TRANSFORMING DATA CULTURE AT MERCK WALID MEHANNA
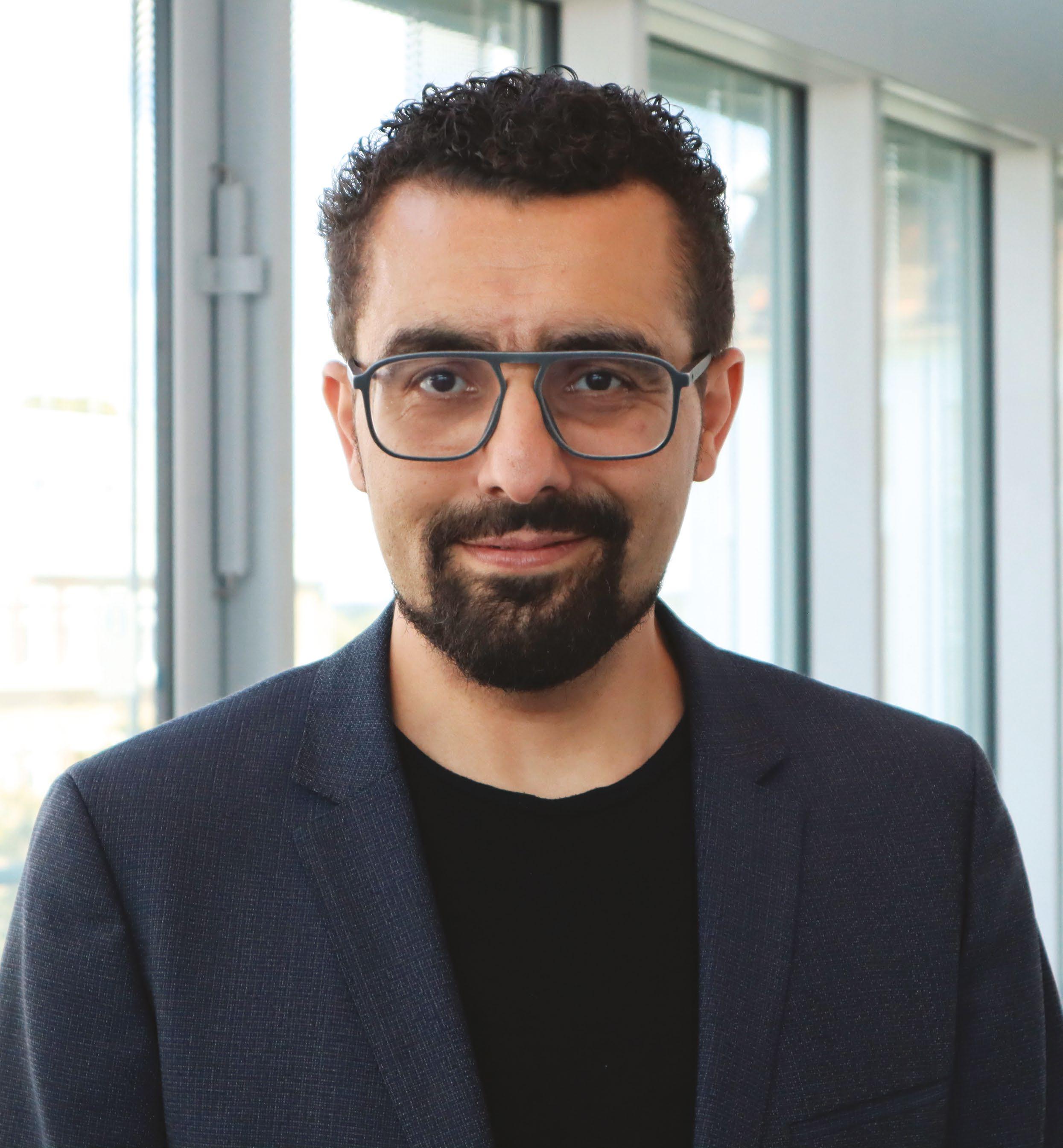
FROM HUMANS TO HYBRIDS: PREPARING YOUR WORKFORCE FOR AI and more.. .
HARNESSING GEN AI TO SUPPORT
THE COST EFFECTIVE METHOD FOR RETRAINING LLM’S
FROM HUMANS TO HYBRIDS: PREPARING YOUR WORKFORCE FOR AI and more.. .
HARNESSING GEN AI TO SUPPORT
THE COST EFFECTIVE METHOD FOR RETRAINING LLM’S
Expect smart thinking and insights from leaders and academics in Data Science and AI as they explore how their research can scale into broader industry applications.
The Biological Model is the idea that all decision-making power and resources should be consolidated into a single epicentre... Data Strategy Evolved: How the Biological Model fuels enterprise data performance with PATRICK MCQUILLAN
Papers that get retracted in the future have a wider attention or are shared more broadly after publication. How Science is (Mis)communicated in Online Media with ÁGNES HORVÁT
Observability really is the idea that you’re able to measure the health of your data system.
How Observability is Advancing Data Reliability and Data Quality with LIOR GAVISH and RYAN KEARNS
Helping you to expand your knowledge and enhance your career.
CONTRIBUTORS
Walid Mehanna
Mathias Winkel
Harsha Gurulingappa
Stefanie Babka
Lin Wang
Aakash Shirodkar
Filipa Castro
Tanmaiyii Rao
Andreu Mora
Faisal Wasswa
Martijn Bauters
Tarush Aggarwal
Francesco Gadaleta
Philipp M. Diesinger
Elle Neal
Sarah-Jane Smyth
Jasmine Grimsley
Katherine Gregory
EDITOR
Anthony Bunn
anthony.bunn
@datasciencetalent.co.uk
+44 (0)7507 261 877
DESIGN
Imtiaz Deighan
PRINTED BY Rowtype
Stoke-on-Trent, UK
+44 (0)1782 538600
sales@rowtype.co.uk
NEXT ISSUE
20TH NOVEMBER 2023
The Data Scientist is published quarterly by Data Science Talent Ltd, Whitebridge Estate, Whitebridge Lane, Stone, Staffordshire, ST15 8LQ, UK. Access a digital copy of the magazine at datatasciencetalent.co.uk/media.
IN
DISCLAIMER
The views and content expressed in The Data Scientist reflect the opinions of the author(s) and do not necessarily reflect the views of the magazine or its staff. All published material is done so in good faith.
All rights reserved, product, logo, brands, and any other trademarks featured within The Data Scientist magazine are the property of their respective trademark holders. No part of this publication may be reproduced, stored in a retrieval system, or transmitted in any form by means of mechanical, electronic, photocopying, recording, or otherwise without prior written permission. Data Science Talent Ltd cannot guarantee and accepts no liability for any loss or damage of any kind caused by this magazine for the accuracy of claims made by the advertisers.
Whilst we focus heavily on this particular uber-hot topic in this issue, we don’t do so exclusively. You’ll find other articles that examine different areas of Data Science, and a few of our regular features, too.
Before anything else, can we firstly thank those of you who have played such an important part in the magazine - in contribution and readership. And thanks also for the fantastic feedback that we have had so far. As we always say, a magazine is only as good as those who write for it and those who read it.
Generative AI has already made a significant impression in various domains, affecting many parts of work and everyday life. Looking ahead, the potential impact of it is vast and transformative and will touch everything from design, to music, to entertainment and healthcare.
In this issue we take a look at what large companies such as Merck and Continental are up to with LLM’s as they get started on building enterprise AI products that deliver value. We also delve into important technical areas such as training LLM’s with LoRA and how the semantic layer is going to help companies connect their domain specific datasets to generative AI systems.
After editing this issue, it’s great to see that as we navigate this evolving landscape, many are prioritising ethical considerations and ensuring that it serves humanity’s best interests, too. By harnessing the power of Generative AI responsibly, we can embark on a journey towards an exciting and creative future. One that we will be writing about for many issues to come.
We hope that you enjoy this issue.
The Data Scientist Editorial TeamAs the digital landscape continues to evolve, companies are constantly looking for new and innovative ways to incorporate technology into their operations. At MERCK KGAA , Darmstadt, Germany, a team of experts has been working on a new tool that promises to take data culture to the next level.
myGPT @ Merck is a generative AI tool that is changing the game for employees at Merck.
TO LEARN MORE ABOUT THIS EXCITING DEVELOPMENT, WE SPOKE WITH THE TEAM BEHIND MYGPT.
Walid Mehanna is the Chief Data & AI Officer at Merck. Together with his team, he establishes the enterprise-wide data strategy, a comprehensive Data & Analytics ecosystem, operating model, and data culture at Merck. He also chairs the Merck Digital Ethics Advisory Panel, guiding the company’s ethical standards in the digital realm.
How is the Data, Analytics & AI topic strategically set up at Merck?
Leveraging the immense potential of Data and AI is integral to both our present and future business success. To this end, we have crafted a comprehensive, organisation-wide data strategy and an integrated Data and Analytics Ecosystem, at the heart of which are Palantir Foundry and Amazon Web Services. We defined common ways of working for everything related to Data and Analytics and ignited a lot of excitement for the topic through various data culture activities. Our ambition is to pioneer digital ethics, and in pursuit of this, we have implemented a Code of Digital Ethics and assembled a Digital Ethics Advisory Panel.
As we continually refine our data strategy, we are now
expanding it to encompass the AI domain. We also took our organisational setup to the next level and recently announced the formation of the Merck Data & AI Organisation which I have the pleasure to lead. We also have an AI Research team in our Science & Technology Office that is looking into new trends and emerging technologies.
We organise ourselves in a federated operating model that we call hub-hub-spoke. Everything that makes sense to only do once on the enterprise-level is done in the corporate center I head up. Then we also have hubs in the different business sectors and one for the group functions and, of course, many people that operate directly in the businesses and functions. We call them “spokes.” And we have a regular decision committee that we call the Merck Data Council. In this committee my peers in the sectors as well as representatives from IT, Security, Data Privacy, and I jointly shape and execute our data strategy.
myGPT @ Merck is an artificial intelligence-based digital assistant designed to support Merck employees in their daily work. It is powered by the GPT (Generative Pretrained Transformer) language models developed by OpenAI, and it can understand natural language queries and provide information and helpful responses. The current version of myGPT @ Merck uses the model gpt3.5-turbo.
What were your strategic thoughts behind launching myGPT @ Merck ?
We are a vibrant science & technology company with many curious minds. When ChatGPT came up, of course, those curious colleagues started to play around with it. We wanted to encourage them to embrace the new possibilities, but at the same time, we were concerned about our data security and the potential leakage of internal or even confidential information through a tool like this. This is why we decided to make this technology available in a Merck-compliant way and created our own GPT chatbot in collaboration with Microsoft.
What is the most exciting development around Data & AI, in your opinion?
The domain of Data & AI is full of exhilarating developments, making it difficult to pick just one. However, as someone deeply immersed in data, I find the growing recognition of the importance of high-quality data particularly gratifying. The increased interest in AI has made it clear that effective data management
and governance are critical. After all, the quality of our insights directly mirrors the quality of our data - if we input garbage, we’ll output garbage. One promising aspect of AI is its potential to streamline and enhance our data supply through automation.
Equally exciting is the increasing integration of Large Language Models (LLMs) into various services via APIs. This promises significant advancements, although it requires rigorous testing and governance to ensure seamless and reliable operation. The potential benefits to streamline and automate operations, however, are tremendous.
Looking further into the future, the digital representation and simulation of drugs, materials, and, eventually, the human body, maybe represents the final frontier for us. This possibility, which may seem like science fiction today, could become a reality with the continued development of massive compute, data and AI. To me, it represents the ultimate challenge and aspiration for us as Data & AI professionals in science and technology.
Mathias Winkel leads the AI & Quantum Lab at Group Digital Innovation within the Merck Science and Technology organisation. With his team of experts in many scientific disciplines, he is continuously scouting for new and exciting technologies in AI and novel methods of computing. Matching them to real business problems, the team is propelling innovation powered by data and digital for the company, its customers, and patients.
Tell us about the AI & Quantum Lab at Merck Merck has more than 350 years of pharmaceutical and chemical tradition and corresponding expertise. The company’s ongoing success as a leading science and technology company has only been possible through permanent innovation. Today, this innovation imperatively needs to be powered by data and digital.
The AI & Quantum Lab within Group Digital Innovation at Merck is one of the essential building blocks to bring this aspiration to life. It is a team of highly specialised experts on the permanent lookout for new and exciting digital technologies that are documented in scientific publications, are developed by startups, or are industrialised by established
companies. We assess methods in fields ranging from artificial intelligence and machine learning to quantum computing, fit them to existing business problems at Merck and transfer them into the company.
What makes ChatGPT so special and why did you decide to do something similar for Merck?
When the global hype about ChatGPT started, the technology behind it was not new: the potential of transformer architectures was already demonstrated by Google researchers at NeurIPS 2017 (Conference on Neural Information Processing Systems) and ChatGPT was preceded by several influential LLMs (large language models) developed by OpenAI as well as other wellkown companies. However, the specific way of training the model through RLHF (reinforcement learning with human feedback) with the specific goal to create a conversational AI that answers user prompts in a way, that cannot be distinguished from a human, has been a unique idea and finally lead to ChatGPT being the most influential development AI in 2022.
This tremendous impact resulted from three aspects. First, the training method and underlying data as well as the extreme size of the model with its 175B parameters made it indeed extremely powerful. For a long time, competing developments were unable to win against GPT-3.5, the underlying model of ChatGPT, in many relevant benchmarks.
Second, the very simple user interface made ChatGPT extremely approachable for everybody. Suddenly, artificial intelligence neither was some specialised black-box algorithm shipped as a hidden part of a huge software suite, nor some magic program that required special knowledge and a level of black art for getting it to do something useful. Instead, people who barely used a computer before, were able to interact with powerful artificial intelligence. Finally, these two properties, paired with initially very restricted access, lead to self-propelling excitement all over the world and an exponential growth in the user base.
As mentioned before, Merck is positioned globally as a leading science and technology company. Equipping our employees with the most powerful and most efficient tools available for serving our customers and patients is a crucial cornerstone to fulfill this ambition. To also protect our customers and patients and their data as well as our company internals, it is however critical to ensure that usage of digital tools at the workplace happens in full compliance with our company’s regulations and within the applicable legal limits. Considering and balancing both aspects lead to the decision to set up myGPT, our own Merck-specific version of ChatGPT.
At the AI & Quantum Lab, we were already actively following the technology and analysed its capabilities and limits at least half a year before ChatGPT was announced. Already since then we were in very good contact with the developers and could build up internal expertise on large language models. This allowed us to understand their potential and limits early on and embark swiftly when we saw a perfect fit of the technology to our business needs. Today, we are actively developing internal applications using LLMs that go far beyond the well-known chat bot capabilities. The global excitement around ChatGPT has been a surprise, but due to our technological foresight we were well prepared.
In today’s rapidly changing world, this foresight is imperative not only to make best use of emerging technologies. It is also necessary to ensure business continuity. Just like digital cameras eradicated the market for classical photography only to be soon replaced by smart phones, every business model is under permanent risk of severe disruption due to technological advancements. This development has become even more serious during the last few years as traditional business limits are diluting more and more: developers of consumer devices look into autonomous driving, developers of electric cars build rockets, social media companies consider developing drugs, etc.
While innovation and technology scouting has to happen permanently at all levels and in all sectors at a company like Merck, it is the task of the enterprise level functions to take the perspective of the company as a whole to ask: which technologies are most promising for all sectors and which ones would be most disruptive for the company in general.
Answering this question is tough, because we are living in extremely exciting times where development cycles have become much quicker than before and nothing seems older than yesterday’s news about novel technologies. When concentrating on LLMs, I see three primary trends that we should expect to have some impact very soon. First, I am sure that these models will dramatically change the way we interact. We will be
ChatGPT discussions will help us as humans to better understand ourselves and maybe even spark insights into what human intelligence is and how the brain is working.
able to describe the desired output in natural language, and AI systems will spare us from most of the mechanic mouse and keyboard interaction to produce our digital creation.
Second, multi modality of models will again open a multitude of new application areas beyond natural language. Think about explanation and generation of images, audio, videos. Imagine the possibilities if these models enter the domain of science, start generating new materials or explaining fundamental laws in a way a human might never have thought of.
Finally, the human-like performance of these models on many tasks and their surprising capabilities as autonomous agents or when it comes to logical reasoning
Harsha Gurulingappa operates as Head of Text Analytics at Merck Data and AI Organisation and located at Merck IT Center, and was instrumental in establishing NLP as one of the core capabilities and service within Merck. In his current role, he is responsible for technological enhancements and adoption of NLP across business sectors within our organisation.
already lead to passionate discussions about General Artificial Intelligence, i.e. AI that can autonomously plan and solve problems it has not seen before.
Independent of their outcome and potential further developments in this direction, these discussions will help us as humans to better understand ourselves and maybe even spark insights into what human intelligence is and how the brain is working. It will also raise new ethical questions about the border between human and machine, about creativity that we consider a unique feature of intelligence, and about how we as humans interact with each other through these technologies. Thus, ChatGPT and its descendants and siblings will challenge humans on multiple levels.
Tell us about your work with NLP topics at Merck The ability to find precise information, facts or figures in time is one of the fundamental requirement for any business or individual. At Merck, we are an organisation with diversified businesses, functions and processes. Having capabilities to process complex data which is multi modal, multilingual and significantly unstructured, and transform them into actionable information and insights is a necessity.
The NLP team holds expertise in development and industralisation of data products and solutions leveraging practices of AI/ML/NLP works towards establishing NLP technical capabilities as part of Merck’s central Data and Analytics ecosystem. By
consulting, partnering as well as upskilling citizen data scientists and data practitioners operating within or in proximity to business functions, we partner in rolling out sustainable NLP solutions which eventually gets integrated into business processes for generating distinguished value.
How did you make myGPT @ Merck work?
The success of ChatGPT and its strong adoption within the market raised an appetite within Merck to have comparable technology which is safe, secure, compliant and easily accessible within Merck.
A team of experts from different functions under the leadership of Chief Data & AI Officer, proactively assembled to drive this journey within a short timespan of less than two months. The team was a composition of colleagues from diverse expertise such as (but not limited to) cloud architecture, NLP engineering, AI/ML scientist, cybersecurity, data privacy, legal, communications as well as experts from Microsoft OpenAI service. myGPT @ Merck was designed, developed, tested, industrialised and released to a cohort group of over ten thousand users. It leverages secure Microsoft OpenAI service in the background and offers a custom designed front-end for the end users with features and functionalities similar to OpenAI’s ChatGPT.
On the other hand, partners from legal, data privacy and communications ensured development of internal policies and procedures, as well as training programs to ensure myGPT @ Merck is used in a compliant way. Altogether, myGPT @ Merck has been in production over a month and there is a surging adoption by the community of users for various purposes to boost efficiency and productivity in their daily business.
myGPT @ Merck is an iconic solution and symbolisation of Consumable AI delivered to be available for every Merck employee. However, as part of the NLP environment within central Data and Analytics ecosystem, there is availability of various generative models offered by industry leading technology providers such as HuggingFace and John Snow Labs.
These models can be finetuned on specific data and tasks. Our NLP environment
allows deploying language models as APIs which can be consumed through data pipelines or custom applications requiring real-time response for end-users.
Large Language Models (LLMs) constitute a family of models which can cover different data modalities, domain specificity (e.g, general purpose or domain specific) and task specificity (e.g., Multi-tasking, text generation only, and more). They can range from having developed with few million parameters (e.g. BERT) to many billion parameters (e.g. GPT-3).
The NLP environment within our Data & Analytics ecosystem allows training, fine-tuning, and transfer learning language models. Such training or fine-tuning tasks have successfully been executed and deployed for various business cases. Most of the applications of LLMs fall in the category of information extraction from semior unstructured documents and retrieval augmented generation (RAG, aka. Retrieval augmented questionanswering).
From the LLM perspective, we are investigating leading proprietary API providers which can offer multilingual and multi-modal operations with the ability to fine-tune and deploy business specific data at viable costs. We are curiously observing the market for applications of GenAI and LLMs across various stages of pharmaceutical product development such as drug discovery, drug development, patient recruitment, regulatory process automation as well as post-market surveillance.
One other exciting development we are monitoring is integration of GenAI and LLMs into workplace technologies (e.g. email, Webex, Office tools, and more).
As the Global Head of Data Culture, Stefanie Babka is responsible for communication, change management and upskilling programs on Data & AI including the Merck Data & Digital Academy and the enterprise-wide Data & Digital community. The data culture activities are aiming to bring together artificial intelligence and human intelligence to drive meaningful insights and data-driven decision-making.
Tell us about Data Culture. Why is this important?
Data Culture is a skillset and mindset. You might have heard that saying that “culture eats strategy for breakfast”. According to studies culture is the biggest impediment for organisations to become data-driven. The digital transformation is not only about technology and processes. Indeed it is mostly about the people.
You need to take them with you otherwise the best technology will not bring any value. The beauty of myGPT @ Merck is that the entry barrier to the technology is very low which is why it is easy for people to embrace the tool.
How is myGPT @ Merck influencing the Data Culture at Merck?
I have to say it is a game changer. All of our activities in the data culture team aim to make people aware of the possible with Data & Analytics and AI. With the rise of generative AI tools like ChatGPT, all of a sudden people are able to understand the potential and the value that this can bring to them personally but also for our business. With my GPT @ Merck employees have a tangible application at hand where they can use conversational AI in a safe environment. They can experience it themselves. That is worth more than a hundred hours of theoretical training. And of course they get curious and want to learn more and hopefully they will develop the data creativity to embark on their own Data & Digital exploration journey looking into new ways of working with AI and creating new business models or products.
What Governance do you have around the tool?
The most important rule is that the human person is always going to be in the driver’s seat and myGPT @ Merck is only an assistant helping to do the work in a more efficient way. It is required to validate the outcome and in some use cases also necessary to check back with our legal teams. The tool is available for confidential and internal data with the exception of personal information.
What training and enablement sessions are you providing on the topic?
We currently focus on teaching our guardrails for the tool as well as making people understand how it works. This means we do trainings on demystification of AI that explain how machine learning, deep learning and large language models work. We also offer hands on training for prompt engineering and getting the best value out of this tool.
What is the most exciting development around data culture from your perspective?
I think it is very interesting that this topic has now reached a broader audience and it has been discussed also in popular media for example and all of a sudden even my family comes and asks me about my opinion on, for example, ethical questions with regards to AI. The AI revolution will increase the need for general data literacy.
IN EACH ISSUE OF THE DATA SCIENTIST , WE SPEAK TO THE PEOPLE THAT MATTER IN OUR INDUSTRY AND FIND OUT JUST HOW THEY GOT STARTED IN DATA SCIENCE OR A PARTICULAR PART OF THE SECTOR .
My path into tech was unconventional. I originally pursued a bachelor’s degree in Accounting, Finance & Economics, with the intention of obtaining a Masters in Finance and landing a quantitative role at an investment bank. However, after much soul searching, I decided on a masters in Data Science. This was tough initially due to my lack of computer science background, but my thesis on detecting biomarkers for Parkinson’s disease motivated me to keep going and persevere. After completing my master’s degree, I joined KPMG UK as a consultant.
During my time at KPMG, I worked on multiple projects across various data pillars, including data engineering, business intelligence, data science, and cloud. This allowed me to develop a holistic and comprehensive view of the data journey, picking up new skills in SQL, R, PowerBI, Star Schema dimensional modelling, VBA, and creating machine learning (ML) models for location analytics in R, Python, and building
on Azure. I also worked across different industries, such as retail, healthcare, and supply chain, to name a few.
In addition to technical and industry knowledge, I learned the untaught soft skills that you tend to pick up when you start working in the fast-paced consulting environment. Working with some really good people, and having a super supportive mentor who played an instrumental role in guiding and shaping my early career was a valuable experience. I still remember their advice:
“
Sometimes you need to be patient and do things that you do not necessarily enjoy in order to get to where you want to be or do what you like ”.
This struck a chord with me, and even though it was tough at the time, in hindsight, I fully relate to it. In the corporate world or life in general, you’ll go through moments that make you question why and what you’re doing. But it’s essential to do things that are out of your comfort zone, or that seem monotonous, in order to reach your desired destination.
4, TANMAIYII RAO FROM SNOWFLAKE TALKS ABOUT HER JOURNEY INTO DATA SCIENCE AND BEING A WOMAN WORKING IN THE FIELD.
I first heard about sales engineering from a shared connection who worked at Google Cloud and was kind enough to refer me. Although I had no experience with Google Cloud, I knew that it was one of the top three public cloud providers, alongside AWS and Azure. My knowledge in cloud computing came from my work at KPMG, where I focused on Azure and obtained a few certifications. With my consulting experience and data background, I found pre-sales to be the perfect fit for me. I loved that it was at the intersection of people, strategy, and technology. I could help customers by understanding their needs and advising them on solutions that leveraged technology to drive business value.
At Google Cloud, I worked as a Customer (Sales) Engineer Specialist. The main difference between generic pre-sales and a specialist was the product focus and knowledge. As a specialist, I was expected to have a deep understanding of the domain I was responsible for. I was the Data Analytics and ML Specialist for the Digital Natives cluster in the UK and Ireland. My main role was to guide our customers on best practices using Data Analytics/ML on Google Cloud, demonstrate the Data Analytics/ML capabilities, and show how they were relevant for their specific use case. In addition, being part of Digital Natives, I had the opportunity to work with a number of startup unicorns spanning FinTech, MedTech, retail/e-commerce, MarTech, and Technology. Throughout my time at Google, I led or contributed to several initiatives with a focus on community events and public speaking that also included collaborating with various Google Cloud partners.
I had an amazing time at Google, both on a professional and personal level. I am immensely grateful for having had that opportunity and for having worked with some of the smartest and googliest people I know. The best part of having worked at Google is the lifelong network. There is a large alumni community of Xooglers (ex-Googlers) who help each other throughout their careers. Recently, the community has been proactively helping people impacted by the mass tech layoffs.
Though I really enjoyed Google, I knew that I wanted to do something different in terms of my role. I wanted to retain my core specialist skills in data/ML, while being more closely aligned to the accounts and customers I was working with. As a Pre-Sales Specialist, although I was aligned at an opportunity level (if anything related to data/ML), I was not involved at the full account level unless it was solely focused on data/ML.
Typically, moving to a generalist role meant I would be covering the entire cloud portfolio, which meant I would not be as focused on data/ML as I would have liked to be. When I got the opportunity to work in a Sales Engineering role at Snowflake, I discovered that not only do they have an amazing product, but also the company values and the team are exceptional and aligned with what I was looking for. I was excited to be part of Snowflake, especially with the organisation growing at such a rapid scale and expanding its ML capabilities.
Snowflake is a data platform built in the cloud, which has a unique, highly scalable architecture that supports multiple workloads. Snowflake originally started with the goal of disrupting data and analytics silos, and then expanded into collaboration with data sharing, and most recently has focused on breaking down ML and development silos with products like Snowpark, the Native App Framework (currently in preview), Streamlit, and more. I love the fact that I get to work closely with Snowflake customers, while also building my expertise across the data and ML lifecycle. The scope is only getting bigger, and with Snowflake the best part is that everything is connected to data at its core.
As a Sales Engineer at Snowflake, I am aligned to various customer accounts and I get to be a part of the full customer journey (which is what I was looking for compared to my previous role). I now work closely with customers end-to-end, while also using my technical knowledge in data analytics and data science. I currently work with customers in the energy space who are leveraging data and/or ML to make data-driven decisions and realise value. Besides data and tech, it’s been fascinating to immerse myself and learn how the energy industry works and the interconnected channels. In addition to my day-to-day activities, I have been involved in leading or contributing to multiple initiatives including data science and MLOps enablement for UK and Ireland Sales Engineering teams, community events such as the Snowflake Python meetup with London Python, and speaking at Snowflake developer events (BUILD.local), amongst other events.
With the growth of big data and ML, there are definitely more opportunities and job prospects for women. Despite this growth, it is disappointing that the gender
A 2021 survey from Deloitte reinforced that a diverse team is better equipped to address the biases in data and AI to build efficient systems.
gap is still very much prevalent, especially in technical roles. Women only account for 26% in IT (womenintech. co.uk, 2023) and only 20% within Data and AI roles (Alan Turing Institute, 2023).
I have been incredibly fortunate to have some amazing mentors, managers, and peers who have been instrumental in my development and success at work. It is very important to have a strong support system and network to be successful at work and for career growth.
A trait that I observed in women across the workforce is that they sometimes underplay their accomplishments and skills and don’t speak up as often. My advice for young women starting out in tech would be to not be afraid to reach out to their network (build a network of supporters in the first place), ask for help, share thoughts (speak up), and celebrate success. It is important to remember that one person cannot know everything, so it’s okay to ask for help. It is also important to acknowledge and accept that we know more than we think we know, and to share our thoughts and views at the appropriate time.
As a woman in data, I have been in numerous situations where I tend to be the only woman, and sometimes the only woman of colour. This doesn’t bother me. I have been fortunate enough to be surrounded by very supportive colleagues throughout my career. Having said that, I must acknowledge that there were moments when I felt that I wasn’t being taken seriously because of my gender and/or age. This made me doubt myself and my capabilities, and this can have quite a negative impact - especially in the early career stages. Unfortunately, I also quickly learned to navigate patronising behaviour towards me. I now understand my boundaries, and how to stand up for myself to avoid situations like this in the future. I am often reminded of this analogy: whereby in the majority of restaurants, the bill is naturally presented to the man first when they’re dining with a woman. It sometimes happens in organisations too that when a man and woman work together on a project, there’s an assumption that the man is the lead. Many of these experiences have shaped me. I work even harder to prove myself and always try to be on top of my game because I have this fear of not being taken seriously. It is a sad state of affairs that women sometimes seem forced to prove themselves again and again, because of various in-built assumptions and stereotypes prevalent in society.
I believe that it takes conscious learning and unlearning by everyone to break these biases and perceptions about women and the types of jobs they do, alongside the contributions they make. Our lived experience is different from men and a diverse and inclusive team can massively contribute to better problem solving and drive innovation. A 2021 survey
from Deloitte reinforced that a diverse team is better equipped to address the biases in data and AI to build efficient systems. We do see progress compared to previous years, however, there is definitely room for improvement in the diversity, equity, and inclusion space. It is good to see most companies now investing time and resources to make their organisation more inclusive. Creating a safe space where you are encouraging collaboration and curiosity, recognising contributions, not penalising people for speaking up, and offering support goes a long way in creating a culture of inclusivity and belonging. Secondly, although it is difficult and it doesn’t come intuitively to most people, practicing empathy contributes to fostering an inclusive culture - especially in data and technology.
Last but not the least, it’s critical to remember that representation matters. I have noticed the lack of women/women of colour role models in leadership roles across both the data and technology landscape. In fact, most of my mentors and managers at work have been men (although strong allies!). Having women leaders share their stories and time would significantly encourage more women to pursue careers in data and tech. Whether through a STEM degree or a non-linear path into tech, having relatable role models and mentors would be an inspiration.
IN RECENT MONTHS, WE’VE WITNESSED A SEISMIC SHIFT IN ARTIFICIAL INTELLIGENCE.
This transformation, resembling a grand renaissance, has been sparked by large language models (LLMs) like OpenAI’s GPT series. What was once considered simple pattern prediction has now unveiled emergent capabilities that have taken centre stage, revolutionising our conception of AI’s potential. The prospect of achieving Artificial General Intelligence (AGI) has rocketed skyward, setting us on an accelerated path of adaptation and adoption that has left many astounded, eager, and even fearful.
By LIN WANGAs a people leader interested in the developing talent of Data Scientists, I’ve observed a wave of change sweeping across the tech sector. Companies are in full sprint, vying to stay ahead of the technological curve. In the scramble to adapt, however, there’s a blind spot emerging: the crucial element of human potential seems to be getting sidelined.
This brings us to a critical juncture. With the rapid pace of AI evolution, what does the future hold for our Data Scientists? Through the lens of this article, I aim to offer my perspectives on how the roles and responsibilities of Data Scientists may evolve in the coming years. I invite you to join me as we explore this exciting future landscape, teeming with promises and opportunities.
Let’s take a moment to peel back the layers of the world of Data Science in an industry, which, at its heart, is dedicated to solving problems and driving tangible outcomes. If you’re peering into this world from the outside, you might imagine a Data Scientist’s day is filled with intellectual battles over complex problems, meditating over the merits of Data Science techniques, and crafting the perfect implementation tactics.
However, the reality can often be far less glamorous and somewhat surprising to those not entrenched in the field. The truth is that Data Scientists often find themselves more like explorers in a vast wilderness, dedicating substantial time to the arduous task of hunting, gathering, and refining the raw materials of their craft: the data itself. They then spend hours coding and troubleshooting to extract the insights before finally weaving those into stories with narratives that non-data savvy stakeholders can understand and act upon.
The advancements in AI might just be the game-changer we need to tackle these less-visible inefficiencies. They equip Data Scientists with powerful tools to harness their core competencies fully. They will have more time and focus on employing cutting-edge analytical methods to derive actionable insights and address real-world issues. This is the “future” many Data Scientists envisioned when starting their journeys, and we’re journeying back to that future now.
AI advancements are triggering significant productivity boosts and impact acceleration in Data Science. Let’s delve into a few recent AI-enabled innovations that illustrate this point.
Take, for instance, GitHub’s Copilot. This AI-powered
coding aide serves as a steadfast companion for every Data Scientist, offering instant code suggestions and considerably reducing their workload. Imagine the convenience of telling Copilot your coding objective in layman’s terms, and it responds with the necessary subroutines or functions. Of course, sanity checks remain crucial even when using AI. This isn’t science fiction - it’s the reality we are experiencing today. Several similar coding assistants are emerging, including DeepMind’s AlphaDev, which impressively identified sorting algorithms boasting a speed and scalability improvement of up to 20% compared to leading human-designed benchmarks. Such AI-enabled coding assistants empower our Data Scientists to dedicate more time to discovery and problem-solving, thus boosting their efficiency.
Let’s also consider the potential of a tool capable of swiftly skimming through lengthy reports or intricate technical documents, identifying key points to form hypotheses or spotlighting opportunities for system improvement. This is now feasible thanks to AI’s phenomenal prowess in summarising vast bodies of text. This area is rapidly growing, with paid and open-source options becoming available. Notable newcomers include Jasper (formerly Jarvis), a GPT-3 model-based tool adept at tackling generalised summarisation tasks. There’s also Scholarcy, tailored for academic use, including direct PDF ingestion capabilities. Scholarcy appears to operate based on a proprietary algorithm, albeit drawing inspiration from Google’s PageRank algorithm and ‘bottom-up attention’ research. While these tools may overlook nuances requiring deep domain knowledge, their abilities are continually improving. It’s just a matter of time before we have access to embedded summarisation tools for industrial settings capable of meeting requirements for IP capture and incorporating profound industrial knowledge. Such tools will assist Data Scientists in navigating information more promptly and efficiently.
AI’s transformative potential also impacts how insights are communicated and implemented. AIgenerated presentations and visuals enable Data Scientists to distill intricate insights into digestible narratives. For instance, Beautiful.ai provides a userfriendly platform for creating vibrant presentations, eliminating the need for meticulous crafting in PowerPoint. Another example is SlidesAI.io, integrated
Companies are in full sprint, vying to stay ahead of the technological curve. In the scramble to adapt, however, there’s a blind spot emerging: the crucial element of human potential seems to be getting sidelined.
into the Google Docs ecosystem, making visually appealing slides easy to create. Granted, these tools focus more on the aesthetic aspect than the content, but just think about the potential when you pair these capabilities with AI’s text summarisation prowess, as previously mentioned.
Imagine a scenario where Data Scientists can articulate their findings to business stakeholders using the specific lingo or style that encourages understanding, support, and rapid implementation. This approach will undoubtedly expedite the journey from insight discovery to solution implementation.
These increasingly advanced AI-enabled tools are becoming more sophisticated and more widely accessible, which is an exciting development. We’re seeing an array of AI-powered tools integrating seamlessly into familiar software like Microsoft’s Office Suite, which now includes built-in AI features. The open-source world is also teeming with
groundbreaking innovations, drawing inspiration mainly from Meta’s recently “leaked” LLM model, known as LLaMA.
Rumours are starting to circulate that Meta may be looking into offering commercial licenses, which could open the door for companies to integrate AI into their operations natively. This development is exhilarating and signals a future where cutting-edge AI technology is not solely within reach of tech giants but is a shared resource available to all.
Indeed, we are on the brink of a new era. AI is helping Data Scientists not only return to their original mission but it’s also helping them unlock new opportunities. Rather than being confined to the analytical sidelines, Data Scientists are now stepping into strategic roles, spearheading business decision-making processes. AI acts as their navigation system, guiding them through uncharted territories toward a future teeming with promise and potential.
As we navigate this AI revolution, the role of a Data Scientist is undeniably shifting. Elements that were always vital are now spotlighted, while others previously in the shadows are stepping into the limelight. Living in the heart of this transformation, I’d like to discuss the evolving requirements and personal attributes that can help Data Scientists thrive in this AI-empowered era.
Firstly, a broad understanding and expertise across various fields has become crucial to the role of the Data Scientist. It’s no longer enough to be proficient in just one area. Being limited to one domain could be the biggest hurdle moving forward. While industry-specific knowledge can be seen as a domain and holds significant importance for a Data Scientist (due to its role in setting constraints and charting practical implementations), I am explicitly highlighting traditional academic disciplines here. These include fields such as biology, physics, chemistry, etc., which extend beyond the core disciplines of Data Science; like statistics, mathematics, and computer science.
Let’s delve into a rather technical example within biology to demonstrate this point: consider the scenario of modelling gene functions. It becomes imperative to understand how genomic repeats factor into the model. When a DNA segment is repeated, it sometimes leads to null functions (often triggering gene silence, a
common organismal mechanism to combat viruses). At other times, it can enhance the function by duplicating essential genes, making the underlying function more robust and diverse. This intricate understanding plays a crucial role when it comes to accurate modelling. For instance, how much weight should we attribute to these repetitive observations in our model? How do we tune the hyperparameters within a deep neural network to capture these complexities? This example underscores the need for a profound understanding of the specific domain (in this case, biology) and Data Science techniques to effectively excel in our roles.
If my earlier points seemed obvious, I’d like to offer a deeper insight into the hidden significance of profound domain knowledge in the context of the AI revolution. As we delve into the world of Large Language Models (LLMs), a key objective is to align AI-generated recommendations or solutions with the benefits and intentions of human users. This is known as the alignment problem. While we can mitigate the alignment problem to an extent with human feedback and reinforced learning approaches, it doesn’t address the underlying issue: we often don’t understand how these recommendations are made and whether they could potentially lead to unforeseen and harmful outcomes. Using my earlier example of DNA duplication, what if an AI model considered all DNA duplications detrimental or useless? How could we be sure that the model wouldn’t
make incorrect recommendations based on this assumption?
I’m convinced that a profound understanding of the domain, combined with a thoughtful application of this knowledge when employing AI tools, arms us with the necessary tools to ensure our models are not only interpretable but also capable of making sound decisions. More importantly, they are aligned with our overarching goals. This focus on multidisciplinary expertise will transition from being a ‘nice-to-have’ attribute to an indispensable requirement in the age of AI, underscoring its transformative potential.
The next crucial quality for Data Scientists to hone is a humble readiness to embrace change. This trait complements the knowledge depth of domain experts, a group that includes many of our Data Scientists with advanced degrees. It’s natural to take pride in reaching the zenith of one’s field. However, this pride can sometimes evolve into arrogance, leading to skepticism when novel methods, understandings, or perspectives arise. Given the rapid advancements in AI, these moments of surprise and potential shifts are only set to increase.
I vividly remember my initial skepticism toward ChatGPT and its earlier versions when they first became accessible. My LinkedIn posts from that time reveal this skepticism as I dismissed it as mere “simple pattern recognition.” Only later did I realise I had underestimated its significant emergent abilities. I’ve since openly shared this learning journey. While I’ve never considered myself overly arrogant, this experience was humbling. New breakthroughs can seem like magic at first. Without a willingness to understand and adapt, these innovations will remain misunderstood — like magic, captivating but not taken seriously. This mindset ultimately holds us back, preventing us from realising the full potential of AI.
new field of knowledge or pursuing another degree? Remaining relevant requires an ongoing commitment to learn and adapt, and Data Scientists find themselves at the epicentre of these new demands. We stand as the orchestrators of this evolution, but we also risk being the most significantly impacted unless we welcome and adopt new mindsets.
An often-overlooked aspect in our field is the significance of Emotional Intelligence (otherwise known as emotional quotient or EQ), particularly in the industry setting. Historically, Data Science has been a highly technical domain where practitioners take pride in resolving complex and challenging problems. The spotlight has rarely been on the necessity of EQ for a Data Scientist, but this needs to shift. Although EQ is not a unique requirement for Data Scientists, it will become an essential prerequisite. This human-centred attribute possesses the greatest resilience against the disruptions brought on by the AI revolution.
EQ is of paramount importance in Data Science for several reasons. Firstly, EQ goes beyond understanding numbers and statistics; it involves grasping the human influences behind these figures. Take the stock market as a prime example. If you base your financial models solely on fundamentals such as profit margins and market share, you will likely underperform in the long run. Why? Because stock prices are primarily driven by human decisions, which can often be irrational. They are swayed by word of mouth, sentiment, or even simple human errors.
A striking instance of this occurred in January 2021 when investors mistakenly bought shares in Signal Advance, a small components manufacturer, which led to an over 5000% increase in its stock price at the time. These buyers were under the false impression that they were investing in Signal, the encrypted messaging service. This mix-up happened following a tweet by Elon Musk encouraging the use of Signal due to privacy concerns about WhatsApp, leading to significant confusion.
Following the path of continuous learning and openness to new ideas, we must also overcome our inherent resistance to adopting new methods and altering our established ways of working. Are we fully using AI-based coding assistants like GitHub’s Copilot in our day-to-day Data Science tasks? Have we integrated the innovative sorting algorithm discovered by AlphaDev into our pipelines? Have we considered delving into a
This event underscores the crucial role of EQ in a field like Data Science. To be truly effective, Data Scientists must work closely with others to identify information, devise solutions, and understand different perspectives. This requires not just technical skills but also the ability to read others’ thoughts and preferences and empathise with them. Developing EQ will lead to better decision-making and a more substantial business impact.
We are on the brink of a new era. AI is helping Data Scientists not only return to their original mission but it’s also helping them unlock new opportunities.
In conclusion, I often find myself addressing a recurring set of questions from my Data Science team“how can I get this person to listen to me?”, “where can I find this information?” or “why aren’t they responding to my emails?”. I wish I could offer a ground-breaking revelation as a solution, but the truth is much simpler. Data Science is more than science; technical expertise alone will never suffice, not just in our field but in any role in an increasingly interconnected and collaborative future. Creating and nurturing human connections is vital for our work, and it might be one of the few sanctuaries that allow us to flourish when Artificial General Intelligence (AGI) is fully realised.
As a Data Science lead and people manager at an agriculture company, I often recount one experience to my new hires. I take pride in the time I spent walking through corn fields alongside our commercial teams, breeders, and farmers. While some might wonder why I consider this experience so significant, and others may even think it’s pretentious, the truth is that it’s deeply intertwined with our work. It allows me to witness firsthand how decisions are made and how opinions form, but more importantly, it provides an opportunity to build trust and forge connections with key stakeholders. These relationships are the cornerstones that have defined the success of my career. Therefore, I encourage every Data Scientist to embrace the human factor, for these personal interactions genuinely make the difference.
The rise of AI is ushering Data Science back to its roots, empowering us to do what we are supposed to do, but with remarkable efficiency and precision. It is reshaping our careers in profound ways; we are evolving beyond just being number crunchers to become strategic thought leaders, facilitators of business decision-making, and navigators through unexplored
territories. Simultaneously, the AI revolution demands the development of new competencies. A multifaceted approach that combines interdisciplinary training with an empathetic understanding of human connections is now essential. It is as vital as mastering our mathematical and technical skills.
As we stand on the brink of this exciting new era, our vision only extends to the event horizon of Artificial General Intelligence (AGI). What lies beyond it - the Singularity - remains a mystery. It’s the equivalent of peering over the edge of a cliff, unsure of what’s underneath but filled with a sense of anticipation, maybe even fear of height.
Nevertheless, let’s approach this precipice with optimism and a willingness to adapt. Let’s harness the power of this AI revolution, guide it towards constructive paths, and increase our chances of creating a future that benefits all of humanity. The future of AI is, after all, a mirror reflecting our collective actions and decisions. Let’s ensure the image that emerges is one we can be proud of.
Based in the USA, LIN WANG is the Data Science Lead for Analytics for Bayer; a global company with core competencies in the life science fields of healthcare and agriculture.
The views and opinions expressed in this article are solely Lin’s and do not reflect his employer’s views, official policy, or position. The information presented is based on Lin’s personal research and understanding and should not be interpreted as definitive advice or recommendations.
Creating and nurturing human connections is vital for our work, and it might be one of the few sanctuaries that allow us to flourish when Artificial General Intelligence (AGI) is fully realised.
By now, we have all become accustomed to the idea that our capacity and ability to harness data and develop AI solutions have a profound impact on businesses and society alike. It is propelling us, at pace, into what is often described and considered to be a modern-day equivalent of an Industrial Revolution. And just as with the previous Industrial Revolution, it is generating positivity and fear.
For many years now businesses have been increasingly tapping into the power of data to derive valuable insights that are specific to their business needs. These insights have been revolutionary in transforming their decision-making, their processes and propelling them forward. The recent surge in the use of generative AI has further accelerated this trend. However, it is important to recognise that while AI excels at optimising output and generating insights, it falls short in explaining the underlying rationale or evaluating associated risks and assumptions. It is not uncommon to come across stories where AI
has been portrayed as a failure, often due to unrealistic expectations or its lack of training for a specific use case. Within the AI and Data industry, we need to collectively recognise the importance of explaining to users how AI generates its output and the level of certainty involved, we need to be building AI that delivers accessible and trusted insights. Currently, there appears to be a challenge among data leads, particularly those working with generative AI, in effectively communicating the inner workings of their AI models. Transforming raw data into trusted insights demands a strategic approach that surpasses the boundaries of conventional analytics.
Here we will explore some of the key aspects surrounding AI ethics and dive with a deeper focus into one of the less acknowledged elements of data ethics, sustainability, providing suggestions and tools to help you be part of the solution.
AI ethics is far more than control of personal information, or representation of at-risk groups. Though these are crucial, to truly establish trust in the AI solutions we create, we must adopt a broader perspective on AI ethics. Issues such as bias , discrimination , and privacy breaches have rightly gained significant attention in recent years. As data practitioners, it is our responsibility to address these ethical challenges headon and ensure that our models and algorithms are fair , transparent , and capable of being explained to the users of our solutions.
In addition to these concerns, we must also acknowledge the growing ethical threat posed by adversarial attacks. These attacks manipulate data to deceive AI systems, potentially compromising the accuracy and reliability of the insights generated. To address this challenge, it becomes imperative to develop robustness in our AI systems . We need to ensure that we have thoroughly tested and are confident our solutions are resilient to adversarial attacks, whilst also not prone to being over-trained. By doing so, we can maintain the integrity of organisational data and
safeguard against unauthorised modifications. We must also be cognizant of the future-proofs of our solutions as ensuring the accuracy of our AI solutions over time is an ethical focus. Many Data Scientists will have found themselves in a situation where they have built an ethical explainable model that they are rightly proud of, only to hand it over to a customer who does not have the capability and/ or capacity to maintain it effectively. The delivery of a data solution is not a one-time endeavour, most, (if not all) require ongoing maintenance and updates to remain effective and crucially to ensure they don’t drive misinformation. To address this ethical challenge, we need to prioritise the maintainability of our data solutions. This involves incorporating built-in continuous monitoring, evaluation, and mechanisms for identifying areas that require improvement or updates. By doing so, we collectively ensure that our data solutions remain adaptable to changing requirements and evolving data quality. By striving for maintainability , we can guarantee the longevity and long-term value of our data solutions. Sustainable practices are crucial in this endeavour, as we must be aware and vocal about the future impact of our AI solutions.
When we are designing ethical AI solutions and looking at that future impact, sustainability has to be a primary consideration. With more and more data being
generated, processed, stored and backed up, the way we consider and plan for this impact in our AI designs will be crucial for future generations.
As data practitioners, it is imperative for us to openly discuss and address the sustainability and environmental consequences of our AI solutions. The exponential growth of data storage and usage, especially with the rise of generative AI, poses a significant threat to global warming (Constance et al., 2021). The sheer volume of data worldwide is expanding rapidly, leading to a substantial increase in electricity consumption by data centres. Between 2005 and 2010, global electricity consumed by data centres surged by an alarming 56%, accounting for 1% of global electricity consumption in 2020. It is estimated that this trend will persist,
with data centres forecast to contribute around 13% of global energy consumption by 2030 (Huang et al., 2020, Haddad et al., 2014, Jouhara et al., 2014, Güğü et al., 2023). While the adoption of green energy by many data centres is commendable, sustainability in data extends much beyond the simple metric of how energy centres are powered, we must also consider the impacts from the extraction of precious metals in hardware production, the land-footprint, water consumption and the environmental impact associated with constructing and operating the growing volume of data centres.
The exponential growth of data, data analytics and AI presents a large challenge to the CO2 generated and its storage and processing. Figure 1 shows the dramatic growth in the volume of data created, captured, copied, and consumed worldwide from 2010 to 2020, with forecasts from 2021 to 2025 in Zettabytes (Taylor, 2022).
As of today, a significant amount of data remains unused, contributing to negative environmental impacts and escalating storage costs. This surplus data, known as dark data, continues to accumulate, primarily due to the vast amount generated by the Internet of Things and sensor devices. Astonishingly, it is estimated that up to 90% of data generated by IoT devices go unused (Gimpel and Alter, 2021). Moreover, a substantial portion of this data loses its value, up to 60%, within milliseconds of its generation (Corallo et al., 2021). If not managed effectively in the future, the worldwide CO2 emissions resulting from storing dark data could exceed 5.26 million tons per year (Kez et al., 2022). It’s worth noting that the associated CO2 emissions for this dark data are
again not the only environmental concern; for dark data alone, the estimated water used for data centre cooling and the land footprint are also significant factors, amounting to 41.65 billion litres and 59.45 square kilometres respectively.
Hao (2019) highlighted the environmental risk of CO2 emissions generated by the use of AI technologies. It has been estimated that energy use is split between 10% on training a model and 90% on serving it. This highlights that it is critical to consider the whole life cycle as a model when thinking about sustainability. A model may have a higher energy consumption during training, but it could reduce overall total carbon emissions if that model also cut serving energy by 20%
(Patterson et al., 2021). Patterson et al. (2021), estimated carbon emissions due to training GPT3 are 552 tCO2e, this would require 9,127 tree seedlings to be grown for 10 years to offset it (computed from Greenhouse Gas Equivalencies Calculator | US EPA). That’s just for getting the model trained, not served, and used by so many of us. By optimising energy consumption, reducing data redundancy, employing responsible data management practices, and being thoughtful when developing and maintaining models organisations can help reduce the environmental impact of data.
While in the UK there is no specific legislation on making AI sustainable, the Well-being of Future Generations (Wales) Act 2015, is a legislation enacted in Wales that aims to promote sustainable development and ensure the well-being of future generations. This applies to data-driven solutions. It emphasises the importance of sustainable practices and decisionmaking that balances the needs of the present without compromising the ability of future generations to meet their own needs. It serves as a framework for creating a sustainable and inclusive future for the country.
While acknowledging that data can contribute to environmental damage, it is crucial to recognise the significant positive role that data-driven insights can play in addressing sustainability challenges. At LDCO, we firmly believe that as data practitioners, we have the power to be part of the solution and we are vocal advocates for using data for good. By implementing sustainable practices across the entire data lifecycle, from collection, storage, processing and disposal, we can make a positive impact and contribute to a more sustainable future. By using the most efficient algorithms possible, minimising data collection, storing only the data we need, and using emerging technologies like Edge analytics, we can mitigate the environmental footprint of the projects we work on. Each of us has the potential to reduce the overall environmental impact of data storage and insights. Balancing the benefits of data-driven insights with responsible practices ensures that AI becomes part of the solution, not the problem. By sharing knowledge on methods for energy efficiency, establishing best practices, and promoting transparency in CO2 use, we can all harness the potential of data while minimising potential environmental impact. We can make this explainable by reporting the CO2 impact of training our models, and storing our data using tools like this one; carboncalculator.ldco.ai/home
We all have a role to play and a personal impact we can achieve by embracing responsible and ethical AI practices, prioritising maintainability, and integrating sustainability into our data strategies. By implementing robust frameworks for ethical AI development,
conducting regular audits to identify and mitigate biases, and fostering diversity and inclusion in our data teams, we can build AI systems that promote social good while upholding ethical and sustainable standards. One way we have been able to support this at LDCo is by developing an AI health check, we are excited about the good this does in as little as 10 days.
Constance Douwes, Philippe Esling, Jean-Pierre Briot. A multiobjective approach for sustainable generative audio models. 2021. (hal-03296897)
Corallo, et al. (2021). Understanding and defining dark data for the manufacturing industry. IEEE Trans. Eng. Manag. (2021), pp. 1-13
Gimpel, A. Alter. (2021). Benefit from the Internet of Things right now by accessing dark data. IT Professional, 23 (2) (2021), pp. 45-49
Güğül, Gül Nihal & Gökçül, Furkan & Eicker, Ursula, 2023. “Sustainability analysis of zero energy consumption data centres with free cooling, waste heat reuse and renewable energy systems: A feasibility study,” Energy, Elsevier, vol. 262(PB).
Hao, K. (2019, June 6). Training a single AI model can emit as much carbon as five cars in their lifetimes. MIT Technology Review. www.technologyreview.com/2019/06/06/239031/training-a-singleai-model-can-emit-as-much-carbon-as
Heidorn, Patrick. (2008). Shedding Light on the Dark Data in the Long Tail of Science . Library Trends. 57. 280-299. 10.1353/ lib.0.0036.
Huang, Pei & Copertaro, Benedetta & Zhang, Xingxing & Shen, Jingchun & Löfgren, Isabelle & Rönnelid, Mats & Fahlen, Jan & Andersson, Dan & Svanfeldt, Mikael, 2020. “A review of data centres as prosumers in district energy systems: Renewable energy integration and waste heat reuse for district heating,” Applied Energy , Elsevier, vol. 258(C).
Jouhara, Hussam & Meskimmon, Richard, 2014. “Heat pipe based thermal management systems for energy-efficient data centres,” Energy , Elsevier, vol. 77(C), pages 265-270.
Al Kez, D., Foley, A. M., Laverty, D., Del Rio, D. F., & Sovacool, B. (2022). Exploring the sustainability challenges facing digitalization and internet data centres. Journal of Cleaner Production , 371, 133633.
Maroua Haddad & Jean-Marc Nicod & Marie-Cécile Péra & Christophe Varnier, 2021. “Stand-alone renewable power system scheduling for a green data centre using integer linear programming,” Journal of Scheduling , Springer, vol. 24(5), pages 523-541, October.
Patterson, David, Joseph Gonzalez, Quoc Le, Chen Liang, LluisMiquel Munguia, Daniel Rothchild, David So, Maud Texier, and Jeff Dean. “ Carbon emissions and large neural network training .” arXiv preprint arXiv:2104.10350 (2021).
Taylor. (2022). The volume of data/information created, captured, copied, and consumed worldwide from 2010 to 2020, with forecasts from 2021 to 2025 , Statistics, Total data volume worldwide 20102025 | Statista
While acknowledging that data can contribute to environmental damage, it is crucial to recognise the significant positive role that data-driven insights can play in addressing sustainability challenges.
Can you give us an overview of what you see companies currently doing with Generative AI? Unless you’ve been living under a rock, generative AI has been massively transformational, and when we think about data and generative AI there haven’t been a lot of obvious use cases.
In terms of figuring out how AI will enter the data space, companies are working on a few different things. AI does extremely well in data in certain domains which are closed. For example, writing code using copilot or for content marketing generation.
Then there’s Text-to-SQL, where a bunch of different players are thinking about this notion of being able to ask any question of your data by using AI to generate the SQL needed to run that query. Personally, I’m not very bullish on that. When you think about answering a business question, this is extremely open-ended and I don’t think AI has proven to be very effective there yet.
But, what I am very bullish about is being able to plug in generated AI, or smaller expert language models
(ELM’s), or a private LLM on top of your semantic layer. Being able to push this on top of your metric definitions and then being able to ask very, very open-ended questions such as “what was revenue like last month?”, “where did it come from?”, “is this channel going up?”, “is this channel going down?”, “is this increase in revenue actually statistically significant?”, or “is it just a sort of one-time blip?”
I think using AI on top of the semantic layer is the most exciting application of AI in the data space today.
Can you define what you mean by the semantic layer?
The semantic layer has been around forever but at a very high level. You have raw data coming into your data warehouse or your data storage layer, and this data is messy and unstructured. It’s typically not built in a way to answer business questions.
inside your BI tools, then every time you want to do Data Science, or every time you want to push this data back inside your production systems, you’re going to have to duplicate this logic because they only exist inside reporting.
Recently, we have started to pull the metrics layer out from the BI tools to become a stand-alone layer. Now you have BBT, one of the most famous modelling tools. We have many companies, such as Cube and Transform, which have built semantic layers. However, metrics in the semantic layer have become an individual layer and BI is one of the consumers of the semantic layer. What becomes really interesting as we think about the future, is being able to plug-in an LLM, or an ELM, on top of your semantic layer and use more intuitive interfaces such as Slack.
To model this layer, we clean it, join it, and reframe it in a way which makes sense for the business. The semantic layer is essentially just a definition layer. It allows us to interpret what that data is. The semantic layer would contain what your definitions are. These could be, for example, your definitions of revenue, or what you call an active user, or revenue from a particular channel. It allows us to figure out how to compute one sort of business metric in a way which becomes very consistent.
If anyone wants to compute a metric, they refer to the semantic layer. The semantic layer will give us the definition of that metric from a perspective of SQL or from a coding perspective. We can then have all the consumers who want to consume what that metric is, and instead of reading it directly from the raw data, they would read it from the semantic layer. This means that everyone would have the same definition of that metric, and if you ever wanted to change that, you would just change it in one place and it would propagate into all of your different consumers.
One of the questions I’m thinking about is, “are we approaching the end of BI tools and are they going to be replaced by having an ELM or an LLM on top of your semantic layer” because that’s a better interface? We’re starting to see a few players doing this, and new startups like Delphi Labs are building special products on top of a semantic layer.
The idea is that the end user directly leverages an LLM to get around having to engage with a Data Science department. They can get reports, visualisations or insights from the data on demand when they need it through the LLM connecting directly to the semantic layer and the source data.
The reason you are building a BI dashboard is to answer questions, and that just means that you need to know what those questions are. However, gaining access to information leads to asking better questions. For instance, I get some information, I think about it, and then because of that, I now have a new question. And when I have a new question, I don’t want to go to a data team and say, “Great, I have a new question now.” The issue with putting your LLMs or your ELMs on top of your raw data, is that you aren’t sure if the ELM or the LLM has just made up a business definition. It could be just hallucinating, which is why I like having it on the semantic layer so you can be assured that whenever it computes it, it’s computed in the right way.
stepping into this?
Many years ago, the semantic layer was really part of business intelligence. It was part of what we call ‘BI tools’ or ‘reporting tools’, including the likes of Tableau, and more recently, Looker and Open Source ones like Preset and Light Dash and Sigma. Your metrics used to exist inside your BI tool.
In the last few years, we realised that if they exist
I can now be very specific. I’m not just asking what the revenue was last month, and what was the revenue by channel - but if I see some channels going up and down, I can see if this is significant. Has this been happening periodically? What percentage of that revenue went up from existing customers or new customers? Or what percentage of that went up from existing customers in this new channel, compared to how much money we spend on this channel? Is this channel worth it or am I better off using another
I think using AI on top of the semantic layer is the most exciting application of AI in the data space today.
channel? These are highly contextual questions which I’m just combining, and that is just not possible in the old paradigm of building reporting inside BI tools.
Are there any specific changes you see happening to semantic layers to get them fit for LLMs?
No. I think at a high level, it’s probably best to pull the semantic layer out from BI, and have it as a standalone layer. It’s not complicated to go and plug in an ELM or an LLM on top - which is where two things come to mind.
Firstly, we are very early in the adoption lifecycle of the semantic layer. DBT Labs launched their semantic layer last year and that was a big failure. They then acquired Transform, and in some ways they’re deprecating the semantic layer and replacing it with Transform. So, one of the biggest companies behind data models got it wrong, and because of that we’ve seen companies like Cube and others launch a semantic layer.
But - if I am able to deploy this layer and I do it right, I can truly build an organisation whereby anyone in the company can ask any question, which is really exciting.
Secondly, we are not talking about using a public version of an LLM (such as using ChatGPT and connecting that to your semantic layer, which is probably not a good idea). There is now a version of ChatGPT which can be deployed for your company, or you can use like an open source ELM. The main difference in ELMs and LLMs, is that ELMs are built on top of your data sets. They’re Open Source, and they’re
more focused on your business. Whereas a large scale language model has got a lot more information. I think either can work, but you should use a private version of LLM or your own open source ELM.
I think that what BI tools have done really well for many years is allowing you to control the data. An example of this would be a sales manager of a store only seeing data for their store, but their manager can see data for a number of stores, and then their manager can see it for the city and for the state. We can then filter all of these things inside a BI tool by having different layers of access control.
So, how do we do this with semantic layers? There are a few different ways. Even though semantic layers are pretty new, they are starting to build out role-based access controls and who accesses different information. Another question is, do you want this in the semantic layer for enterprise-wide adoption, or do you want it inside your AI layer? These are some open questions and I don’t think any of these are really hard problems to solve. But, because we are in the infancy stage, best practice examples of how to do this haven’t yet been clearly defined.
What is required to connect an LLM to the semantic layer to make sure it can navigate the data in a robust way?
I think the first version of this is going to be to export data to a Google sheet, and then put this inside your
own private LLM and ask questions on top of that. The issue is again, you don’t have a semantic layer on top. There’s always some fear that it’s hallucinating a definition of your metrics. I wouldn’t recommend going down that route as you want to have it plugged on top of your semantic layer.
Now, you may have the expertise where you can use these APIs to actually go and connect this on top of your semantic layer. As I mentioned, there are some companies that are doing this, Delphi Labs being an example. You can sign up for their service online and just connect your slack on top of your semantic layer, and then you can start asking questions. We’ve seen this inside content marketing, and in writing code with Copilot. There are more and more of these kinds of vertical applications built on top, and I think the next tier of these will be applications like Delphi, which are able to connect your semantic layer through a very easy intuitive interface - whether it’s Slack or whether it’s text-to-speech.
Do you see a clear path for the connection between the LLM and semantic layer in the complex enterprise setting?
I think it’s a really interesting question. The semantic layer implies that you’re talking about a very focused set of questions, which makes sense for your business. The revenue of my business has got nothing to do with the many other things that an LLM knows. Other typical applications, such as generating content, requires a lot of training of the LLM. I think the problem of answering questions, which are very specific to my business, is a much easier domain for generative AI to do, which is why I’m also bullish about actually deploying your own open-source ELM. If you had the technology to connect this on top of the semantic layer, it would still be powerful even without the hundreds of thousands of hours required to train LLMs.
Can you talk a little bit about the advantages and disadvantages of ELMs versus LLMs?
Recently, there was a famous memo leaked from Google, stating they don’t think that they, or OpenAI, had any long-term, sustainable competitive advantage versus the open-source LLM world. I think this goes back to that bigger question around LLMs that require a massive amount of data to train, versus training a smaller ELM on top of your own company’s data sets. The question is, how close can these ELMs (especially for highly contextual use cases), get to an LLM, and how much work is involved in deploying these open-source models to the Last Mile.
It’s easy to go and deploy the model, but the Last Mile means you have to start training it and giving it
access to your data, and actually being able to get value from it. On the infrastructure side, there’ll be plenty of companies which are thinking about Last Mile. It’s very difficult for a business today to go and build an AWS, or to go and build a GPT3 because you need a lot of application development. But if it’s not very difficult for a business to deploy their own ELM which they fully control, compared to even a private version of ChatGPT, then it means that you can deploy this very cheaply. We will find out whether this goes one way or the other in the next six to 12 months, but I can see a world where these big companies do not have as big a lead as they thought, and that ELMs become just as powerful.
I think that 99% of companies will be consumers of AI, and 1% of companies will actually create AI. Everyone is now AI-driven, but only a few percentage of companies will actually go and build the infrastructure for it and the majority of companies will just use it. If you build it, obviously you have a much more competitive advantage. But having said that, around five years ago we saw where every single e-commerce company wanted to build their own recommendation engine, and the reality is that 99% of these companies should not be building their own recommendations engine, but they should be using pre-existing tools.
Everyone is now AI-driven, but only a few percentage of companies will actually go and build the infrastructure for it and the majority of companies will just use it.
I see that same analogy inside ELMs versus LLMs. We’re still very early, right? We haven’t yet defined who the category winners are in many of these areas. If you are able to do this yourself, you have more flexibility on what this looks like, and you can customise it in a way which makes sense for your business.
It’s not very clear that an LLM is a hundred times better than an ELM. It’s just too early to actually see what happens.
How does a company get started with deploying the semantic layer and what advice have you got for them?
I think Cube is our favourite semantic layer right now. It’s actually based on LookML, the semantic layer for Looker. It’s an open-source project, as is the DBT Labs-acquired Transform. Both of these are really good if you want a deployed version. I don’t think you can use a managed version of Transform at the moment, although it will be available later this year. If you need more expertise, then at 5X, we can assemble the entire data layer for you. This is something we could help out with as well.
A city of rich history and cultural heritage, Warsaw is strategically placed to build on its emergence as a European hotspot for thriving innovation and Data Science.
The capital and largest city in Poland (with a population of over 1.7 million people), Warsaw is located in the heart of the country and is a city with a rich cultural heritage and a thriving modern economy. Warsaw has a long and fascinating history, dating back over 1,000 years and has been the capital city of Poland since 1596.
Warsaw has been through many difficult times over the centuries, including the devastation of World War II. During the war, over 800,000 of its residents were killed, however the city emerged stronger and more resilient than ever and was rebuilt in the decades that followed, and today it is a thriving, modern metropolis - perfectly located for a thriving Data Science hub.
The city has a rich cultural heritage, with The Royal Castle one of the most famous landmarks in the city, whilst The Warsaw Uprising Museum is a must-visit attraction for anyone interested in the city’s history.
Warsaw is also home to many art galleries and museums, including the National Museum, the Museum of Modern Art, and the Zachęta National Gallery of Art. The city hosts many cultural events throughout the year, including the Warsaw Film Festival, the Warsaw Book Fair, and the Warsaw Autumn International Festival of Contemporary Music.
Warsaw is a city that is constantly evolving and innovating, with a thriving modern economy driven by technology and innovation. The city is home to many start-ups and tech companies and has a growing reputation as a hub for innovation and entrepreneurship.
Warsaw is also home to many companies that specialise in artificial intelligence, including deepsense. ai, which is a leading AI company that focuses on machine learning and big data analytics. Other notable AI companies in Warsaw include Brainly, an online education platform that uses AI to help students learn more effectively, and Infermedica, a healthcare AI company driving machine learning to help diagnose and treat diseases.
Many large international and national corporations have also chosen to establish offices in the city. These include: Goldman Sachs, Procter and Gamble, GSK, Citi, Mars, Allegro, PKN Orlen, PGE Group, PKO Bank Polski, PZU, and LOT Polish Airlines.
For Data Science, one of the most significant hubs in Warsaw is the Warsaw Data Science Center. This centre is a joint initiative between the University of Warsaw and the Polish Academy of Sciences and offers a range of Data Science courses and workshops. It’s also a noted research centre focused on developing new technologies. Another important Data Science hub is the Warsaw Technology Park, which houses several companies that are focused on
1. Warsaw boasts the tallest building in the EU. Standing at 310m tall, Varso Tower is an office tower in Centrum.
2. Warsaw is known as the “Phoenix City” due to the number of times it has been destroyed and risen again.
3. Warsaw is home to the narrowest house in the world. Keret House is a two-storey building - at its slimmest, it measures just 92 cm (36 inches) and at its widest, it is only 152 cm (59 inches).
4. There are officially 82 parks in the capital city of Poland, and green space covers over 25% of the city.
5. The Old Town ‘Square’ is actually a rectangle. Traditionally, Old Town squares in Poland should have been squares with the exact same dimensions on each side. Warsaw breaks the rule, as the square is 90 metres by 73 metres.
6. Warsaw is the birthplace of Marie Curie who achieved international recognition for her research on radioactivity and was the first female recipient of the Nobel Prize.
7. About 85% of the city was destroyed during the Second World War.
developing new technologies. The Park also offers a range of services for startups and entrepreneurs.
Warsaw is quickly becoming a hub for Data Science, AI, and technology in Europe. With more and more major companies investing in the field and moving into the city, and a growing number of Data Science and tech hubs located throughout the city, Warsaw is poised to become a leader in the industry.
Warsaw is home to a number of world-class universities, including the University of Warsaw, which is one of the largest and most respected in Europe. The university’s Faculty of Mathematics, Informatics, and Mechanics is world famous, with many world-class researchers and scientists based there. The university runs Data Sciencefocused degree courses such as Bachelor’s programmes in Applied Data Science, and Master’s programmes in Data Science, Big Data Analytics, Machine Learning, and Artificial Intelligence and Data Analytics.
The Warsaw University of Technology is another major university in the city, with a strong focus on engineering and technology. The university is home to many research centres and institutes, including the Institute of Computer Science and the Institute of Control and Computation Engineering. Courses include Bachelor’s programmes in Data Science and Business Analytics plus Master’s programmes in Data Science for Society and Business and Artificial Intelligence in Business Intelligence.
Kozminski University is a private, non-profit business school in Warsaw, and is rated by the Financial Times as “Poland’s highest rated private university”. It offers the likes of a Bachelor’s programme in Data Science and
DSW is the largest data science community in Poland. Based in Warsaw, organisers Dominik Batorski and the Academic Partners Foundation schedule large meet ups of around 250 people. The community an informal, non-profit community working to exchange ideas and knowledge about Data Science, data engineering and artificial intelligence. DSW discusses tools, technologies and business opportunities related to data collection, processing and visualisation, as well as machine learning and deep learning.
Business Analytics, and Master’s programmes in Data Science in Business and Business Analytics and Big Data. Lazarski University and SGGW Warsaw University of Life Sciences also cater for those seeking Data Science qualifications.
Overall, Warsaw’s universities offer a range of comprehensive and industry-relevant Data Science programmes to prepare students for successful careers in this rapidly growing field.
In November 2022, ChatGPT was released and made available to the public, marking a significant milestone in the world of artificial intelligence. Within a mere five days, the model had already amassed one million users, and within two months, it had reached an impressive 100 million users. This unprecedented level of success has positioned ChatGPT as one of the most successful products in history, catching many of us by surprise with its sudden rise to prominence.
Although ChatGPT has garnered significant attention, it is essential to note that it is merely a product that utilises Large Language Models (LLMs) technology. The rapid evolution of LLMs in recent years has undoubtedly contributed to the current revolution in the field. OpenAI, the company that owns ChatGPT, released the first LLM in 2016 and 2018. Other significant tech players, including Google, Microsoft, Meta, AWS -and more recently Hugging Face- have been announcing new research breakthroughs and releasing new LLM models to the world.
According to Sam Altman, the CEO of OpenAI, the usability of ChatGPT is what sets it apart from other models. While GPT-1, Transformers, and LaMDA were relatively unknown to the general public, ChatGPT’s accessibility has marked a new era in the field. ChatGPT is an excellent representation of the direction that AI is likely to take, with an emphasis on making it accessible and useful to anyone, regardless of technical expertise. OpenAI’s success in creating the most successful AI product ever by making it user-friendly and practical for the general public serves as an example and inspiration for other companies in different fields.
The rate at which technology is evolving can be overwhelming. In such a fast-paced environment, it is understandable that many companies may be struggling with how to leverage the full potential of large language models, where to invest the time and effort and stay ahead of the curve in this ever-evolving field.
There are various options available. Commercial tools such as the ones provided by OpenAI-now also available via Azure, Github Copilot, or Amazon Code Whispererare one such option. However, it remains unclear whether these tools are the ideal and only solution, or if developing our own models and utilising opensource options is a feasible alternative. Some of the questions that persist include data confidentiality, cost, responsibility, and provider-dependency.
Data confidentiality is a crucial issue. It is then important to consider whether the services being used share fine tuned models with other customers or use data to improve their own models. While some services claim not to do so, there are others that clearly state that data might be used for further product development. For instance, OpenAI’s initial free subscription included a disclaimer that user data could be used to improve existing models or answer other users. This has resulted in several instances where proprietary data, such as code, has been made available
to everyone. OpenAI has since introduced the ability to turn off chat history which, according to them, prevents your conversation from being used for training their own models. In regards to privacy, hosting your own models in private servers brings the advantage that inferences can run locally, without the need for sending out your data to external services.
Regarding costs , it is essential to evaluate how much and in which context the company will use the technology. Software services, such as the ones provided by Microsoft or AWS, should be compared against deploying open-source models; which require paying for the underlying infrastructure. Such a comparison needs to be continuously assessed, given that both sides (paid services and open-source alternatives) are in continuous change. As an example, OpenAI switched from a free service to a paid subscription, and later announced significant price reduction, in just a few months.
Another crucial issue is responsibility for biased and wrong information. As deep learning models are still black boxes, they can learn incorrect and biased concepts from uncurated datasets, making them prone to hallucination and providing misleading information. Companies that integrate such models into their products or use them in development should be aware of who is responsible for such outputs and their consequent outcomes. A good practice is to start with use cases where we can directly evaluate the output of the model and easily identify any errors.
Lastly, provider-dependency is a consideration that needs to be addressed. Being agnostic is often a wise choice in technology, thus the same applies to LLMs. Relying on one product makes companies dependent on the model’s performance and prone to external service outages with little control. Running local models adds complexity, but it makes companies more agnostic, as such models can be deployed in any cloud provider.
Given the fast-paced nature of the technological landscape, policies and costs are continually changing. It takes time for companies to evaluate and mitigate risks, make decisions, and close contracts with service providers. That’s why, at Continental, we like to explore several alternatives: paid services and in-house model development. This approach allows us to move quickly and learn together.
In such a large organisation, with around 200,000 employees in 57 countries, there is a wealth of curiosity and expertise about large language models (LLMs). To share this knowledge, we have created an internal opensource initiative to combine efforts in learning about
exploring, and deploying, open-source LLMs. We are also interested in fine-tuning these models to meet our specific needs.
We were able to quickly set up the initiative thanks to the existing toolset that our employees are familiar with: our own platform for open-source projects, tools for code versioning and collaboration, and communication.
As an example, we made use of our private social network to communicate about the initiative, which helped us to quickly grow a community of over 100 people in just a few days and almost 500 people in two months. People are invited to share the latest news and models on generative AI, but mostly to exchange their ideas and on-going user experience with use cases.
The initiative has been a great success so far. We have gained practical knowledge about the potential and limitations of LLMs and made significant progress towards deploying an internal GPT-like model. We are now able to fairly compare such an approach with the available services out there, both in terms of cost and performance. This makes us more knowledgeable, more responsible, and mindful as an organisation.
Once again, this was unlocked by the existing technology stack and in-house AI expertise. This means expertise from hundreds of experts in data science and AI, as well as the required tools ready to use for model
training and evaluation, data versioning, experiment tracking and model comparison, deployment of models in several cloud providers, among others.
After the first deployment was made available for a small group of people, the overall interest started to increase as well as the exchange and learning experience from using it. The first challenge was clear: scaling the application would be required to make it available to several users simultaneously without hurting the user experience. We worked with our software architecture experts to build a scalable and elastic solution. This experiment allowed us to measure costs and usage, and to study what setup really makes sense for each use case.
The second challenge is data privacy . As in any big organisation, there is some data that only certain teams can access. This realisation led us to the key idea of having separate sessions, models and knowledge bases for different users.
Naturally, new requests for new features came from the community. The most popular ones are Question & Answers (Q&A) for documents and databases, content generation, and coding assistance. In parallel, it’s important to work together with legal, compliance and cybersecurity teams, among others. We must also be mindful and discuss the expected impact of this and similar tools in the workplace.
I’m struggling to defeat this AI-powered Death Star. My team of Data Science rebels needs to work faster and achieve more. How can we finish our Rebel AI Interceptor in time? Any advice, YodaGPT?
Luke, in troubled times you find yourself. Strong, your Data Science rebels are, but in the right formation they are not.
The DST Profiler you must use, a creation of Data Science Talent it is.
Understand the unique strengths of each member, it will. In positions where they truly excel, it will guide them. Enhanced productivity, harmony, and innovation it will bring. More successful projects they will deliver, and the AI-enabled Death Star you will defeat.
Hold on, Yoda. Are you suggesting recruiters created a tool that can help me lead better?
Doubtful, you sound, young AI-Walker. Much more than mere recruiters, they are. To craft this powerful tool from ancient days, a Senior Data Scientist and two engineers they engaged. A beacon of knowledge, this Data Scientist is, with a PhD in statistics and two decades of wisdom. Over 250 Data Scientists he has assessed, in roles of leadership. Underestimate them, you must not.
Alright, I’m intrigued. How does this help us outsmart the Death Star?
Questions, you have. Answers, I will provide… Each team member’s profile, a beacon of insight it is, showing their unique strengths and talents. The dashboards, like the Force, bestow you with knowledge and its visualisations immediately reveal the path. Guide you to intelligent decisions about which missions to undertake and with whom, it will.
The dashboard possesses a potent power - the team profile function. A group, when chosen, reveals its synergy. You’ll discern if together they can stand against the darkness of the Death Star, or if other alignments are needed.
Trust in the DST Profiler, you must. Help you optimise your current team, it will. Time is of the essence, young AI-Walker.
May the data be with you...
ANDREU MORA IS SVP OF ENGINEERING AT ADYEN. HE IS RESPONSIBLE FOR DATA (PLATFORM, ML, AI, EXPERIMENTS AND ANALYTICS) AND WAS PREVIOUSLY VP OF ENGINEERING FOR DATA SCIENCE AND ML.
ANDREU HAS ALSO HELD ROLES AS TECH LEAD, DATA SCIENTIST, AND ENGINEER WHERE HE WORKED ON PRODUCTS RELATING TO NETWORKBASED PATTERN RECOGNITION AND SCALABLE TIME SERIES
FORECASTING.
Bef ore Adyen, Andreu worked for the European Space Agency and private aerospace companies in the area of mission performance algorithms and mission design. Andreu holds an MSc. in Telecommunication Engineering from Universitat Politecnica de Catalunya.
My wife and I are quite different in our shopping habits. She likes analysing the market trends and she’s good at deciding what she likes. She’s even better at deciding whether to buy or not. Disclaimer: usually she does buy it, and then our entrance at home looks like a package pickup point (not true, but also not entirely not-true).
I, however, have a different poison. When I’m looking for something (say a new set of headphones), I analyse and analyse again, and at some point I conclude that a certain product is probably the best fit for what I am looking for. Then, again, I check the distance for the second choice. After that, I might end up going back to the fundamental question of whether I actually needed it in the first place.
I am quite frugal, so if I am not very enthusiastic about the top choice I will probably end up discarding it and bloating those non-conversion metrics for the A/B testing behind the e-commerce site (leaving the team scratching their head about what’s not working).
In my defence, I will say that if I am sure I need something and there is a clear market winner, I buy immediately and I do not think about it anymore.
The point is, choosing what to buy and when to buy it is quite transcendental. Choosing your tech stack is a similar problem. You need to understand and reflect very well on a number of things:
● Whether you actually need it - or is it just a fun, exciting, but limited-value exercise (hello ChatGPT demos)?
● How do you sweep and track the market and assess which tool or framework to adopt?
● Should you build or buy?
● How fast do you need it and whether you are sacrificing something instead?
Without thorough consideration of the above, a leadership team (often proud of their choice and/or invested in another way) may spiral the team downwards in terms of productivity and motivation. That’s why it’s important to choose your tech stack with the right amount of love, and eventually make a well-informed choice together with the technical experts.
It is remarkably difficult if you end up in a situation where there’s a clear need for something better than what you have now, but there is no industry standard or a clear choice. That’s where my shopper-persona would collapse. The feature store, or should I say platform (will come onto that later), has been a primary example of this sort of conundrum.
At Adyen we have gone through this exercise a number of times, and in some cases we’ve learned the hard way in how to go about these choices. It really boils down to two things that we embrace in our ethos: iterating and control.
Let me use an example of a use case for a feature store at Adyen. Every payment that goes through Adyen - and we do a lot of those ($860 billion in 2022) - undertakes a journey. This is where a number of decisions are made through an inference service, fueled by a machine learning model that we have trained and deployed. We have a few instances of those services with different purposes: our risk system (is the transaction fraud or legit), our authentication service (should we authenticate the user, and if so in which way), our routing algorithm, our transaction optimiser, our retry logic and others.
We are talking about a service that can take several thousand requests per second per model, and respond in less than 100 milliseconds. The final goal of all these models is to land as many good transactions as possible in the most efficient way, without ending up in a chargeback, a retry, or higher costs.
These models need features. They need features both at training time and at scoring time.
Let’s zoom in on the risk system. The service was initially built through rules across three different data sources:
● Block/allow look-up-tables, powered by PostgreSQL.
● Velocity database, powered by PostgreSQL, and able to provide information such as how many times has this card been used in this last minute.
● Our “shopper” database, also powered by PostgreSQL.
The ‘shopper’ database deserves its own paragraph. We have used, and maybe even abused, PostgreSQL in a very beautiful way; to identify shoppers in real time. This system ultimately provides an elegant and simple graph algorithm by identifying communities of attributes (such as cards and emails) that relate to the same person. It does that very efficiently and very quickly, but it has its own complications around flexibility and scalability. My colleague Burak wrote a great article about it. [1]
When we introduced a new approach based on a supervised classifier, we thought “hey, let’s include features about the merchant, that’ll boost AUCPR”.
To note, a merchant in fintech jargon is a seller or a company such as Uber or Spotify. In this instance we’d include (as a feature), datapoints such as the size of the merchant, the country of the merchant, how long have we been processing for this merchant, the authorisation
rate of that merchant across different sliding windows and so on.
However, many of these example features are slowmoving, medium cardinality and have high data volume. PostgreSQL wasn’t going to cut it in terms of crunching all that volume.
We added a new database called ‘feature store’ (read that as Dr. Evil with his famous quotation mark hand gesture).
We took advantage of the fact that we were sending all our transaction events to our Big Data Platform via Kafka. We collected all of this info in the form of Hive tables and then we use Spark to crunch the information;
all beautifully orchestrated through Airflow. We had all of this information and tooling available in our Big Data Platform because that’s where we train our models. We just need to use an abstraction layer to define the features (we chose Feast) and then deploy on another posgresql instance in the real-time flow. We love PostgreSQL, in case you haven’t figured this out yet. The ML artifacts are deployed to our real-time platform through our wrapper around MLflow, called Alfred, that allows us to stage their rollout into ghost, test, canary live settings and default live modes.
The final picture from our first crack at a ‘feature store’ looks like this.
Hidden in all of this is something that might have passed unnoticed. The redux of a very important lesson that we have learned through the years: our build-vs-buy trade-off.
There are great vendors that promise and deliver a seamless turn-key experience that’s fast and just works. It’s a very amenable choice, and I can only show my honest respect for these startups. They are like rain in the middle of a drought for a lot of companies that want to instantly get in the gig of feature stores, MLOps, Experimentation, Data Governance and any other sweet problem to solve. And they do a good job at it.
At Adyen, we like to stay in control and understand what happens under the hood. We also have a very solid principle - we won’t use a vendor for anything touching our core business. In this case, processing a payment is indeed core business and we don’t want to introduce a dependency on a third-party. That has two important
implications that are worth calling out:
● Firstly, we do use vendors, but we are critical about which part of the system they impact. On the one hand we buy our laptops from a vendor - it wouldn’t be optimal if we had a team building laptops. On the other hand, because we are set in building for the long term, we believe in controlling all our supply-chain (take SpaceX, who procure their own screws).
● Secondly, If we don’t use vendors, then do we build in-house? We have made that choice in the past, and now in perspective, it was a mistake. Picture a top-performing engineer, machete in their teeth, mumbling a classic “hold my beer”, and then proceeding to build, from scratch, something that already exists because “it will only take me a week to do it better”.
● We have been there and after the first month, I can guarantee the fun is over. Looking ahead at the feature parity roadmap leaves you in despair; the operational debt and preventable bugs itch you more than usual, and you end up going to bed every night thinking “why did we do this?”.
I already gave away one part of our ethos, which is based on strong control of our dependencies, fueled by longterm thinking. A second big trait of our way of thinking is the iteration culture.
At some point building software, and even hardware, someone figured out that working in waterfall contracts doesn’t really help. Instead, working in an agile way gets you further and faster, and it’s also more fun. The point of agile is not to adopt scrum. The point of agile is to embrace that the MVP is minimal (and therefore rusty and barely presentable) and also viable (it works, it’s not a WIP commit), and from there onwards, you have to quickly iterate.
We took a cold look at what we had proudly built as the feature store, tried to remove any emotional attachments to it, and ended up concluding that it wasn’t actually great. We also concluded that we might want to do some soul-searching and write a requirement list about what we want the whole thing to do.
We ended up with a letter to Santa detailing everything we wanted. At least from there, we could make a conscious choice about what we will not get given the cost and possibilities:
● Feature parity: the features and values on the training and scoring flows must be identical.
● Retrieval latency: we need the inference service to work under 100 ms.
● Recency: the features should not be old and we should be able to refresh them quickly.
Based on this, we also saw the need to have a system that spans two different platforms; our real-time platform (where payments, KYC, payouts, refunds and financial interactions happen with the world), and our big data platform (where we crunch the data). That’s not a surprise given that you have a need in two very different flows - your inference flow in real-time and your training flow off-line.
We needed an abstraction layer that would glue both systems together, so we chose to keep on using Feast, the open source package that allows Data Scientists
So what’s the answer? Well, open source. We use open source as much as possible to build our infra and rails, and then we build our core business on top. We also contribute merging PRs and adding new features that we found useful. At the end of the day, the internet runs on open source.
● Cardinality: we want to be able to store billions of features.
● Distributed: we need instances of the feature store around the world, because we process globally.
● Storage /scalability: our transaction volume grows quite a lot every year, and we build for two.
● Availability/uptime: we need the system to be there 99.9% of the time.
● Self-service: ideally we want Data Scientists to help themselves when prototyping and deploying new features.
● Complex calculation: some of these features can be complex to compute, which should be accepted.
● Feature diversity: it’d be great if we didn’t have to maintain three different databases and we just had one endpoint with all sorts of data inside.
After seeing that list, we thought “wow, it’s a long list”, but we also figured out there was an underlying difference between a pure storage place and a place where things are computed. That’s where we read Chip Huygen’s fantastic article on Feature Platforms. It was one of those ‘a-ha’ moments, when you can confidently say out loud that “we were building a feature platform, not a feature store”, and you can hear the non-existent triumphant music behind you.
The main difference lies in facilitating the computing of features, apart from the storing and serving which is captured under the definition of the feature store.
and Engineers to uniquely define features and ensure consistency across the two environments. We also evaluated LinkedIn’s Feather, but deemed it too opinionated and opted for the openness of Feast.
The general idea behind synching happening on two environments is that some features will be computed on the real time flow and stored on hot storage and synced back to the cold storage (big data platform). The slowmoving features will then be computed on the big data platform, stored there (cold storage) and synced to the hot storage for inference.
While the batch computation engine and storage were already there in Spark and Hive/Spark/Delta, we still had a few choices to make regarding the online flow. For stream computing, we use Apache Flink – but we can define that later. For the storage layer we hit a dilemma across a few contenders: Redis, Cassandra, Cockroach and sweet old PostgreSQL.
Here is where I will circle back to where I started. You need to make good decisions, and that probably means you need to involve technical experts. Even then, you
also want to be able to tap into the wider organisation to make sure that you are not biased or forgetting anything. That’s why we have a TechRadar procedure where engineers can share ideas for technology contenders, spar, benchmark and eventually decide on which to adopt.
We decided on Cassandra. Redis’ in-memory storage makes it quite expensive at the cardinality we are looking for; Cockroach is really keen on readwrite consistency at the expense of speed; and well, PostgreSQL didn’t cut it for our needs.
We are still evaluating choices for online computing engines (as said, Flink looks good) and feature monitoring where it might be that we just use the monitoring stack available. This largely consists of Prometheus, Elastic and Grafana.
That’s an honest look at where we are today. We are making an informed choice and not shooting from the hip. We have determined what we need, and we have also determined what is important to us and what we
are willing to pay for. We have analysed the market and open-source offering and are back to our beloved execution mode.
Even if there’s no clear and obvious choice, my shopper persona is still happily going through this procurement journey and enjoying the benefits of learning, discovering the possibilities and deciding. Because if we don’t get it right at first, we will build, fail, learn and iterate.
In the 1920s, John Wanamaker is famously quoted for stating that “half the money I spend on advertising is wasted; the trouble is I don’t know which half”. More recently in 2013, Martin Sorrel (previous CEO of WPP –the largest ad agency holding company in the UK), stated that his clients are wasting 15-25% of their advertising budgets. He just doesn’t know which 15-25%. Without knowing what works and what doesn’t, how can we optimise our spending and get the most out of every pound, euro, or dollar? Not knowing can lead to wasted advertising spend, as mentioned by Wanamaker and Sorrell. For FTSE 100 companies andbrands, the average annual advertising spend is £100 million, resulting in £15-£25 million wasted per brand each year.
effectively measure the impact of marketing efforts, a model needs to capture these touchpoints.
A robust market mix model includes all the drivers. It is this inclusion of disparate and diverse datasets which makes market mix models a golden source for evaluating the effectiveness of marketing spend.
Market mix modelling (known colloquially as MMM), is an interdisciplinary field that combines concepts from Economics, Econometrics (Statistics), Marketing, and Data Science to address this challenge. A market mix modeller can answer:
● How each media activity/campaign is performing, by providing a return on investment (ROI) for each marketing activity used. This provides a backward lens.
● How to provide a forward lens for a scenariosetting application (an Optimiser App) that allows the marketeer to forecast how best to allocate media spend amongst multiple media channels. This allows them to optimise the impact of their marketing spend:
What is the impact of my pricing (brand and competitor) – i.e., what is the price elasticity, and depending on the type of model done, how has this evolved over time?
• What is the impact of distribution?
• What is the impact of seasonality? Can I leverage seasonality and by how much?
A customer’s conversion journey is complex and holistic, and is influenced by several factors such as the economy, seasonal events, competitors, and our own actions as a brand. Market mix models condense this complex real-world into a mathematical model. To
The model, in its simplest form, is an overtime linear regression where a Key Performance Indicator (KPI) is explained by a base value and several drivers. It goes beyond correlation and aims to uncover causality, allowing us to infer the relationship between each driver and the KPI. In mathematical terms, the KPI is referred to as the ‘dependent’ or ‘explained’ variable, as it is determined by the drivers. The drivers, on the other hand, are referred to as the ‘independent’ or ‘explanatory’ variables, as they explain changes in the KPI and are not influenced by other variables or the KPI within the model. In the equation, the KPI is represented as Y and the explanatory variables as X. The model typically uses linear regression or ordinary least squares (OLS) to analyse the causal relationship between the drivers and the KPI.
Based on Binet & Field’s research on the long and short-term effects of media advertising, we developed two types of models to better understand its impact. One type of model focuses on estimating the short-term effects of marketing, while the other type estimates the long-term effects of media advertising. This approach allows us to provide insights into what works in the short-term (three months or less, plus what works in the long-term (up to three years).
A key consideration is the use of digital attribution, which requires less historical data and is faster to produce. Bluntly, that would be the wrong question. This isn’t a zero sum game – it’s not one or the other, it’s both. MMM and digital attribution should coexist in a system, where digital attribution guides the operational performance (the day to day) and MMM provides the strategic guidance. The one caveat to be mindful of is the impact that privacy regulations will have on the feasibility of multi-touch or single touch attribution going forward.
To produce robust models, it is imperative that the data encompassing each driver or variable spans a minimum of three years, preferably at a weekly level. The rationale behind this ‘magic number’ is best exemplified by seasonality. If our sales or key performance indicator (KPI) metric is influenced by events such as Christmas, having three instances of this event in our market mix
Withoutknowing what works and what doesn’t, how can we optimise our spending and get the most out of every pound, euro, or dollar?
models allows us to gauge the impact more accurately. For instance, if we observe two instances with positive effects and one with negative, we can deduce that, on average, Christmas has a positive impact on our KPI, and vice-versa. As time series regression is centered on the estimation of the average impact of each activity, having less than three years can reduce the robustness of the models.
When collecting data for our models, they fall into three buckets:
● The first covers data that looks at the state of the wider economy, i.e., economic performance metrics (inflation, GDP, unemployment), COVID, seasonality (Christmas, Easter, Summer holidays) and temperature.
● The second covers data that answers what are we doing, i.e., what is our price, what is our promotion strategy and activation, what is our distribution and what is our marketing activity.
● The third covers data that captures what are our competitors are doing, i.e., competitor pricing orcompetitor marketing.
We collect and compile data on a weekly basis, which includes information on seasonality such as Christmas and Easter, macroeconomic data such as interest rates and unemployment rates, as well as data on the impact of COVID. Some of this data is readily available through various Python packages, while other data can be accessed from publicly-available websites and directly integrated into our modeling pipeline.
In marketing, the 4Ps (Price, Product, Place, Promotions) are essential for effective promotion. Similarly, our models must include these data assets to accurately capture the KPI drivers.
● Price (pricing and product promotions – for banking this is interest rates, switcher offers (£125 if a customer switches, etc.)
● Product (how good a product is - the type of current accounts we offer, the type of mortgages we offer, any changes to the product needs to be accounted for in the models)
● Place (distribution, for example Branch openings and closures, regions of the UK that get the same product)
● Promotions (this is the advertising/marketing element such as ad media spend). This includes all our marketing activity, above the line (ATL), below the line (BTL), and digital. It is crucial that we gather both media spend and audience metrics when collecting data. The audience engagement metrics are used within the models to assess incrementality and media
spend for ROI calculations. This is because, at the heart of our market mix models, we are assessing the relationship between the public and the take-up of our KPI. For example, for TV, we would use Television Ratings (TVRs), for radio we would use Gross Rating Points (GRP), and so forth. Although given different names, each media format will have data metrics that indicate how much attention a particular campaign or activity received. In most cases, we can access this data via our media agencies or directly connect into the platforms to extract the data via application programming interface (APIs).
Equally crucial are the actions of our competitors. This becomes even more vital in the case of banking services, where the product offerings are highly homogeneous. The banking sector in the UK is known for its intense competition, with each competitor employing their own pricing, product, and marketing strategies to secure a larger share of the market. Exclusion of this data could potentially lead to omitted variable bias.
We automated our Extract, Transform, and Load (ETL) process through the collaborative efforts of our skilled data engineers and Data Scientists. This has streamlined the extraction of data from disparate sources, as well as the cleaning, data enrichment and standardisation of the data, making it ready for modeling. With automation, we can efficiently handle large volumes of data, resulting in time and resource savings. Our automated ETL process ensures that the data is clean, consistent, and analysisready, leaving our Data Scientists to focus on building models and generating actionable insights.
Ensuring the accuracy and reliability of data is crucial in any data pipeline. To achieve this, we leverage data science techniques to automate the process of quality assurance (QA). First, we implement checks to verify that the processed data matches our expected results, such as comparing the sum of raw input to the sum of raw output. Additionally, we use visual cues and exploratory data analysis (EDA) techniques to thoroughly inspect the data. Python offers several modules that expedite the creation of PowerPoints from the data pipeline, enabling us to easily share snapshots of data assets with internal and external stakeholders. This early engagement with stakeholders also helps solidify the insights they can expect from the models.
In collaboration with our data engineers, we’ve developed data products that serve as reusable assets
across the bank. Once the data is prepared, it is made accessible through our data visualisation platform, enabling a transparent culture that enhances the quality assurance process. This openness to the wider bank increases the chances of identifying and correcting data errors, as more eyes can review the data.
A prerequisite within market mix models is the transformation of our media data metrics to account for the nonlinear relationship between media advertising and our Key Performance Indicators (KPIs), as observed in real-life scenarios. In other words, in most, if not all, media channels, there is a saturation point where each additional pound we spend will have a diminishing impact on our KPI.
This phenomenon can be likened to the concept of diminishing returns in economics, where the media activity we engage in reaches a point of diminishing effectiveness. As an illustrative example, I live in Rugby, a town famous for the sport, but with a relatively modest population. If I were to place a press ad in the Rugby Advertiser, a publication that primarily circulates to the local population, I would eventually reach a point where the number of additional customers generated by the ad would plateau, regardless of the amount of money invested.
An additional aspect that needs to be incorporated into our media metrics is the concept of memorability/
adstock/decay carryover. This captures the lasting impression and presence that creative advertisements leave in the subconscious mind, also known as mental availability, even after they have stopped airing or been activated. This transformation allows us to better understand the true impact and effectiveness of our advertising beyond their initial airing or launch.
Producing our modelling framework, we integrated speed, reproducibility, consistency (to reduce biases of individual Data Scientists), and governance. To this end, we developed a customised Python-based auto modeler tool (AMT) that generates market mix models based on predefined benchmarks. With thresholds in place, the AMT allows us to iterate through a wide range of adstock and diminishing returns parameters for media activity to identify the optimal model. Even with modest compute power, we can iterate through 10,000 models within five minutes, enhancing the robustness of the final model. This capability provides valuable insights to stakeholders, helping them understand the long-term memorability of marketing activities or campaigns, as well as identifying the optimal diminishing returns for each media channel.
The validation process involves adhering to standard econometric principles to ensure model interpretability
and accuracy. We use well-known metrics such as R-Squared (R2 - coefficient of determination) and MAPE (mean absolute percentage error) to assess model performance. The R-Squared (R2) helps us understand the proportion of variance in the dependent variable explained by the model, while MAPE calculates the average percentage difference between predicted and actual values. These metrics provide a quantitative measure of the model’s predictive performance.
For each driver, we assess its statistical significance by examining its t-statistics and p-values. This helps us determine if its estimated coefficient is statistically different from zero (statistically significant). We layer on business context to evaluate its magnitude and sign of coefficient, to ensure this aligns with the expected relationship between variables.
In assessing the ROIs, we benchmark the results against previous outcomes and compare them with industry standards. Accounting for key drivers and if any are missing, we initiate further investigation and interrogation to ensure a clear rationale. Additionally, for the estimated diminishing returns of each media channel, we examine saturation points to ensure that these make sense.
An essential final stage of the validation process is peer review. Our Data Scientists review each other’s models’ methodology, assumptions, and results to identify potential biases or limitations. This process enhances the credibility of our findings and ensures that the models are reliable and accurate for informed decision-making.
Overall, by combining quantitative metrics and qualitative assessments, we incorporate econometric best practices, business context and peer review to ensure that the robustness of the models is maximised.
As we actively collaborate with our business stakeholders throughout the entire modelling phase, our philosophy is to share initial models with them at the first possible instance. This approach increases transparency and provides an opportunity for stakeholders to pose important questions that they would like answered. Furthering our commitment to deliver actionable insights that address their needs.
Equipped with insights and contextual knowledge obtained from stakeholders, our models go through a final modeling phase, where variables are included or excluded based on their relevance. This approach combines human expertise with machine learning, resulting in modeling outcomes that make sense and provide insights that are relevant to the business.
In the final stage, post creation of the econometric
models, the results are output into two formats: PowerPoint presentations (decks) and data visualisation dashboards. Output of the decks is automated via python scripts as much as possible. These include automated charts, tables, and key statements, providing a timestamped snapshot of the outputs and insights that can be easily shared with internal and external stakeholders. One drawback, however, is that they are not dynamic. To address this, we also provide results in a data visualisation dashboard. This allows our internal stakeholders to navigate and explore the results in more detail. Providing them with a self-serve option, giving them access to granular data behind the tables and charts. With the advancement of AI Language Models, we aim to further automate the generation of insights in the decks, increasing the efficiency of this process even further.
One of the key advantages of using market mix models is their ability to empower stakeholders, and to forecast and optimise their marketing media budgets. By simply clicking a button, marketers gain valuable insights, allowing them to make data-driven decisions on how to allocate media spend across different channel options. To facilitate this process, we developed an application that leverages our web development skills and uses a Platform-as-a-Service (PaaS) to host our optimisation models (embedded with response curves or diminishing curves estimated from the market mix models).
This approach involves solving a non-linear optimisation subject to constraints problem. Currently we use Python packages to find the optimal solution in scenarios such as:
1. When the budget controller has allocated £X million for the next year and we need to determine the most effective allocation of this spend across media channels to optimise revenue.
2. When the budget for the next year has already been set but an additional £Y million is available for spend, and we need to identify the most optimal allocation for maximum impact.
3. When there is a desire to spend an additional £Z million in TV advertising, but the current spend is already £T million, and a cost-benefit analysis is needed to understand the potential impact of the additional spend.
Our living, breathing optimisation apps enable us to constantly adapt and optimise our media strategies, ensuring that our marketing efforts are data-driven and yield the best possible outcomes. This reduces the wasted advertising spend to 0%.
CURRENTLY WORKING AS HEAD OF DATA AND DIGITAL FOR CERBA RESEARCH, MARTIJN BAUTERS OVERSEES THE EXISTING DATA AND BI TEAM AND LEADS THE COMPANY’S DIGITAL TRANSFORMATION. HE IS ALSO EXPERIENCED IN SECTOR-SPECIFIC PUBLIC SPEAKING.
Over the last few years I have specialised in leading data projects from both a business and technical point of view while transforming data lab teams into real delivery factories. We have witnessed multiple (r)evolutions within the realm of analytics. We transitioned from handwritten tables during the early days of professional businesses to Excel spreadsheets with the advent of computers. Following Excel, we embraced Kimball’s principles and moved towards enterprise data warehouses that facilitated data aggregation and manual value creation. In recent times, we have progressed from automated dashboards to self-service analytics, guided by the principles of effective storytelling. Now, a new era of analytics dawns, where generative AI plays a pivotal role in shaping interactive insights.
We have been astounded, surprised, and captivated by the music and art pieces generated by generative AI.
We have been astounded, surprised, and captivated by the music and art pieces generated by generative AI. The traditional GANs have evolved into more comprehensive concepts such as LLMs and LLaMas, culminating in the release of GPT models to the public towards the end of 2022, with offerings like ChatGPT and similar competitors such as Bard.
If AI can create music and art, it can also generate and interpret data from well-designed data platforms, harnessing this information to produce valuable insights. This potential, combined with new AI applications like Whisper or Meta’s latest text-to-speech model, will revolutionise the way we interact with our insights. Welcome to the era of Interactive Insights. The concept behind interactive insights is not merely connecting our modelled data (the serving layer) to a traditional data visualisation tool, but rather building an interactive layer between the data platform and the enduser. The latest LLM models can effortlessly interpret well-designed data platforms, extract meaningful data and transform it into understandable and actionable messages. Moreover, these models can comprehend questions posed to them, retrieve relevant data and transform it into information for the requester.
This integration can seamlessly fit into any company’s existing technology landscape, envision a chatbot within your communication tool (Slack, Teams, etc.); or imagine generated information embedded within your dashboards, explaining what you observe and helping to identify anomalies.
When we combine the power of these algorithms with text-to-speech capabilities, we suddenly have the ability to engage in a phone conversation during public transport or while stuck in traffic on our daily commute. Just imagine every decision-maker having a Data Scientist in their pocket, ready for use whenever needed. The amalgamation of LLMs, text-to-speech models, and well-designed data platforms will usher in a paradigm shift in the insights industry and potentially eliminate the necessity for traditional dashboards
and data analysts. In the coming months, the industry will embrace interactive insights due to the following advantages:
● They offer a simplified interaction compared to selfservice analytics.
● They do not require adaptations when business needs change, unlike traditional dashboards.
● They have the flexibility to generate reports in a versatile manner.
These benefits will result in cost and resource optimisation, empowering decision-makers to gain faster insights and make better-informed decisions.
Nevertheless, these technologies are still relatively new and prone to errors, as evident in some of the inaccuracies produced by ChatGPT. To overcome these challenges, we need better algorithms and robust enterprise data models that can effectively support interactive insights. It is crucial to avoid falling into a ‘chicken-or-the-egg’ situation, whereby decision-makers act based on imaginary information, inadvertently shaping the future of the business.
In conclusion, the mainstream adoption of interactive insights as the primary method of interpreting information will take some time considering the necessary setup of our data platforms and the ongoing challenges faced by current generative AI platforms. However, we can observe significant shifts within the data visualisation tools landscape, such as PowerBI’s integration with OpenAI in the Azure Fabric, as well as emerging startups like Ficus Analytics venturing into this space to offer interactive insights.
Likely a 3D world, an immersive video game, or virtual reality. But the Metaverse isn’t confined to science fiction or gaming anymore; it’s steadily infiltrating the business world through ‘digital twins.’ These progenies of the Metaverse are revolutionising enterprises by optimising design, processes, systems, and assets. They’re enabling businesses to lessen environmental impact, enhance customer experiences, and streamline operational costs. Allow me to demystify the concept of digital twins and explain how you can get started with a digital twin for your enterprise.
Hello, and welcome to this fascinating tale of the enterprise metaverse.
By AAKASH SHIRODKAR , Senior Director of AI & Analytics at CognizantThe term ‘digital twins’ originated at NASA in 2010 as an effort to improve the simulation of physical models of spacecraft. John Vickers, who worked at NASA at the time, coined the term. Digital twins are a far cry from their early days and have evolved beyond static, 2D replicas.
Today, they’re dynamic 3D digital clones that can learn, adapt, and predict. They mimic their physical counterparts so accurately that they are, in essence, creating a bridge between our world and the digital realm of the Metaverse.
A common misconception is that a digital twin is nothing more than a glorified CAD 3D model, a simple simulation model, a common data environment, or an eye-catching telemetry visualisation. The reality of digital twins, on the other hand, is far more complex and infinitely more exciting.
In essence, a digital twin is a symphony of data, models, and real-time information. It’s a digital entity that breathes and evolves, fed by numerous data sources and dynamically processing live data. It’s not a mere snapshot frozen in time but a living, evolving replica of its physical counterpart.
Enterprises have invested in IoT by installing sensors in the real world, and data is now being collected from thousands of devices. They can now use IoT data to sense physical events in real time.
As you install an increasing number of IoT sensors, it becomes necessary to have context about their location and the structures to which they belong.
If we take a smart skyscraper as an example, and your goal is to monitor its occupancy, simply installing a thousand sensors in the building is insufficient. Instead, a virtual model of the skyscraper with rooms, elevators, lobbies, and other relevant areas must be created, and each sensor must be placed in the appropriate context.
In this sense, a digital twin is a digital replica of a physical object that provides context for all IoT devices reporting on it. In our skyscraper example, using the sensors contextualised by the digital replica, we can determine that there are six occupants in room sixteen on the sixty-sixth floor.
Generative AI (a term you may not be familiar with, ha) is an umbrella term used to describe any type of AI that can be used to create new text, images, video, audio, code, or synthetic data. Generative AI models use deep learning to identify patterns and structures within existing data in order to generate new or desired content.
In enterprises, generative AI can perform a variety of tasks such as classifying, editing, summarising, answering questions, and creating new content.
There are many foundational models, also known as Large Language Models (LLMs), that can serve as a starting point. These LLMs can be used as a base for AI systems capable of performing multiple tasks. However, these LLM models require fine-tuning and learning through human feedback. Once they have undergone this process, they can contextually understand input at an enterprise level, enabling them to perform multiple tasks and reducing hallucinatory outputs.
The evolution of generative AI is accelerating. With proper guardrails in place, it can be used to automate, augment, and accelerate work. Each of these actions has the potential to add value by altering how work is performed at the activity level across enterprise business functions and workflows.
Generative AI is now poised to play a pivotal role in both the creation and operation of digital twins.
How, you ask?
Read on to find out.
Therefore, digital twins are defined as:
A dynamic virtual mirror representation of a physical asset, process, system, or environment that looks and behaves exactly like its physical counterpart.
A digital twin, by ingesting data and replicatin processes, enables real-time simulation, analysis, and prediction of the performance, outcomes, and issues that a real-world environment may encounter.
These aren’t static models; they evolve and learn from data to accurately similate the behaviour of their physical counterpars over time.
An everyday simplistic example of a digital twin is Google Maps. It is a virtual representation of the Earth’s surface that uses real-time data on traffic to optimise driving routes and share other relevant information.
However, it is the world of Formula 1 that offers an unadulterated glimpse into the pinnacle of digital twin maturity, providing an emblematic illustration of the remarkable strides we have made in bridging the chasm between the real and the virtual.
To fully comprehend these technologies’ transformative potential, let’s delve into their application in the fast-paced, high-stakes domain of Formula 1 racing.
In Formula 1, every millisecond counts. From car design to simulators, efficiency to analysis, and real-time decision making, digital twins have transformed the world of Formula 1. They are so critical to the success of Formula 1 teams that they are used in every facet of the Formula 1 value chain.
Formula 1 teams and drivers use digital twins to produce high-performing cars, as simulators for drivers practice, to optimise efficiency, a tool for scenario planning as well as real-time decision making in high-pressure racing conditions and lastly for post-race analysis to better calibrate feedback and understanding of the data coming from the car.
HOW DIGITAL TWINS HAVE REVOLUTIONISED FORMULA 1
DATA COLLECTION FOR A FORMULA 1 DIGITAL TWIN FROM OVER 150 SENSORS
DETERMINING RACE STRATEGY IN REAL TIME USING A DIGITAL TWIN:
In the realm of Formula 1, we now understand the profound impact of digital twins. However, there are challenges that arise when attempting to implement this cutting-edge technology.
While the advantages of implementing digital twins are evident, it’s crucial to recognise the challenges.
1. Executive endorsement : To ensure cooperation at all levels across the enterprise, which is essential to facilitate the adoption of digital twins.
2. Upfront investment: The creation of a digital twin requires people, tools, technology, and processes. Although the digital twin can be developed in stages, an initial budget is necessary to get the project started.
3. Digital maturity: A robust data infrastructure and access to high-quality data that is ingested onto a data platform are the cornerstones of a digital twin. Fundamentally, a higher level of digital maturity will make implementation easier.
4. Tools and technology: Choosing the right technology is crucial to avoid limiting the enterprise to a single, inflexible technology solution with limited integration capabilities. To build a long-term strategy, a combination of different tools and technologies that offer a high degree of flexibility in integration and scalability is essential.
5. Talent: To fully benefit from digital twins, you need more than just the right tools and technology; you also need a team of skilled resources, including data engineers, ML engineers, 3D modellers, and data scientists.
6. Compute: In complex digital twin scenarios, compute power is necessary to speed up the inference process. For instance, creating a twin of a manufacturing facility would require significantly more computing power than twinning a single asset within that facility. Firming up your cloud strategy and evaluating cloud partners is therefore key.
7. Security: To prevent unauthorised access that could potentially cause damage and disruption, enterprises must take a proactive approach to securing their digital twins. This includes implementing a robust role-based authentication, authorisation, access, and management policy as part of basic hygiene practices. It is also important to encrypt data and APIs end-to-end.
8. Change management: Adoption is an important consideration when starting with digital twins. If the digital twin is not adopted within the enterprise workflow, the investment will not see any ROI, and the enterprise will not benefit in any way. Including a strong change management process to accelerate adoption and provide a feedback loop back into the digital twin is crucial.
9. Learning curve: Successfully integrating a digital twin into the operations of a company requires not only learning how to operate it but also understanding how it will impact the organisation’s workflow and processes. To prepare for potential setbacks during the implementation process, it is recommended to allocate enough time for training and familiarisation. Therefore, enterprises should not underestimate the amount of time and effort required for the successful integration of a digital twin into their operations.
10. Partnering with the experts: While the digital twin can be considered intellectual property, which justifies allocating internal resources for its build and operation, the process can be time-consuming and tedious. Additionally, learning while building can carry risks. Choosing not to seek assistance may not be the best approach and could be counterintuitive. An ideal approach is a hybrid one where enterprises use a core of internal resources supplemented by a partner.
11. Ethical considerations: Data privacy and security are critical issues that require careful consideration, especially in scenarios involving healthcare, personal information, and other sensitive data. However, it’s also important to evaluate other aspects, such as data and model bias. Additionally, ethical considerations should be made regarding the identified use cases, as they should maintain confidentiality and sensitivity. As a result, a comprehensive enterprise digital twin policy and proper guardrails are necessary to prevent misuse.
12. Regulatory and Legal Considerations: It is important to comply with relevant laws and regulations when dealing with data and data storage, especially in digital twin scenarios with societal applications. This applies to both input and output data.
Enterprises must address several questions, including: Who owns the data generated by a digital twin? Who is responsible if a digital twin’s predictive model fails, causing harm or financial loss? Is the twin connected
to any licensed or proprietary systems? Are there any contractual obligations?
When embarking on the transformative journey of implementing a digital twin, these are some of the challenges that enterprises need to take into consideration.
To overcome these challenges, enterprises should start small by a) identifying a suitable use case and b) defining the goal they want to achieve.
To put it simply, enterprises should ask themselves, what are we trying to accomplish? Are we aiming to improve efficiency or reduce something? If we are reducing, what specifically are we trying to decrease?
Complex or dynamic environments that can greatly benefit from real-time optimisation have emerged as prime candidates for the implementation of digital twin use cases.
Once you have defined your use case and objective, it is important to have a clear business case tied to value. This is critical for maintaining your focus on achieving tangible results.
Then understand your starting position, such as your current digital maturity. Evaluate your strengths and weaknesses to determine if you’re ready to adopt digital twins based on your objectives or if you need to build up certain data, infrastructure, skillsets, cyber security, or other policy and ethical protocols.
Then focus on building a Minimum Viable Product (MVP). This pragmatic approach allows for learning and expansion because you can gradually add features to your digital twin setup rather than building the twin in one fell swoop, which could prove to be a minefield.
Learn from your MVP, set improvement goals and let it grow organically.
TO SUMMARISE THIS SIMPLY :
STEP 1: Identify what you want to achieve (use case and objective).
STEP 2: Tie down a clear business case to the objective.
STEP 3: Understand your current state of digital maturity.
STEP 4: Take an incremental MVP approach and model the environment with the help of data.
STEP 5: Gain operational awareness.
STEP 6: Establish a maturity arc in which your digital twin capabilities are incrementally improved to meet your enterprise objectives.
The implementation of digital twins should fit seamlessly into your broader digital strategy. It’s important to consider how this technology can be used to outpace competitors and secure a unique market position. For example, if a digital twin can be used to improve product quality faster than your competitors, it should be prioritised.
As enterprises continue to adopt digital twins, understanding the different stages of maturity is crucial to developing a successful strategy that enables them to unlock the full potential of this technology.
In conclusion, integrating digital twins and Generative AI into your Enterprise’s strategy can unlock a wealth of opportunities, helping you navigate the digital landscape with agility and innovation.
A convergence is taking place right before our eyes, as the capabilities of generative AI make it a prime candidate for integration into an enterprise digital twin strategy.
The potential for generative AI to democratise design, data, information, and insight is remarkable. It can accomplish this in two ways:
1. During its “Build Time,” it has the potential to democratise the creation of the digital twin.
2. During its “Run Time,” it can democratise the digital twin’s data, insights, and operations.
Creation of a digital twin, the “Build Time”: Imagine the savings that can be realised during the creative stage by exploiting the multi-modal capabilities of LLMs to develop real-time 3D digital designs, interactive experiences, and environments. As LLMs improve, these digital models are likely to get richer and more immersive, and designers might do this by merely describing what they want to build rather than painstakingly creating everything from the bottom up. Generative AI serves as a co-pilot, augmenting designers, saving enterprises time, optimising costs, and increasing productivity.
This is resulting in an increase in the number of digital twins created before new initiatives are launched. Before a shovel ever touches the ground, the digital twins of a facility or infrastructure are developed. The digital twin of Vancouver Airport had been developed before construction began, and simulations were utilised to complete the final design aspects.
Operation of a digital twin- the “Run Time”: At the operations stage, the twin can be queried for insights, goal-seeking objectives, or to augment, allowing the human to do the job more quickly and effectively.
Imagine a digital twin connected to real-time data, resulting in a digital 3D engine with the ability to emulate and simulate. This allows enterprises to simulate future time periods under specific conditions based on real-time data. The twin can be queried using generative AI. For example, imagine being able to ask the twin, “What does this data tell us, and where should we take action?”. This type of information flow throughout the enterprise enables everyone to make faster and more informed decisions.
Generative AI has the potential to transform the digital twin value chain. By automating, augmenting, and
accelerating from the creation stage to the operational stage, generative AI can unlock new levels of creativity and problem-solving.
Essentially, Generative AI has the potential to make English the lingua franca through which humans engage with digital twins, reducing most barriers to adoption.
For products , digital twins allow virtual simulation of the manufacturing process, identifying potential flaws in design prior to production. Real-time analysis and adjustments thereby enhance the product’s quality while accelerating its entry into the market.
Similarly, Service Twins serve as valuable tools for examining design functionality and implementing real-time redesigns where necessary. This approach yields a higher-quality product, meets customer needs more effectively, and provides an enterprise with a competitive edge.
Meanwhile, Customer Twins have revolutionised customer engagement by providing fully immersive product interactions, contributing to significant revenue boosts. A notable illustration of this can be seen in the automotive sector, where virtual test drives have amplified sales volumes.
In the pursuit of sustainability, digital twins aid in reducing material use and route optimisation, thus mitigating environmental impact. This technology has resulted in significant cost reductions and has aided the circular economy across all industries.
The reach of digital twins and Generative AI extends far beyond a single industry.
In manufacturing , they allow real-time monitoring and management of processes, and these insights can then be used to improve production efficiency and shop floor performance. Through predictive maintenance, it is possible to reduce asset downtime.
Healthcare applications include enhancing operational efficiency of healthcare operations, which is the foundation for offering personalised care, more precise treatment plans, and disease management, thereby substantially improving the patient experience.
Digital twins of human bodies or organ systems are being developed, with revolutionary implications for medical education and training.
In supply chain and logistics , a virtual model of the entire supply chain or logistics network can be developed to anticipate performance and optimise routes and resource allocation, lowering costs. Another application could be optimising warehouse design for better operational effectiveness.
In construction , a digital twin can help construction firms with building design elements, enabling better planning by modelling human footfall effects, light, wind, and other aspects. Digital twins for constructed infrastructure can comprehend how a facility is doing in real-time, allowing them to stay ahead of the curve and manage potential events before they occur.
In retail , people twins are used for customer modelling and simulations, enabling retailers to create customer personas to improve the experience they deliver.
Aerospace, automotive, education etc... I could go on, but I am sure you get the picture. Digital twins have gained widespread use across industries.
Digital Twins are growing in capability, performance, and ease of use. As technology continues to advance, the future of digital twins is bright, with endless possibilities for new applications and capabilities.
One trend that we may anticipate seeing as a result of substantial developments in process mining and process capture is how enterprises will create simulations for entire business functions or clusters of business processes rather than a single business process. To achieve superior outcomes, leaders will explore ways to incorporate multiple technologies into digital twins, such as machine learning,
process mining, risk analysis, and compliance monitoring.
As a result, more efficient methods of connecting data across these organisations will be pioneered, connecting digital twins and digital threads across various enterprise activities. The glTF file format is gaining popularity for exchanging 3D models across tools.
Another area where digital twins may evolve is in the realm of virtual reality. Enterprises have started using the confluence of these technologies to augment their engineers with holographic lenses or other similar devices so that they can interact with faulty assets in a more realistic and intuitive way when they are in the process of fixing them.
Digital twins will increasingly transform how we run companies. This necessitates a high level of specialisation, and no one provider provides an end-toend digital twin solution. To produce the best fit-forpurpose solution for their organisation, enterprises will need to integrate numerous capabilities. Part of that strategy will necessitate more modular, open architectures as well as the flexibility to create an ecosystem-based system.
For enterprises, the digital twin is more of a mindset than a tool. They are a formidable instrument with the ability to transform how we build, run, and maintain complex physical assets, processes, systems, or environments.
We’ve seen how digital twins can improve the performance of everything from skyscrapers to Formula 1 cars, industrial assets, airports, and even cities.
Lastly, as we navigate through the dawn of the Metaverse era, digital twins and Generative AI are poised to be vital players in bridging our physical and digital realities. The enterprise metaverse will surpass the gaming metaverse in the coming years, and digital twins will be the first fruits of this metaverse.
Digital Twins are growing in capability, performance, and ease of use. As technology continues to advance, the future of digital twins is bright, with endless possibilities for new applications and capabilities.
BPA Quality’s ELLE NEAL is a Data Scientist with a passion for problemsolving and a desire to learn. She is passionate to and a desire to learn. She is passionate about building AI solutions from Large Language Models, turning unstructured data into contextual insights. Her journey into Data Science isn’t common, but has enabled her to apply Data Science in a way that is meeting the needs of both her clients and their customers. This conviction also reflects in her work as a community champion for Cohere and Databutton, where she builds applications and shares tutorials, breaking down barriers to accessibility in AI.
When Elle isn’t elbows-deep in data, you can find her sharing her love for Science, Technology, Engineering, and Math (STEM) with young, eager minds as a STEM Ambassador, running a Robotics and Coding club. Her passion for making STEM activities accessible and free for all is as deeply rooted as her belief in using AI to make life easier.
My path to the present has been a challenging one. At the age of 37, amid grappling with extreme anxiety post the birth of my son, I was diagnosed with ADHD. Initially a daunting revelation, this diagnosis prompted me towards acceptance, understanding, and innovation, thereby shaping my personal and professional life in unforeseen ways.
For a significant part of my life, I found myself engaged in a ceaseless battle with structured academic environments, seemingly fighting against my own brain. This internal strife felt like a competition that I couldn’t win. However, my path changed when I was able to share these struggles with my coach and mentor at Cambridge Spark. Their empathy and support were instrumental in connecting me to necessary mental health resources, leading me to consult my doctor and eventually, to receiving my ADHD diagnosis.
Receiving this diagnosis marked a pivotal moment in my life. I was determined not to let it define me; instead, I chose to redefine it in my terms. I delved into the science behind ADHD, and this exploration allowed me to adopt a kinder perspective towards myself. More importantly, it enabled me to leverage the creative and problem-solving facets of my brain that are often overshadowed due to the stigma associated with ADHD. This journey of acceptance and understanding became a turning point in my life, transforming the seemingly insurmountable challenge of ADHD into an empowering discovery.
In my exploration of artificial intelligence, I quickly became intrigued by the transformative potential of generative AI models like GPT-3. This expansive model, with its billions of parameters trained to understand linguistic patterns, opened a new world of possibilities for me. It excelled in answering questions, generating code, and facilitating natural language conversations - areas that often presented challenges due to my ADHD.
In particular, one tool that became a game-changer
for me was GitHub’s AI code completion tool, Copilot. This tool leverages a powerful AI model similar to GPT-3, trained on a vast range of public code repositories. Copilot provided invaluable support during my coding activities, predicting the code I intended to write and providing real-time suggestions. It proved to be an excellent companion in navigating complex coding tasks, helping to streamline my work and improve my productivity.
Simultaneously, I found a valuable partner in ChatGPT. This advanced AI model, trained by OpenAI, became an integral tool in my problemsolving arsenal. Often, with ADHD, brainstorming sessions can lead to a whirlwind of ideas, making it challenging to structure them coherently. However, ChatGPT served as a remarkably effective tool for organising my thoughts. It helped me flesh out my ideas, refine them, and structure them logically, thus significantly aiding my problem-solving activities. The conversational nature of ChatGPT allowed for an interactive brainstorming experience, giving me insights and suggestions that often catalysed unique solutions.
The synergy of Copilot and ChatGPT was nothing short of revolutionary for me. These generative AI models didn’t just help overcome some of the obstacles posed by ADHD but also amplified my strengths. They served as a testament to the farreaching potential of AI to support and even augment cognitive diversity, marking a significant stride in my productivity and the quality of my work. Harnessing the power of these tools, I began to see challenges not as roadblocks, but as opportunities for innovation.
My newfound understanding of ADHD sparked a unique synergy between my hyperfocus periods and my data science pursuits. This confluence unearthed a multitude of opportunities, ultimately leading to the development of AI applications that not only mitigate the challenges presented by ADHD but also leverage its unique strengths. From a mind-mapping tool to an app finding relevant content, these applications represent my journey towards turning neurodiversity into a strength.
[Elle’s] passion for making STEM activities accessible and free for all is as deeply rooted as her belief in using AI to make life easier.
The first of these creations is an AI-powered mind-mapping tool. This application uses AI summarisation and code generation to convert text into mind maps, offering a supportive tool for neurodiverse readers and learners.
Throughout my apprenticeship, I faced challenges due to my ADHD, struggling with focus during video calls, journaling, and reading research papers. Realising that my brain processed information
differently, I found a solution by using a large whiteboard to create mind maps and flowcharts, aiding my understanding and retention of complex information. This experience inspired me to develop an AI-powered application that automatically generates mind maps and flowcharts to support neurodiverse learners, enhancing learning efficiency and effectiveness. My personal journey with ADHD has driven me to create a tool that can help others with unique learning needs and strengths.
APP DEMO: Databutton
AI Mermaid.js
Visual Generator
VIDEO WALKTHROUGH: Databutton
AI Mermaid.js
Visual Generator
Watch Video
TUTORIAL: Mind
Mapping with AI: An Accessible Approach for Neurodiverse Learners by Elle Neal
May, 2023
Medium
Next in line is Cofinder, an application designed to find relevant information to answer questions related to learning content. This tool comes in handy when navigating through extensive articles, research papers, or learning materials, making the process less overwhelming and more efficient.
Cofinder is my solution to address the struggles faced by the Cohere Community, especially for individuals with ADHD, in accessing relevant content efficiently. I built this application with the vision of simplifying the process of finding specific information on the platform, recognising through my own experiences that
despite Cohere’s wealth of knowledge and resources like product explanations, tutorials, open repositories, and Discord channel, locating relevant content could still be time-consuming and challenging. Leveraging text extracted from these sources, Cofinder uses Retrieval Augmented Generation methods, employing a semantic search approach, allowing users to ask natural language questions and receive the most pertinent content and context. By bringing together information from multiple sources in one place, Cofinder aims to enhance the Cohere community experience, ensuring that developers, entrepreneurs, corporations, and Data Scientists can easily find what they need, ultimately saving them valuable time and effort.
APP DEMO: Cohere LLM University
Semantic Search
With AI by Elle Neal
VIDEO WALKTHROUGH: Databutton Cohere LLM University
Semantic Search
With AI by Elle Neal Watch Video
TUTORIAL Cofinder cohere.com
Learners by Elle Neal May, 2023 Medium
Each of these applications is a testament to the power of aligning neurodiversity with AI, creating tools that empower and inspire. Together, they encapsulate my mission to break down barriers, drive accessibility in AI, and shed light on the strengths derived from neurodiversity. Each of these creations served to empower not just me, but potentially anyone else who shares my struggles.
In my journey, support from the community has been invaluable. Through the Access to Work scheme, I have applied for an ADHD coach, a resource that promises to provide an accommodating environment tailored to my unique neurodiversity. However, I recognised that there was a greater need for such resources to be readily available for a wider audience.
Driven by this realisation, I took on the role of a community champion for Cohere and Databutton, striving to build applications and share tutorials that make the wonders of AI accessible to all. My aim is to break down the barriers to accessing and understanding
AI, and by doing so, help others realise and harness their unique potentials.
My journey with ADHD and AI has been transformative. Understanding my diagnosis and learning how to leverage AI to augment my work, I've witnessed the immense potential of combining neurodiversity and AI. It is this revelation that I now want to share with the world. Through the apps I build, my advocacy work, and the story of my journey, I hope to inspire others, showcasing that neurodiverse individuals can leverage their unique strengths to bring about exceptional results.
And as I look towards the future, my mission is clear: to continue tearing down barriers in AI accessibility and to illuminate the strengths and potentials that arise from neurodiversity.
In this journey, connection and conversation are key. I invite you to connect with me on LinkedIn. Let's discuss how we can harness AI to bring out our unique strengths, regardless of whether we are neurodiverse or not. Together, we can reshape the narrative surrounding ADHD and neurodiversity, and pave the way towards a future where everyone can tap into their unique potential.
My personal journey with ADHD has driven me to create a tool that can help others with unique learning needs and strengths.BY PHILIPP M. DIESINGER , DATA AND A.I. ENTHUSIAST; CURRENTLY PARTNER AT BCG X
Consistent and sustainable growth of the world economy will remain critical to overcome the significant challenges that lie ahead of human kind. One of the most promising drivers for economic growth is the creation of new efficiencies in the workforce with AI. The next decade will present the opportunity to move from a human to a hybrid workforce where GenAI technologies support us seamlessly.
Over the next few decades, humanity will face significant global challenges; including the need to transform economic models towards more sustainable growth (not relying on large-scale resource exploitation) and to address global environmental pollution. Meeting these challenges will require significant resources and a sustainable, growing world economy.
History has shown that extended periods of economic growth can not only provide the resources needed to solve urgent problems, but also reduce tensions and create opportunities for global collaboration. Such periods of sustained growth are often based on significant technological breakthroughs that boost workforce productivity across sectors.
The anticipated transition from a purely human workforce to a hybrid workforce, where humans and AI-systems collaborate closely and seamlessly, qualifies as such a technological leap. This new type of workforce will be supported by AI systems that effectively provide every worker with an expert companion for almost every field imaginable, leading to significant jumps in efficiency. GenAI companions will be able to write emails, organise calendars, draft presentations, write code, produce ad-hoc reports leveraging complex data analysis, search through vast amounts of unstructured data and provide relevant information for insightful and fact-based decision making. They will complement human weaknesses by not only providing strong communication skills but also expert domain knowledge
where it is needed. This may even turn formerly unsuited candidates into potential hires.
Organisations must begin preparing their workforce to enable a smooth and successful transition to the new way of working. Roles and responsibilities, ways of working, policies, processes and hiring practices need to be adapted for the transition to a hybrid workforce if companies want to benefit from increases in efficiency, robust economic growth and ensure competitiveness.
The integration of human and AI workers into a ‘hybrid workforce’ represents a unique opportunity for sustainable economic growth and addressing global challenges in the coming decades. Urgent workforce transformation is required to enable this transition and to boost productivity; ensuring growth and competitiveness across most industry sectors and professions globally.
The future work model will feature close collaboration between humans and AI systems, similar to having an expert for every field available at all times. AI can handle routine and repetitive tasks, freeing up human workers to focus on more complex and creative work requiring human judgment and creativity. This model is expected to significantly improve productivity and quality.
In a hybrid workforce, tasks can be completed much more quickly while also achieving higher levels of quality. A hybrid workforce is anticipated to convincingly outperform traditional human teams, as AI can compensate for an individuals’ weaknesses and unlock their full potential. This combination of human expertise and AI assistance can result in highly efficient and effective workflows, leading to superior outcomes for organisations.
To successfully transition to a hybrid workforce, organisations must tackle numerous transformational challenges:
● Understanding the potential challenges and risks of the transition towards a new work model and addressing these proactively.
● Investing in talent and skills and updating roles and responsibilities to encourage collaboration between humans and AI. Investments in training and development programs to build the necessary skills within the organisation.
● Understanding the potential impact of a hybrid work model in their respective industry sector and forming a clear ‘hybrid workforce strategy’ to maximise benefits. This includes identifying areas where AI can be most effective and training workers to collaborate with AI tools while ensuring these align with the organisation’s overall goals and values.
● Recognise the potential of AI to augment human
abilities: instead of viewing AI as a threat to jobs, organisations can recognise the potential for AI to augment human abilities, allowing workers to be more efficient, effective, and creative.
● In the future, organisations will likely connect and train existing third-party large-scale AI systems (“LFMs”) with their in-house data to develop unique AI systems that can strongly support their specific needs. To achieve this, organisations must prioritise data collection and management to ensure that they have high-quality data to train such models. Given that AI systems are heavily dependent on data quality, organisations might need to consider establishing efficient data management practices and invest in data analytics to derive insights that can inform decision-making processes. By doing so, organisations can ensure that their hybrid workforce model is built on a solid foundation of accurate and reliable data, which is essential for achieving optimal performance and productivity.
● The development of new frameworks will be required to deal with rapid advances in technology in a timely manner. These include: regularity compliance, data privacy and security, ethical considerations and technological considerations.
● Seamless and efficient collaboration between human and AI workers requires a culture that values and encourages collaboration and communication. Organisations can promote such hybrid collaboration and provide opportunities for workers to learn and develop together.
● As the field evolves rapidly, it is important to foster collaboration with other organisations and share knowledge and best practices. This can include participating in industry groups, attending conferences, and collaborating with academic institutions.
● It will be critical to ensure ethical and responsible use of AI as well as transparency and safety. A hybrid work model raises important ethical and social considerations, such as bias and the potential for misuse. Organisations should be proactive in addressing these issues. As AI becomes more integrated into the workforce, it is important to prioritise transparency and ethical use. Organisations can be transparent about how AI is being used and ensure that workers understand and are comfortable with the technology.
Transitioning towards a hybrid workforce is a very significant opportunity for sustained economic growth. The process towards workforce readiness needs to begin now, in order to ensure a smooth and successful transition.
There are methodologies that actually allow large language models to run and be retrained quickly - and there is a lot of activity and research in this area. There’s one method in particular, LoRA, that reveals the secret behind how large corporations like OpenAI can provide large language models to millions of people, and can retrain these models over and over again.
How can these models stay up to date with whatever is going on in the world, and how is it possible that a 175 billion parameter model can be constantly retrained and fine-tuned with minimal effort? In fact, it’s not actually about fully retraining these models - which is where the secret sauce is.
The problem with large language models is the very fact that they are large. 175 billion parameters is a number that we could not conceive of until a few months ago. We were running many of these models at home or in our jobs, work infrastructure, or AWS in the domain of several millions, not billions, number of parameters. With ChatGPT, we broke that ceiling, which is a good thing, because now there is a trend to make the model smaller.
We know that if we increase the number of parameters, the model becomes more powerful. That means we have more data, more parameters, more degrees of freedom, and potentially more sophistication in the answers that large language models (in the case of NLP) can give. But the bigger the model, the less scalable the model is. From a practical perspective, this might be a way to keep other companies out of the competition. Only the big players who have the financial and infrastructure capacity can actually do research that brings results to the world of AI. Even more so with building massive models.
A few years ago, practitioners and researchers made a non-written promise to democratise data science. This was clearly not happening, at least until LoRa came out. Fortunately, mathematics and computer science come to the rescue. LoRA is one of the most important methodologies behind the powerhouse of such big models, and is the reason why these models can be fine-tuned constantly with minimal effort.
By minimal effort, I mean several orders of magnitude less parameters to be fine-tuned.
It’s worth stating that there’s no magic here, there’s no AGI, there’s no religion behind deep learning and
artificial intelligence. There is mathematics and then there is linear algebra, optimisation, and computer science. With this said, let’s get started.
LoRA comes from a two-year old paper that was written by researchers at Microsoft. The title of the paper is ‘LoRA, Low-Rank Adaptation of Large Language Models ’. Of course, it is built on several other concepts that come from even earlier than 2021.
For example, the concept of transformers is even older than that - it originates from Google, back in 2017. Seeing Google lagging behind in the AI or ChatGPT/LLM Models race when they actually invented the transformer, one of the most used architectures in Large Language Models today, is rather bizarre. For some, this is actually expected when research is made publicly available and paves the way to anyone who can create new tools, methods and models. This is the beauty of research and healthy competition that both keep raising the bar and make people more ambitious.
LoRA is one of the most important methodologies behind the powerhouse of such big models, and is the reason why these models can be fine-tuned constantly with minimal effort..
Remember the size of models from the Computer Vision field of research?
The typical classifier or object recogniser could sport something like a few dozen layers and several million parameters, in order to convert pixels into more and more abstract representations until assigning a label (in the case of an object classifier). Even though we were used to considering such models ‘large’ already back in the day; a concept that was already heavily applied to classify animals or tumors, cars or people with the same model was transfer learning.
Transfer learning has been the trick to move from one domain to another (from analysing general purpose images to, for example, medical images) for many years. It consists of maintaining some of the initial layers or a network ‘untrained’, and retraining the remaining layers until the output. It seemed to work really well, due to the fact that all images share the low-level pixel representation, regardless of their type or domain.
However, one issue with such a technique is that applying transfer learning to, for example, 10 domains would require 10 different models, that, except in the first layers, would be completely different. If the space to store large numbers of parameters is no longer a problem for many, the size that such models require in memory (RAM or GPU) suddenly becomes prohibitive.
Back in the day, there was no way to retrain just a tiny bit of the weights of the initial model and proceed with transferring the model to other domains. LoRA solves many of such limitations. Any large model can essentially be extended with just a tiny amount of weights, without being limited on, for instance, the length of the input sequence (context or prompt). At the same time, fine tuning such models would not incur any loss of accuracy or increase in inference time.
In general, extending a model with additional parameters means having longer predictions at inference time, due to the higher amount of matrix calculations. So, how can one get the best of both worlds, namely not being forced to retrain a model from scratch and, in case of no retrain, not paying a prohibitive cost in terms of accuracy? There can be only one answer: mathematics.
the concept of low-rank matrix. A low-rank matrix is a matrix where the number of linearly independent rows or columns is much smaller than the number of rows or columns. A matrix with a lot of linearly independent rows and columns, is more difficult to factorise. In a matrix with a number of independent rows and columns much smaller than the size of the matrix itself, there is a lot of room for factorisation in very efficient ways. The curious reader who wants to know more about low-rank matrices and linear algebra, can get access to many sources, from Wikipedia to calculus books. This is such an amazing field, at the core of pretty much any machine learning operation. Not being familiar with linear algebra, one is usually missing the nitty gritty of machine learning algorithms, from logistic regression, to deep learning, to ChapGPT, and anything that can be built on top.
As a matter of fact, LoRA can be applied to any dense layer of models, although the authors initially focused on certain weights in the transformer language models only. In particular, they ran experiments and performance benchmarks on ChatGPT 3 with 175 billion parameters, the most advanced model at the time. As a generic mathematical model, it applies to other models too.
Running a neural network in training or inference mode, basically means performing matrix multiplications in the background. During inference, the input is (generally) transformed into a matrix, and such matrix is multiplied with other matrices that represent the inner layers of the network. There are usually hundreds, even thousands of such layers. Hence, the output depends on the specific task (also called downstream task) - that could be a probability, a vector representing probabilities, a label, an index, etc.
A pre-trained weight matrix (usually called W0) has a certain dimension and rank. The key observation from the authors of LoRA is that pre-trained language models usually have a low intrinsic dimension. This means that it is possible to learn efficiently regardless of a random projection of such a matrix to a smaller subspace.
Before explaining how the LoRA methodology works, I need to explain what low-rank means. With the LoRA approach, low-rank adaptation enforces and exploits
In other words, even by projecting to a much smaller dimensional space, these models don’t lose accuracy. Such an intrinsic dimension is usually much lower than the initial dimension. A hidden layer of a neural network is usually represented by a matrix W, also called the weights matrix. During training, such a weight matrix receives constant gradient updates via back propagation and changes accordingly. Typically one retrains a network, updates the gradients, and back propagates everything again. Researchers found that one can actually
With LoRA one no longer needs hundreds of costly GPUs, or expensive cloud infrastructure.
constrain the updates of a weight matrix, representing it with its low-rank decomposition (B.A or B dot A, where B and A are multiplied with the same input vector/ matrix).
Representing a weight matrix W (as an initial pretrained matrix W0 plus B.A), one can retrain only a subset of all the parameters. This is usually a very tiny number. Therefore, the trainable parameters are lowered to the B.A rather than W0, which stays untouched. To make such a concept even more clear, one essentially trains a vector or a matrix, which is way smaller than the initial trainable weight matrix of the same model. In order to transfer to other domains, or fine tuning the model, requires to retrain just the matrix B.A.
As this is a very quick operation with very little memory overhead, retraining regularly would be absolutely possible. Moreover, as the initial weight matrix would stay basically untouched, large language models would not be affected by storage limitations, due to the fact that the initial weight matrix will be stored once and for all.
One can, in principle, apply LoRA to any subset of weight matrices in a neural network in order to reduce the number of trainable parameters. But, in their paper, the authors apply the method only to the transformer architecture.
In particular, the researchers only studied the attention weights for downstream tasks and they froze the multi-layer perceptron layers or modules - meaning these are not retrained in downstream tasks at all. After all they just had to prove that the method works and it can be generalised.
To put things in perspective, GPT-3 with 175 billion parameters, might require approximately one terabyte of memory. Reducing the memory footprint to 300GB would immediately cut the costs of orders of magnitude. By lowering the rank even more and sacrificing accuracy for a different downstream task, one could get about 10,000 times less parameters than what is initially required.
Such an impressive reduction could benefit all smaller companies that need LLMs for their research or core business, while not having access to the financial resources of the bigger players.
With LoRA one no longer needs hundreds of costly GPUs, or expensive cloud infrastructure. We all know how costly retraining machine learning models is, especially because they represent trial-anderror science.
Last but not least, low-rank matrices are at the core of another essential improvement: researchers measured about 25% increase in speed during training.
I strongly believe LoRa is one of the most important methods out there since the transformer architecture was introduced. Researchers are more and more interested in reducing the number of parameters of large models, while maintaining the same level of accuracy and power. Observing low rank structures
in deep learning is expected. But acknowledging the existence of it and measuring its practical benefits is a completely different story.
The LoRA paper finally proves and shows that what was an opinion back in 2018 and 2014 is now a fact.
Remember that every time you chat with ChatGPT.
FRANCESCO GADALETA is the Founder and Chief Engineer of Amethix Technologies and Host of the Data Science At Home podcast. datascienceathome.com
ISSUE 5: 22nd November 2023
ISSUE 6: 21st February 2024
ISSUE 7: 8th May 2024
ISSUE 8: 4th September 2024
ISSUE 9: 20th November 2024
We will consider taking a very small amount of exclusive,sector-specific advertising for future issues. For our Media Pack, please email the Editor.
We are always looking for new contributors from any Data Science or AI areas including:
Machine Learning and AI
Data Engineering and platforms
Business and industry case studies
Data Science leadership
Current and topical academic research
Careers advice.
If you or your organisation want to feature in a future issue(s) then please contact the Editor: anthony.bunn@datasciencetalent.co.uk
The Data Scientist magazine is a niche, highquality publication that is produced in two formats: print and digital.
The magazine is read by thousands within your sector, including leading companies, Data Science teams and Data Science leaders. Print copies are posted to leading, selected Data Science and AI experts, influencers and organisations and companies throughout the world.
We also send digital copies out to our large and growing subscription list, whilst each issue is available online on Issuu.
Someone who knows their stuff and just fits in like they’ve been with you from the start?
QUICK HIRES : With us, you’ll find the right person in just 48* hours. Guaranteed.
THE PERFECT FIT : We’ll help you find the contractor with the right skills who’ll get along with your team. NO MORE HIRING FAILS : You can seriously reduce the chance of a bad hire.
We’ve built the world’s first profiling system made just for Data Science and Engineering teams. It’s based on eight important profiles that exist in these teams. This software gives you a clear picture of what kind of Data Scientist or Engineer someone is, what they’re really good at, and the stuff they like to work on. Find
*In the first two weeks - If we provide you with a contractor who is not a fit, we will replace them immediately and we won’t bill you.
Are you trying to find the perfect contractor for your Data Science team?