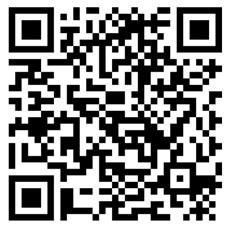
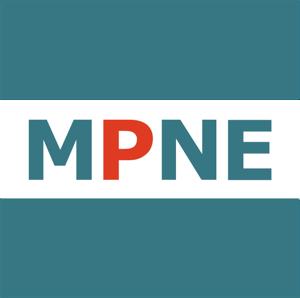
Melanoma Patient Network Europe
Legal entity
MPNEsupport
Fjällbo Selknä 152, 74177 Uppsala, Sweden
Org 168024921069
https://www.mpneurope.org/
disclaimer
MPNEconsensus 2024 was conducted as part of MPNE's deliverables for the iToBoS project under WP11 'patient engagement and education, Task 11.1.
iToBoS is a project funded under the European Horizon 2020 research and innovation program under grant agreement 965221.
The information and views provided in this document are solely the authors' and do not constitue endorsement by the European Comission.
B. Ryll11, G. Spurrier-Bernard¹ 2, A. Evans1, R. White1, V. Astratinei1 3 4, F. Östman1 5, Tamás Benkó1 6, H. Boetel1 7, K. Curtin1 8, I. James1, M. Pajunen1 9, A. Skoutari1 10, S. Szókovács-Vajda1 6, O. Valciņa1 11, A. Wispler1,7, L. Zielinska1,12
affiliations
1 Melanoma Patient Network Europe, www.mpneurope.org
2 Melanome France, France
3 Melanom Romania, Romania
4 Stichting Melanoom, Netherlands
5 Melanomföreningen, Sweden
6 Magyar Melanoma Alapítvány, Hungary
7 Hautkrebs-Netzwerk Deutschland e.V., Germany
8 Melanoma Support Ireland, Ireland
9 Cancer Patients Finland - Suomen Syöpäpotilaat, Finland
10 PASYKAF CY, Cyprus
11 Melanoma Patient Network of Latvia “Step Ahead of Melanoma”, Latvia
12 Polish Sarcoma and Melanoma Patients Association /Stowarzyszenie Pomocy Chorym na Mięsaki i Czerniaki Sarcoma/, Poland
www.mpneurope.org
MPNEconsensus 2024, a patient consensus process on data, AI and data-dependent business models was conducted by MPNE, the Melanoma Patient Network Europe, in early 2024. The objective was to develop an independently generated patient position as part of the patient engagement of the iToBoS- intelligent Total Body Scanner- project as part of MPNE's responsibilities. iToBoS has received project funding from the European Union’s Horizon 2020 research and innovation programme under grant agreement No 965221 und which the consensus meeting was funded. The event was generously hosted by the iToBoS partner Fraunhofer Institute for Telecommunications, Heinrich-Hertz-Institut, HHI, Berlin.
This White Paper summarises the consensus statements formulated based on the discussions at MPNEconsensus 2024 and constitutes of 10 core consensus statements capturing over-arching principles and consensus statements on 8 sub-sections:
Data governance and future-proof ‘zero-trust’ systems; Privacy-preserving AI in healthcare; Ethical frameworks for data use and AI; Trustworthy and reliable AI beyond explainability; Regulatory frameworks, independent process oversight, effective enforcement and punitive sanctions for misconduct; Mitigation, proactivity and agility; New models for data-dependent business and research and Patient-led independent spaces for learning and debate to advance complex issues.
The White Paper is intended as a 'Living document' and aims to contribute an independently formulate patient perspective to the general data discussion, e.g. on the European Health data Space, the AI Act, the European Cancer Patient Data Centre and other data-related initiatives and projects.
The objective of an independent patient consensus process is to provide the community of those, who both stand to considerably benefit but who also carry the ultimate risk, with a self-owned process to build community consensus and to arrive at substantiated positions free from the habitual framing imposed by other stakeholder groups. MPNEconsensus 2024 is MPNE's second consensus process, a third one is in preparation and will be published in due course, together with the overall methodology.
The consensus process has recently been recognised by the EU Innovation Radar as Innovation under the title: Socio-Cultural and Ethical Guidelines for Future Implementation of AI in the Medical Context1
This work would have not been possible without the contribution of those willing to make their technical expertise accessible to a non-specialist audience and to engage in discourse over extended periods of time.
We therefore thank all internal and external speakers and in particular, our iToBoS colleagues from the Fraunhofer Institute for Telecommunication Berlin, IBM Research in Haifa and the Hannover Centre for Optical Technologies, for their effort, time but above all: enthusiasm! to make their technical fields of work accessible to the MPNE community.
Bettina Ryll MPNE founder
1 Innovation radar > Innovation > Socio-Cultural and Ethical Guidelines for Future Implementation of AI in the Medical Context. https://innovation-radar.ec.europa.eu/ innovation/57594.
impressum 1
executive summary 3
table of contents 6
MPNEconsensus 2024 7
Consensus phases 9
Problem statements 11
MPNE core consensus statements 17
MPNE specific consensus statements 19- 40
Data governance and future-proof ‘zero-trust’ systems 19
Privacy-preserving AI in healthcare 23
Ethical frameworks for data use and AI 25
Trustworthy and reliable AI beyond explainability 27
Regulatory frameworks, independent process oversight, effective enforcement and punitive sanctions for misconduct 33
Mitigation, proactivity and agility 35
New models for data-dependent business and research 37
Patient-led independent spaces for learning and debate 39
conclusions 41
next steps 42
annex 44
consensus meeting program 45 about 47
The MPNEconsensus2024 took place at the Frounhofer Institute for Telecommunications, Heinrich-Hertz-Institut, Berlin 31st January- 2nd February 2024.
Today, research and innovation projects in health nearly invariably rely on some form of health data acquisition and analysis. Discussions about the harmonisation of data acquisition, data sharing and access rights between countries and legislations, privacypreserving methods and strategies, data analytics and validation of methods and models, including AI models are therefore common and shared between different research projects.
Consensus building is an iterative process starting with observation and understanding of patient need (1), followed by a phase of learning with consulting of expertise and debate (2) and a phase for formulating decisions and actions (3), to then observe the
Patients are the stakeholder group to ultimately benefit most from successful researchbut also to carry most of the unintend consequences. Striking a successful balance that both enables research while mitigating and addressing risks is therefore a core patient interest.
The objective of the MPNEconsensus meeting was to generate independent patient consensus on data-related topics identified as problematic by the patient community.
impact on patients again (1). The process is protocolled to trace the development of the consensus to improve the process itself.
This is MPNE's second Consensus process.
The consensus meeting was recognised in 2024 as innovation by the EU innovation radar under the title: Socio-Cultural and Ethical Guidelines for Future Implementation of AI in the Medical Context2.
A process to help patient communities develop valid and substantiated positions on complex topics. A valid position on complex topics requires background research, reflection and debate and is therefore not suitable for ad hoc single positions and majority votes; it rather requires
Making patient concerns accessible for other stakeholders. The interaction with diverse stakeholders in the consensus building process creates a bi-directional information exchange, providing outsiders with access to the insights, reflections and the working of a patient community and the latter
deliberation and a consensus-building process involving a sufficiently large proportion of the advocacy community and methodology open for analysis and continuous improvement.
with access to expertise, interests and constraints of the former. ‘Pre-decision’ safe spaces allow to formulate positions that can then be tested and re-worked in subsequent exchanges, continuously improving and refining them.
November 23- January 24
Preparation
During the preparation phase of the meeting, MPNE advocates formulated statements capturing the concerns of the patient community; these were presented in form of an Oxford Debate at the consensus meeting.
January - February
March- April
MPNEconsensus Documentation
MPNEconsensus 2024 took place in Berlin 31st January- 2nd February 2024. Consensus statements were formulated after each of the specific sections and reviewed in total during the final day of the conference.
Based on the meeting notes and the consensus statements formulated at the MPNE consensus meeting, Version 1.0 of this White Paper was drafted.
‘This house believes that patients should be compelled to give up their data for whatever purpose the state sees fit; if they wish to benefit from solidarity-based funded healthcare'
Version 1.0 was circulated internallly to different audiences and adapted based on the feedback received. A first version of the core consensus statements was published on the MPNE website.
The White Paper v 2.0 will be circulated to internal and external communities for discussion. Previously involved and new experts will be contacted for further discussion/ elaboration/ action on respective sections for a future version 3.0 May-
The consensus sections on specific topics were expanded, adding background and references, the document was re-circulated internally for feedback, resulting in the present version of the Consensus White Paper.
MPNE is a volunteer-based European network of Melanoma patients and family members.
The network operates across language and healthcare systems barriers and works principle-based, ensuring patient-centricity, focus on solutions, evidence-basis, proactivity and constructiveness.
Starting points for the MPNEconsensus 2024 meeting were broad, datarelated problems the MPNE community had identified in their work; these were brought forward in the Oxford Debate.
Problematic access to personal data and publicly financed data sources casting doubt on the motives and credibility of the parties involved.
Patients struggle to access their own medical records, including pathology, laboratory and imaging files and reports. Patients are thereby often left in the dark about their personal rights and GDPR is falsely invoked to withhold data.
Research data and results are routinely withheld from patients,
even if they contain clinically relevant or actionable information such as pathological germline variants or prognostic factors.
Publicly funded data sets are not inaccessible even for other established, publicly funded research consortia, leading to avoidable duplication and undermining rapid progress in research.
This casts serious doubts on the intentions and credibility of the parties involved.
The MPNEconsensus 2024 meeting was conducted within the iToBoS project.
Problem statements were however deliberately broadened to ensure relevance beyond the well-defined data-related scope of iToBoS; statements are based on the MPNE community’s long-standing involvement in diverse data-related activities.
Patients’ desperation and dependency is unduly exploited to force consent under the cover of ‘altruism’.
By the time you know enough, it’s too late. Patients, dealing with disease and treatment uncertainty in health landscapes that they don’t fully understand, can hand over control of their lives and information, simply because they do not feel equipped to take responsibility or even capable of questioning, as they often would in other choice situations such as lifestyle, financial or commercial “transactions”.
Especially at first diagnosis, cancer patients frequently have to make far-reaching decisions without having had the time to sufficiently educate themselves. This leads to the tendency to heavily rely and trust those who speak authoritatively around them, whether or not that is merited.
With time and the initial shock of a cancer diagnosis receding, patients’ understanding of the disease and familiarity with the health system increases, making them more critical and discerning. However at that point in time, the ‘horse has bolted’ and patients have already made decisions before being aware of potential consequences to themselves or their families.
Dependency on care team. Craving a good relationship with their clinical team on which they ultimately depend for their care makes patients vulnerable to decisions that please their team and to consent for fear of negative repercussions. Decisions under these conditions of dependency cannot be considered independent nor free.
Unjustified moral pressure. Despite the fact that they themselves, as European patients, are contributing to solidarity-based healthcare systems, especially patients in tax-financed healthcare systems are then made to feel as petitioners for charity provided by others rather than as the entitled users they are, further increasing moral pressure on patients to comply with the wishes of others.
This is often exacerbated by the way health systems view patients who question, as“difficult”, “time-wasters” or “antisocial”: there is frequent gaslighting of patients by healthcare professionals, the health system and even their own communities to ‘behave altruistically’ and to e.g. share their time, data and tissue without concern for themselves.
The combination of information asymmetrie, dependency on care providers and moral pressure can make patients easy targets for exploitation for the interests of other
Data needs to generate true value for all parties involved, not just unllaterally.
The MPNE community is acutely aware that improving patient outcomes relies on impactful research and swift and effective translation of research findings into processes, products and services that can ensure research.
At the same time, the community is frustrated about current approaches that mainly result in siloes ‘everyone their own data platform or registry’, access control and lack of
stakeholder groups and against their personal best interest; almost uniquely in human experience, in social healthcare, it continues to be encouraged by societal leaders.
collaboration- ‘Everyone wants our data but no one wants to share theirs’.
Financial models were recognised as problematic as data platforms were mainly driven by the interest to monetise data while offering patients services of limited interest in return.
In contrast, business models that a good for patients because they are not reliant on selling data struggle to find investment and don’t survive, e.g. ImputeMe1
1 Folkersen, L. et al. Impute.me: An Open-Source, Non-profit Tool for Using Data From Direct-toConsumer Genetic Testing to Calculate and Interpret Polygenic Risk Scores. Front. Genet. 11, 578 (2020).
Appeals for ‘trust’ are no replacement for transparency and accountability.
In the current data debate, the MPNE community missed concrete value propositions to patients and saw a clear gap between vague promises
such as ‘scientific progress’, ‘better patient outcomes’ and the reality of the community e.g. looking for second opinions, access to clinical trials and treatment and specific expertise such as radiotherapy or palliative care.
In addition to the disregard of the valid expectation of patients to see concrete benefit from sharing their data, the equally valid concerns and reservation regarding risks and negative consequences to themselves but more often, their families, are brushed aside. Instead, patients are encouraged to place their ‘trust’ in organisations that in the past have rather demonstrated their inability to place citizen rights and interest first.
The community felt strongly that trustworthiness therefore had to be enshrined into the system itself in a trust-by-design approach, ensuring auditability, transparency of decision-making and accountability, and needed to be above the reach of individuals or organisations that might be willing to forgo citizens’ rights for personal, economic, political or other interests.
Constructive contribution to discussions and policies on data and AI is time-consuming and requires learning, access to expertise and debate.
In the past years, the MPNE community has been contacted with increasing frequency to comment on policy drafts, white papers or other statements concerning data and AI. We are regularly invited to conferences and workshops for a patient position on data, AI and related topics.
MPNE has actively participated in initiatives such as GetReal1, multiple initiatives on the use of Real World Evidence in decision-making at
regulatory and HTA level and closely followed European data-related initiatives, e.g. TEHDAS1 and 22, GA4GH3 or TEF-Health4 and the development of the ECPDC5
We believe that civil society needs to be an integral part of decision-making in democracies and therefore highly value these opportunities. However, it also became clear that meaningful and constructive contribution critically relies on iterative processes of a) accessing diverse expert knowledge and b) broad discussion within the community, requiring considerable effort and time.
1 GETREAL | IHI Innovative Health Initiative. https://www.ihi.europa.eu/projects-results/projectfactsheets/getreal.
2 Second Joint Action Towards the European Health Data Space – TEHDAS2 - Tehdas. https://tehdas. eu/.
3 GA4GH. https://www.ga4gh.org/.
4 TEF-Health - Testing and Experimentation Facility for Health and Robotics. https://tefhealth.eu/ home.
5 An operational concept for a European Cancer Patient Digital Centre - Publications Office of the EU. https://op.europa.eu/en/publication-detail/-/publication/495c3a52-2161-11ef-a251-01aa75ed71a1/languageen.
1- Data use is an ethical imperative as people are dying and we as society have data that could save them.
2- Data should be used but not abused. Individuals and societies need to be effectively protected against abuse; this will require new ethical frameworks for the use of data and AI, diverse risk mitigation strategies, effective enforcement and proactivity and agility in the approach.
3- The approach to data use should be risk-appropriate, similar to the benefit/ risk trade-offs seen with drugs. Data is not a one-size-fits all category: differentiation of different data sets and the risks associated with them is critically needed.
4- Health data is common goods - it is not acceptable to extract value for a few and settle patients and society with the risks; this will require new models for business and research beyond platformization.
5- Altruism gaslighting is unacceptable: people have valid concerns and reservations, e.g. with regards to their privacy, protection against misuse and onesided value extraction.
6- Citizens need ‘zero-trust’ data environments beyond the reach of single parties, institutions or governments, in particular, for sentitive data such as genomic data, to protect the rights of citizens.
7- Governance needs to ensure that those most affected by the risks have a voice.
8- It is evident that tech is not able to self-regulate, control therefore has to occur at the level of laws and regulations. Citizens and patients need hard guardrails of what is permissible when it comes to data-use; realtime monitoring and effective enforcement, e.g. existential fines to ensure compliance with laws, regulations and ethical standards..
9- Society and patients need future-proof approaches, taking into account ‘the unknown unknowns’ of future technologies. Society, regulators and decisionmakers need to consider the risks for today’s but also for future generations.
10- We as a patient community need independent spaces for learning, exchange and debate to develop understanding and positions on complex topics that affect patients, such as data and AI.
• Human governance needs translation into the digital space, with layered, distributed and democratic governance models instead of strongly centralised, techno-autocratic models.
• The approach to data use should be risk-appropriate, similar to the benefit/ risk trade-offs we see with drugs.
• “Zero-trust” environments enable trust-by-design and be beyond the control of single individuals, authorities or institutions, and governments to ensure that the rights of the individual are protected
• Approaches should be future-proof, taking into account ‘the unknown unknowns’, and not only consider risk to today’s but also to future generations.
• Governance needs to ensure that those most affected by the risks have a voice.
• Broad ethical debate is now needed to keep pace with technological progress
• Proactivity is critical, TestBeds and regulatory Sandboxes can help to systematically evaluate new technologies and increase the likelihood to also uncover unexpected consequences while providing all stakeholders involved with valuable learning opportunities.
The community agreed that there was a moral imperative to use data while patients and societies stood to benefit from it. The value of the data thereby did not reside in the data itself but rather the insights generated from it, rendering data access, data flow as well as quality and quantity important value components. It was pointed out that data was not a homogenous category but rather that different types of data came with different levels of sensitivity and risk to the individual or groups of inviduals, such as genomic data. This should warrant a risk-based approach to data-use, not unlike in the regulation of medicines where benefit/ risk tradeoffs are strongly context-dependent: a treatment for an untreatable cancer will be met with different expectations than a vaccine intended for use in healthy individuals.
It was further noted that, while there might be a moral imperative for use, the consequences of accidental or malicious misuse were unevenly born and go beyond the original core population in question, so while a situation of high unmet need might justify the acceptance of a high level of risk, for example by broadly sharing genomic and clinical information, inheritable germline information derived from that setting could then be used not only for the
patient but also against family members and descendents, e.g. in the form of discrimination in an insurance setting.
It was pointed out that negative consequences could arise despite original good intent; systems therefore needed to monitor not only where and how data was collected, used, transformed and re-used but also how and for what purpose the resulting information and insights were used, with the capability and mandate to intervene, within an overarching legal and governance framework.
Data governance thereby referred to both data management and the overarching regulation. Participants highlighted that, given the current speed of technological development, such a governance model needed to be flexible and agile enough to anticipate and respond to future developments to avoid becoming obsolete too soon. The core idea was that governance models and ethics needed to co-evolve with technological advances to ensure data regulation was ‘future-proof’. Governance frameworks should thereby be able to address not only expected but also unforeseen risks, ‘the unknown unknowns’ and not only consider the present risks but also that to future generations.
The community agreed that in order to be trustworthy, such a governance model could not depend on trust in single individuals, institutions, authorities or governments but needed to be enshrined in the structure of the system itself, ensuring ‘trust-by-design’.
Centralisation was seen as a major risk because of the undue concentration of power and as a barrier to equitable development. In contrast, the community saw a layered governance model that distributed decision power and authority across multiple entities or individuals in a ‘checks-and-balances’ type of fashion as considerably more capable of promoting transparency, accountability, and collaboration, ultimately resulting in trust across the entire (healthcare) ecosystem.
Broadest possible multi-stakeholder engagement, involving patients, healthcare providers, researchers, innovators, the commercial sector,
regulators, policymakers and other relevant actors, across all levels was seen as important to ensure legitimacy of the system and as essential for ensuring that the needs and concerns of the respective communities were appropriately .
In light of the far-reaching implications of data use and in particular, AI, broad ethical debate involving society at large was considered important, with particular attention to ensuring that those who carry the largest risk had a voice.
Overall it was felt that proactivity, rather than reactivity, was critical and that more research, systematic testing and learning, e.g. in the form of Testbeds or regulatory Sand Boxes was needed to develop effective governance mechanisms that safeguard patients and society while enabling innovation in healthcare delivery and research.
• Diverse mitigation: we need more research and concepts to address the handling of data and in particular, AI, in a way that is privacy-preserving and protects the individual and societies. The approach should be systematic and forward-looking, with clear assurance levels and leveraging diverse mitigation strategies as no single solution will be able to address everything.
• Anticipation: Monitoring and anticipation of future privacy breaches through new technologies or methods is needed to ‘stay ahead of the curve’.
• Accountability: Incentives and legal frameworks are critical to hold entities accountable ‘it’s all about security, not about privacy’.
• Data minimisation: payment could be an effective incentive for data minimisation, increasing privacy and reducing environmental impact.
The European Artificial Intelligence Act12 provides comprehensive rules for trustworthy AI, including a risk-based approach, the obligation that highimpact General Purpose AI models with systemic risk must conduct model evaluations, assess and mitigate systemic risks and must conduct adversarial testing and requires that data used to train such models are subject to appropriate state-of-the-art security and privacy measures.
Anonymous data is GDPR-exempt, making it attractive for developers and theoretically, also patients.
However, privacy attacks on Machine Learning Models have been able to reveal private information from training data, making privacy-preservation an important topic for AI in healthcare due to the particular sensitivity of the underlying data.
Privacy thereby finds itself in a trade-off with accuracy and performance: these need to be explored at different stages
in the lifecycle of a machine learning model and require a set of different measures to ensure that a model is privacy-preserving. For example, a combination of data masking, modelguided model anonymisation and model data minimisation is used for iToBoS.
Privacy-preservation is a research topic in itself that needs to keep pace with the development of new machine learning models and needs to anticipate future privacy attacks. Incentives and legal frameworks are thereby critical to hold entities accountable for privacy breaches. Data minimisation is part of the privacy-preserving toolkit, financial incentives (pay for the data ‘unit’ used) could provide an effective stimulus for the development of machine learning models relying on as little data as possible, simultaneously reducing the environmental impact of AI.
1 EU AI Act: first regulation on artificial intelligence | Topics | European Parliament. https://www.europarl.europa.eu/topics/en/article/20230601STO93804/eu-ai-act-firstregulation-on-artificial-intelligence.
2 Artificial intelligence (AI) act: Council gives final green light to the first worldwide rules on AI - Consilium. https://www.consilium.europa.eu/en/press/pressreleases/2024/05/21/artificial-intelligence-ai-act-council-gives-final-green-light-tothe-first-worldwide-rules-on-ai/.
www.mpneurope.org
• Data use for societal good is an ethical imperative; it must however be balanced with cautionary measures to protect from undesired consequences and abuse.
• Health data is common goods; it is not acceptable that value and benefit is extracted for a few while society is settled with the risks and negative consequences.
• Inherent tensions between individual and societal interests need to be recognised and addressed fairly, according to ethical principles based on broad societal consensus.
• Bias in medical datasets is of particular concern as it exposes subpopulations of patients to increased risk and can increase inequity.
• Independent ethical frameworks for data use and AI are needed; these frameworks need to be based on broad societal consent.
• We need citizen ethics and independent citizen ethics boards to ensure citizens have an effective voice.
• We need effective, ethical frameworks for AI and data use that keep pace with the current technical development.
• Ethical and legal frameworks need to be integrated to ensure ethical principles are implemented.
The community agreed that it was an ethical imperative to use data and technology for the good of society and patients as a particularly vulnerable group. At the same time, effective measures needed to be in place to protect individuals, groups of individuals and societies from undesired unintended consequences and from deliberate misuse.
The community considered health data as a common good and that the equitable distribution of benefits and risks across all members of society needs to be assured1.
The group acknowledged that the interests and needs of the individual and those of the group could be at tension or at odds with each other and that processes needed to be in place in order to resolve these situations to prevent ‘stalemate’ situations harming everyone.
Further, the ethical implications of bias, e.g. ethnic, gender, geography, socioeconomic, in datasets was discussed and of particular concern to the
community. Considering melanoma care, bias in datasets could result in missed diagnoses, inappropriate treatment, or overlooked treatment options, potentially jeopardising patient outcomes and resulting in inequity as novel algorithms e.g. worked better for some patient populations than others.
Ethical frameworks for data use and AI should therefore provide guardrails ensuring that technologies are designed, implemented, and utilised in alignment with consensus-based ethical considerations to guarantee fairness and accountability.
While multiple ethical frameworks are being developed, e.g. frameworks for data use in the European Health Data Space EHDS1 2, the Data Ethics Framework for the US Federal data strategy3 and the UNESCO AI stewardship rules4 , the community felt that, considering the profound effect of data and AI on humanity, broader societal involvement and a new form of Citizen Ethics was needed.
1 Staunton, C., Shabani, M., Mascalzoni, D., Mežinska, S. & Slokenberga, S. Ethical and social reflections on the proposed European Health Data Space. Eur. J. Hum. Genet. 32, 498–505 (2024).
2 Dataetisk Tænkehandletank. https://dataethics.eu/.
3 fds-data-ethics-framework.pdf. https://resources.data.gov/assets/documents/fds-data-ethicsframework.pdf.
4 Ethics of Artificial Intelligence | UNESCO. https://www.unesco.org/en/artificial-intelligence/ recommendation-ethics.
www.mpneurope.org
• In medical care, errors in AI risk to cause direct patient harm. Validation strategies of medical AI algorithms are therefore extremely important, including model interpretability, explainability and reliability.
• Explainability depends on the audience and AI models are increasingly becoming incomprehensible for humans. Society needs assurance and security in AI that go beyond explainability.
• Medical data is sensitive by nature and AI models trained on it can inadvertently reveal privacy-endangering information. Strong guidelines and methods for the development of AI training data need to ensure that the privacy of the people whose data is used is ensured, by e.g. ensuring that personallyidentifiable information (PII) is excluded and/or adequately anonymized.
• Bias in data sets can place patients at risk. AI models would benefit from greater peer review of training strategies, including system prompts, reinforcement learning parameters, and fine tuning, to catch the potential for bias.
• Patients should be made aware when and where AI has been used in their care pathways.
• Over-reliance on algorithms represents a danger in itself as it creates dependencies on the availability of those algorithms, e.g. also sufficient energy supply, and as the unfettered amplification of hidden biases in datasets can lead to grave errors and harm.
In light of recent developments, Artificial Intelligence occupied a large part of the discussion at the consensus meeting.
‘Artificial intelligence systems have become increasingly prevalent in everyday life and enterprise settings, and they’re now often being used to support human decision-making. These systems have grown increasingly complex and efficient, and AI holds the promise of uncovering valuable insights across a wide range of applications. But broad adoption of AI systems will require humans to trust their output.
When people understand how technology works, and we can assess that it’s safe and reliable, we’re far more inclined to trust it. Many AI systems to date have been black boxes, where data is fed in and results come out. To trust a decision made by an algorithm, we need to know that it is fair, that it’s reliable and can be accounted for, and that it will cause no harm. We need assurances that AI cannot be tampered with and that the system itself is secure. We need to be able to look inside AI systems, to understand the rationale behind the algorithmic outcome, and even ask it questions as to how it came to its decision’
Source https://research.ibm.com/topics/trustworthy-ai
According to the Ethics Guidelines for Trustworthy Artificial Intelligence of the High-Level Expert Group on AI set up by the European Commission, trustworthy AI is lawful, ethical and robust1
The Guidelines put forward a set of 7 key requirements that AI systems should meet in order to be deemed trustworthy:
Human agency and oversight: AI systems should empower human beings, allowing them to make informed decisions and fostering their fundamental rights. At the same time, proper oversight mechanisms need to be ensured, which can be achieved through human-in-the-loop, human-on-the-loop, and human-in-command approaches
Technical Robustness and safety: AI systems need to be resilient and secure. They need to be safe, ensuring a fall back plan in case something goes wrong, as well as being accurate, reliable and reproducible. That is the only way to ensure that also unintentional harm can be minimized and prevented.
Privacy and data governance: besides ensuring full respect for privacy and data protection, adequate data governance mechanisms must also be ensured, taking into account the quality and integrity of the data, and ensuring legitimised access to data.
Transparency: the data, system and AI business models should be transparent. Traceability mechanisms can help achieving this. Moreover, AI systems and their decisions
should be explained in a manner adapted to the stakeholder concerned. Humans need to be aware that they are interacting with an AI system, and must be informed of the system’s capabilities and limitations.
Diversity, non-discrimination and fairness: Unfair bias must be avoided, as it could could have multiple negative implications, from the marginalization of vulnerable groups, to the exacerbation of prejudice and discrimination.
Fostering diversity, AI systems should be accessible to all, regardless of any disability, and involve relevant stakeholders throughout their entire life circle.
Societal and environmental well-being: AI systems should benefit all human beings, including future generations. It must hence be ensured that they are sustainable and environmentally friendly. Moreover, they should take into account the environment, including other living beings, and their social and societal impact should be carefully considered.
Accountability: Mechanisms should be put in place to ensure responsibility and accountability for AI systems and their outcomes. Auditability, which enables the assessment of algorithms, data and design processes plays a key role therein, especially in critical applications. Moreover, adequate and accessible redress should be ensured.
1 EU COMMISSION, ETHICS GUIDELINES FOR TRUSTWORTHY AI . https://digital-strategy. ec.europa.eu/en/library/ethics-guidelines-trustworthy-ai.
www.mpneurope.org
Trustworthiness includes independent validation and verification
Essential aspects of trustworthy AI were reviewed, such as AI systems that demonstrate dependability, transparency, precision, coherence, and ethical conduct throughout their entire lifecycle, spanning from conception and design to deployment and operation.
Several strategies to achieve trustworthiness in AI were discussed, such as the concept of explainable AI, establishing clearer guidelines and consensus regarding training data sets, independent validation of training strategies, implementing privacy safeguards through the utilisation of AI models with anonymised datasets, and adopting practices to mitigate the risks associated with data bias.
Independent validation of AI models was discussed as a measure to increase
trustworthiness in AI. However, closedsource model code and weights make it impossible to independently verify algorithms.
Explainability- analysing based on which factors an algorithm makes decisions- was discussed as another way to increase the precision and trustworthiness of AI. It was however noted that the approach was slow and relied on open models.
It was also pointed out that as model sophistication scales up by orders of magnitude in the coming years, models will reach a point where human model comprehensibility will no longer be practical nor even possible1 , 2, increasing the importance of tools that allow to evaluate AI algorithms in ways that go beyond explainability.
1 Introduction - SITUATIONAL AWARENESS: The Decade Ahead. https://situational-awareness.ai/.
2 OpenAI Believes Superhuman AI Could Emerge Within the Decade and is Giving $10M in Grants to Solve the Alignment Problem. https://www.maginative.com/article/openai-launches-10-million-in-research-grants-foraligning-superintelligent-ai/.
The uncritical use of AI has been reported to lead to discrimination in e.g. hiring processes1 or insurance decisions2 , 3, caused by biases in the training data sets and lack of human oversight, raising concerns for ‘algorithmic discrimination in health care’4. In medicine, the uncritical use of AI can lead to direct patient harm and therefore requires particular attention to accuracy, reliability and sensitivity to biases.
Model alignment ensures that AI models are consistent with ethical principles but remains a very difficult area of AI research, with considerable resources required to ensure model safety and transparency.
The community was therefore concerned about recent developments in the commercial AI world that, in the heated
race to ever more powerful AI models, downsized rather than increased their safety and alignment teams5 , 6
A further topic of discussion was the danger of over-reliance on AI to answer in particular difficult questions where the underlying dataset might not provide sufficient information and models started producing hallucinations that could lead to serious human harm if left unchecked.
The community felt it important for patients to be aware when and where AI had been used during their care and that the use could be both positive and negative.
1 Chen, Z. Ethics and discrimination in artificial intelligence-enabled recruitment practices. Humanit. Soc. Sci. Commun. 10, 567 (2023).
2 Obermeyer, Z., Powers, B., Vogeli, C. & Mullainathan, S. Dissecting racial bias in an algorithm used to manage the health of populations. Science 366, 447–453 (2019).
3 Celi, L. A. et al. Sources of bias in artificial intelligence that perpetuate healthcare disparities—A global review. PLOS Digit. Heal. 1, e0000022 (2022).
4 Wójcik, M. A. Algorithmic Discrimination in Health Care: An EU Law Perspective. Heal. Hum. rights 24, 93–103 (2022).
5 OpenAI’s Long-Term AI Risk Team Has Disbanded | WIRED. https://www.wired.com/story/openaisuperalignment-team-disbanded/.
6 Microsoft lays off AI ethics and society team - The Verge. https://www.theverge. com/2023/3/13/23638823/microsoft-ethics-society-team-responsible-ai-layoffs.
• Data should be used but not abused. Individuals and societies need to be effectively protected against abuse; this will require new ethical frameworks for the use of data and AI, diverse risk mitigation strategies, effective enforcement and proactivity and agility in the approach.
• It is evident that tech is not able to self-regulate, control therefore has to occur at the level of laws and regulations. Citizens and patients need hard guardrails of what is permissible when it comes to data-use, real-time monitoring and effective enforcement, e.g. existential fines to ensure compliance. datasets can lead to grave errors and harm.
It has become evident that Big-Tech is unable to self-govern, e.g. in the recent case of 23andMe1. The community agreed that as ‘the horse had bolted’ and the technology was already widely available, the only way forward was appropriate regulation, independent expert supervision of processes and effective enforcement of sufficiently punitive up to existential sanctions to the offending organisation to ensure compliance.
Protections therefore have to be enshrined in law and would ideally be based on global agreements and treaties to ensure encompassing enforcement.
While patients and citizens will have the final say when it comes to sharing their data, the community felt that additional, independent expert oversight was needed to protect patients and citizens. The community is concerned that the additional burden of consenting placed on patients who are already preoccupied with their personal disease might lead
to default reactions, either systematic opt-out with the risk of insufficient contribution of data or systematic optin, exposing patients to risk. Further, consequences, in particular, longterm consequences, can be difficult to oversee and to judge as a lay person, warranting independent expert oversight safe-guarding in particular, vulnerable populations such as patients.
The community discussed that this independent oversight of processes to ensure that data use, e.g. for AI, does not harm citizens needed to be ongoing as current- day ethics boards only provide a single point assessment and are often mainly concerned with mitigating risks to organisations.
The community agreed that oversight needed to be balanced, fair and appropriate not to unduly deter progress- ‘Just saying NO all the time is no option’.
1 As 23andMe Begins Its Death Spiral, Your Genetic Data is Up for Grabs | American Council on Science and Health. https://www.acsh.org/news/2024/10/10/23andmebegins-death-spiral-your-genetic-data-grabs-49043.
• Data use is an ethical imperative as people are dying and we as society have data that could save them.
• Data should be used but not abused. Individuals and societies need to be effectively protected against abuse; this will require new ethical frameworks for the use of data and AI, diverse risk mitigation strategies and proactivity and agility in the approach.
• We need proactive and inventive, not just defensive approaches to AI.
• Regulatory and ethical frameworks are not keeping pace with current technological development, we need more agile processes involving society at large.
• Protected experimental environments and regulatory sandboxes, e.g. TEF Health1, are one important tool to monitor for unintended consequences of AI technologies.
• The combination of genomic/ molecular data and AI is impactful and a topic of major importance in the near future; it brings the specific issues and tensions with regards to data ownership, risk, privacy and right of the individual and value extraction and business models to the point.
The community felt very strongly that in the face of suffering, the use of data for societal good was a moral imperative. At the same time, the community was concerned about the risks to patients themselves and in particular, their families. It also noted that the ‘horse had bolted’ and that society and regulators now needed to be faster, more innovative and agile to take a proactive, rather than reactive stance on, in particular, AI.
The community felt that regulatory and ethical frameworks were lagging behind current developments and that a broader societal consensus was needed to make sure that new technologies aligned with societies’ needs and interests as well as personal rights.
The community agreed that even with best intent, unintended consequences of AI should be expected and therefore
needed to be anticipated with sufficient monitoring and e.g. protected experimental environments and regulatory sandboxes.
The community was particularly concerned about the combination of genomic data and AI as it brings the specific issues and tensions with regards to data ownership, risk, privacy and right of the individual, and value extraction and business models to the point.
• Current business and research models based on platforms holding patient data, in particular genomic data, are problematic as they pose a security risk by design and undermine research as they incentivise proprietary behaviour, effectively locking away information that is valuable to patients and society and creating dispersed, non-interoperable data sets.
• We need new business and research models that do not rely on the selling of data or lock-in into non-compatible data platforms or systems; other industries and initiatives could provide interesting models for that.
• Publicly-funded research should not only stipulate rules with regards to data (e.g. FAIR principles) but also provide the necessary support and incentives for researchers, clinicians and institutions; contribution of high-quality datasets should be valued and recognised, e.g. in the form of ear-marked research funding and dedicated support.
The consensus meeting underscored the need for new models for business that relied on data as part of their business model as participants strongly objected
to the current trend of ‘platformization’1 due to its negative impact on patients and society in general.
1 Introduction to the special issue on Locating and theorising platform power. https://policyreview.info/pdf/policyreview-2024-2-1781.pdf.
www.mpneurope.org
It was noted that a lot of research shared similar issues with regards to data-dependency; the consensus scope was therefore extended to ‘new models for data-dependent businesses and research’.
Business and research models based on platforms were considered problematic due to 1. Their inherent security risk due to data aggregation, in particular, of genomic data 2. Their skewed model for value extraction based on platform ownership and access control rather than excellence 3. Their implicit incentives for siloed, non-compatible datasets and lock-ins undermining progress and 4. The resulting risk of bias, further amplified by the use of AI, in research findings, products and services.
‘Competition on brain, not on access’
Platform-based approaches effectively lock valuable datasets away and limit it to the capabilities, resources and personal interests of the commercial or academic platform owner, instead of leading to an open competitive scientific process as e.g. witnessed after
the completion of the human genome project in 2003 that thanks to its open availability dramatically accelerated research across diverse fields.
This (platform-based approach) therefore not only slows but also biases scientific progress as data use and collection will be driven by commercial, rather than societal interest and the desire to slow down unwanted competition. At the same time, the provision of high-quality data including collection and curation needs to be supported and appropriately rewarded and other industries, e.g. the royalty system of the music industry, could provide interesting models for that.
Wanted: viable, alternative business models
At the same time, businesses intending not to sell e.g. genomic or other data currently struggle to develop viable business models and to attract investment, as e.g. also previously seen with the open-source platform IMPUTE.ME1. There is therefore a great need to come up with viable, alternative business models that do not rely on the commercial exploitation of data itself.
1 Folkersen, L. et al. Impute.me: An Open-Source, Non-profit Tool for Using Data From Direct-to-Consumer Genetic Testing to Calculate and Interpret Polygenic Risk Scores. Front. Genet. 11, 578 (2020).
www.mpneurope.org
• We as patient community need self-directed settings for learning and exchange with experts to analyse and discuss relevant complex topics to develop informed, independent positions in the sense of boundary integration.
Patients frequently observe that a diagnosis renders them 'lay per definition' in the eyes of other stakeholders, irrespective of their personal professional background, experience and expertise.
This creates two false dichotomies: a patient is not a lay person per definitiona cancer diagnosis does not remove a professional background. At the same time, any expert will only be an expert in their usually narrow subject of expertiseand a lay person for anything else concerned.
That misconception is perpetuated by the fact that, despite the known heterogeneity of patient communities with regards to background, patient representatives are solely selected based on their disease experience, not whether they possess the relevant technical expertise to meaningfully contribute to a given topic.
In reality, we frequently witness a process of boundary integration between a person's disease experience and professional background, similar to the phenomenon described between work and private life1
According to MPNE principle No 5 'it's a feature, not a bug', MPNE considers the heterogeneity of its network an opportunity and seeks to actively leverage the diverse professional backgrounds, including for MPNEconsensus 2024.
The patient consensus on Data, AI and data-dependent models for business and research was MPNE's second consensus meeting and the first time leveraged internal as well as external expertise.
It demonstrated yet again the value of having a patient-led convening platform for the patient advocacy community as well as relevant experts around items identified as relevant by the community itself.
The MPNE community believes that creating these patient-led spaces for learning and debate are critical for patient advocates to integrate between their patient advocacy expertise and diverse technical areas.
Meaningful and constructive positions need to be able to demonstrate how a certain factor- policy, technical design, business model- affects patients and in return, translate patient prefences into appropriate and feasable solutions, e.g. trial designs.
The MPNE community does not only see its patient-led spaces important for its own community but also as an opportunity for other stakeholders to experience a patient-defined framing of a given topic.
1 Cobb, H. R., Murphy, L. D., Thomas, C. L., Katz, I. M. & Rudolph, C. W. Measuring boundaries and borders: A taxonomy of work-nonwork boundary management scales. J. Vocat. Behav. 137, 103760 (2022).
Providing effective feed-back for current European and national data projects has been challenging for our communities. While initiatives tended to clearly articulate the political, economic and strategic interests of data sharing and in particular, the European Health Data space (EHDS), they failed to provide an equally compelling and concrete value proposition for individual patients and citizens. At the same time, altruism was considered as sufficient motivator to gain individuals’ support, with appeals to trust rather than effective mitigation strategies against harm being in place.
That omission appears rather careless, as thanks to the foresight of those who drafted the General Data Protection Regulation (GDPR), patients and citizens hold the final say on the use of their data, making consent granted at the level of the individual the critical ‘lynchpin’ to the success of the entire EHDS.
For us as patient community, this comes as a major concern.
While survival in Melanoma has dramatically improved in the last decade, we still lose half of our patients with advanced cutaneous Melanoma and an even higher proportion of those suffering from rare Melanomas. At the same time,
optimisation of treatment and care is still needed to improve the Quality of Life in those who survive.
Our community sees the EHDS as a critical enabler to generate better, faster and more equitable solutions for patient communities like ours. Tangibly improved patient outcomes would present one of the most concrete examples possible for how the EHDS created value for our societies.
As the realisation of that value critically relies on individuals’ consent, benefit/ risk assessments have to be positive at the level of the individual. This means that benefit needs to be tangible for the individual who also needs to feel protected from potential risk.
The MPNE patient consensus version 2.0 will be published on MPNE’s issuu channel and shared with the MPNE community and interested parties.
It is planned to to further build on and expand the separate sections of the MPNEconsensus 2024 for a future version 3.0, alternating between internal and external rounds of consultation and deliberation.
To move from abstract position to concrete solutions, we are exploring
2 options: to work through concrete, existing data proposals and projects to better understand the pros and cons from a patient perspective and to further explore user-driven prototyping/ design of data-based applications, e.g. GILLYWEED 'Sync not Sink our data'1
1 Sync not Sink our data – we need more cancer patient agency in health data use. https://www.mpneurope.org/post/sync-not-sink-our-data-we-need-morecancer-patient-agency-in-health-data-use.
Wednesday, 31st January 2024
18:00 A Pint of Science with Andrew Evans on Large Language Models
Thursday, 1st February
9:00 Welcome and Introduction
Bettina Ryll MD, PhD, Founder MPNE
9:30- 10:30 Oxford debate on
“This house believes that patients should be compelled to give up their data for whatever purpose the state sees fit; if they wish to benefit from solidarity-based universal healthcare”
Moderated by Robert White, MPNE
11.00-11.45 Data security and privacy.
Ariel Farkash, IBM and IToBos project partner
13.30-14.00 Dark Side of AI: Tricky poems and why patient advocates should care about Large Language Models
Andrew Evans, MPNE
14:00 - 15:30 Overview and progress on TEF-Health (Testing and Experimentation Facility for Health AI and Robotics)
Johanna Furuhjelm, TEF-Health
15:30 - 16:30 Sharing data is hard, sharing genomic data is….maybe not even a good idea?
Marie-Laure Yaspo, Max Planck Institute for Molecular Genetics and Alacris Theranostics
17:00 - 18:30 What is the ethical compass when we talk about health data in the pursuit of EthicalAI?
Robin Renwick and Sarah Murray, Trilateral Research, IToBoS Project Partner
Friday, 2nd February
9:00 - 10:30 Artificial Intelligence We Can Trust
Sebastian Roland Lapuschkin, Fraunhofer Institute, iToBoS partner
11:00 - 12:30 Trust by Design in No-Trust Environments
Philippe Page, The Human Colossus Foundation
13:30 - 15:00 MELCAYA: Healthcare System Implementation of New Technologies and AI
Lukas Heinlein and David Krauss-Roskamm, German Cancer Research Center DKFZ and MELCAYA
15:30 - 17:00 Practical Session - Shaping AI for Melanoma Patients
Facilitated by Philippe Page, The Human Colossus Foundation
Saturday, 3rd February
9:00 - 12:30 Review of the consensus statements Consolidation
Melanoma Patient Network Europe, MPNE, is a community of Melanoma patients, carers, and patient advocates focused on collaborative learning and sharing knowledge related to Melanoma. Their mission is to systematically address problems faced by the European Melanoma community in a constructive, result-oriented and evidence-based manner. MPNE collaborates to (1) effectively share knowledge relevant to Melanoma such as novel therapies, clinical trials, access schemes, research results, updates about regulatory and Health Technology Assessment decisions, (2) build capacity by sharing successful advocacy projects, knowledge and resources and support others to get started in Melanoma advocacy and (3) create a platform to interact as a group with other stakeholders and to serve as a neutral convenor.
https://www.mpneurope.org/
Intelligent Total Body Scanner for Early Detection of Melanoma (iToBoS) is an AI-driven project funded under the EU Horizon 2020 research and innovation programme. Composed of a consortium of 20 partners, the project aims to develop a diagnostic platform for early detection of melanoma. It involves creating a total body scanner and a Computer Aided Diagnostics (CAD) tool that integrates various data sources like medical records and genomics data. This approach aims to provide highly personalised, early diagnoses by empowering healthcare practitioners with risk assessments for every mole.
https://itobos.eu/
www.mpneurope.org