Automating 3D scanning of built environments
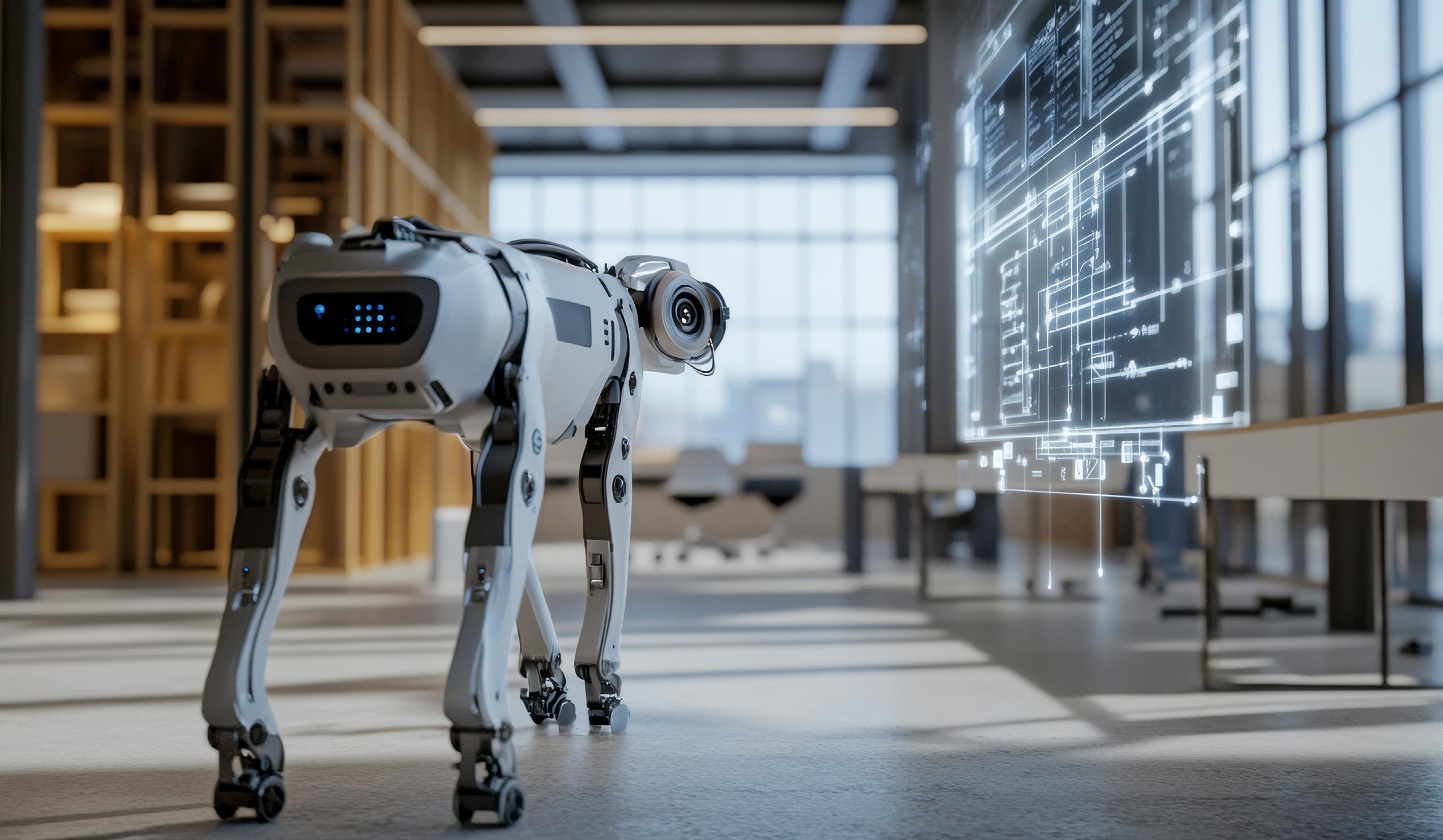
By integrating building information with indoor spatial data, robots are equipped with the capability to navigate and map complex indoor spaces more efficiently, advancing how industries capture the digital representation of the built environment.
Imagine a sleek robot navigating narrow corridors and cluttered spaces, automatically mapping architectural and structural details precisely as it moves — driven by intelligent planning algorithms instead of human intervention.
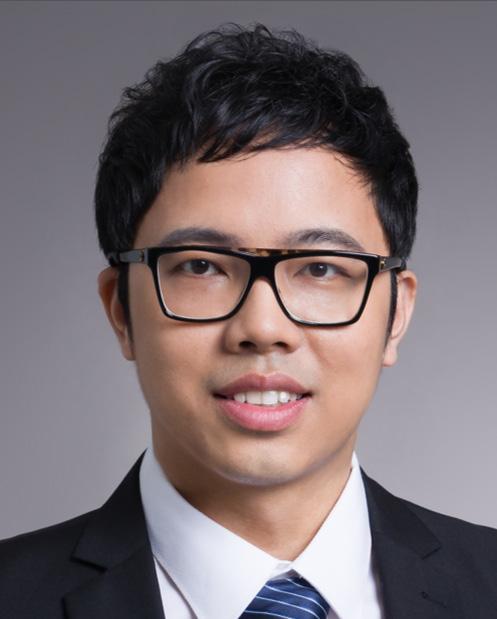
This is the vision Assistant Professor Vincent Gan and his team are working to realise at the Department of the Built Environment, College of Design of Engineering, National University of Singapore.
Scanning and 3D reconstruction in the built environment present unique challenges, including irregular building layouts, weakly-textured elements like white walls, dynamic obstacles such as people and furniture, and diverse material properties like concrete and glass. These complexities not only present significant opportunities for technological innovation but also demand robustness in algorithms and adaptability in hardware. Key questions emerge: How can scanning paths be optimised to achieve both efficient and comprehensive coverage? How can building information be integrated to enhance robots’ understanding of built spaces, enabling smarter navigation and operations?
By integrating Building Information Modelling (BIM), a commonly used digital model that represents a building’s physical and functional characteristics, with spatial data from IndoorGML, a standard for indoor spatial information, the team has developed a new approach that allows quadruped robots to optimise their routes and scanning at the upfront. This reduces the need for manual oversight and enhances scan data quality — a boon for industries that rely on accurate 3D insights, especially in GPS-limited spaces like indoor environments.
The team’s findings were published in Automation in Construction on 11 July 2024.
Reimagining 3D reconstruction
Aligned with Singapore’s Smart Nation initiative, the concept of digital cities is gaining prominence. There is a growing momentum to harness automated, intelligent technologies to capture and reconstruct 3D digital representations of the built environment, integrating this data into virtual platforms. Such platforms potentially facilitate information management, condition monitoring, assessment and maintenance of the built facilities. For example, 3D scanning enables surveyors to conduct precise as-built surveys, allowing early identification of errors during the design-to-construction phase to mitigate costly rework. This technology is equally valuable for legacy buildings and infrastructures in urban areas, where detailed scans play a critical role in documentation and preservation efforts, ensuring the longevity and integrity of these assets.
04 | January 2025 Forging
Traditionally, 3D scanning has been labour-intensive, relying on stationary or mobile laser scanning tools that require manual setup and positioning. This becomes especially cumbersome in cluttered environments without GPS access. While robotics offers a better way, automated navigation and scanning in such spaces remain challenging.
Many robotics-enabled methods struggle to capture the nuances of indoor spaces — whether tight passages, machinery or furniture — often leading to incomplete scans that require extensive postprocessing.
“We wanted to develop a new approach that enables robots to navigate and scan automatically, ensuring high-quality scan data with enhanced coverage, without human intervention,” says Asst Prof Gan.
“We wanted to develop a new approach that enables robots to navigate and scan automatically, ensuring high-quality scan data with enhanced coverage, without human intervention.”
To achieve this, the researchers integrated BIM with IndoorGML. BIM provides detailed geometric information, but it lacks the detailed spatial connectivity needed for robotic navigation. “Enriching BIM with IndoorGML enables the creation of a dynamic indoor navigation model that integrates building geometry with spatial topological data. This equips robots with the ability to interpret multi-scale spatial networks,” explains Asst Prof Gan.
The team also infused the navigation model with an algorithm that guides the robot to optimal scanning positions along its path. This algorithm strategically optimises the key scanning positions and traversal sequences that reduce the number of scans needed while maximising the scan coverage. Additionally, a sensor perception model has been incorporated to address potential occlusions in scanning, ensuring high-quality data acquisition.
The researchers then put their system to the test, deploying a quadruped robot equipped with a 3D LiDAR sensor at an NUS building. The robotic scan demonstrated accuracy comparable to conventional terrestrial laser scanning while enhancing scanning efficiency and coverage. By facilitating the reality capture of built facilities,
this approach reduces the time and costs associated with traditional manual data acquisition and processing, transforming workflows in the digitalisation of the built environment.
Advancing robot navigation with BIM
Automating 3D scanning brings multifaceted benefits across different industry applications. Faster, more comprehensive scanning helps robots operate in unstructured, dynamic environments such as construction sites.
“Real-world environments could be complex — think moving furniture, workers, fluctuating light conditions or doors opening and closing. These variables present new challenges for robots in the built environment.”
“Real-world environments could be complex — think moving furniture, workers, fluctuating light conditions or doors opening and closing. These variables present new challenges for robots in the built environment,” says Asst Prof Gan.
Moving forward, the team plans to leverage BIM-derived ‘as-planned’ information to rapidly generate virtual point clouds that accurately represent the geometry of built environments. These virtual point clouds can then be used to train AI models to classify point clouds with semantic labels, producing 3D semantic maps of the surrounding structure. This capability enhances a robot’s understanding of its environment and supports the development of semantic-aware algorithms to improve situational awareness and navigation in complex spaces. Another recent study by the team published in Automation in Construction, looks into this challenge.
“We will work closely with experts in mechanical engineering, electronics, and computer science to advance the hardware and software aspects to facilitate the adoption of robotics and automation in the built environment,” shares Asst Prof Gan.