
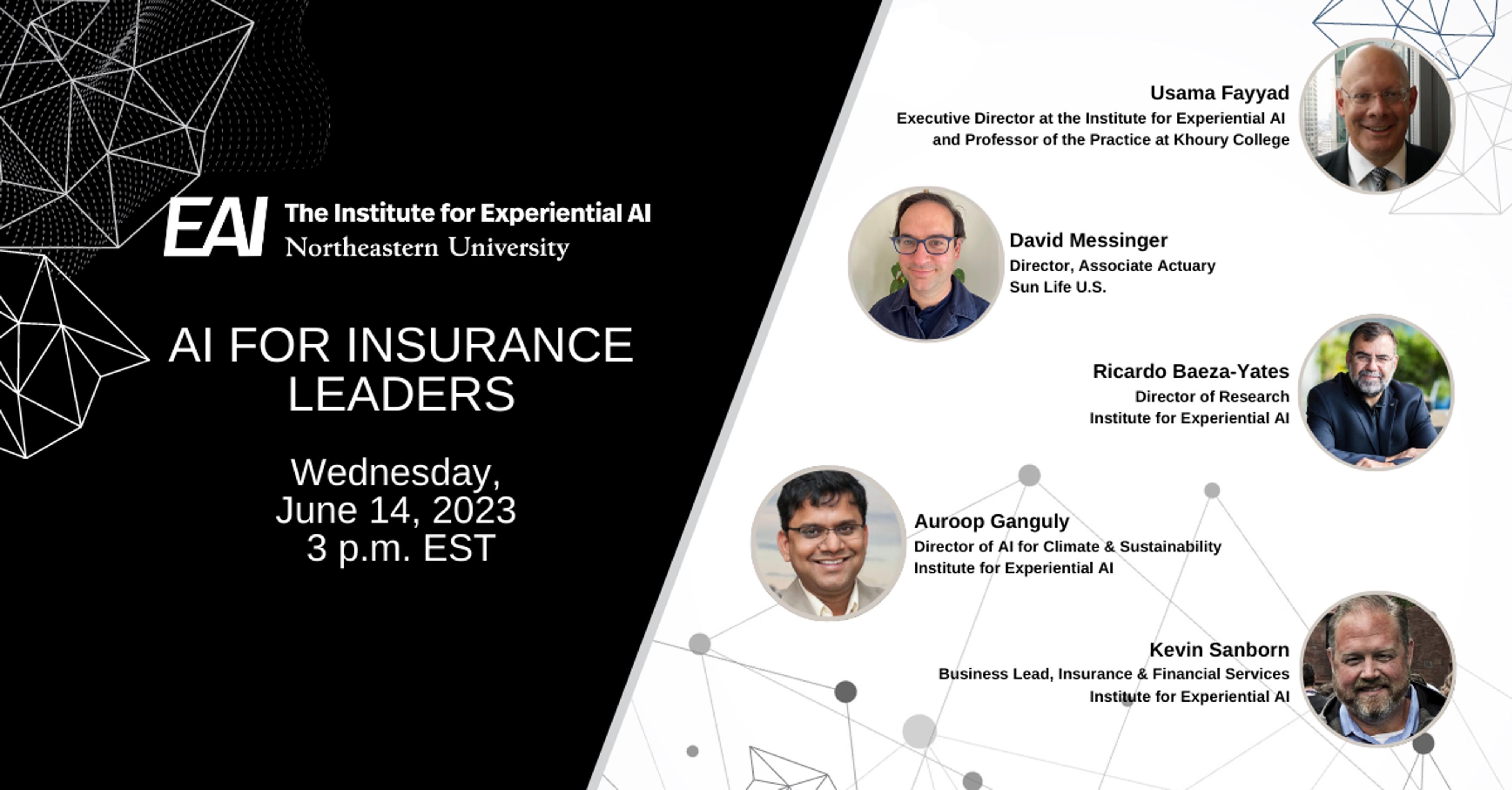
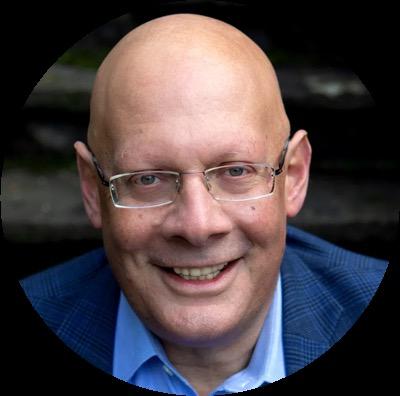
The Institute for Experiential AI at Northeastern University researches and develops humancentric AI solutions that leverage machine technology to extend human intelligence.
Successful AI is not “set it and forget it.” To create real impact, AI must prioritize the human experience and utilize a human in the loop to consistently evaluate and improve its outcomes.
We believe the biggest breakthroughs in the art and science of AI have come and will come through applied projects with real-world data that generate tangible results.
The Institute for Experiential AI exists at the intersection of academia and business. We deliver ROI while integrating industry expertise and talent pipelines to deliver unique value to your most pressing AI challenges.
Usama Fayyad, Executive Director, Institute for Experiential AI at Northeastern University and Professor of the Practice, Khoury College of Computer Sciences
Fireside Chat and Case Study Discussion
David Messinger Director and Associate Actuary, Sun Life U.S. and Usama Fayyad
Responsible AI Governance for Insurance Companies
Ricardo Baeza-Yates, Director of Research and CoChair, AI Ethics Advisory Board, Institute for Experiential AI at Northeastern University
Climate & Weather Modeling in Insurance
Auroop Ganguly, Distinguished Professor of Civil and Environmental Engineering, Northeastern University
Director, AI for Climate & Sustainability (AI4CaS), Institute for Experiential AI
Moderator: Kevin Sanborn
Business Lead, Insurance and Financial Services
Institute for Experiential AI
Large Orgs
Goal: Make AI and Data usable, useful, manageable - democratize the responsible use of AI across fields
Education
● Ph.D. Computer Science & Engineering (CSE) in AI/Machine Learning
● MSE (CSE), M.Sc. (Mathematics)
● BSE (EE), BSE (Computer Engin)
Academic achievements
● Fellow: Association for the Advancement of Artificial Intelligence (AAAI) and Association for Computing Machinery (ACM)
● Over 100 technical articles on data mining, data science, AI/ML, and databases.
● Over 20 patents, 2 technical books.
● First in industry: Chief Data Officer at Yahoo!
● First Global Chief Data Officer & Group Managing Director at Barclays Bank, London
● Chaired/started major conferences in Data Science, Data Mining, AI
● Founding Editor-in-Chief on two key journals in Data Science
Muchofdigitaltransformationhasfocusedonworkflows,butlittleadvanceoncustomerexperienceand interactions
Front Office
Need to scale the human intelligence/ understanding in customer interactions
Back Office
Still expensive and complex and needs intelligent automation
New Frontiers
Going beyond human servicing capabilities
● Understanding customer context
● Understanding intent, challenges, and issues
● Reduce costs of customer service operations (chatbots, problem understanding, problem resolution)
● X-sell, up-sell, reactivation, nextbest-action, etc… at scale
● Compliance
● Claims processing: speed and cost
● “Operations” – e.g. forms into actions
● Step counters linked to insurance risks
● Tracking safe driving with IoT
● Leveraging networks – social and otherwise, to embed financial transactions
Scaleis a must – humanprocessingisnotscalableorfeasible
● AI algorithms are only feasible approach to deal with “understanding” – of context and customer
o Leverage human judgement in delivery to build the right training data sets and KB
o Need to make sure AI algorithmss are subject to Responsible AI criteria (often overlooked)
● Complexity of products requires “reasoning”: still a big challenge in AI
● Multiple ways of recognizing identity: vision, fingerprint, voice recognition, keystroke analysis, etc.
Newcapabilitiestech/networkrequireautomation
● Novel risk and credit scoring opportunities
● Integration into microservices
● Leverage networks (e.g. social networks) and other viral services
● Modeling risk and future scenarios bettersuited for algorithms – aslongasalgosare fair/responsibleAI
o New ways of scoring risk, credit, and needs
o Leveraging all the information available publicly – e.g. LinkedIn and many other networks
o Better understanding of behavioral data
Fairnessandbiasinalgorithmscanhavebigconsequences
● Financial decisions are much more consequential than e.g. targeting ads
● Algorithms have little commonsense reasoning (or any reasoning) –typically all they know is data (with almost no context)
● Modeling complex decisions and consequences is still a hard problem
● Advances in tech and data enable potentially deep intrusion on privacy and civil rights
SuccessfulAIisheavilydependentonML/DataScience, henceneedgoodtrainingdata:Dataremainsahuge challengeformostorganizations
● Good training data is extremely expensive to get
● Just collecting and managing raw data is a challenge for most; growing exponentially with digitization, cloud, and IOT
● Data manipulation is very difficult, few understand unstructured data
Much speculation about pure AI (AGI) or much human intervention?
• Human editorial review is applied
• Some questions are answered by humans
• This is actually a best practice – we call it Experiential AI – many do it:
• Google MLR
• Amazon recommendations
• Many intervention-based relevance feedback
• Curating “just the right training data” for the GPT3 LLM is a costly human-driven activity - essential to LLM performance.
https://mindmatters.ai/2023/01/found-chatgpts-humans-in-the-loop/
● Financial Services has been undergoing major digital transformations for the last 7 decades
● FinTech/InsurTech is a term that represents the super-accelerated tech transformation of the last 2 decades
● AI has gotten a lot of attention in recent FinTech/InsurTech efforts
Simple Example of dramatic transformation in traditional finance: Accounting
○ Think about Accounting of 60 years ago vs today
○ Excel completely replaced many old jobs and “technologies” (like Index cards) but created many more higher value jobs
○ Production chain, supply chain, and inventory management has been completely changed
Incentive-based solution lead to:
● Kodak had a business built on intimate customer relationships
○ Customer goes to store to purchase film
○ Returns to drop off film
○ Returns again for prints
Theshifttodigitaltechnology eliminatedthisecosystem
Kodak invented the digital camera - but was too late to release their own
2012 Bankruptcy
@ 124 years old
Losses double as digital transformation remains at the "thoughtful" stage 2015-2019 competitors embraced on-line and invested in it
2019: revenues drop 5.6%
COVID-19 forced the resolution
2020 Bankruptcy
@ 118 years old
40% reduced cost in hospital admissions
14% cost reduction per patient
25% reduction in hospital stays
Customer interactions data allowed increase its customer retention by 87%
> 80,000 big data experiments a year
> 70% prediction accuracy
83% cost reduction of customer acquisition in 2 years
Vitality integrates with over 100 wearable technologies
Discovery launched its car insurance product (Similar to Drive Safe and Save) in 2011
$4.7M medical cost savings
By 2012 - DBS was considered a failing bank. Start Digital Transformation in 2013
By 2017, application costs reduced by over 80%
Record net profit of SGD $4.39B income up > 4% over last year
9% CAGR in income and 13 % in net profit
> 10,000 training sessions by DBS Academy yearly
-
AI Enablement top priority now
“Ithinkit’sabitofafool’serrand…to chasedigitalforthesakeofcost reduction”
RichardFairbank,CEO
Knowledge worker tasks - 15% to 80% acceleration - an efficiency driver → new types of jobs
Newprocessesfromcustomeracquisition,toretention,toservicing,togrowth
Leveraging BigData and new information sources - Data assets drive new models
Dataoncustomersandbehaviors
○ DataontheWorld - e.g.climateandsustainability
● New ways of risk modeling and assessment
○ Anewgenerationofriskmodelsandstrategiesareenabled
○ Anewgenerationoffrauddetectionandcounter-measures
● A deeper understanding of the customer
○ Consumers,businesses,enterprises
Underwriting, Pricing & Risk
● Underwriting & pricing model optimization
● Use of 3rd-party and unstructured data
● Climate and catastrophe modeling
● Risk modeling and scenario analysis
Customer Service & Personalization
● Dynamic customer profiling & segmentation
● Product recommendation engine
● Intelligent call routing and service intervention
● Agent support
● Chatbot service augmentation
Responsible AI
● Data ethics & privacy
● AI governance
● Model bias audit
● Model transparency and explainability
● Training
Claims Process Improvement & Automation
● Document capture & review
● Claims fraud detection
● Claims scoring and triage
● Intelligent IBNR & Reserving
● Parametric Insurance
● Extracting insights from call/chat logs to drive customer insights and new product ideas
● Climate prediction
● ESG and Sustainability
● New data sources on everything - hyper local and extremely granular
ChallengersusinginnovationsinData,AIandDigitaltodisruptInsuranceindustry
Online Only Insurance Providers - Zhong An is the first online-only insurance provider in China, and since 2013 has sold 7.2 billion insurance products to 429 million customers. DataandAIforonboarding, risk,fraud,underwritingandclaimsprocessing.
Insurance company that’s also hardware company - Neos provides tech that makes gas leaks, water damage and home intrusions less likely, they pass those savings in the form of lower premiums to their customers. AIforinterceptionofissuesandChatbotforcustomerinteractiontoavoidlossandclaims
• Digital, self serve buying process - In January 2017, the life insurance startup Lapetus made headlines by offering a service for people to buy life insurance using a selfie. Biometricanalyticsusedforrisk profiling,coverageandpremiumusingAIandML,enablingdigitalflow.
• Faster, customized claim settlement - Lemonade’s AI Jim made headlines in January 2017 by purportedly settling a claim in less than three seconds. Chatbot,AIforfraudandclaimprocessing.
Improve customer satisfaction and reduce fraud by reducing manual touchpoints in the end-to-end process
User records video footage of the damaged car and uploads on the App
Based on the previous recordings, app identifies the damage
Real time claim assessment is provided to the customer
Customer approves the assessment
Near by service Centre details are provided and claim settled.
Video is stored in the central location
• Tagsgenerated
• Basicmetadata collected
• Entityextraction
• Entityrelationship
• Video Analytics
• Entity Resolution
• Video/Image comparison
Customer 360 provides the Claim history
Real time decisioning engine
• Case flow management system
• Captured document proof for future reference
Traditionally, businesses relied on employee interactions to understand (formally or informally)
Digitization of workflow → can no longer know:
● Are customers happy ?
● Are services and products being delivered effectively ?
● Why are customers leaving us ?
● Where are they going ?
● What is making customers unhappy ?
● What is delighting them?
How can we restore this intimacy on the digital channels?
IllustrativeExample: consider a typical Insurance transaction
Kelly buys new home insurance coverage with her husband
What the insurance agency knows about Kelly
● Kelly buys new home insurance
● Kelly has good standing with the agency
End of Story...
Hadtheagencysynthesizedvarious interactionsandbuiltanunderstandingof Kellyandherintents -
What they could have known
● Kelly just got married
What they could have inferred
● Kelly just started a new family
● Kelly is thinking of buying a new bigger car
What they could have done
● Insurance agency could have offered home and auto insurance bundling offers
● Insurance agency could offer better rates for cars with certain dealers
Butmostbusinessesarenotequippedtoeffectivelymanagedataasanasset
New economy of Interactions is rich with unstructured data in fact, 90% of Data in any organization is UNSTRUCTURED Without proper Data, AI cannot work: ML needs high quality and granular training data
Professor of the Practice, Khoury College of Computer Sciences, Northeastern University
Fullscope, a division of Sun Life U.S.
Industry
Non Profit
Goal: Make Responsible AI the Norm
Education
● Ph.D. Computer Science
● MSE (CSE), M.Sc. (Computer Science)
● BSE (EE)
Academia
● Fellow: Association for Computing Machinery (ACM) and Institute of Electrical and Electronic Engineers (IEEE)
● Over 500 technical articles on search, data mining, data science, AI/ML, NLP, databases, and algorithms.
● Over 40 edited proceedings, 12 patents, and 8 technical books,
● Chaired major conferences on the Web, Search, Data Mining, and Data Science.
● Member of ACM Technology Policy Committee, IEEE Ethics Committee, Global Partnership on AI, among others.
Why Responsible AI?
• Ethical AI?
• Ethics, justice, trust, etc. are human traits
• So, we should not associate “ethical” to a machine
• Trustworthy AI?
• Trust something that does not work all the time?
• Puts the burden in the user
Systems do not need to be perfect, but seems that people wants them to be better than us [Hidalgo
• Belmont Report for biomedical and behavioral research (1979)
• 3 Basic Values
• Autonomy
• Beneficial & No harm
• Justice
• Applications
• Informed consent
• Risk & Benefits Assessment
• Subject selection
Principles Conflict!
Algorithmic Transparency and Accountability (1/2017)
Responsible Algorithmic Systems (10/2022)
e[Baeza-Yates & Matthews, 2022]
1. Awareness
2. Access and redress
3. Accountability
4. Explanation
5. Data Provenance
6. Auditability
7. Validation and Testing
1. Legitimacy and competence
2. Minimizing harm
3. Security and privacy
4. Transparency
5. Interpretability and explanation
6. Maintainability
7. Contestability and auditability
8. Accountability and responsibility
9. Limiting environmental impacts
Awareness
Data provenance
Legitimacy & Competency
Minimizing Harm
Safe & Effective Systems
Algorithmic Discrimination
Protection
Security & Privacy
Transparency
Data Privacy
Notice & Explanation
Explanation
Interpretability & Explainability
Access & Redress + Auditability
Contestability & Auditability
Human Alternatives, Consideration & Fallback
Accountability
Accountability & Responsibility
Validation & Testing
Maintainability
Limiting Environmental Impacts
Idea Design & Development Operation When It Fails When It Harms
Ethical Risk Assessment
Algorithmic Audit Validation & Testing Monitoring Tools
Minimizing Harm
Legitimacy & Competence
Transparency
Security & Privacy
Interpretability & Explanation
Limiting environmental impacts
Contestability & Auditability
Accountability & Responsibility
Maintainability
● 40+ multidisciplinary experts
● Helps organizations develop and deploy AI responsibly
● On-demand, top-level independent ethics guidance
● Tailored to meet company needs
RAI in Insurance:
• Key for underwriting and pricing models adopted by insurance regulators
• Key for model development process without discrimination
Based on the Puzzle-Solving in Ethics (PiE) Model, developed by the AI Ethics Lab.
The PiE Model lays out four core components of AI ethics implementation: Roadmap, Strategy, Analysis, and Training.
PI, Sustainability & Data Sciences Laboratory, Northeastern University, Boston, MA
Chief Scientist, US DOE’s Pacific Northwest National Laboratory
Career Highlights
Goal: Developtransformative HybridKnowledge–AI solutionswithHuman-in-theLoopforgrandchallengesin ClimateandSustainability
● Ph.D. from MIT (USA), B.Tech. (Hons.) from IIT (India)
● Fellow, AmericanSocietyofCivilEngineers(ASCE)
● Senior Member, IEEE
● Senior Member, ACM
● Award winning Climate-AI paper in ACM KDD
● Climate & ML papers in journals such as Nature
● 5+ years at OracleCorporation
● 7 years at US DOE’s OakRidgeNationalLaboratory
● 2 US Patents : Climate Risk & Infrastructure Resilience
● Climate Analytics Startup: risQ(Fortune 500 Acquisition)
● Weather AI Startup: Zeus AI (NASA SBIR Phase II)
● United Nations : Environmental Panels & IPCC Citations
● Climate Assessment (BRAG): ClimateReadyBoston
● Climate Risk (AGU TEX): Town of Brookline, MA
Funding Sources
● Media: New York Times , Newsweek , Independentetc.
Natural Catastrophe (“Nat Cat”) models translate disaster risks to insured losses
Catastrophe modelers, suchasAIR Worldwide, compute risks,insured losses,and insurance Premiums
Performancebaseddesign, gray-greensolutions,and strategicbutequitableretreat canimproveresilience
Intensification of extremes, increased variability,and challengesin predictability,are thehallmarks of globalweirding
The flashiness of flashfloods show an increasing patternacross the continental United States
weather has more than doubled the insured loss over the last 1.5 decades
The US housing market is severely overpriced from unpriced climate risks
Private companieshave developedAI informed software for floodingin countries acrossthe globe
AI-informed data-driven solutions are being developed to manage flood hazards
Ourphysics-aware BayesianDeep Learningenhances functionalmapping andreduces computation
State of the art climate modeling, NatureEducationKnowledge
DeepSD:Generatinghigh resolutionclimatechange projectionsthroughsingle imagesuper-resolution.
Award-winning paper at ACM KDD Conference; Highlighted in Nature 2019 Perspectives
Ourphysics-guided anduncertainty awaredeeplearning methodsmotivated byadvancesin computervision
DeepLearningforDownscalingandBayesian DeepLearningforUncertaintyonSkewed
Data:
• 2017 KDD Conference Award-Winning Paper in the Applied Data Track
• 2018 KDD Research Track Article
Statistical downscaling fills a critical stakeholder gap in climate models
Our award-winningdeeplearning basedstatisticaldownscaling methodshaveshownpromise
Oceanof data from sea surfacetemperatures based onsimulationsand observationshelps process
Explainable deep learning improves water projection from models and data
Ourmethodsforexplainabledeep learningprovidesimproved projectionsandunderstanding
ThesuccessofanSDSLabstartupfocusedonclimateand citiesdemonstratesthepossibilities
Lab students focused on AI and satellite data for renewableenergy sector
Northeastern University engineering alumni
Kate Duffy, PhD’21, and Thomas Vandal, PhD’18, both worked as NASA scientists before creating the new start-up, Zeus AI, which uses AI and machine learning to analyze data from satellites to improve weather forecasting.
• AI is becoming an imperative in the digital ageyet challenging to make work:
• Human intervention a mustforcontinuous correctionofthealgorithmicissues.
• ResponsibleAIisamusttopreserve customertrust,avoidregulatoryand reputationalrisks.
• Customer experience is a key business driver
• No Data ⇒ no working AI
• Getting the data/context story right is the key enabler - for business insights and for AI
• Climate change is increasing the volatility and severity of weather events. New AI methods show promise in helping insurers manage climate risk.
● We at the Institute for Experiential AI are here to work on real challenges so we both learn:
○ Howdoweidentifyopportunitiesfor innovationtogether?
○ Howdoweupskillthein-house talent and leverageresearchersandfacultyaspartof ourbusiness?
● The Institute seeks to work with you to solve real problems to
○ Drive new researchdirections
○ Driveanewwayofexperientialtrainingof studentsand learners
● Get in touch! - Let’sAITogether!