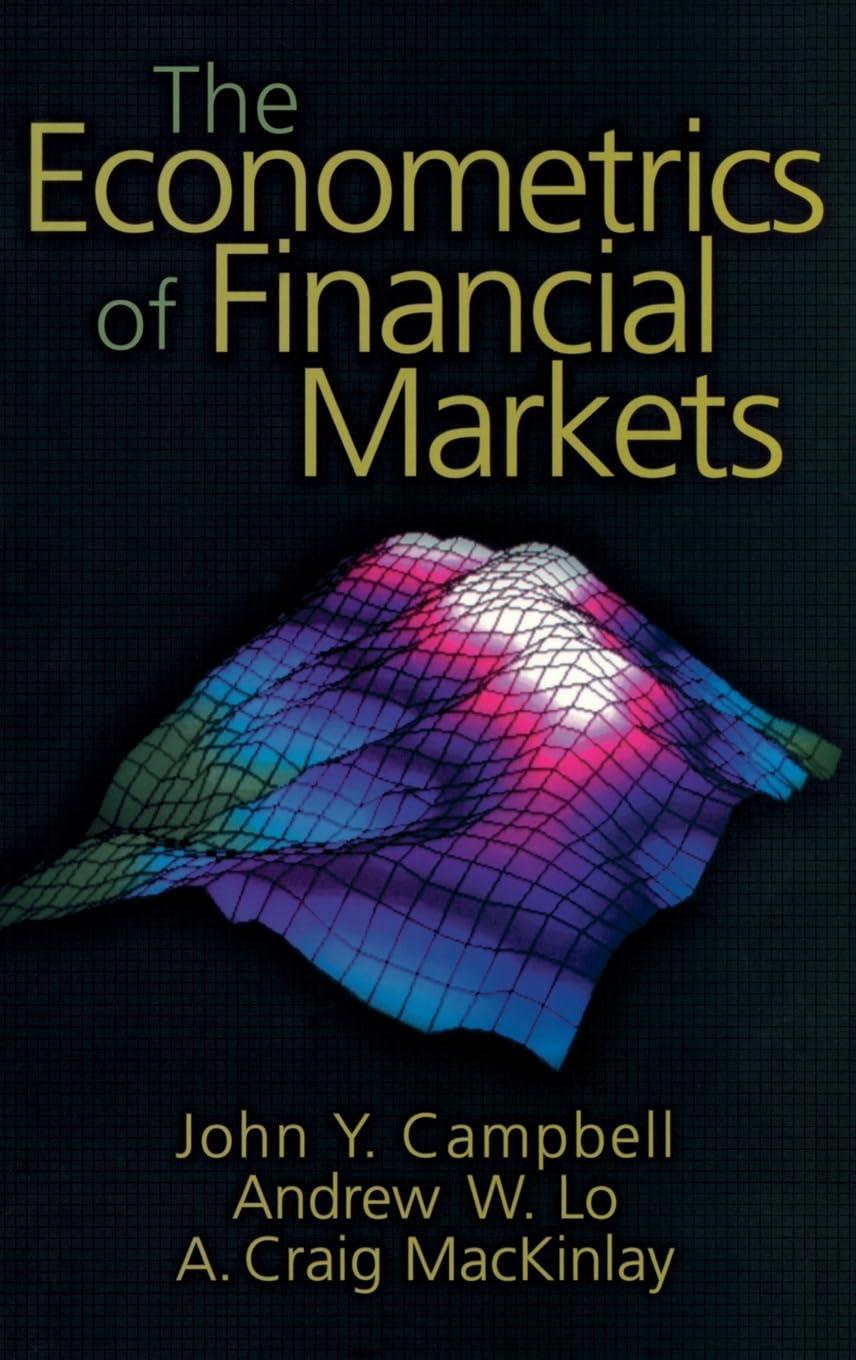
"The Econometrics of Financial Markets" (2nd Edition) by John Y. Campbell, Andrew W. Lo, and A. Craig MacKinlay is a comprehensive resource on the application of econometric techniques in understanding and modeling financial markets. The book explores how econometrics, the statistical analysis of economic and financial data, can be used to measure, model, and forecast financial market behavior. It covers a wide range of topics, including asset pricing models, market efficiency, volatility, and time series analysis, providing both theoretical frameworks and empirical methodologies for analyzing financial data.
This book is highly regarded for its thorough and systematic treatment of financial econometrics, making it a vital resource for students and professionals in finance and economics. Here’s a detailed summary of its key sections:
Part 1: Financial Theory and Econometric Models
Chapter 1: Introduction to Financial Econometrics
The first chapter sets the stage for the entire book by discussing the intersection of financial theory and
econometrics. It introduces readers to the main questions financial econometrics seeks to answer, such as how asset prices are determined, how markets react to new information, and how risks can be modeled and quantified. Campbell, Lo, and MacKinlay stress the importance of econometrics in validating financial theories with empirical data, while also highlighting the challenges of doing so in the complex, dynamic environment of financial markets.
Chapter 2: Review of Financial Theory
This chapter provides a brief but essential review of fundamental financial theory, which forms the backbone of econometric modeling in finance. Topics include modern portfolio theory, the Capital Asset Pricing Model (CAPM), the Arbitrage Pricing Theory (APT), and the concept of market efficiency. These theories describe how investors make decisions under uncertainty, how assets are priced, and how risks are managed in financial markets. The chapter sets up the framework for the econometric techniques discussed later, emphasizing how these theories can be tested using real-world data.
Part 2: Time Series Analysis in Financial Markets
Chapter 3: Time Series Models in Financial Econometrics
The book introduces time series econometrics as a key tool for analyzing financial markets, where asset prices and returns are typically represented as time series data. The authors cover the basics of time series analysis, including autoregressive (AR) models, moving average (MA) models, and autoregressive integrated moving average (ARIMA) models. They emphasize the importance of stationarity (i.e., whether statistical properties of a time series, such as mean and variance, remain constant over time) in financial data analysis.
Chapter 4: Volatility Models
Volatility, or the degree of variation in asset prices, is a crucial concept in financial markets. This chapter dives into models designed to capture and forecast volatility, such as ARCH (Autoregressive Conditional Heteroskedasticity) and GARCH (Generalized ARCH) models. These models account for the "volatility clustering" phenomenon often observed in financial markets, where large changes in asset prices are followed by large
changes, and small changes are followed by small changes. The chapter also introduces more advanced volatility models, such as EGARCH and GARCH-in-Mean, which incorporate the effect of volatility on returns.
Part 3: Empirical Tests of Asset Pricing Models
Chapter 5: Empirical Tests of the CAPM
In this chapter, the authors present a detailed review of empirical tests of the Capital Asset Pricing Model (CAPM), one of the foundational models of finance that relates the risk of an asset to its expected return. Using econometric techniques, the authors show how to test the CAPM by estimating the relationship between an asset's returns and the market portfolio’s returns (beta). They also discuss the limitations of the CAPM, such as the assumption of a single-period investment horizon and the difficulty of defining the "market portfolio."
Chapter 6: Multifactor Models and Arbitrage Pricing Theory
The Arbitrage Pricing Theory (APT) is an alternative to the CAPM that assumes multiple risk factors drive asset returns, rather than a single market factor. This chapter explores how to
empirically test the APT and other multifactor models by identifying the relevant risk factors, estimating factor loadings, and determining how these factors explain variations in asset returns. The authors also examine empirical challenges, such as choosing the right factors and dealing with measurement errors.
Part 4: Market Efficiency and Predictability
Chapter 7: Efficient Market Hypothesis (EMH)
The Efficient Market Hypothesis (EMH) posits that asset prices fully reflect all available information, making it impossible to consistently achieve abnormal returns. This chapter reviews the theoretical basis of the EMH and presents empirical tests of market efficiency using econometric methods. The authors discuss three forms of market efficiency—weak, semi-strong, and strong—and describe statistical tests to detect inefficiencies, such as serial correlation tests and event studies. They also critically examine evidence of market anomalies (e.g., the January effect, momentum, and overreaction) that challenge the EMH.
Chapter 8: Forecasting Financial Markets
Forecasting future asset prices and returns is a key application of econometric models in finance. This chapter explores different techniques for forecasting financial markets, such as regression models, autoregressive models, and nonlinear models. The authors emphasize the importance of out-ofsample testing and evaluating forecast accuracy using measures like the mean squared error (MSE) and the out-of-sample R-squared. They also discuss the limits of predictability in financial markets, as suggested by the EMH.
Part 5: Market Microstructure and High-Frequency Data
Chapter 9: Market Microstructure
Market microstructure refers to the way financial markets operate at a granular level, focusing on the process by which prices are determined and trades are executed. This chapter delves into the econometric analysis of market microstructure, covering topics such as bid-ask spreads, price impact of trades, and information asymmetry. The authors examine how trading mechanisms, order flows, and liquidity affect price formation and
market efficiency, providing empirical techniques for analyzing high-frequency data.
Chapter 10: High-Frequency Financial Data
The availability of high-frequency data (e.g., secondby-second or minute-by-minute prices and volumes) has opened new avenues for financial econometrics. In this chapter, the authors discuss the challenges of working with high-frequency data, such as market microstructure noise and the potential for spurious correlations. They introduce advanced econometric techniques for modeling high-frequency data, such as multivariate GARCH models and nonlinear time series models, and demonstrate their application in areas like volatility forecasting and intraday trading strategies.
Part 6: Risk Management and Derivatives
Chapter 11: Risk Management
Risk management is central to both financial theory and practice, and this chapter provides an econometric approach to measuring and managing risk. The authors introduce different types of financial risks, such as market risk, credit risk, and liquidity risk, and discuss econometric techniques
for quantifying these risks, such as Value at Risk (VaR) and expected shortfall. They also explain how risk models can be validated using backtesting and stress testing, helping financial institutions ensure that their risk management strategies are robust.
Chapter 12: Econometrics of Derivatives Markets
Derivatives, such as options and futures, are complex financial instruments whose values are derived from underlying assets. This chapter explores the econometrics of derivatives pricing and risk management, focusing on models such as the Black-Scholes option pricing model and extensions like stochastic volatility models and jump-diffusion models. The authors also discuss how econometric methods can be used to estimate the parameters of these models and test their predictive power in the real world.
Part 7: Long-Run Relationships and Cointegration
Chapter 13: Cointegration and Long-Run Equilibrium Relationships
Cointegration is a powerful econometric concept used to analyze the long-run relationships between financial variables. This chapter introduces the
theory of cointegration and explains how it can be used to model long-term equilibrium relationships between asset prices, interest rates, and exchange rates. The authors cover the Engle-Granger and Johansen cointegration methods and provide examples of how cointegration is used in asset pricing, portfolio management, and term structure modeling.
Chapter 14: Applications of Cointegration in Financial Markets
Building on the previous chapter, this section explores empirical applications of cointegration in financial markets. The authors demonstrate how cointegration can be used to model the relationship between stock prices and dividends, interest rates and bond yields, and exchange rates and purchasing power parity. They also discuss the implications of cointegration for investment strategies, such as pairs trading and mean reversion strategies.
Part 8: Advanced Topics in Financial Econometrics
Chapter 15: Nonlinear Models in Finance
Financial markets often exhibit nonlinear behavior, such as volatility clustering and jumps in asset
prices. This chapter explores advanced econometric techniques for modeling nonlinearities, including nonlinear autoregressive models, threshold models, and Markov switching models. The authors show how these models can be applied to analyze regime shifts, market crashes, and other non-linear phenomena in financial markets.
16: Machine Learning and Financial Econometrics
The final chapter discusses the integration of machine learning techniques into financial econometrics. With the growing availability of large financial datasets, machine learning methods, such as neural networks, random forests, and support vector machines, are increasingly used to analyze and forecast financial markets. The authors explore the advantages and limitations of machine learning in finance, emphasizing the importance of combining these techniques with traditional econometric models to improve predictive performance and decision-making.
Conclusion
"The Econometrics of Financial Markets" provides a thorough and accessible guide to the econometric methods used in finance, bridging the gap between financial theory and empirical analysis. The book equips readers with the tools to analyze financial data, test financial theories, and develop strategies for risk management and asset pricing. With its blend of theoretical foundations and practical applications, it remains a valuable resource for students, researchers, and practitioners in financial econometrics.