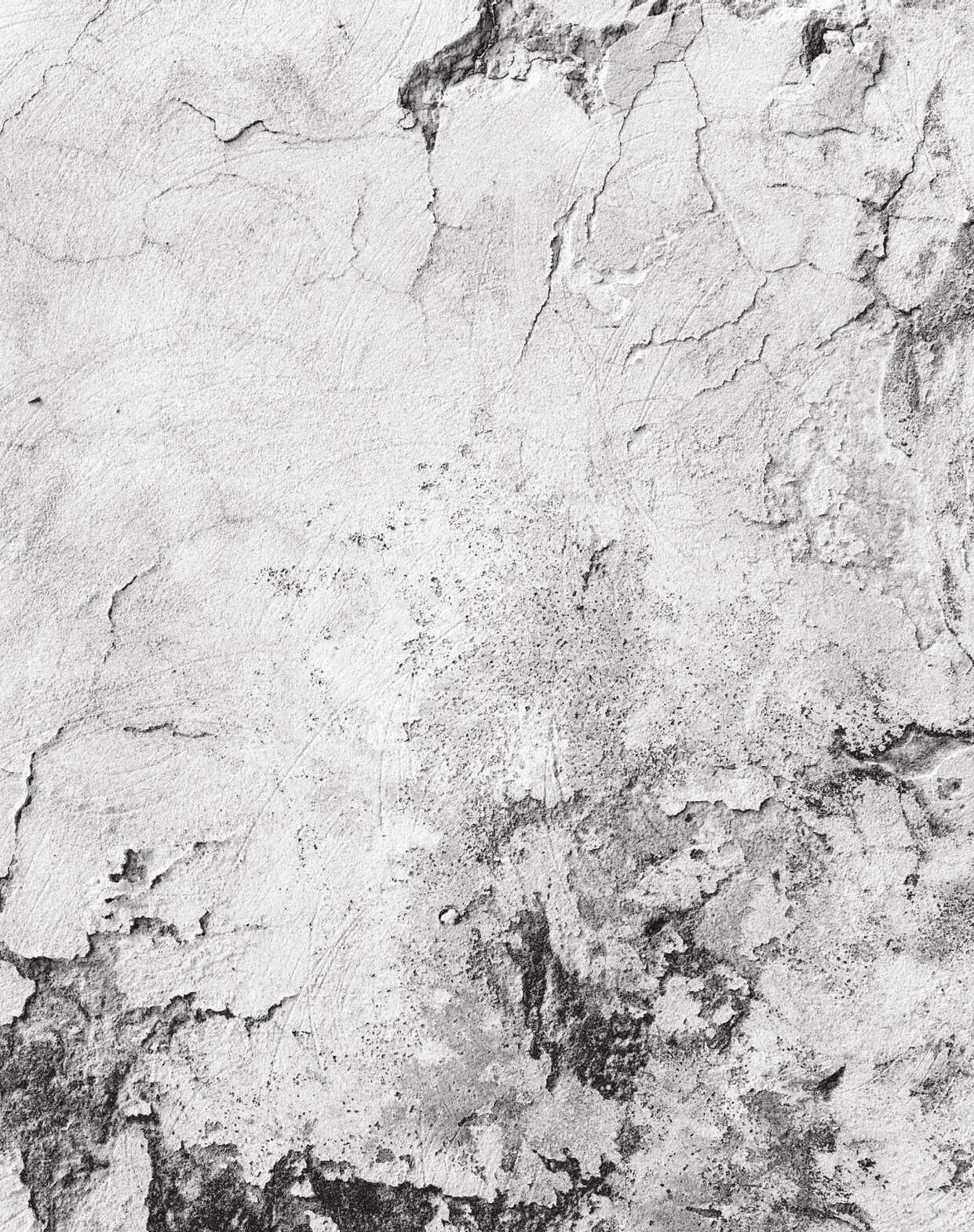
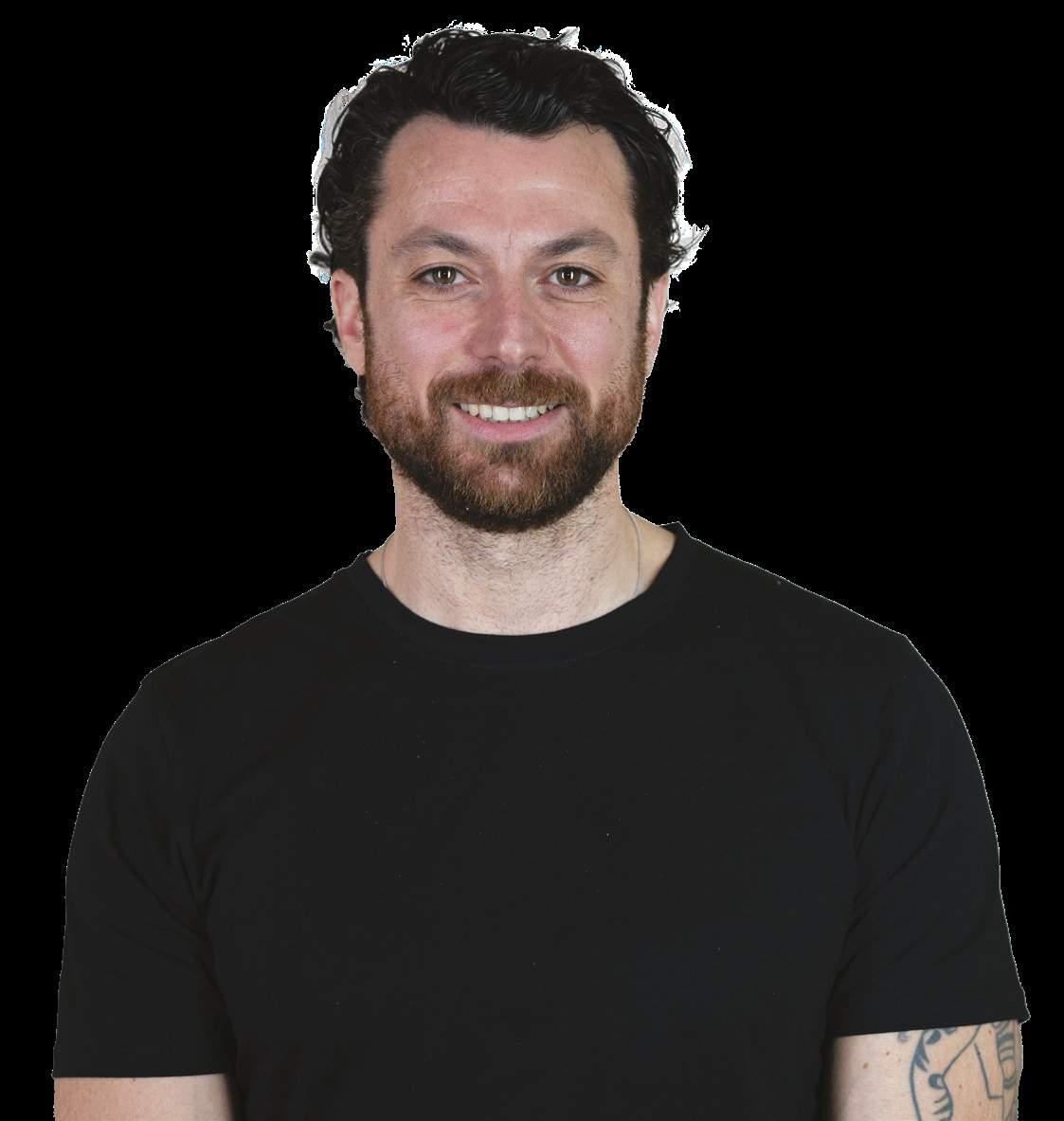
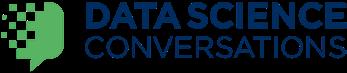
Expect smart thinking and insights from leaders and academics in data science and AI as they explore how their research can scale into broader industry applications.
“Using Open Source LLMs in Language for Grammatical Error Correction (GEC)
BARTMOSS ST. CLAIR
‘One essential aspect is that we don’t just correct the grammar, but we explain to our user why; we give the reason for the correction.’
The Path to Responsible AI
JULIA STOYANOVICH‘Responsible AI is about human agency. It’s about people at every level taking responsibility for what we do professionally… the agency is ours, and the responsibility is ours.’
Transforming Freight Logistics with AI and Machine Learning
LUÍS MOREIRA-MATIAS
‘We’re not designing AI algorithms to replace humans. What we’re trying to do is to enable humans to be more productive.’
Helping you to expand your knowledge and enhance your career.
CONTRIBUTORS
Francesco Gadaleta
Iaroslav Polianskii
Luca Traverso
Anthony Alcaraz
Philipp Diesinger
Gabriell Fritsche-Máté
Stefan Stefanov
Andreas Thomik
Sahaj Vaidya
Gareth Hagger-Johnson
Zana Aston
Georgios Sakellariou
Sandro Saitta
Claus Villumsen
James Duez
Colin Harman
EDITOR
Damien Deighan
DESIGN
Imtiaz Deighan imtiaz@datasciencetalent.co.uk
The Data Scientist is published quarterly by Data Science Talent Ltd, Whitebridge Estate, Whitebridge Lane, Stone, Staffordshire, ST15 8LQ, UK. Access a digital copy of the magazine at datatasciencetalent.co.uk/media.
To set up a 30-minute initial chat with our editor to talk about contributing a magazine article, please email imtiaz@datasciencetalent.co.uk
The views and content expressed in The Data Scientist reflect the opinions of the author(s) and do not necessarily reflect the views of the magazine, Data Science Talent Ltd, or its staff. All published material is done so in good faith.
All rights reserved, product, logo, brands and any other trademarks featured within The Data Scientist are the property of their respective trademark holders. No part of this publication may be reproduced, stored in a retrieval system, or transmitted in any form by means of mechanical, electronic, photocopying, recording or otherwise without prior written permission. Data Science Talent Ltd cannot guarantee and accepts no liability for any loss or damage of any kind caused by this magazine for the accuracy of claims made by the advertisers.
I’m delighted to share our latest issue with you, which features some really insightful articles from our top data experts about the progress of generative AI. Recently, the data community has seen a particular interest in the potential use cases of GenAI, and this issue investigates some of these potential applications – together with its current limitations – in detail. There’s no denying that GenAI has the potential to be of huge benefit to organisations, but it’s just one element of the AI picture.
Generative AI is useful, but it’s just one element of the AI picture and needs extensive work to deliver significant enterprise value. In previous issues, we have tried hard to avoid fuelling GenAI hype and focus on presenting articles that showcase tangible benefits of the technology. Our cover story continues that theme as Francesco Gadaleta, a long-time collaborator of the magazine, delivers an excellent piece on how and why LLMs are promising when used in conjunction with artificial languages in the area of robotics.
LLMs implemented without additional layers will continue to produce underwhelming results that many are experiencing in their enterprise projects. Knowledge graphs are showing a lot of promise in dealing with the hallucination problem, and we double down on our knowledge graph focus in this issue with two features on the topic from James Duez and Anthony Alcaraz that come from different angles. Colin Harman is also back again this issue with a superb summary of why certain GenAI projects fail.
As always, we continue to provide you with content on the latest developments in industry from the world of more traditional data and machine learning approaches. The Fraud Protection team at the Forex technology company Wise have produced a high-quality deep dive into their approach to fraud protection across international boundaries.
Meanwhile Gareth Hagger-Johnson from The Nottingham Building Society brings much-needed attention to the often overlooked role of data quality in algorithmic bias. This is important because this type of bias tends to affect minorities and the vulnerable the most.
Continuing the theme of data quality, Philipp Diesinger and his team have provided an excellent overview of the current challenges that exist in the realm of clinical trials data, which directly impacts the success or failure of a large number of clinical trials every year.
Away from the technical side of data and AI, we have two excellent articles in this issue that focus on the bigger picture of the development of AI. Zana Aston & Georgios Sakellariou give us a rundown of which AI topics were hot on the agenda with world leaders and business leaders at Davos, with sustainability and the need to build trust front and centre. Sahaj Vaidya explains AI constitutionalism and how that can help us make sure that future AI systems are ethical.
Claus Villumsen has written a great overview of what a leader of technology teams needs to get right in order to deliver high performance teams. Data leaders need to have strong communication skills and Sandro Saitta tells you what you need to know about how to communicate well as a data professional.
The AI World Congress takes place in Kensington, London on Thursday 30th and Friday 31st May.
I am really looking forward to speaking there again. The diversity of topics and speakers at the 2023 conference was excellent and the line-up for this year’s event is equally as strong.
In today’s digital age, it is arguably even more important to attend inperson events. Face-to-face interactions are often the most effective way to forge new personal connections and gain new insights. The Congress is one of the highlights of the industry calendar, and I hope to see some of you there.
You can find out more about the conference and grab the last remaining tickets here: aiconference.london
I hope you enjoy this issue of the magazine. If you would like us to run a feature on you or your company in our next issue, we would love to hear from you.
Damien Deighan EditorThe realm of language, encompassing both natural and artificial forms, presents a fascinating paradox. Natural languages like English or Mandarin boast remarkable flexibility and adaptability, yet this very quality can lead to ambiguity and misunderstandings. In contrast, artificial languages, designed with specific purposes in mind, prioritise clarity and structure, often at the expense of nuance and expressiveness. This article delves into the potential of artificial intelligence (AI) and large language models (LLMs) to bridge this gap, harnessing the strengths of both artificial and natural languages.
ARTIFICIAL AND NATURAL LANGUAGES: IS THERE A DIFFERENCE?
We begin by exploring the fundamental differences between these two language categories: natural and artificial languages.
NATURAL LANGUAGES , products of organic evolution through human interaction, are complex and ever-changing, reflecting diverse communication needs. Unlike their meticulously crafted counterparts, natural languages like English and Spanish evolved organically over centuries through human interaction. This organic development led to intricate and sometimes ambiguous structures, allowing them to serve diverse communication needs. Imagine the difference between a language sculpted with a specific purpose in mind, like the clarity and precision of a computer programming language, and the rich tapestry of a
natural language woven from the threads of human experience. That’s the essence of the distinction between natural and artificial languages.
ARTIFICIAL LANGUAGES are in fact deliberately created with specific goals in mind. Whether it’s the deterministic nature of first-order logic ¹ , the efficiency of Python or Rust, or the global aspirations of Esperanto, these languages prioritise clarity and structure to avoid ambiguity. Think of them as tools designed for specific tasks, in contrast to the naturally flowing and multifaceted nature of the languages that people speak every day.
Some artificial language examples are: the languages used in computer simulations between artificial agents, robot interactions, the messages that programs exchange according to any network protocol, but also controlled psychological experiments with humans.
The presence of ambiguity in natural languages, while absent in most artificial languages, stems from several key differences in their origins and purposes. Natural languages have developed over centuries, leading to flexibility and adaptability, but also to inconsistent and sometimes imprecise rules.
They have served diverse communication needs, including conveying emotions, establishing social rapport and expressing creativity. Such multifunctionality leads to subtleties and shades of meaning that are not always explicitly defined. Moreover, natural languages heavily depend on the surrounding context, including the speaker’s intent, cultural background, shared knowledge, etc. This reliance on context can lead to ambiguity too, as the same words can have different interpretations in different situations, cultures, tribes or geographically separated locations.
In contrast, artificial languages are designed with specific goals in mind, like providing clear instructions or facilitating precise communication. This focus on clarity and efficiency often leads to strict rules and unambiguous structures. These other types of language usually have a specific and well-defined domain of application. This allows them to focus on a smaller set of concepts and relationships, reducing the potential for ambiguity. The controlled vocabulary that typically characterises artificial languages eliminates the confusion that can arise from the synonyms, homophones, and other features of natural languages. As an example, homophones, words with the same sound but different meanings (e.g., ‘bat’ – flying mammal vs baseball bat) are present in natural languages but not in artificial languages designed to avoid such confusion. Natural languages often rely on implicit information conveyed through context, tone of voice, or facial
¹ en.wikipedia.org/wiki/First-order_logic
expressions. This is yet another source of ambiguity, as the intended meaning may not be explicitly stated. In contrast, artificial languages strive to be explicit and avoid reliance on implicit information. Natural languages constantly evolve and change over time, leading to multiple interpretations of words or phrases that may have had different meanings in the past. Artificial languages, on the other hand, are typically designed to be stable and resist change.
It’s important to note that not all artificial languages are completely unambiguous. Some, like logic formalisms, have well-defined rules that prevent ambiguity. Others, like programming languages designed for natural language (like syntax) can still have some level of ambiguity due to their attempts to mimic natural language constructs. Overall, the inherent flexibility and context-dependence of natural languages, in contrast to the focused and controlled nature of most artificial languages, are the primary factors contributing to the presence of ambiguity in the former and its absence in the latter.
The recent surge in large language models (LLMs) has unfortunately led to a widespread misconception that these models can truly understand and learn about the world through language. This belief, however, directly contradicts the inherent limitations of natural languages themselves when used as a sole source of knowledge about the world.
While current LLMs can generate seemingly humanlike responses based on context, they are far from truly understanding the world they navigate. Their ‘common sense reasoning’ relies on statistical patterns, not actual comprehension.
This highlights a fundamental limitation: natural language itself is inherently insufficient for comprehensive understanding. Unlike humans and other intelligent beings who draw upon diverse inputs (including non-linguistic and non-textual information), LLMs are confined to the realm of language. It’s akin to assuming complete world knowledge solely by reading an entire encyclopedia – language simply cannot capture the full spectrum of knowledge. It serves as just one, limited form of knowledge representation.
From a technical standpoint, both natural and artificial languages represent information using specific schemas. These schemas describe objects, their properties, and the relationships between them, with varying levels of abstraction. Consider the difference between reading a poem and playing or interpreting music. The ‘interpretation’ required for music adds a significant gap between the written and performed versions, conveying information beyond the literal text. This gap is less pronounced with artificial languages, particularly programming languages, as
we will explore later. Language inherently transmits information with limited capacity. This is why humans rely heavily on context in communication. Often, this context is implicit or already understood, reducing the need for explicit communication.
As a fundamental concept in formal language theory, languages are classified as follows:
1. REGULAR LANGUAGES : These are the simplest type of formal languages and can be recognised by finite automata. They are less expressive than context-free languages.
2. CONTEXT-FREE LANGUAGES : These languages can be recognised by pushdown automata and are more expressive than regular languages. Context-free grammars are widely use for describing the syntax of programming languages.
3. CONTEXT-SENSITIVE LANGUAGES : These languages can be recognised by linear-bounded automata, and they are more expressive than contextfree languages. Context-sensitive grammars allow for more intricate and nuanced language structures.
An example of a grammar to represent one particular construct of a programming language like Rust is provided below. Generally speaking, a grammar is formed by symbols that can be terminal or nonterminal. Terminal symbols are symbols that terminate the string (called production) and after which there are no other symbols. Non-terminal symbols, on the contrary, are symbols that are followed by other symbols. For instance, to declare an integer variable in a language like Rust, a programmer would type the following string:
let x: int = 42;
Such statement contains non-terminal symbols like let and int and terminal symbols like ;
Another important concept of grammars is the concept of production rules . Production rules represent a formal description of how symbols in a language can be replaced or transformed into other symbols. Technically, what is on the left side of the -> produces the expression on the right.
Producing means transforming the string on the left into the string on the right. Here’s an example of a contextfree grammar and some productions to generate a simple variable declaration in Rust (and other similar imperative programming languages like C/C++, Python, Typescript, Go, Java, etc.):
The hierarchy reflects the increasing computational power needed to recognise or generate languages within each category. Context-sensitive languages are indeed more powerful than context-free languages, and context-free languages are more powerful than regular languages.
Practically no programming language is truly context-free. However this limitation is not really important due to the fact that every programming language can be parsed or it wouldn’t be useful at all. This means that any deviations from context freeness can and will be dealt with by the grammar of the language. The grammar of a language is the set of rules that can produce strings that belong to the language. Strings that violate the grammar, cannot be generated by such grammar (by definition). Hence, such strings would not belong to the language (by definition). An example of a tiny grammar representing a typical construct of programming languages is described as follows:
GRAMMAR:
Non-terminals: S, T, V Terminals:
let , : , mut , identifier , int , float , bool
PRODUCTIONS:
1. S -> let V : T (Start symbol with declaration, variable, colon, and type)
2 . V -> identifier (Variable can be an identifier)
3. T -> int | float | bool (Type can be integer, float, or boolean)
4. V -> mut identifier (Optionally, variable can be declared mutable)
EXAMPLE USAGE:
This grammar can generate simple variable declarations like:
• let x: int (integer variable)
• let mut y: bool (mutable boolean variable)
• let name: String (using standard library String type)
LIMITATIONS:
This grammar is a very simple toy and only generates basic variable declarations. It cannot handle more complex features like functions, loops, or control flow statements which would require additional productions and non-terminals. Real grammars have several hundreds of production rules and symbols in order to express and generate all constructs of a programming language in a non-ambiguous way.
In the study of formal languages and automata theory, grammars are used to describe the structure of a language. Context-sensitive, context-free, and regular grammars are three types of grammars that differ in the way they generate strings.
A regular grammar generates a regular language, which is a language that can be recognised by a finite state machine. As for regular languages, regular grammars are the simplest type of grammar and are often used to describe simple patterns in language. For example, the grammar that generates the language of all words that start with the letter ‘a’ and end with the letter ‘b’ is a regular grammar.
This grammar can be represented as the following production rules: S -> aB, B -> b.
CONTEXT-FREE GRAMMARS generate a context-free language, which is a language that can be recognised by a pushdown automaton. Context-free grammars are more powerful than regular grammars and can describe more complex patterns in language. For example, the grammar that generates the language of all matching pairs of parentheses is a context-free grammar.
This grammar can be represented as S -> (S)S | ε.A
CONTEXT-SENSITIVE GRAMMARS generate a contextsensitive language, which is a language that can be recognised by a linear-bounded automaton. Context-
Context-free grammars (CFGs) are a type of formal grammar used to describe the structure of languages. They offer a balance between expressive power and practicality in various applications, making them important for some of the properties that are reported below:
● Rules and Symbols: A CFG consists of a set of rules that rewrite symbols. These symbols can be terminals (representing actual words or punctuation) or non-terminals (representing categories of words or phrases).
● Hierarchical Structure: The rules define how non-terminals can be replaced by sequences of terminals and other non-terminals, capturing the hierarchical structure of languages. (e.g., a sentence can be composed of a subject and a verb phrase, which can further break down into nouns and verbs).
sensitive grammars are even more powerful than context-free grammars and can describe more complex patterns in language. For example, the grammar that generates the language of all palindromes is a contextsensitive grammar.
This grammar can be represented as S -> aSa | bSb | ε
In human language, regular grammars can describe simple patterns like words that start or end with a certain letter. Context-free grammars can describe more complex patterns like sentences with matching pairs of parentheses. Contextsensitive grammars can describe even more complex patterns like palindromes.
While all three grammar types can generate language, their limitations increase when dealing with the complexities of natural language. Context-free grammars offer a good balance between power and practicality for many applications, while context-sensitive grammars offer the most expressive power but come at a higher computational cost and complexity.
The grammars discussed are examples of formal languages used to describe the structure of natural languages. While these formal languages can capture specific aspects of natural languages, they cannot fully replicate the full complexity and nuances that arise from the organic evolution and diverse uses of natural languages. Indeed, particularly in spoken languages, grammar rules are often bent rather than strictly adhered to. Slang and other language variations not only reflect the culture of the speakers but also serve as highly effective means of communication.
● Context-Free Replacement: Importantly, the replacement of a non-terminal with another symbol can happen regardless of the surrounding context. This means the rule can be applied anywhere the non-terminal appears.
CFGs are more powerful than regular grammars, allowing them to describe the structure of complex languages like programming languages and many aspects of natural languages (e.g., handling nested phrases and clauses in sentences). They provide a theoretical framework for building parsers, which are programs that analyse the structure of text according to a grammar. Parsers are crucial for tasks like compilers (understanding programming code) and natural language processing (understanding human language). CFGs can be used as a starting point for building machine translation systems, where the grammar helps identify the structure of sentences in different languages. While CFGs alone
cannot capture all the complexities of natural language, they serve as a foundation for more advanced NLP techniques. Analysing and understanding sentence structure is a crucial step in many NLP tasks like sentiment analysis or text summarisation. Finally, they play a role in the theoretical study of language structure, helping linguists understand how human languages can be generated and parsed.
While context-free grammars (CFGs) provide a theoretical framework for understanding language structure, LLMs have a different and more complex relationship with grammars when it comes to generating and understanding text.
LLMs don’t explicitly use formal grammars. They don’t rely solely on predefined rules like CFGs to generate or understand text. LLMs are trained on massive amounts of text data, learning statistical patterns and relationships between words and phrases. LLMs use this knowledge to predict the next most likely word in a sequence, considering the context of previous words. Through training, LLMs implicitly capture some aspects of grammars, like word order, sentence structure, and common phrasings. However, this capture is not based on explicit rules like CFGs. Instead, it’s based on the statistical patterns observed in the training data.
One of the most tangible benefits of LLMs with respect to CFGs consists in the fact that LLMs can handle more complex structures. They can go beyond the limitations of CFGs, dealing with long-range dependencies and nuances of natural language that are difficult to capture with formal rules. For instance, while CFGs struggle with agreement between distant words, LLMs can learn these relationships through statistical patterns in real-world text usage.
Of course LLMs don’t come without limitations. In particular they are limited by the quality and diversity of the data they are trained on. Biases and limitations in the data can be reflected in their outputs. LLMs can still generate grammatically incorrect or nonsensical outputs, especially in complex or unfamiliar contexts. Such outputs are usually referred to as hallucinations, and they are inevitable. Therefore, the relationship between LLMs and formal grammars is not a direct one. LLMs learn from data and capture statistical patterns, which indirectly relate to and sometimes go beyond the capabilities of formal grammars like CFGs.
While the answer is nuanced, LLMs generally demonstrate greater power in dealing with artificial languages compared to natural languages for several reasons, such as:
● Well-defined structure: Artificial languages, like programming languages
or logic formalisms, have clearly defined rules and structures that are explicitly designed and documented. This makes them more predictable and easier for LLMs to learn from a statistical perspective.
● Smaller vocabulary and simpler grammar: Artificial languages typically have a smaller vocabulary and simpler grammar compared to natural languages. This reduces the complexity involved in understanding and generating valid sequences in the language, making it easier for LLMs to achieve higher accuracy.
● Limited ambiguity: Artificial languages are often designed to be less ambiguous than natural languages. This means there are fewer potential interpretations for a given sequence of symbols, making it easier for LLMs to identify the intended meaning.
However, this doesn’t imply a complete inability to handle natural languages. LLMs have shown remarkable progress in dealing with natural languages, mainly due to:
● Massive training data: They are trained on vast amounts of real-world text, allowing them to capture complex statistical patterns and nuances that may not be explicitly defined by grammar rules
● Adaptability: They can adapt to different contexts and styles based on the data they are exposed to, providing a more flexible approach compared to the rigid rules of artificial languages.
Therefore, while LLMs generally perform better with artificial languages due to their well-defined nature, their ability to handle natural languages is constantly improving due to advancements in training data and model architecture, making them increasingly effective in dealing with the complexities of human language. It’s crucial to remember that LLMs are not perfect in either domain. They can still struggle with complex tasks, generate nonsensical outputs, and perpetuate biases present in their training data.
While LLMs generally perform better with artificial languages due to their well-defined nature, their ability to handle natural languages is constantly improving due to advancements in training data and model architecture, making them increasingly effective in dealing with the complexities of human language.
An aspect that remains to be fully explored and can play a fundamental role in understanding and generating languages, especially artificial ones, is the realm of compilers.
Compilers are programs that translate code written in a high-level programming language (source code) into a lower-level language (machine code) that a computer can understand and execute.
Compilers and LLMs can form a potent combination for several compelling reasons:
1. Enhanced Code Generation: LLMs can facilitate pregenerating code skeletons or suggest completions based on the context of existing code, as is already the case. Moreover, considering the grammar and potential production rules of the language can further elevate the quality of the generated code.
2. Improved Error Detection: LLMs, trained on extensive datasets of code containing identified errors, can effectively pinpoint potential issues in code that traditional compilers might overlook. This capability extends to detecting inconsistencies, potential security vulnerabilities, or inefficiencies in the code structure.
3. Natural Language Programming: Integrating LLMs with compilers can enable the use of more natural language-like instructions for code generation. This approach holds promise for making programming more accessible to individuals who are not proficient in conventional programming languages.
While these ideas serve to illustrate the possibilities, the technology is already sufficiently mature to realise these objectives, except in the realm of natural language. This implies that, probably, we are employing LLMs to address a problem for which they were not originally intended.
At Intrepid AI (intrepid.ai), we envision a future where AI systems not only excel in comprehending and generating artificial languages but also demonstrate remarkable proficiency in navigating the complexities of natural languages.
We invite researchers, developers, and enthusiasts alike to join us² on our journey towards unlocking the full potential of AI-assisted language understanding and generation. Together, we can harness the power of LLMs and compilers to create intelligent robotics systems that redefine the way we interact with and interpret language in the digital age.
² Intrepid AI Discord Server https://discord.gg/cSSzche6Ct
Our vision at Wise is to create a world where money moves without borders – instant, convenient, transparent, and eventually free. We strive to make it as easy as possible for people and businesses around the world to use our products, so they can swiftly move and manage money internationally. However, we also understand that our platform’s size makes it a target for payment fraud and cybercrime. Every fraudulent transaction or action we prevent contributes to our mission by ensuring a positive experience for all involved. In conjunction, we also place emphasis on balancing the negative impact of our preventative measures on genuine customers. To combat fraud within our platform, we have developed an advanced, multi-level system designed to prevent and detect malicious activities. Additionally, we have implemented a comprehensive system of different controls for risk management, which effectively
Online payment fraud is a challenging problem to solve, involving a significant class imbalance (i.e. a data set with skewed class proportions). It’s a highly dynamic environment where bad actors constantly adapt and complicate their methods of attack. Above all, a fraud protection system needs to be accurate, fast, and scalable for scoring large volumes of events. It needs to balance blocking and limiting fraudulent behaviour and minimising false positives that impact the experience of genuine customers.
On the way to creating such systems, data science has emerged as an indispensable asset, empowering analysts, product managers, engineers, designers, and operation agents in their collaborative efforts to combat fraudulent activities. The need for robust fraud protection mechanisms has never been more important. This article explores the typical approaches to fraud protection in the online payments domain, and how these can be extended to tackle the growing challenges of a connected and digital world.
is the senior data scientist in the Compromised Accounts and Scam Prevention team at Wise, where he develops and implements new fraud protection solutions. Prior to this, Iaroslav worked on developing cyber security products, including platforms for identifying threats and conducting OSINT (open source intelligence). He also successfully managed the development of a grant project funded by the Cybersecurity Agency of Singapore.
LUCA TRAVERSO is the lead data scientist of the Servicing function at Wise. He holds a PhD in Applied Mathematics from Cardiff University (UK). Prior to joining Wise, Luca worked in various engineering consultancy companies and governmental institutions across the UK and Australia. He has 20 years of experience working with and developing complex numerical models in various fields of application. At Wise, he oversees the development of machine learning across many domains including fraud prevention and detection.
Fraud is often broken down into distinct typologies, including chargeback fraud, account takeover, and others. This segmentation serves a dual purpose: it not only enhances the precision in monitoring, but also aids in the development of countermeasures against them. The fraud volume is influenced by factors such as seasonal peaks during holidays or sales, the activity level of fraud groups, or the advent of new tools like stealers or phishing kits. However, even within the same typology, different fraud schemes can be applied. In essence, these schemes are descriptions of the specific steps required to successfully carry out an attack.
Fraudulent schemes can be broken down into tactics, similar to those in the MITRE ATT&CK® matrix, which together form a chain of attack. An example of these
tactics could be the interaction with the victim, gaining access to an account, withdrawal and laundering of funds, and other actions (see Figure 1). In turn, each of the tactics can be implemented using one or more techniques. The set of tactics and the type of techniques used can vary depending on the fraud typology (for example, the steps involved during the execution of remittance fraud are generally different from those followed during a scam or an account takeover). Fraud protection systems aim at identifying the weak points in the chain of attack and implementing controls that disrupt (or significantly complicate) the execution of the attack. Well-developed fraud protection systems allow the identification of the intersection of tactics across different typologies, as this enables for developing controls that mitigate multiple fraud schemes at the same time.
At each stage of a chain of attack, protection systems implement solutions that can be divided into two main levels: a prevention stage and a detection stage. The goal of the prevention stage is to develop technical measures and solutions that reduce the range of attack vectors (for instance, using a two-factor authentication (2FA) at login instead of a single password authentication). This security method requires two forms of identification to access an account or system, providing an extra layer of protection beyond a password. Typically, priority is given to these measures, as they prevent the occurrence of fraud and eliminate the task of having to detect it as well as dealing with its consequences.
The goal of the detection stage is to identify fraudulent activity as it occurs. Transactional analysis forms the core of fraud detection systems for payments. While rule-based systems are still widely used by financial institutions for the detection task, in recent years the industry has seen the emergence of detection systems based on sophisticated machine learning (ML) models. Rule-based systems (i.e. static rules) are based on a predefined set of business rules that compose a
risk score for each payment carried out on the platform. Conversely, ML systems analyse vast amounts of data in real-time, including transaction history, user behaviour, and other significant factors to compose a risk score and identify patterns and anomalies that may indicate suspicious activity. Based on this data and calculated risk scores, a fraud protection system generates alerts to carry out further investigations or take actions such as suspending transactions or requesting additional authentication.
Rule or ML-based systems have advantages and disadvantages. Rule-based detection can be implemented quickly and easily. However, rules target specific patterns and thus can be circumvented by bad actors and become outdated as the bad actors’ strategy evolves. Governance and maintenance of rules can also be challenging, especially when a large number is developed over an extensive period of time. In contrast, ML-based systems generalise well to new and emerging patterns as they learn continuously from a vast amount of data. On the other hand, ML model development can be labour-intensive as this requires collecting and
preparing vast amounts of data, and the training and deployment of new models. Some of these limitations in ML systems can be overcome with the addition of automation of some or all of the steps involved in a model development cycle. Figure 2 provides an example of transaction analysis architecture used at Wise.
Models (primarily supervised learning) are developed for specific fraud typologies and product offerings; model scores are used in combination with transaction segments (a sequence of payment characteristics) as well as static rules to provide an effective and scalable transaction monitoring system.
While the above described system is typical in the industry, at Wise we are moving towards the development of a multi-stage, comprehensive fraud protection system that allows for the implementation of preventative and detection methods. The system enables adding controls at different steps in a chain of attack in a way that different fraud schemes can be neutralised. The system makes use of rules, machine learning and a combination of both, thus combining the advantages and strengths of the state-of-the-art technologies currently employed across the industry.
Most often transactions are the last step in the chain, and this is where transaction analysis typically takes place. The team at Wise has worked towards enabling preventative and detective controls at various
pre-transactional stages. Adding targeted frictions and ensuring protection at each stage of the chain ensures that bad actors are not successful at scaling their attacks, which in turn ensures that the overall return on investment of attacks is unprofitable for the perpetrators, leading to an overall reduction of fraud in the system. Wise’s system assesses customer’s risk at several stages (see Figure 3 below) which include (but are not limited to) transaction monitoring, the signup process, verification (Know Your Customer, KYC) and behaviour analysis at several interactions with our platform during login sessions. At each stage, based on the customer’s actions, we can obtain an estimate of the associated risk (this can increase or decrease based on the customer actions and data points considered) and, based on these assessments, enact certain controls such as providing or limiting access to certain product features and services.
The comprehensive pre-transaction monitoring system at Wise consists of several models at different stages of the customer lifecycle. These include the onboarding model, computer vision models for KYC processes, device identification and session analysis.
The onboarding model serves as the first stage in preventing fraud from infiltrating the platform. As already noted, it’s more important to prevent fraud from entering the platform than to fight it later. However, it’s also important to remember that at the onboarding stage, we don’t have much information about the customer. Among the information that’s used at this stage are device information and network connection data. Although this allows for detection of only the simplest fraud patterns, this model serves as a good initial filter. Such capabilities increase the cost of conducting attacks, as it requires additional expenses for bad actors to complicate and automate their processes.
After the initial onboarding, verification models (KYC) come into play. For the most part, the solution is built around the analysis and verification of uploaded documents. The task is inherently complex due to the global nature of operations. For example, Wise allows customers to send money to more than 50 countries across the world, serving over 10 million customers. Each country can have its own unique document types and languages, making the processing and understanding of these documents a significant challenge. Documents first need to be verified, then data extracted and checked. Additionally, models for document processing can be specialised to identify fake documents generated through artificial intelligence, a growing risk and concern in the payment industry.
The primary purpose of a device identity model is to authenticate devices in order to address various applied tasks, for example fraud prevention or detection. It’s important to distinguish between device identification and device fingerprinting. Typically, identification involves comparing an ID of a device with the previously seen ones, as well as verifying the application for originality. On the other hand a device fingerprint is created by considering various device parameters such as hardware identifiers, the operating system version, the browser version installed on the device, and other system and hardware characteristics. The main challenge in creating a digital fingerprint is to strike a balance between the uniqueness of the fingerprint and the frequency of changes in the parameters used to create it. Device fingerprints are used to monitor user behaviour to detect anomalies, for example in scenarios of unauthorised access to customer accounts (i.e. account takeovers). Fingerprinting is a fundamental method of fraud detection that allows users to be linked by using the same devices.
Session analysis, the process of analysing a series of customer application interactions, provides the ability
to detect malicious activity before the actual execution of fraud, such as accessing compromised user accounts or transferring proceeds of fraud to other accounts (also known as money muling). This involves detecting suspicious sessions based on a defined sequence of events, identifying typical bad actors’ patterns and behaviour, and recognising signs of device emulators, malware, or remote access tools. It also involves building an individual behavioural user profile. Typical scenarios involving anomalous behaviour identifiable through session analysis are the transfer of account ownership from one customer to another, and account compromise. The first scenario is often organised with one team of bad actors specialising in creating verified fake accounts, while another focuses on executing the fraud schemes. At the time of change of ownership, the account often undergoes ‘configuration’ changes (such as changing the password, phone number, email, etc.) and execution of a test payment (for testing purposes). In the second scenario when a customer account is compromised, the unauthorised access to the account is usually achieved through a new device followed by a change in account security settings to restrict access for the genuine owner. Session analysis helps in identifying anomalous and sudden changes in accounts data and sequence of events, thereby allowing appropriate controls and safeguards to be triggered.
As Wise operates a diverse range of markets and offers a variety of services to its customers, it necessitates a fraud protection system that is both versatile and marketspecific. This system must efficiently incorporate new solutions, which can range from machine learning models to rules-based approaches or a combination thereof, through streamlined and automated processes. Moreover, it is crucial for the system to scale effectively, managing and supporting numerous models and rules concurrently while maintaining specific service-level agreements.
To achieve these goals, the team at Wise has invested considerable resources into the development of sophisticated internal tools and automation of processes to enhance operational efficiency. This includes the development of tools designed to streamline the collection of transactional data from fraud investigations, which are crucial for the ongoing development and refinement of machine learning models. Additionally, the team has created advanced tools to facilitate the intricate and demanding task of feature engineering. This process involves the collection of new data points and generation of aggregations on streaming data with the objective to enhance the overall prevention and detection intelligence. Furthermore, the team has implemented scalable data pipelines to automate the collection and update of large datasets essential for model training, ensuring that the
data remains current at all times. Automation extends to the periodic retraining of models, guaranteeing that the latest versions are always prepared for deployment in a production environment, thereby enhancing the efficiency and reliability of model deployment and evaluation processes.
Furthermore, Wise places a strong emphasis on the governance and assessment of the models and rules, recognising the importance of these elements in maintaining a complex system’s integrity. To this end, every model’s retraining is logged, capturing not only the standard machine learning metrics, but also the specific datasets employed in the model’s creation. This approach ensures the reproducibility of models at any future point. Continuous monitoring of the models and rules once deployed in production is critical to aligning actual performance with the expectations set during testing phases and to promptly identify any deviations in the performance. Additionally, the system includes mechanisms for the ongoing surveillance of the quality of features and data points that underpin the models. This includes monitoring within data gathering pipelines to detect any shifts in data distribution or potential anomalies, thereby safeguarding the integrity and effectiveness of the machine learning models in a dynamic and evolving environment.
Fraud detection and prevention is a dynamic and evolving field. Traditional methods of fraud detection, such as transactional analysis with rule-based system and machine learning models, remain essential but are now being extended. By focusing on prevention by pretransactional monitoring through onboarding models, device identification, and session analysis, the chain of fraudulent activities can be disrupted before they reach the transactions stage. Furthermore, the creation of such systems needs to be supported by internal tooling and automation to make processes streamlined, reduce manual workload, and enhance the efficiency and accuracy of fraud detection systems. A comprehensive fraud protection system combining robust traditional methods with innovative strategies and automation, such as the one developed at Wise, is critical for mitigating risks and maintaining trust in the increasingly digital financial landscape.
We extend our deepest gratitude to the entire Wise team – data scientists, analysts, engineers, agents and managers – whose dedication and experience enable Wise to provide a safe and reliable service to its customers.
COLIN HARMAN is an Enterprise AI-focused engineer, leader, and writer.
He has been implementing LLM-based software solutions for large enterprises for over two years and serves as the Head of Technology at Nesh. Over this time, he’s come to understand the unique challenges and risks posed by the interaction of Generative AI and the enterprise environment and has come up with recipes to overcome them consistently.
It’s 2024 and every enterprise is talking about using generative AI. Even last year, 50% of companies said they were piloting generative AI projects [1] , and that’s a technology that few even knew about a year before. Rest assured that, at the conclusion of 2024, that figure will be approaching 100%. Very impressive!
There’s a firehose of content discussing potential areas of value and how to succeed with generative AI. Would you believe that innovative companies do better at deploying generative AI than… non-innovative companies [2]? Shocking! And that generative AI will transform some industries [3]? Bring it on!
But if you’re a curious person, you probably have a couple of questions that haven’t been answered by the consultants. First, what are companies actually deploying when they pilot generative AI? And second, what causes these projects to fail, so that mine can succeed?
Let’s start by illuminating exactly what these projects consist of,
beyond simply ‘generative AI.’
As a generative AI & software provider, I’ve witnessed firsthand what enterprises want, buy, and commission. First, we’ll restrict our focus to primarily text-based generative AI models – large language models (LLMs). There are certainly examples of other modalities of models being used (video, audio, mixed), but with 50%+ of companies piloting ‘generative AI’ this year, you should read that as being text-based. It’s simply the most accessible and broadly applicable form of data. Within text-based use cases there are 5 major ways to implement and benefit from generative AI [4], that range from exceedingly simple (giving employees access to ChatGPT) to extremely complex (experimental AI software developers). What the vast majority of enterprises are doing is splitting it right down the middle and implementing NLIs (natural language interfaces) , which provide a text interface to a corpus of company data through a technique called RAG (retrievalaugmented generation) . This is equivalent to using ChatGPT with browsing enabled, where
it can search the web before responding to your input. However, enterprises want to enable that same functionality on their own internal data, like document stores or databases. (RAG allows that data to be ingested into a search engine, and the results to be interpreted by a LLM).
There are several reasons that companies choose to implement natural language interfaces instead of the other use case types:
1. They can easily be introduced as a separate tool or minor augmentation on an existing one, limiting the risk of disrupting a business process by inserting an unproven technology.
2. In theory, they give enterprises easy access to massive stores of knowledge that they have heretofore been ignoring, or spending unnecessary time sorting through.
3. The benefits of 2. are extremely generic and can apply to any business area, irrespective of its function.
In summary, NLIs are chosen because they are very generic and low-risk. So what could possibly go wrong?
Ideally, a generative AI project involves implementation and evaluation, which culminates in the project being chosen to scale up for long-term adoption and value. But many don’t, even when the technical implementation is executed to perfection! Here are what I’ve observed, across many projects, to be the biggest non-technical risk factors. You’ll note that some of the buzzier topics in current discourse are missing, like cybersecurity, intellectual property, bias, etc. [5] This is because, in practice, these obstacles are either easily overcome or quite rare. Instead, the factors that follow are relevant to most projects you might encounter.
The clearest problem with tackling a generic, low-risk use case is that it may not be very important. While it’s tempting to target ‘safe’ areas, these often don’t align with strategic business goals or have significant impact, leading to projects that fade into obscurity without delivering meaningful value. Successful generative AI projects target use cases that are core to the business. By choosing a highvalue area, even if it’s higher risk, the project receives more attention, support, and resources, increasing the chances of business impact.
Another way this failure risk manifests is with leaders proposing a one-size-fits-all GenAI solution. The technologies involved (LLMs, RAG) can be used in myriad ways and if a single solution is expected to serve the needs of a large, diverse organisation, it’s unlikely to do it well. Rather than thinking of generative AI as something that a company does once, it should be considered a general technology to be leveraged in many different ways. Think of these technologies like databases – they will eventually permeate nearly every system we use and it’s silly to limit usage of
them to a single implementation. Solutions that are tailored to the needs of a small group of users with similar objectives will always provide more value per user than those tailored to the needs of a large group of users with disparate goals.
Because these natural language interfaces operate over some corpus of data, their value is closely tied to the usefulness of that corpus. Many leaders see GenAI as an opportunity to mine the mountains of data that they have accumulated, but look past the fact that previous enterprise search projects (the precursor to NLIs) may have failed spectacularly due to messy, incomplete, incorrect, bad data. Or maybe, nobody was crazy enough to even try to marshal that data before, knowing how disorganised it was! But here we are in 2024, throwing terabytes of files into a search engine and then asking a poor LLM to make sense of the results. A rule of thumb: If your data wouldn’t be useful in a search engine, it won’t be useful in an NLI, and therefore it won’t be useful for GenAI! Here are some data readiness red flags, each of which contributes to an increased risk of project failure:
● Multiple versions: In organisations, the truth changes over time. Employees spend some of their time keeping track of
what the current truth is, and documenting it. But often, what’s given to the GenAI system is a collection of all of the different versions of truth. Instead, the solution should be given the same courtesy as a new employee, that is: pointed to only the latest version of truth.
● Large volume: Since NLIs are usually built upon search engines, they are subject to the same limitations. One such limitation of search engines is that, as the amount of data grows and grows, the usefulness of the top responses decreases. This relationship is nearly as inevitable as entropy, and all you can do is be aware that as the corpus grows, more and more search optimisation may be required to maintain the same level of performance.
● Complex formatting : There’s also an additional limitation that NLI systems have beyond search engines: Since an LLM needs to read the search results, to interpret them and generate a response, the text in those search results needs to be extracted in a semantically coherent way that preserves the meaning (while many search engines are happy with a jumble of words). This can get very difficult when documents contain tricky
formatting in the shape of tables, columnar layouts, image-only text, and more.
● ‘Incorrect’ data: Data is oil, right? The more data, the better? It turns out, that thinking is very dangerous when it comes to GenAI. Since the output of an NLI is completely derived from its data sources, an incorrect data source means an incorrect output. Often, companies don’t know how much incorrect data they have until they start generating incorrect outputs left and right, only to discover that it’s coming from their own documents!
● One big data dump: Often a company will identify a massive share drive of data and say, ‘Let’s use that for GenAI!’ without caring much about what’s in it. Without fail, it will contain a healthy dose of the issues listed above.
Project Framing
In order for a GenAI project to be successful, the solution it provides should delight its users, and definitely not disappoint them. Unfortunately, it’s extremely easy to disappoint users by making promises that the solution cannot satisfy. These claims usually arise from a misunderstanding of how NLI systems work, and lead to users thinking that the solution should be able to perform complex workflows beyond the solution’s capabilities,
simply because they are able to command it and haven’t been instructed on its limitations.
The key point is this: these popular GenAI systems are search engines with a language model on top. If the task cannot be performed by executing a search and then interpreting the search results, it is probably not possible for a basic NLI system to complete it. This can result in some shocking limitations, like the inability to perform complete rankings (which is the top X) and aggregations (how many of Y) unless the answer is explicitly mentioned in the corpus, respond to a command that would require multiple steps to perform, and summarise the entirety of a large document. Some advanced solutions and those tailor-made to specific use cases can overcome such limitations, but projects can still succeed in the presence of them – they just need to inform users of what’s
[1] Boston Consulting Group, 2024 ‘What GenAI’s Top Performers Do Differently’ bcg.com/publications/2024/what-gen-ais-top-performers-do-differently
possible. But without this guidance, users will make demands of your solution that would baffle even a human expert.
However, many of those projects will not lead to immediate value, scaling, and adoption, due to poor choice of use case, messy data that’s not ready for prime time, and lax project framing that allows user expectations to expand beyond solution capabilities. I’ve seen these factors arise in nearly every GenAI project I’ve been involved with. However, with a proactive approach that emphasises strategic use case selection, meticulous data preparation, and realistic project framing, companies can not only avoid these pitfalls but also unlock transformative value from their generative AI initiatives. Now that you know these critical factors, you’re better equipped to guide your projects to success.
[2] McKinsey & Company, 2023 ‘Companies with innovative cultures have a big edge with generative AI’ mckinsey.com/capabilities/strategy-and-corporate-finance/our-insights/companies-with-innovative-cultures-have-a-bigedge-with-generative-ai
[3] Bain & Company, 2024 ‘Generative AI will Transform Healthcare’ bain.com/insights/generative-ai-global-healthcare-private-equity-report-2024/
[4] Colin Harman, 2023 ‘The 5 Use Cases for Enterprise LLMs’ colinharman.substack.com/p/the-5-use-cases-for-enterprise-llms
[5] Forbes, 2024 ‘Revealing The Dark Side: The Top 6 Problems With ChatGPT And Generative AI In 2024’ forbes.com/sites/glenngow/2024/01/28/revealing-the-dark-side-the-top-6-problems-with-chatgpt-and-generative-ai-in2024/?sh=68f38b04349adoi.org/10.1371/journal.pone.0193088
PHILIPP DIESINGER
CPHILIPP DIESINGER
is a data and AI enthusiast, having built a career dedicated to serving clients in the life science sector across diverse roles. He is driven by his passion for leveraging data-driven decision-making to deliver tangible results with real-world impact.
STEFAN STEFANOV
is a senior software engineer who brings seven years of experience in developing software solutions in the domain of life science. His passion lies in perfecting UI/UX design and transforming intricate data into user-friendly, insightful visualisations.
linical trials are research studies conducted to evaluate the safety, efficacy, and potential side effects of medical treatments, drugs, devices, or other interventions. Such studies are crucial for advancing medical knowledge, developing new therapies, and improving patient care. Clinical trials typically follow a structured protocol that outlines the objectives, methodology, participant eligibility criteria, treatment regimens, and data collection procedures.
Regulatory environments play a crucial role in overseeing and governing clinical trials to ensure
participant safety, data integrity, and ethical conduct. Regulatory bodies such as the Food and Drug Administration (FDA) in the United States, the European Medicines Agency (EMA) in Europe, and similar agencies in other countries, set forth relevant guidelines, regulations, and approval processes. These regulations cover various aspects, including trial design, participant recruitment, informed consent, data collection and reporting, and adherence to good clinical practice standards.
Trial ‘sponsors’ are individuals, organisations, companies, or institutions that take primary responsibility for initiating,
GABRIELL FRITSCHE-MÁTÉ works as a data and technology expert in the pharma industry, solving problems across the pharma value chain. He has a PhD in Theoretical Physics and a background in physics, computer science and mathematics.
ANDREAS THOMIK is a senior data scientist driven by a passion for leveraging data and AI to generate business value, and has done so across multiple industries for close to a decade, with a particular focus on the life sciences field.
managing, and funding the clinical trial. The sponsor plays a central role in all phases of the trial, from protocol development to study completion and reporting. It is a sponsor’s responsibility to ensure the registration of every clinical trial and report its results with accuracy and comprehensiveness in relevant clinical trial registries such as the US NIH platform ClinicalTrials.gov [1]
Trial registries play a crucial role in the process of clinical trials. They promote transparency by providing a centralised platform for researchers and sponsors to publicly register details about their clinical trials even before they begin.
This includes information such as the study objectives, methodology, participant eligibility criteria, interventions, outcomes, and contact information. Trial registries help prevent publication bias, which occurs when studies with positive results are more likely to be published than those with negative or inconclusive results.
Trial registries serve as valuable repositories of information about ongoing, planned and completed clinical trials. Researchers, healthcare professionals, patients, and the general public can access trial registries to identify relevant studies, learn about study design and objectives, assess eligibility criteria for participation, and obtain contact information for trial investigators or sponsors. This helps to facilitate collaboration, improve awareness of available research opportunities, and support informed decision-making by stakeholders.
Trial registries help prevent unnecessary duplication of research efforts by enabling researchers to identify existing trials on similar topics or populations. This is especially important amidst everincreasing regulations as well as economic considerations that
influence clinical research [2]
Like many other big data sets, clinical trial registry data suffers from accessibility, quality and completeness issues. Many clinical trials are conducted across multiple countries resulting in fragmentation of relevant information across multiple separate registry databases. This fragmentation poses several challenges. With clinical trial data spread across registries, researchers, healthcare professionals, policymakers, and patients may face difficulties conducting comprehensive searches or analyses. Fragmentation can result in an incomplete picture of the overall research landscape within a particular disease area, intervention type, or population group.
The World Health Organisation (WHO) has identified 17 public registries as so-called Primary Registries. For these registries, the WHO has established a set of minimal standards [3] which these registries are required to adhere to.
In addition, the WHO mandates a minimum amount of information that individual registries need to collect to consider a clinical study as ‘registered’, known as the Trial Registration Data Set (TRDS). But
whereas the TRDS qualifies the ‘what’, it does not qualify the ‘how’, leaving substantial room for interpretation to the registries, along with the possibility to individually set requirements for additional data. Some of these differences can be small, e.g. using Roman vs Arabic numerals for phase numbers.
Other differences, however, can be substantial. For instance, differing data models increase the difficulty of comparing trial information across registries. One registry might have individual ‘rows’ for study endpoints, timepoints and metrics, whereas another might simply have a large text field containing all the information at once. Registries might not be internally consistent, e.g. allowing different spellings for the same condition.
Addressing fragmentation of clinical trial data requires efforts to promote data standardisation, interoperability, and collaboration among registries and stakeholders. Initiatives such as the World Health Organisation’s International Clinical Trials Registry Platform [4] aim to improve the accessibility and quality of clinical trial data
A wealth of data: Clinical trial data submissions by year (all registries combined)
by facilitating the harmonisation of registry standards, promoting data sharing, and enhancing transparency in clinical research. Other initiatives have outlined the advantages of combining clinical trial data into a single platform [5, 6] A centralised repository for clinical trial data may help to identify opportunities for collaboration, share data across studies, or conduct meta-analyses to synthesise evidence from multiple trials, increasing the potential for scientific advancement and innovation in healthcare.
Harmonising registry data poses a significant challenge [7]. Simply using the ‘union’ of all registries’ data models as the basis for a unified data model would not only be prohibitively complex to understand but also extremely inefficient. Instead, it would be important to build it around an understanding of how data fields in individual registries map to each other, what fields are commonly reported, and which ones are so uncommon or specific that they are of limited relevance to the average user.
Beyond that, harmonisation of
terminology is required. Medical condition terminologies are currently inconsistent across registries. For instance, the NIH’s ClinicalTrials.gov uses Medical Subject Headings (MeSH) terms, while the EU Clinical Trial Registry recommends Medical Dictionary for Regulatory Activities (MeDRA). Other registries may not name or enforce a standard. Sponsor names may appear in various forms, pharmaceutical companies may be referred to under different variations of their legal entity names and names of clinical sites appear to be misspelled frequently. In addition, some information might be missing entirely.
Emerging technologies offer promising solutions to address the challenges associated with clinical trial data, unlocking its full potential. A significant portion of clinical trial data exists in unstructured formats, posing barriers to efficient analysis and utilisation. However, recent breakthroughs in GenAI and natural language processing present opportunities to effectively harness this wealth of unstructured
data. Furthermore, rapid progress in vector database storage capabilities has enabled scalable embedding, storage, and retrieval of unstructured data, paving the way for more comprehensive and insightful analyses on a large scale.
Conducting clinical trials is a resource-intensive process. Balancing cost considerations with the need to maintain rigorous scientific standards and ethical practices is a significant challenge in the design and execution of clinical trials.
Utilisation of new technologies and methods can help alleviate cost pressure. Digitisation of clinical trials offers opportunities to enhance trial efficiency, data quality, participant engagement, and regulatory compliance while driving innovation and accelerating the development of new therapies and treatments in healthcare. Digitisation of clinical trials utilises the integration of digital technologies and tools into various aspects of the clinical trial process to enhance efficiency, data collection, analysis, and patient engagement. This transformation
involves leveraging digital platforms, software applications, electronic devices, and data analytics to streamline trial operations, improve data quality, and accelerate the development and evaluation of new medical interventions. Key components of digitisation in clinical trials include: electronic data capture (EDC), remote patient monitoring (RPM), telemedicine and virtual visits, data analytics and artificial intelligence, and electronic informed consent (eConsent).
Advancements in data technologies and the digitisation of clinical studies are
revolutionising the utilisation of vast datasets, analytics, and insights to enhance decisionmaking processes. Within this landscape, clinical trial registry data emerges as a pivotal resource. It serves multiple crucial functions, including optimising site selection to ensure robust and timely participant recruitment and identifying potential risks such as competitors aiming to recruit patients from the same sites. Moreover, clinical trial registry data facilitates the evaluation of a trial’s success rate by comparing it to similar studies conducted in the past. Given that trial data
is continuously updated, it can also be leveraged to monitor ongoing trials for potential risks. Furthermore, insights gleaned from trial data shed light on the activities and portfolios of potential competitors, aiding in strategic decisionmaking processes.
Despite strong opportunities, trial registry data remains underutilised by the industry. However, the emergence of new technologies presents exciting opportunities to overcome the challenges associated with harnessing the full potential of global clinical trial registry data.
Trial data can be used to derive insights and establish benchmarks and KPIs across clinical studies
Diabetes studies are typically shorter than oncology studies. Diabetes studies: median 645 days, mean 787 days, sample size 1432 studies. Cancer/neoplasm studies: median 1644 days, mean 1817 days, sample size 3048 studies. (Of each distribution a random sample of 1200 studies was visualised below)
[1] Tse T, Williams RJ, Zarin DA. Reporting “basic results” in ClinicalTrials.gov. Chest. 2009 Jul;136(1):295303. doi: 10.1378/chest.08-3022. PMID: 19584212; PMCID: PMC2821287.
[2] Elisabeth Mahase, Clinical trials: Number started in UK fell by 41% in four years, finds report BMJ 2022; 379 doi: doi.org/10.1136/bmj.o2540
[3] World Health Organisation (2018) International Standards for Clinical Trial Registries who.int/publications/i/item/international-standardsfor-clinical-trial-registers
[4] International Clinical Trials Registry Platform (ICTRP who.int/clinical-trials-registry-platform
[5] Venugopal N, Saberwal G (2021) A comparative analysis of important public clinical trial registries, and a proposal for an interim ideal one. PLoS ONE 16(5): e0251191. doi.org/10.1371/journal.pone.0251191
[6] Goldacre B, Gray J. OpenTrials: towards a collaborative open database of all available information on all clinical trials. Trials. 2016 Apr 8;17:164. doi: 10.1186/s13063-016-1290-8. PMID: 27056367; PMCID: PMC4825083. pubmed.ncbi.nlm.nih.gov/27056367/
[7] Fleminger J, Goldacre B (2018) Prevalence of clinical trial status discrepancies: A cross-sectional study of 10,492 trials registered on both ClinicalTrials.gov and the European Union Clinical Trials Register. PLoS ONE 13(3): e0193088. doi.org/10.1371/journal.pone.0193088
SAHAJ VAIDYA is a research collaborator at AIAAIC, where she contributes to enhancing transparency and openness in AI, algorithms, and automation. She’s also part of the IEEE P7003 group, which is currently drafting a proposal for design-centred humanrobot interaction (HRI) and governance, with a focus on algorithmic bias considerations.
At the World Ethical Data Forum, Sahaj is developing a taxonomy for AI risks and challenges. She’s a doctoral student of Data Science at the New Jersey Institute of Technology (NJIT), and has dedicated her research to ethical AI governance, data-driven decisionmaking, and the effective communication of scientific insights, which are pivotal aspects of responsible AI implementation in the public sector. Her research project, the Open Explainability Protocol (OEXP) aims to establish a universally accepted standard for conveying the outputs of autonomous systems.
Artificial intelligence (AI) is rapidly weaving itself into the fabric of our lives, from the algorithms that curate our social media feeds to the self-driving cars transforming our transportation landscape. As AI’s influence expands, so too does the urgency to ensure its development and deployment are guided by ethical principles. AI constitutionalism emerges as a critical framework in this endeavour, emphasising the process, values, and societal impact of AI, not just the technological feats it achieves.
BEYOND EFFICIENCY: THE ETHICAL IMPERATIVE OF AI CONSTITUTIONALISM
Traditionally, discussions surrounding AI tend to focus on the end results: accuracy, efficiency, and innovation. While these are undeniably important goals, AI constitutionalism argues that a singular focus on outcomes overshadows a crucial aspect – the means by which AI systems arrive at these results.
As AI’s influence expands, so too does the urgency to ensure its development and deployment are guided by ethical principles.
This framework emphasises the need for a comprehensive ethical lens throughout the entire lifecycle of AI, encompassing data collection, model training, deployment, and ultimately, its impact on society.
Imagine an AI-powered criminal justice system touted for its predictive capabilities in identifying potential recidivists. While a high accuracy rate might seem impressive, AI constitutionalism would prompt a deeper examination. How is the data used to train this system collected? Does it inadvertently perpetuate historical biases present in the criminal justice system, leading to the unfair targeting of certain demographics? Does this technology erode trust in law enforcement and the justice system as a whole? By analysing the ethical means employed by AI systems, we can identify and mitigate potential harms before they manifest.
AI systems don’t operate in isolation. They interact with a complex web of people, institutions, and ecosystems. AI constitutionalism emphasises the importance of recognising these interconnected relationships. The objective becomes developing ‘relational AI’ technologies that strengthen human connections, foster trust, and promote collaboration across all levels of society.
Consider AI-powered language learning platforms that facilitate intercultural communication and understanding. Imagine AI tools that connect students from diverse backgrounds, fostering collaboration on global projects and fostering a sense of global citizenship. By focusing on relational AI, we can
SAHAJ VAIDYAleverage technology to bridge societal divides and build a more inclusive future.
FOR PROGRESS
AI should serve as a force for positive change, contributing to the well-being of all communities. AI constitutionalism advocates for the prioritisation of AI applications that address pressing societal challenges, such as climate change, poverty, and access to education. Furthermore, it emphasises the equitable distribution of benefits, ensuring that all communities can leverage AI advancements to improve their lives.
ACTION: AI FOR SUSTAINABILITY AND ENVIRONMENTAL STEWARDSHIP
Imagine AI-powered systems that optimise renewable energy production, monitor deforestation patterns, and predict environmental hazards. Consider AI tools that empower local communities to develop sustainable agricultural practices and mitigate the effects of climate change. By focusing on community well-being, AI becomes a catalyst for environmental stewardship and a more sustainable future.
PARTICIPATORY DECISIONMAKING: A COLLECTIVE VISION FOR THE FUTURE
Inclusive governance is the cornerstone of AI constitutionalism. Shaping the future of AI requires the collective wisdom and diverse perspectives of a broad range of stakeholders. This includes academics, policymakers, civil society organisations, industry leaders, and most importantly, the communities directly affected by AI.
APPROACH: MULTISTAKEHOLDER FORUMS FOR INCLUSIVE AI DEVELOPMENT
Envision multi-stakeholder forums where decisions concerning AI
are made collaboratively. These forums should actively seek the participation of diverse voices, including those from historically marginalised communities. Through inclusive dialogue and collective deliberation, we can develop AI policies and regulations that are truly representative and reflect a shared vision for an ethical and equitable future with AI.
Let’s delve deeper into the example of using AI for healthcare access in underserved communities. AI constitutionalism wouldn’t simply focus on improving appointment scheduling or diagnosis accuracy. It would consider the following aspects:
● Means: How does the AI collect health data while respecting patient privacy? Does it offer culturally sensitive medical recommendations, taking into account local practices and beliefs? Does it ensure that data is anonymised and securely stored, protecting patient confidentiality?
● Relationality: Does the system strengthen communication between healthcare providers, community health workers, and patients? Does it facilitate trust-building and personalised care, considering the specific needs of each community? Does the AI empower local healthcare professionals by providing them with decision-making support tools? Does it promote preventative care initiatives tailored to the specific health challenges of the community? For instance, the AI system could analyse local health data to identify patterns of chronic diseases and recommend targeted preventative measures. Additionally, it could
be used to develop culturally appropriate educational materials to raise awareness about these health issues.
MEASURABLE IMPACT: QUANTIFYING THE BENEFITS OF ETHICAL AI
AI constitutionalism compels us to move beyond mere technological feats and assess the tangible impact of AI on communities. Here are some potential metrics to evaluate the success of the AI-powered healthcare system in our case study:
● Reduction in preventable disease rates: Track the incidence of diseases that can be mitigated through preventative measures, such as diabetes or heart disease.
SAHAJ VAIDYA● Increased access to healthcare services: Monitor the number of individuals in the community who are now receiving regular healthcare checkups and screenings.
● Improved patient outcomes: Analyse data on patient health outcomes, such as mortality rates or length of hospital stays, to assess the overall effectiveness of the AI-powered healthcare system.
● Community satisfaction: Conduct surveys and focus groups to gather feedback from community members on their experience with the AI healthcare system. This helps identify areas for improvement and ensure the system is truly meeting the needs of the population.
AI constitutionalism offers a roadmap for navigating the complexities of AI development and deployment. By prioritising ethical means, fostering relationality, ensuring community wellbeing, and embracing participatory decision-making, we can harness the power of AI for good.
By adopting these principles and metrics, we can ensure that AI is not just a technological marvel, but a powerful tool for advancing healthcare equity and improving the well-being of all communities.
CONCLUSION: A CALL TO ACTION
AI constitutionalism offers a roadmap for navigating the complexities of AI development and deployment. By prioritising ethical means, fostering relationality, ensuring community well-being, and embracing participatory decision-making, we can harness the power of AI for good. Let us embark on this journey together, shaping a future where AI serves as a force for positive change, benefiting all of humanity.
To hire the best person available for your job you need to search the entire candidate pool – not just rely on the 20% who are responding to job adverts.
Data Science Talent recruits the top 5% of Data, AI & Cloud professionals.
Fast, effective recruiting – our 80:20 hiring system delivers contractors within 3 days & permanent hires within 21 days.
Pre-assessed candidates – technical profiling via our proprietary DST Profiler.
Access to talent you won’t find elsewhere – employer branding via our magazine and superior digital marketing campaigns.
Then why not have a hiring improvement consultation with one of our senior experts? Our expert will review your hiring process to identify issues preventing you hiring the best people.
Every consultation takes just 30 minutes. There’s no pressure, no sales pitch and zero obligation.
AWARD-WINNING AI & IOT SOLUTIONS COMPANY EMRYS PROVIDE FIRST-HAND EXPERIENCE OF INDUSTRY SENTIMENT AT DAVOS AND THEIR POINT OF VIEW ON WHAT COMES NEXT.
Founder and CIO of Emrys Consulting, part of Emrys Group.
Having an outstanding track record of leadership and mentorship, ZANA ASTON EMBA is a vocal champion of the use of technology to create new opportunities for women. She contributes to the community supporting women in tech as a Country Director and Women on Boards, WIT Chair.
Zana takes to the international stage at events related to digital transformation, AI, cyber security, sustainability, and female leadership, as a speaker and moderator.
A strong networker, Zana sits on the advisory board for the Finance and Fintech Group within the Institute of Directors, London and is a member of the prestigious City Livery Club.
Recently, we attended the annual meeting of the World Economic Forum in Davos.
We approached Davos 2024 with high expectations; if there ever was an AI winter, there is no denying that AI has recently made a mighty comeback. It has now been a few weeks since the 2024 World Economic Forum annual meeting closed its doors, and a good time for a level-headed second reading of broad consensus-reached outcomes. It has, after all, been the year of ‘rebuilding trust’, with more technology-focused objectives supporting this theme.
Since joining the firm, GEORGIOS SAKELLARIOU has been shaping our approach to helping clients demystify and adopt AI, whilst having a keen eye for the fast-paced world of technology innovation.
Georgios sits on startup advisory boards, is often invited as a speaker at events, and champions initiatives within the broader AI community.
Georgios holds a PhD in Artificial Intelligence from Imperial College London, and has held senior positions at distinguished consulting firms.
As the snow settles following the gathering of thousands of entrepreneurs, business leaders and government officials in the otherwise quiet and picturesque mountain resort, one cannot but wonder what practical steps could be taken to realise this year’s ambition of rebuilding trust at a global scale.
Beyond calls for global convergence in the context of conflict avoidance, will AI maintain its current position and take centre stage next year as well, and will trust in AI itself emerge more explicitly as the theme of next year’s summit?
This year, some leaders have been eager to emphasise the practical applications of AI. More interestingly, nuanced conversations have moved further, debating its link to the energy transition; such conversations are particularly intriguing, as not only are they a recognition of the priority nature of both topics, but also a realisation of the opportunities for innovation that emerge as ideas are approached from different angles.
Some business leaders and government officials readily identified several opportunities for AI in both business and government, with an explicit focus on shifting the dialogue towards what can be achieved in the present, and in the anticipation of AGI maturity. It could then be argued that this could be the year of such implicit links becoming explicit, though such connections are not limited to the sustainability agenda. Healthcare and financial services are two more examples of the prevalent use of AI. The novelty of direct user communication with generative AI systems in particular has fuelled recent activity in these industries.
How do this year’s takeaways compare with the state of the nation a year ago, at the end of the 2023 summit, and the perceived need for a reinvigoration of the ESG agenda? With now sufficient distance from both events and the continued manifestation of the collateral consequences of unsustainable economies, it may be the right time for leaders to concentrate on the topic of sustainable AI.
It is clear that AI has been top of mind this year, but identifying and acting on macro-trends, while strengthening the link to other business imperatives, will reinforce its potential for impact. It is our view that the growing popularity and business uptake of AI technology will act as a catalyst for resurfacing ESG sensibilities and re-establishing their business relevance. What is more, such renewed focus would be in support of the intended ‘for-good’ agenda of AI and provide new purpose to most technology optimisation and innovation initiatives.
The need to better understand the mathematics behind generative AI and the shifting balance between an ML model and the data it relies on has been a topic of discussion amongst experts. In the aftermath of Davos 2024, it is perhaps topical to further build on these ideas in business terms and better align to this year’s theme of rebuilding trust, as well as the convergence of benefits for both AI and the ESG agenda. Could this also be a case of a multi-objective optimisation? After all, is the reconciliation of sometimes conflicting priorities not at the heart of good leadership?
Existing applications in industries as diverse as healthcare and retail show in real terms the market relevance of AI today. While degrees of maturity vary, and not all AI is created equal, the ‘North Star’ paradigm of AI omnipresence continually encourages AI fluency and convergence across diverse businesses.
Both this year’s Davos summit and the collective experience we have acquired through our engagements suggest several key insights into the future trajectory of AI and its impact on businesses:
Continued Momentum: The momentum behind AI shows no signs of slowing down. As we move further away from the initial excitement surrounding generative AI, there’s an opportunity for further refinement and maturity in the field.
Altruistic AI: Discussions about altruistic AI need to mature, with a focus on explaining limitations more effectively to non-technical audiences. This will accelerate the identification of meaningful use cases and ensure that AI technologies are used responsibly.
ESG Integration: Environmental, social, and governance (ESG) considerations are becoming increasingly important, especially in relation to the energy consumption of AI technologies. Businesses need to incorporate ESG principles into their AI strategies to ensure sustainable growth.
Multimodal AI: The emergence of multimodal AI presents new opportunities for multichannel experiences. Regulation and adoption efforts should take into account this natural evolution in AI capabilities in the short to medium term.
Interdisciplinary Collaboration: Multidisciplinary approaches are essential for understanding the long-term implications of AI integration into society. It’s crucial to articulate the implications and dilemmas of AI adoption in ways that resonate with a broad audience, drawing on impactful messaging to capture societal anxieties.
In summary, staying abreast of the rapidly evolving AI market and focusing on solving real business problems will be crucial for businesses to unlock lasting positive impact. Balancing innovation investment with safe adoption practices will be a central theme in navigating the AI landscape in 2024.
Prototype, simulate, and deploy solutions for the most challenging problems in drone, ground vehicle, and satellite application.
Seamlessly integrate all components into one platform.
Shape the future of autonomous robotics with us.
Join Intrepid AI to revolutionise robotics. START TODAY!
When I initially began as a leader at a software firm, I would claim that my drive came from developing new features and seeing them implemented. But as I advanced in my role, my thinking shifted. I began to concentrate much more on the people who were developing and executing the product. I recognised that I should focus on developing their careers, and in turn, they would help my firm grow.
CLAUS VILLUMSEN has worked as a Chief Technology Officer in Copenhagen for almost 20 years. He’s the Founder of Kodecrew, a productivity application for software development teams. Claus’ prior roles include CTO at RushFiles and Director of Operations at e-conomic. Throughout his career, Claus has helped hundreds of new employees develop into amazing leaders and professionals. Claus earned a Bachelor of Engineering (specialising in Environment Engineering) from the Technical University of Denmark.
These days I take note when an employee succeeds above and beyond expectations. Those folks represent the pinnacle of my professional achievements.
In this article, I’d like to share the insights I’ve gained over the years, so you can seamlessly mix hard facts with the soft abilities required for effective team leadership. I’ll explain how to manage your team for the mutual benefit of individual members and the organisation as a whole.
Let’s get into it:
A growth mindset is a game-changer, not just a buzzword. Imagine believing you can get smarter or better at something with effort. That’s a growth mindset. It’s about embracing challenges, persisting when things get tough, learning from criticism, and finding lessons and inspiration in others’ successes.
In leadership, feedback isn’t just a tool: it’s the cornerstone of growth and development. It’s about guiding your team towards their best selves, and it’s deeply rooted in the principles of a growth mindset. Feedback, when anchored
‘Effort is one of those things that gives meaning to life. Effort means you care about something, that something is important to you and you are willing to work for it.’
Dr Carol S DweckDr Carol Dweck, a psychologist who developed the phrase after discovering this idea through her research. She showed that people with a growth mindset achieve more than those with a fixed mindset who believe their abilities are static. It’s not about telling yourself you’re the next Einstein because, let’s be honest, that’s a tall order. It’s about the belief that effort makes you stronger. And it applies to everything: learning maths, playing guitar, even improving relationships.
The growth mindset has its roots in decades of research on achievement and success. Studies show that students who were taught about growth mindsets improved their grades and motivation to learn. It tells us that our brain can grow and change through practice and persistence.
It’s not magic. It’s mindset. Embracing a growth mindset means seeing yourself as a work in progress. It’s about celebrating the journey towards improvement, not just the destination. And the best part? It’s accessible to anyone willing to put in the effort and embrace learning, one step at a time.
in real data, moves beyond the realm of subjective opinions to concrete, actionable insights. It’s not about pointing fingers but about paving a path forward together.
Giving feedback, both positive and negative, requires a delicate balance. Positive feedback should be specific and tied to real achievements or behaviours. It’s not just ‘Good job!’ but ‘Your approach to solving that problem was innovative because…’. It reinforces the growth mindset by acknowledging effort and strategy, not just innate talent.
Negative feedback, on the other hand, is where the growth mindset really shines. It’s not a verdict, but an opportunity. Start with the data to keep it objective: ‘I noticed the project timeline has been extended multiple times.’ Then, make it a two-way conversation about learning and growth: ‘Let’s explore how we can address these challenges together.’ It’s about focusing on future actions and solutions, not past mistakes.
Incorporating growth mindset principles means you believe in the potential for development and improvement. Feedback becomes a constructive dialogue, fostering resilience, encouraging risk-taking, and ultimately leading to personal and professional growth. It’s leadership that doesn’t just aim to correct but to inspire and transform.
Imagine your journey to personal fitness or how you express love to those closest to you. Now, think about applying that same principle to the way we evaluate and foster growth within our teams. Just as going to the gym four times a year won’t get you in shape and telling your loved ones you care only twice a year won’t build strong
Creating a work environment that minimises distractions and allows for deep focus is akin to providing an artist with a serene studio or a writer with a quiet retreat. It’s a clear signal from management that they understand the
relationships, infrequent evaluations don’t support continuous growth or team cohesion.
Frequent evaluations, especially when done weekly, are like having a real-time GPS for your team’s performance and well-being. This approach doesn’t just tick boxes; it provides ongoing, actionable insights that drive improvement, engagement, and alignment with goals. It’s about creating a culture where feedback is not feared but welcomed as a tool for personal and professional development.
This method saves money by optimising productivity. When team members know where they stand and what they need to improve, they can adjust their efforts in real time, ensuring that projects stay on track and resources are used efficiently. It reduces employee churn by demonstrating that you’re invested in their growth and value their contributions, making them more likely to stay and thrive within your organisation.
Moreover, it helps quickly identify non-performers – not to penalise them, but to offer targeted support, retraining, or realignment of roles based on their strengths. Just as regular gym visits contribute to better health over time and consistently expressing love strengthens bonds, the continuous cycle of evaluation and growth builds a stronger, more resilient, and more productive team.
value of concentrated effort and the profound impact it can have on both the quality and quantity of the work produced.
When employees are allowed to immerse themselves in their tasks without constant interruptions, they’re not just more likely to meet their targets – they’re able to exceed them, often with superior quality results. This uninterrupted work time is not a luxury; it’s a strategic advantage. It enables individuals to engage in deeper problem-solving, fosters creativity, and leads to innovations that can set a company apart.
Moreover, this approach demonstrates a level of respect and trust from management towards employees. It acknowledges that professionals know how to manage their workload and can be trusted to deliver without being constantly overseen or disrupted. This trust builds a stronger, more confident team, where individuals feel valued and understood.
In essence, allowing employees to focus without distractions is not just about getting more work done –it’s about getting better work done. It’s a commitment to excellence, a nod to the importance of mental well-being, and a testament to the belief that when given the right environment, employees will not only meet expectations but will soar beyond them.
Imagine having a year’s worth of conversations, achievements, and growth packed into data that truly understands your journey. That’s what one-on-one meetings offer when they’re built on a foundation of
As you embark on the journey to foster a culture where people are priorities over processes, it’s essential to remember that the heart of innovation, productivity, and retention lies within the team itself.
Through my years of experience, I’ve witnessed the transformative power of focusing on individual growth, open communication, and collective effort. This approach
trust and detailed records. These meetings are not just a check-in; they’re a deep dive into what makes your work meaningful and how you can grow even more.
Before the meeting, jot down what’s on your mind. What challenges have you faced? Where have you shined? This isn’t just a chat; it’s your moment to steer the conversation towards what matters to you in your career.
Here, we talk about your ambitions and setting goals that align with where you want to be and who you want to become. This isn’t about hitting company targets; it’s about hitting your personal milestones and expanding your mindset to welcome growth and learning at every turn.
And when you do something amazing, we shout it from the rooftops – well, maybe just on LinkedIn for now. But it’s our way of saying we see you, we appreciate you, and we’re here to support you, not just as a valuable part of our team but as the individual you are, striving for greatness.
These one-on-one meetings are our commitment to you. They’re how we ensure you feel seen, heard, and supported, not just in reaching for the company’s goals but in achieving your own personal and professional aspirations.
not only maximises productivity but also nurtures an environment where team members feel valued, understood, and motivated to contribute their best.
Embrace the notion that each person’s unique skills, perspectives, and potential are the keystones to building a resilient and dynamic team. Encourage continuous learning, celebrate small wins, and provide constructive feedback. These practices help cultivate a growth mindset, where challenges are seen as opportunities for development rather than obstacles.
Remember, the strongest teams are built on trust, respect, and mutual support. As tech leaders, our role extends beyond managing projects and meeting deadlines; it involves inspiring our teams, fostering a sense of belonging, and empowering each member to achieve their full potential.
By placing people at the core of your leadership strategy, you’ll not only see remarkable results in your team’s performance but also in their loyalty and commitment to the organisation’s vision. So, as you move forward, let the principles of empathy, empowerment, and engagement guide you towards creating a workplace where everyone thrives. Together, you’ll navigate the path to maximum productivity and retention, setting a new standard for what it means to lead with purpose and humanity.
Quickly recruit an expert who will hit the ground running to push your project forward
Don’t let your project fall behind any further. You can access the top 5% of pre-assessed contractors across the entire candidate pool - thanks to our exclusive DST Profiler® skills assessment tool. You can find your ideal candidate in 48 hours* - GUARANTEED
We recruit contractors to cover:
Skills or domain knowledge gaps Fixed-term projects and transformation programmes Maternity/paternity leave cover Sickness leave cover Unexpected leavers/resignations
DR GARETH HAGGERJOHNSON is a data professional with over 15 years of experience in data analysis, research methodology, and leadership roles spanning both public and commercial sectors. With a strong track record in generating actionable insights for internal and external stakeholders, Gareth has made significant contributions across diverse industries such as financial services, fast moving consumer goods (FMCG) and academic public health and epidemiology. He has a particular interest in methodology and in issues of representation of minority groups in statistical analysis.
AThe pervasive issue of algorithmic bias, with its documented consequences particularly affecting minority groups in areas such as housing, banking, health, and education, has spurred increased attention and scrutiny
lgorithmic bias concerns unfair decisions about real people, which explains why there is much interest in avoiding it. These concerns are not new and are not specific to AI algorithms – traditional algorithms have long been shown to produce biased predictions or classification
decisions. The pervasive issue of algorithmic bias, with its documented consequences particularly affecting minority groups in areas such as housing, banking, health, and education, has spurred increased attention and scrutiny (Chin, 2023). Recognised for its inherent unfairness, algorithmic bias manifests when automated systems generate decisions that disproportionately impact individuals based on their demographic characteristics. This
unfairness becomes apparent when a biased algorithm yields distinct scores or classification decisions for individuals who share identical input data. For instance, if an algorithm demonstrates a propensity to deny loans to ethnic minorities with equivalent credit scores, it is deemed biased. This unfairness extends beyond mere disparities and can be characterised by psychometric bias, as observed in aptitude tests where certain test items generate different scores for individuals with the same underlying abilities but belonging to different population groups, a phenomenon known as ‘differential item functioning’. The increasing awareness of these challenges has driven a growing interest in addressing and mitigating algorithmic bias to foster equitable decision-making in various domains. AI has existed for decades and is often an extension of traditional techniques connected to statistics and other disciplines with application in financial services and health (Ostmann, 2021). Recent advantages are innovative but are often incremental improvements, not seismic shifts (Ostmann, 2021).
Bias within algorithms can emerge from a multitude of factors, not limited to the algorithm’s design or unintended usage. Critical contributors include decisions surrounding how data is coded, collected, selected, and for AI algorithms, utilised in the algorithm’s training process (Chin, 2023). The data fed into algorithm design becomes a pivotal factor in shaping biases within the system. This bias may originate from pre-existing cultural, social, or institutional expectations, technical constraints inherent in the algorithm’s design, or its application in unforeseen contexts or by audiences not initially considered during the design phase. The widespread nature of algorithmic bias is evident across
various platforms, including search engines and social media. The impacts are far-reaching, extending from inadvertent privacy violations to the perpetuation of social biases linked to race, gender, sexual orientation, and ethnicity. It underscores the critical importance of addressing bias not only in the algorithmic design but also in the meticulous curation and handling of data throughout the training process. Data quality issues have consistently been shown to prevent optimal use of AI (Ostmann, 2021). Data quality has not received enough attention in relation to algorithmic bias – the algorithms themselves tend to be the focus. Data quality is necessary but not sufficient for unbiased prediction and classification decisions. Data quality encompasses the accuracy, completeness, consistency, and reliability of the data used in machine learning algorithms. While algorithmic bias has traditionally been a focal point, with efforts directed toward fine-tuning models and employing fairness-aware techniques, the significance of data quality in influencing algorithm outcomes cannot be overstated.
For instance, if a hiring algorithm is trained on historically biased data where underrepresented groups are systematically excluded, the algorithm, despite its high quality, perpetuates these biases in predictions.
Biased data acts as a bottleneck, hindering the algorithm’s capacity to deliver fair and unbiased results. Additionally, biased data may introduce or reinforce stereotypes. To comprehensively address algorithmic bias, it is essential to scrutinise and rectify biases within the training data, identifying and mitigating biases, ensuring representativeness across demographics, and incorporating fairness considerations during data collection and preprocessing. In summary, while data quality is
foundational for building robust machine learning models, its assurance alone does not ensure unbiased predictions. A holistic approach involves improving training data quality alongside algorithmic enhancements, recognising both as interdependent components in the pursuit of creating equitable and unbiased AI systems.
Utilising algorithms embedded with inherent biases and coupling them with poor-quality data creates a compounding effect, exacerbating and amplifying the existing biases entrenched within the algorithmic decision-making process. Poor data quality becomes a pivotal factor in this equation, acting as a catalyst for generating unfavourable and skewed outcomes, with its detrimental impact being particularly pronounced among minority groups. The uneven prevalence of inaccuracies or omissions in the data pertaining to these groups contributes to the disproportionate amplification of biases. For instance, in the United States, Hispanic immigrants may face challenges in obtaining accurate social security numbers, introducing inaccuracies into the data. When data having underdone record linkage algorithms are analysed, Hispanic adults appear to live longer than non-Hispanic whites which is the reverse of understood patterns of health – termed the epidemiologic paradox. This is due to data quality issues among Hispanic health records (Lariscy, 2011) and surname conventions that reduce the likelihood of data linkage. Names are more likely to be transcribed incorrectly by third parties for ethnic minorities leading to increased risk of linkage error (Bhopal, 2010). In the United Kingdom, ethnic minorities are more likely to encounter missing NHS numbers in their hospital records, further diminishing data quality
and record linkage – producing biased estimates of readmission rates (Hagger-Johnson, 2017). There is variation in data quality at the source. Variation in data quality between hospitals is comparable in size to the difference between Asian and white groups in relation to missed matches (Hagger-Johnson, 2015). It is crucial to acknowledge that the quality of data might partially reflect the quality of the interaction between minority groups and healthcare services, as difficulties in obtaining accurate identification numbers may stem from systemic issues in the healthcare system.
More fundamental than poor quality data among minority or vulnerable groups is the decision not to measure group membership at all. As put by Karvonen: ‘Having accurate data is a key first step in addressing health inequities, since what is measured influences what is done’ (Karvonen et al., 2024). Put differently, omission is oppression. The exclusion of certain groups from data can perpetuate and reinforce existing inequalities and power imbalances, rendering them invisible. The amplification of bias becomes even more pronounced when information about an individual’s minority status is either missing, incorrect, or inconsistent. In one study with colleagues from UCL, we found that the largest proportion of missed matches occurred when ethnic minority status was missing (Hagger-Johnson et al., 2017). In Canada and France, national health databases do not record ethnicity (Naza et al., 2023). Concrete data quality issues, such as incomplete or inaccurate identification data, contribute significantly to biased algorithmic decisions (Lariscy, 2011). Addressing algorithmic bias necessitates a comprehensive approach that encompasses both refining the algorithms themselves and rectifying the underlying data quality issues, particularly those
GARETH HAGGER-JOHNSONaffecting marginalised communities, to foster fairness and equity in automated decision-making processes. Even small amounts of linkage error can produce biased results (Neter et al., 1965). It is challenging to evaluate algorithmic bias, partly because of commercial sensitivities around data and algorithms, but also because data on protected characteristics (e.g. sexual orientation, religion) might not be available for analysis.
There is documented evidence that women and ethnic minorities find it more difficult to access credit, although this appears to be mostly attributable to real differences in credit risk factors than active discrimination, which has been outlawed. The Markup’s investigation into lending decisions based on ethnicity, analysing over two million conventional mortgage applications in 2019 (Martinez, 2021), suggested lenders were more likely to deny home loans to black compared to white applicants with similar financial profiles, but was criticised for not including one of the key data points – credit score. Much of the apparent disparities in lending decisions by ethnic group is accounted for by genuine differences in credit risk data –white applicants had higher credit scores (Bhutta, 2022). And there are legal safeguards against treating applicants differently based on race or ethnicity. This does not mean that other variables don’t impact ethnic minorities. For example, they may be more likely to have unpredictable and riskier incomes, live in areas with fewer branches, or have less intergenerational wealth to draw on. Nonetheless, there remain some unobservable characteristics which influence credit risk decisioning and there are manual decisions made by underwriters subsequent to an initial approval. A 2018/2019 study of nearly nine million loan applicants found
that lenders are more likely to override automated underwriting system recommendations to deny a minority applicant and to override a negative recommendation to approve a white applicant. Excess denials, while only about 1-2%, were attributed to unobservable characteristics (Bhutta, 2022). Qualitative comments collected during the study suggested potential disparities in the treatment of minority groups, affecting data quality, with references to ‘incomplete application’ or issues with ‘verification’ more likely for Asian and Hispanic groups. Branch availability in areas where certain groups reside may also contribute to these disparities.
In the rapidly evolving landscape of artificial intelligence (AI), the importance of managing data quality cannot be overstated. As Ostmann (2021) points out, it’s often relegated to the background, but it’s a cornerstone of ethical AI. Transparency and explainability hinge on understanding the quality of the data being utilised. To uphold these principles, clear standards and guidelines for assessing and maintaining data quality in AI systems are imperative. Robust data governance frameworks must be established to ensure that the data powering these systems is not only accurate and representative but also free from biases. This necessitates regular audits and evaluations of data sources to identify and rectify any discrepancies or omissions.
[Data quality ] is often relegated to the background, but it’s a cornerstone of ethical AI.
Moreover, in the context of data linkage, open dialogues between data providers and analysts are essential to comprehensively understand how data quality and linkage errors might impact outcomes (Gilbert, 2018). By prioritising data quality within AI initiatives, we pave the way for more trustworthy, accountable, and ultimately ethical AI applications.
BIBLIOGRAPHY
• Bhopal, R. et al. (2010). Cohort Profile: Scottish Health and Ethnicity Linkage Study of 4.65 million people exploring ethnic variations in Bhopal, R. et al. (2010). Cohort Profile: Scottish Health and Ethnicity Linkage Study of 4.65 million people exploring ethnic variations in disease in Scotland. International Journal of Epidemiology , 40(5), 1168–1175.
• Bhutta, N. et al. (2022). How much does racial bias affect mortgage lending? Evidence from human and algorithmic credit decisions. Washington , D.C.: Federal Reserve Board: Finance and Economics Discussion Series.
• Chin, M. et al. (2023). Guiding principles to address the impact of algorithm bias on racial and ethnic disparities in health and health care. JAMA Network Open , 6(12).
• Gilbert, R. et al. (2018). GUILD: Guidance for information about linking data sets. Journal of Public Health , 40(1): 191–198.
• Hagger-Johnson, G. et al. (2015). Identifying possible false matches in anonymised hospital administrative data without patient identifiers. Health Services Research , 50(4), 1162–1178.
• Hagger-Johnson, G. et al. (2017). Probabilistic linking to enhance deterministic algorithms and reduce linkage errors in hospital administrative data. Journal of Innovation in Health Informatics , 24(2): 891.
• Karvonen, K. & Bardach, N. (2024). Making lemonade out of lemons: an approach to combining variable race and ethnicity data from hospitals for quality and safety efforts. BMJ Quality and Safety , 33(2).
• Lariscy, J. (2011). Differential record linkage by Hispanic ethnicity and age in linked mortality studies: Implications for the epidemiologic paradox. Journal of Aging and Health , 23(8), 1263-1284.
• Martinez, E. (2021). The secret bias hidden in mortgage-approval algorithms. Retrieved from AP News: apnews.com/article/lifestyle-technology-business-race-and-ethnicitymortgages-2d3d40d5751f933a88c1e17063657586
• Neter J, et al. (1965). The effect of mismatching on the measurement of response error. Journal of the American Statistical Association , 60(312), 1005-1027.
• Ostmann, F. (2021). AI in financial services . London: The Alan Turing Institute.
DISCLAIMER:
The views expressed in this article are solely those of the author and do not necessarily reflect the opinions or views of any employer, organisation, or institution associated with the author. The author retains full responsibility for the content presented herein.
SANDRO SAITTA is currently AI Advisor at viadata, a company providing executive advising and corporate training. He has 20 years’ experience in data science and is passionate about helping companies to become even more datadriven. Sandro has worked in various industries to foster the usage of data, such as telecommunications, the chemicals industry, an online travel agency and the FMCG industry. He is a lecturer at Business School Lausanne and HEC Lausanne. Sandro is also a member of the executive committee of CDOIQ Europe, an association that supports the role of Chief Data Officer in Europe.
Your data analysis is comprehensive. Your dashboard is ready. Your machine learning model is accurate. Yet, nothing seems to be happening. The issue does not lie in the code or data but within the people dimension. The good news is that you, as a data scientist/analyst, can tackle this challenge by enhancing your soft skills. This article provides tips and tricks to enhance the impact of your data initiatives. The content is divided into five key soft skills categories: data visualisation & storytelling, communication, stakeholder management, adaptability, and business acumen.
By training, we – data scientists – are better with technical skills simply because existing academic curricula mainly focus on statistics, machine learning, and programming skills. However, improving your soft skills will definitely boost your impact within companies.
Let’s remember that all data initiatives need to start with a question. As Hilary Mason stated very well: ‘The truth is that framing the questions is where the challenge is. Finding the answers is generally a trivial exercise or
an impossible one.’ One of the key reasons soft skills are important is they enhance our ability to frame the problem before using any machine learning algorithm, or even preparing the data.
Additionally, we must keep in mind that data projects have an impact only if they are trusted and adopted by stakeholders. Being able to tell a story and convince these individuals is crucial to shift from an interesting proof of concept to a data product that benefits the company.
Part of data literacy is the ability to read and write data, visuals, and dashboards. Data visualisation skills are key to helping your audience grasp the insights from your data. The more you improve your visuals, the easier it is for your audience to understand your point. This also means that you must first know your audience. Before preparing visuals, ask yourself the following questions (see Figure 1):
● What matters to your audience?
● What questions might your audience have?
● What is the level of expertise of your audience?
You can also improve the efficiency of your plots with the following tips:
● Choose the right visualisation: Start by isolating the correct category (comparison, trend, etc.), select the most suitable chart type (scatterplot, bubble, etc.), and eventually fine-tune the options (axes, colours, etc.).
● Remove the clutter: Anything that is not strictly needed in the plot should be removed. Indeed, the more cluttered the graphs are, the harder they are to read. Adopt the motto: if in doubt, leave it out.
● Use preattentive attributes: Utilise whatever you can (colour, size, etc.) to highlight what you want your audience to focus on. The less time they need to think about your visual before getting to the point, the better.
● If you want to go one step further, consider the Gestalt principles (proximity, similarity, closure, etc.) which aid in designing dashboards, for example (see the book at the end of this article).
● A final point to keep in mind related to data storytelling is the distinction between data exploration and data explanation. While analysing data, you generate plenty of plots to gain insights; this is the exploration phase. Once complete, you move to the explanation phase, in which you tell a story by selecting and fine-tuning a tiny portion of these visuals. As proposed by Brent Dykes in his
book Effective Data Storytelling , you are like Indiana Jones, both an archaeologist (exploration) and a professor (explanation).
EFFECTIVELY BY STARTING WITH WHY
Without communication, there is no adoption, and thus no impact. The classic approach is to start with what we do, then focus on how we do it, and – if we have time – discuss the why. A much more impactful approach is to start with why. This is well explained in Simon Sinek’s book Start with Why. Focusing on the why helps your stakeholders understand and adhere to your reasoning.
In terms of communication – and if you like acronyms – I suggest the 3Cs rule: communicate, communicate, and communicate. You must constantly share information about your data initiative – via newsletters, internal chats, and even paper notes – to key people. Another way to think about it is this: if people are asking about the status of your project, then you are under-communicating.
Finally, simplification – the art of making complex concepts accessible to a broader audience – is key to communicating with your audience. Keep in mind that your audience may not be familiar with your project, the tools you are using, or any acronyms you include in your slides.
Your stakeholders are all unique. Think of them as characters from Mr. Men and Little Miss; they all have different expectations. Here are three tips to better understand your stakeholders:
● Put yourself in the shoes of your stakeholders: Try to imagine you are them and predict what they expect from you.
● Ask yourself ‘what’s in it for them?’: If you want people to follow you, consider what benefits they will gain from it.
● Lead change before it occurs: Any data initiative introduces some form of change. Consider what actions you can take to facilitate this change for your stakeholders.
One of the biggest reasons for data project failure is the misalignment between data scientists and internal customers. One way to mitigate this risk is to use a canvas. Figure 2 is an example of the Data Initiative Canvas.
Discussion with your stakeholders is the best way to understand their needs. You would be surprised how often what they request is not what they truly need. Finally, setting the right expectations with your stakeholders, especially top management, is critical. In the data-driven transformation, the mindset shifts from strong scepticism (e.g., ‘this can’t be solved without our experts’) to unrealistic expectations (e.g., ‘AI is going to solve all our problems’), as depicted in Figure 3.
As data scientists, we are accustomed to adapting to new tools or programming languages. Similarly, we should adapt our approach to problem solving. There is a tendency to overuse machine learning (ML) – the hammer – and attempt to solve every problem – the nail – with overly complex approaches. While a junior data scientist knows well how to use machine learning, someone more senior will also know when not to use ML.
Indeed, just because we have easy access to ML algorithms doesn’t mean everything should be solved with ML. When approaching a new problem, check with your stakeholders whether business rules are available. This could solve the problem much more easily than using ML.
Although not usually attractive to data scientists, leveraging off-the-shelf solutions (low-code/no-code tools) can achieve fast impact with minimal effort. Certainly, you can now use generative AI to generate code for you, but start by considering whether you really need code at all.
Simple and imperfect solutions can have a significant business impact. As data scientists, we often seek complex and perfect solutions to our problems. However, it’s important to remember that companies operate in uncertain environments. Perfect accuracy isn’t necessary to generate impact, and a simple, easy-to-understand model is likely to have more impact than a complex, opaque model.
With the recent excitement around generative AI (as was the case with deep learning) it’s easy to lose sight of the business objectives and focus solely on finding exciting tools or algorithms to use. Remember, for your stakeholders, the impact is what truly matters, not the technology itself. When considering what generates impact in any data initiative, focus on how people understand what you do (and why), as illustrated in Figure 4.
Enhancing abilities in areas such as data storytelling, communication, stakeholder management, adaptability, and business acumen can significantly increase the impact of your data initiatives.
One efficient way to build trust is by sharing the insights you generate while preparing and exploring data. Waiting until the end of the project to share predictions or forecasts with your stakeholders is risky, as they may not accept such figures if trust has not been established. By sharing insights about the data –which often your customers are unaware of – you create value for them as they learn something new.
Finally, creating impact is about transitioning from technical results (such as accuracy and ROC curves) to business metrics (like revenue generated and cost reductions). Strive to shift from machine learning outputs to business KPIs. These figures will help you gauge the real impact of your initiative on the company.
In conclusion, developing your soft skills is crucial,
and complements technical competencies like statistics, machine learning, and programming. Emphasising soft skills recognises the importance of the human element in the data-driven transformation of companies. As discussed, enhancing abilities in areas such as data storytelling, communication, stakeholder management, adaptability, and business acumen can significantly increase the impact of your data initiatives.
So, how do you ensure your data scientists possess the necessary soft skills for the job? First, consider soft skills when hiring new data scientists. The usual interview process focuses on solving machine learning problems and writing code. Shifting some of this focus to soft skills will help you build a team with the right talents to make a significant impact within the company. For existing data scientists, soft skills can be developed through training (a course I am passionate about teaching) and by reading books on the topic, such as Business Skills for Data Scientists by David Stephenson. The future belongs to those who can not only analyse data but also inspire action, lead change, and drive business outcomes.
JAMES DUEZ is the CEO and co-founder of Rainbird.AI, a decision intelligence business focused on the automation of complex human decisionmaking. James has over 30 years’ experience building and investing in technology companies with experience in global compliance, enterprise transformation and decision science. He has worked extensively with Global 250 organisations and state departments, is one of Grant Thornton’s ‘Faces of a Vibrant Economy’, a member of the NextMed faculty and an official member of the Forbes Technology Council.
very organisation in the world is focused on leveraging artificial intelligence (AI) and data, and this is only accelerating since the advancement of generative AI and techniques like retrievalaugmented generation (RAG).
Generative AI is at the top of the hype cycle and expectations remain high. If used carefully there is much potential to aid the efficiency of experts, but generative AI alone cannot evaluate problems logically and in context, nor produce answers that come with a causal chain of reasoning that delivers certainty that every answer is 100% explainable and free from the risk of error.
The quest for systems that not only provide answers but also explain their reasoning in a transparent and trustworthy manner is becoming paramount, at least where AI is being used to make critical decisions.
When Air Canada’s chatbot gave incorrect information to a traveller, the airline argued its chatbot was ‘responsible for its own
actions’ but quickly lost its case, making it clear that organisations cannot hide behind their AIpowered chatbots when they make mistakes.
What went wrong is clear. Generative AI is a machine learning technology that creates compelling predictions, but is not capable of human-like reasoning and cannot provide causal explanations.
While many are stunned by the bright lights of generative AI, it’s best leveraged as one piece of a bigger architecture. As highlighted in the previous cover issue, it is a Neurosymbolic approach to decision intelligence that holds the greatest value – to deliver solutions that start with a clear focus on outcomes and work back from that to a hybrid or composite use of AI. Decision intelligence leverages AI models in a configuration that is grounded in trust and transparency, avoiding the perils of noise, bias and hallucination.
This thinking is now validated
by numerous analysts, including Gartner, who convey decision intelligence as being of equivalent importance to generative AI in terms of both its transformational potential and the timescale to mainstream adoption.
What’s more, the issue of accuracy and explainability to drive trust is becoming recognised as a critical component, with knowledge graphs accepted as the primary grounding technology for generative AI and therefore, a key enabler to its adoption.
Organisations have the ambition but also the responsibility to uncover and leverage ways of using AI responsibility in a world that is only becoming more regulated.
As generative AI continues to rapidly evolve, more and more focus is falling on the importance of trust.
The quest for systems that not only provide answers but also explain their reasoning in a transparent and trustworthy manner is becoming paramount, at least where AI is being used to make critical decisions.
AI leaders have continued to grapple with the challenges of leveraging generative AI, specifically large language models (LLMs) which risk outputting incorrect results (known as hallucinations) and lack formal explainability in their answers.
One architecture that has gained momentum is that of retrieval-augmented generation (RAG). It uses well-understood mathematical techniques to identify similar snippets from a reference source of documents, and then injects those snippets into an LLM to help inform outputs.
Although RAG still risks hallucinations and lacks explainability, it has the advantage of being able to make content predictions over targeted document sources and can point at the parts of the document that it used when generating its predicted outputs.
However, RAG is inherently limited by its exploratory nature, which focuses on accumulating knowledge or facts that are then summarised, without a deep understanding of the context or any ability to provide logical reasoning.
LLMs alone are poor at reasoning as was pointed out recently by Yann LeCun, one of the godfathers of AI and Head of AI at Meta. Regardless of whether you ask a simple question, a complex question or even an impossible question – the amount of computing power expended to create each block of generated content (or token) is the same.
This is not the way real-world reasoning works. When
humans are presented with complex problems we apply more effort to reasoning over complexity. With LLMs alone, the information generated may look convincing but could be completely false and demand that the user then spends lots of time checking the veracity of the output.
Architectures are evolving to try and improve the performance of LLMs, including retrieval-augmented thought (RAT) to pull in external data, semantic rails to try and keep LLMs on topic and prompt chaining to turn LLM outputs into new LLM inputs. All of these are designed to diminish risks but cannot eliminate them.
But a new architecture is taking hold, that of retrieval-augmented reasoning (RAR), an innovative approach that transcends the limitations of RAG by integrating a more sophisticated method of interaction with information sources.
Unlike RAG, RAR doesn’t just seek to inform a decision by generating text; it actively and logically reasons like a human would, engaging in a dialogue with sources and users to gather context, then employing logical reasoning to produce answers accompanied by a logical rationale. It requires a symbolic reasoning engine and
uses a very high-level knowledge graph to work.
The distinction between RAG and RAR is not merely technical but fundamentally changes how AI systems can be applied to solve real-world problems. It’s comprehensive, accurate and represents the ultimate in guardrailing, so it cannot hallucinate.
We all now know that LLMs produce answers based on their training from the public internet. Risks of hallucination are high and the lack of explainability is a serious problem.
RAG’s approach, while useful for exploratory queries, falls short when faced with the need to understand the nuances of specific situations or to provide answers
that are not only accurate but also logically sound and fully explainable. While it can point to sources for its predictions, it can still hallucinate and cannot explain its reasoning.
RAR however addresses all these challenges head-on by enabling a more interactive and iterative process of knowledge retrieval, consultation, and causal reasoning.
For example, RAR can enable lawyers to interact with legislation and complex case law in the context of their case, and obtain a causal chain of reasoning for worked answers. RAR can power tax solutions, reasoning over large amounts of regulation to find appropriate tax treatments at a transaction level.
These use cases all represent the ability to reason in a way that is contextually relevant, free from hallucination, and backed by a clear chain of reasoning. It’s sector agnostic and enables the rapid creation of
digital assistants in any domain.
But there is yet another benefit of the RAR architecture.
Because it uses a symbolic reasoning engine and a knowledge graph to navigate document sources, the graph itself can be extended to incorporate human expertise. This enables models to leverage both documented regulation, policy or operating procedure and human tribal knowledge, enhancing its contextual decision-making capabilities.
RAR is particularly valuable in regulated markets, where evidence-based rationales are crucial to trust and therefore to adoption. By providing answers that come with source references and logical rationales, RAR fosters a level of trust and transparency that is essential in today’s data-driven world.
As we continue to navigate the challenges and opportunities presented by AI, approaches like RAR will be instrumental in ensuring that our technology not only answers our questions but does so in a way that we can understand and trust.
While RAG has served as a valuable tool in the AI toolkit, the advent of RAR represents a significant leap forward in our ability to harness the power of AI for complex decision-making.
By offering nuanced answers that are grounded in logical reasoning and contextual understanding, RAR opens up new possibilities for AI applications that
require a high degree of trust and explainability.
As we continue to navigate the challenges and opportunities presented by AI, approaches like RAR will be instrumental in ensuring that our technology not only answers our questions but does so in a way that we can understand and trust.
ANTHONY ALCARAZ is the Chief AI Officer and Partner at Fribl. His work at Fribl is at the cutting edge of integrating advanced AI solutions into HR, streamlining the final stages of candidate evaluation, and ensuring optimal matches between job seekers and available positions.
Beyond his role at Fribl, Anthony is a consultant for startups, where his expertise in decision science, particularly at the intersection of large language models, natural language processing, knowledge graphs, and graph theory is applied to foster innovation and strategic development.
Anthony’s specialisations have positioned him as a leading voice in the construction of retrieval-augmented generation (RAG) and reasoning engines, regarded by many as the state-of-the-art approach in our field. He’s an avid writer, sharing daily insights on AI applications in business and decisionmaking with his 30,000+ followers on Medium. Anthony recently lectured at Oxford on the integration of artificial intelligence, generative AI, cloud and MLOps into contemporary business practices.
LLMs still frequently make basic logical and mathematical mistakes that reveal a lack of systematicity behind their responses. Their knowledge remains intrinsically statistical without deeper semantic structures.
The recent exponential advances in natural language processing capabilities from large language models (LLMs) have stirred tremendous excitement about their potential to achieve human-level intelligence. Their ability to produce remarkably coherent text and engage in dialogue after exposure to vast datasets seems to point towards flexible, general-purpose reasoning skills.
However, a growing chorus of voices urges caution against unchecked optimism by highlighting fundamental blindspots that limit neural approaches. LLMs still frequently make basic logical and mathematical mistakes that reveal a lack of systematicity behind their responses. Their knowledge remains intrinsically statistical without deeper semantic structures.
More complex reasoning tasks further expose these limitations. LLMs struggle with causal, counterfactual, and compositional reasoning challenges that require going beyond surface pattern recognition. Unlike humans who learn abstract schemas to flexibly recombine modular concepts, neural networks memorise correlations between co-occurring terms. This results in brittle generalisation outside narrow training distributions.
The chasm underscores how human cognition employs structured symbolic representations to enable systematic composability and causal models for conceptualising dynamics. We reason by manipulating modular symbolic concepts based on valid inference rules, chaining logical dependencies, leveraging mental simulations, and postulating mechanisms relating to variables. The inherently statistical nature of neural networks precludes developing such structured reasoning.
It remains mysterious how symbolic-like phenomena emerge in LLMs despite their subsymbolic substrate. But clearer acknowledgement of this ‘hybridity gap’ is imperative. True progress requires embracing complementary strengths – the flexibility of neural
ANTHONY ALCARAZapproaches with structured knowledge representations and causal reasoning techniques – to create integrated reasoning systems.
We first outline the growing chorus of analyses exposing neural networks’ lack of systematicity, causal comprehension, and compositional generalisation –underscoring differences from innate human faculties.
Next, we detail salient facets of the ‘reasoning gap’, including struggles with modular skill orchestration, unravelling dynamics, and counterfactual simulation. We consider the innate human capacities contemporary ML lacks, explaining the resulting brittleness.
Seeking remedies, we discuss knowledge graphs as scaffolds for explicit conceptual relationships missing from statistical learning. We highlight approaches for structured knowledge injection – querying interfaces and vectorised graph embeddings – to contextualise neural generation.
We present techniques like dimensional typing in embeddings and parallel knowledge retrieval to improve inductive biases for logical deduction and efficient inference. Finally, we make the case for patiently cultivating high-quality knowledge graphs as strategic assets for enterprises pursuing substantive AI progress.
Knowledge graphs offer a promising method for overcoming the ‘reasoning gap’ plaguing modern LLMs. By explicitly modelling concepts as nodes and relationships as edges, knowledge graphs provide structured symbolic representations that can augment the flexible statistical knowledge within LLMs.
Establishing explanatory connections between concepts empowers more systematic, interpretable reasoning across distant domains. LLMs struggle to link disparate concepts purely through learned data patterns. But knowledge graphs can effectively relate concepts not directly co-occurring in text corpora by providing relevant intermediate nodes and relationships. This scaffolding bridges gaps in statistical knowledge, enabling logical chaining.
Such knowledge graphs also increase transparency and trust in LLM-based inference. Requiring models to display full reasoning chains over explicit graph relations mitigates risks from unprincipled statistical hallucinations. Exposing the graph paths makes statistical outputs grounded in validated connections.
Constructing clean interfaces between innately statistical LLMs and structured causal representations shows promise for overcoming today’s brittleness. Combining neural knowledge breadth with external knowledge depth can nurture the development of AI systems that learn and reason both flexibly and systematically.
Knowledge graph querying and graph algorithms are powerful tools for extracting and analysing complex relationships from large datasets. Here’s how they work and what they can achieve:
Knowledge Graph Querying:
Knowledge graphs organise information as entities (like books, people, or concepts) and relationships (like authorship, kinship, or thematic connection). Querying languages like SPARQL and Cypher enable the formulation of queries to extract specific information from these graphs. For instance, the query mentioned in your example finds books related to ‘Artificial Intelligence’ by matching book nodes connected to relevant topic nodes.
Graph Algorithms:
Beyond querying, graph algorithms can analyse these structures in more profound ways. Some typical graph algorithms include:
● Pathfinding Algorithms (e.g., Dijkstra’s, A*): Find the shortest path between two nodes, useful in route planning and network analysis.
● Community Detection Algorithms (e.g., Louvain method): Identify clusters or communities within graphs, helping in social network analysis and market segmentation.
● Centrality Measures (e.g., PageRank, betweenness centrality): Determine the importance of different nodes in a network, applicable in analysing influence in social networks or key infrastructure in transportation networks.
● Recommendation Systems: By analysing useritem graphs, these systems can make personalised recommendations based on past interactions.
LLMs can generate queries for knowledge graphs based on natural language input. While they excel in understanding and generating human-like text, their statistical nature means they’re less adept at structured
logical reasoning. Therefore, pairing them with knowledge graphs and structured querying interfaces can leverage the strengths of both: the LLM for understanding and contextualising user input and the knowledge graph for precise, logical data retrieval.
Incorporating graph algorithms into the mix can
Knowledge graph embeddings encode entities and relations as dense vector representations. These vectors can be dynamically integrated within LLMs using fused models.
For example, a cross-attention mechanism can contextualise language model token embeddings by matching them against retrieved graph embeddings. This injects relevant external knowledge.
Mathematically fusing these complementary vectors grounds the model, while allowing gradient flows across
further enhance this synergy. For instance, an LLM could suggest a community detection algorithm on a social network graph to identify influential figures within a specific interest group. However, the challenge lies in integrating these disparate systems in a way that is both efficient and interpretable.
both components. The LLM inherits relational patterns, improving reasoning.
So both querying and embeddings provide mechanisms for connecting structured knowledge graphs with the statistical capacities of LLMs. This facilitates interpretable, contextual responses informed by curated facts.
The path towards safe, performant, and explainable AI undoubtedly lies in architecting hybrid systems with distinct reasoning modules suited to their strengths while mitigating individual weaknesses through
symbiotic integration. Knowledge graphs offer the structural scaffolding to elevate LLMs from pattern recognisers to context-aware, disciplined reasoners.
Knowledge graph embeddings can be further enhanced by incorporating additional constraints and structure beyond just encoding factual entities and relationships. This provides useful inductive biases to orient semantic similarity and reasoning in more reliable ways.
Some examples include:
Dimensional typing
Assigning dedicated dimensions in the embedding space to model specific hierarchical knowledge categories (like types, attributes, temporal bins etc.) allows interpreting vector arithmetic and symmetry operations.
Logical rules as vector equations
Modelling logical rules like transitivity as vector equations over relation embeddings bakes in compliance with first-order logic when querying the vector space.
Adding a linkage loss that pulls together vector representations for the same real-world entities improves generalisation across surface forms.
Temporal Ordering
Encoding time series knowledge by chronologically positioning entity embeddings assists in analogical reasoning over time.
Overall, embellishing knowledge graph embeddings with structured inductive biases – whether through typed dimensions, vector logic, or temporal ordering – makes the vector arithmetic better comport with real-world constraints. This strengthens their ability to tackle complex reasoning tasks, providing useful scaffolds that can likewise elevate the performance of integrated language models.
The core benefit is infusing domain knowledge to orient the latent geometry in precise ways, amplifying the reasoning capacity of connectionist models interacting with the vector space. Guiding the primitives the neural engine operates upon through structured initialisation fosters more systematic compositional computation accessible through queries.
First retrieving relevant knowledge graph embeddings for a query before querying the full knowledge graph. This twostep approach allows efficient focus of graphical operations:
Given a natural language query, relevant knowledge graph embeddings can be quickly retrieved using approximate nearest neighbour search over indexed vectors.
For example, using a query like: ‘Which books discuss artificial intelligence’, vector search would identify embeddings of the Book, Topic, and AI Concept entities.
This focuses the search without needing to scan the entire graph, improving latency. The embeddings supply useful query expansion signals for the next step.
The selected entity embeddings suggest useful entry points and relationships for structured graphical queries and algorithms.
In our example, the matches for Book, Topic, and AI Concept cues exploration of BOOK-TOPIC and TOPICCONCEPT connections.
Executing a query like:
This traverses books linked to AI topics to produce relevant results.
Overall, the high-level flow is:
1. Use vector search to identify useful symbolic handles
2. Execute graph algorithms seeded by these handles
This tight coupling connects the strength of similarity search with multi-hop reasoning.
The key benefit is focusing complex graph algorithms using fast initial embedding matches. This improves latency and relevance by avoiding exhaustive graph scans for each query. The combination enables scalable, efficient semantic search and reasoning over vast knowledge.
The key idea behind using multiple knowledge graphs in parallel is to provide the language model with a broader scope of structured knowledge to draw from during the reasoning process. Let me expand on the rationale:
1. Knowledge Breadth: No single knowledge graph can encapsulate all of humanity’s accrued knowledge across every domain. By querying multiple knowledge graphs in parallel, we maximise the factual information available for the language model to leverage.
2. Reasoning Diversity: Different knowledge graphs may model domains using different ontologies, rules, constraints etc. This diversity of knowledge representation exposes the language model to a wider array of reasoning patterns to learn.
3. Efficiency: Querying knowledge graphs in parallel allows retrieving relevant information simultaneously. This improves latency compared to sequential queries. Parallel search allows more rapid gathering of contextual details to analyse.
4. Robustness: Having multiple knowledge sources provides redundancy in cases where a particular graph is unavailable or lacks information on a specific reasoning chain.
5. Transfer Learning: Being exposed to a multitude of reasoning approaches provides more transferable learning examples for the language model. This enhances few-shot adaptation abilities.
So in summary, orchestrating a chorus of knowledge graphs provides breadth and diversity of grounded knowledge to overcome limitations of individual knowledge bases. Parallel retrieval improves efficiency and robustness. Transfer learning across diverse reasoning patterns also accelerates language model adaption. This combination aims to scale structured knowledge injection towards more humanlike versatile understanding.
integration saves the effort of manually handling all symbol grounding and edge cases explicitly.
Leveraging the innate capacities of LLMs for semantic generalisation allows structured programs to focus on providing logical constraints and clean interfaces. The LLM then handles inconsistencies and gaps through adaptive few-shot learning. This symbiotic approach underscores architecting AI systems with distinct reasoning faculties suited for their inherent strengths.
The exponential hype around artificial intelligence risks organisations pursuing short-sighted scripts promising quick returns. But meaningful progress requires patient cultivation of high-quality knowledge foundations. This manifests in structured knowledge graphs methodically encoding human expertise as networked representations over time.
While vector search and knowledge graphs provide structured symbolic representations, LLMs like GPT-3 offer unstructured yet adaptive semantic knowledge. LLMs have demonstrated remarkable few-shot learning abilities, quickly adapting to new domains with only a handful of examples.
This makes LLMs well-suited to act as a fluid semantic glue between structured modules – ingesting the symbolic knowledge, interpreting instructions, handling edge cases through generalisation, and producing contextual outputs. They leverage their vast parametric knowledge to rapidly integrate with external programs and data representations.
We can thus conceive of LLMs as a dynamic, everoptimising semantic layer. They ingest forms of structured knowledge and adapt on the fly based on new inputs and querying contexts. Rather than replacing symbolic approaches, LLMs amplify them through rapid binding and contextual response generation. This fluid
Curating clean abstractions of complex domains as interconnected entities, constraints and rules is no trivial investment. It demands deliberate ontology engineering, disciplined data governance and iterative enhancement. The incremental nature can frustrate business leaders accustomed to rapid software cycles.
However, structured knowledge is AI’s missing pillar – curbing unbridled statistical models through grounding signals. Knowledge graphs provide the scaffolding for injectable domain knowledge, while enabling transparent querying and analysis. Their composable nature also allows interoperating with diverse systems.
All this makes a compelling case for enterprise knowledge graphs as strategic assets. Much like databases evolved from flexible spreadsheets, the constraints of structure ultimately multiply capability. The entities and relationships within enterprise knowledge graphs become reliable touchpoints for driving everything from conversational assistants to analytics.
In the rush to the AI frontier, it is tempting to let unconstrained models loose on processes. But as with every past wave of automation, thoughtfully encoding human knowledge to elevate machine potential remains imperative. Managed well, maintaining this structured advantage compounds over time across applications, cementing market leadership. Knowledge powers better decisions – making enterprise knowledge graphs indispensable AI foundations.