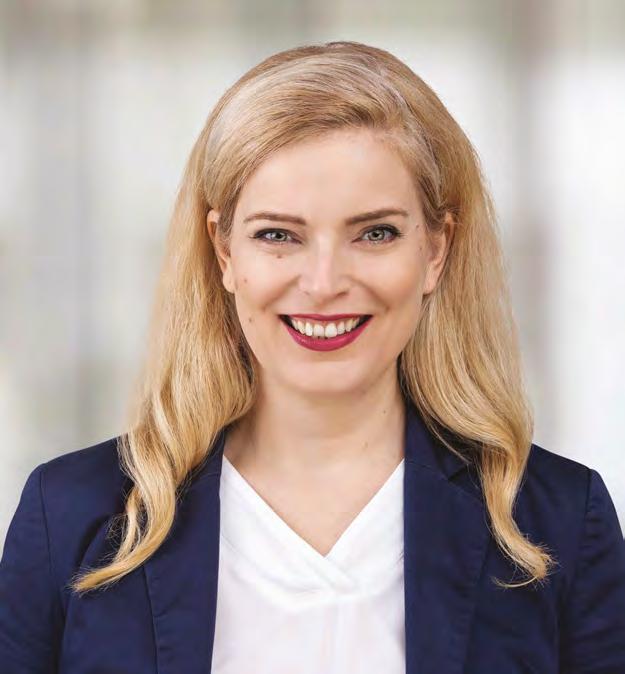
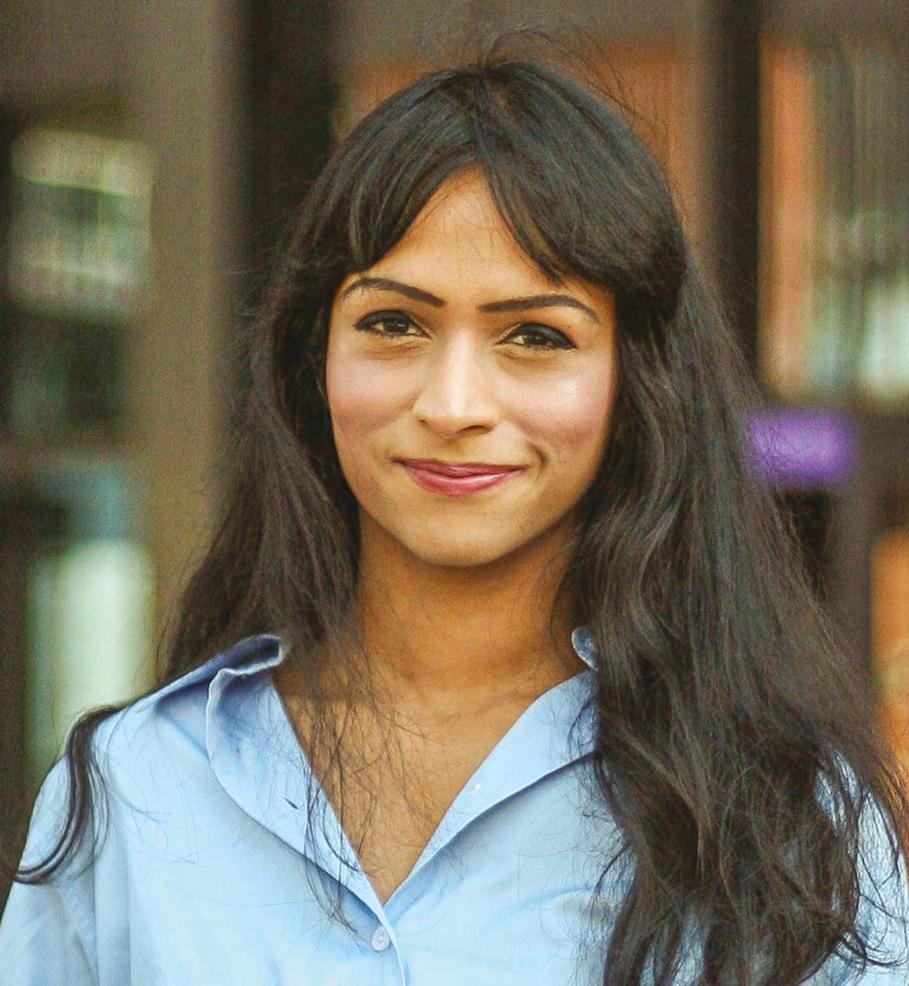
Expect smart thinking and insights from leaders and academics in data science and AI as they explore how their research can scale into broader industry applications.
Key Principles for Scaling AI in Enterprise: Leadership Lessons with Walid Mehanna
Maximising the Impact of your Data and AI Consulting Projects by Christoph Sporleder
How AI is Reshaping Startup Dynamics and VC Strategies by KP Reddy
Helping you to expand your knowledge and enhance your career.
Hear the latest podcast over on
CONTRIBUTORS
Anna Kopp
Maha Reinecke
Stefanie Babka
Tamanna Haque
Didem Un Ates
Nicole Janeway Bills
Sonja Stuchtey
Rosanne Werner
Agata Nowicka
Łukasz Gątarek
Bettina Knapp
Henrieke Max
Philipp Diesinger
Francesco Gadaleta
EDITOR
Damien Deighan
DESIGN
Imtiaz Deighan imtiaz@datasciencetalent.co.uk
DISCLAIMER
The views and content expressed in Data & AI Magazine reflect the opinions of the author(s) and do not necessarily reflect the views of the magazine, Data Science Talent Ltd, or its staff. All published material is done so in good faith. All rights reserved, product, logo, brands and any other trademarks featured within Data & AI Magazine are the property of their respective trademark holders. No part of this publication may be reproduced, stored in a retrieval system, or transmitted in any form by means of mechanical, electronic, photocopying, recording or otherwise without prior written permission. Data Science Talent Ltd cannot guarantee and accepts no liability for any loss or damage of any kind caused by this magazine for the accuracy of claims made by the advertisers.
As we present our 10th issue of Data & AI Magazine , we find ourselves at a meaningful juncture that calls for both reflection and forward vision. Over the past nine issues, we’ve published more than 120 articles, bringing you insights from the forefront of enterprise data and AI transformation. This milestone offers us an opportunity to consider not just how far the field has advanced, but also who has been the catalyst for that advancement.
It was a deliberate choice to dedicate our landmark 10th issue to women leaders in data and AI. The contributions of women in this space have been profound, yet often underrepresented in industry narratives. The leaders featured on our cover and throughout these pages aren’t just excelling in their respective organisations – they’re reshaping how we think about data culture, AI adoption, and technological leadership itself.
In this issue, we hear from Stefanie Babka of Merck on winning hearts and minds to build a true data culture – a reminder that technology transformation is fundamentally human. Tamanna Haque from Jaguar offers a refreshingly candid perspective on balancing professional excellence with motherhood in the fast-paced world of AI. Microsoft’s Anna Kopp provides a framework for successful enterprise AI adoption that emphasises practical implementation over theoretical potential.
A recurring theme in this issue is the crucial role of product management in AI success. Two of our featured leaders highlight this often-overlooked aspect: Maha Reinecke demonstrates how product management principles are transforming data initiatives at Reinsurance Group of America, while Henrieke Max from Delivery Hero addresses the critical gaps in AI product management that can make or break implementation efforts.
We’re also featuring Agata Nowicka who shares how Female Foundry is creating new pathways for women tech entrepreneurs, and Bettina Knapp, another leader with a Product background, whose work at Boehringer Ingelheim demonstrates how integrated data systems are revolutionising biopharmaceutical decision-making. Rounding out our featured contributions, Sonja Stuchtey presents a compelling vision for integrating environmental valuation into investment practices at Land Banking Group – illustrating how data science is expanding into increasingly diverse and impactful domains.
What strikes me about this collection of voices is not just the technical expertise they represent, but the breadth of perspective they bring to our field. These leaders are applying data and AI to solve fundamentally different problems across pharmaceuticals, automotive, technology, insurance, venture capital, and environmental sectors. Their work comes at a critical moment for our industry. As Microsoft CEO Satya Nadella recently acknowledged, despite remarkable technical advancements and massive investments in AI, the technology is still not consistently delivering tangible value for businesses and consumers.
Nadella suggests AI will only prove its worth when it manifests in measurable economic growth and practical efficiencies, and not just in technical capabilities.
This value gap underscores why diverse leadership in data and AI isn’t just about representation. It’s about effectiveness. The leaders featured in this issue approach AI implementation with different questions, priorities, and frameworks for success. They demonstrate that the future of our field depends not just on algorithmic innovation but on diverse thought leadership that can translate technical possibility into practical value.
As Data & AI Magazine enters its next chapter, we remain committed to highlighting the full spectrum of voices advancing our industry. The perspectives shared in this issue remind us that the most transformative applications of data and AI come when we have diverse teams asking different questions and approaching problems from multiple angles.
Thank you for being part of our journey through these first ten issues. We look forward to continuing to bring you insights from the pioneers – of all backgrounds – who are defining the future of enterprise data and AI.
Damien Deighan Editor
ANNA KOPP has been director of Microsoft Digital at Microsoft Germany since 2015 and has been with the company since 2004 in multiple global roles in sales and operations. She’s the branch manager of the main office in Munich, global co-chair of Women@ Microsoft, co-founder and board member of Female CIO Circle, on the advisory board of CIOnet CIDO Women, and vice chair of the Board of Directors for Münchner Kreis.
Anna comes from Sweden where she studied international communication at the University of Stockholm, and has lived in Germany since 1992. In 2020 she was voted one of the most inspiring women in Germany, in 2023 she was chosen by The Promptah AI magazine as one of the Top 100 Role Models for a Better Future, and she has just been named one of the most innovative women in AI and Data by CIO Look magazine. She is a specialist in the new world of work and AI from a cultural, political and practical perspective. She advocates flexible working models and is a gender equality role model in the German tech industry. In her spare time she enjoys off-road motorbiking and sings in a rock band. She even won a Rock Oscar as Germany’s best singer once upon a time!
What’s the most important thing to consider when driving AI adoption in large enterprises?
ANNA KOPP
Apart from the technology itself, transformation and accountability are key. You can’t roll out new technology without guiding people through the change. It’s about more than just introducing tools; it’s also about re-evaluating and adapting processes.
Many companies treat transformation as something they can squeeze in whenever there’s a bit of spare time. But transformation isn’t a hobby. You need to assign someone to take ownership, manage the process end-to-end, and prioritise the starting points. You have to decide what technology will be used, who will use it, and how processes and workflows will need to change.
Another critical aspect is workforce upskilling. Implementing AI means addressing a skills gap because the technology is so new. Prompting AI to do tasks might seem simple at first, but there’s nuance in crafting effective prompts. AI is excellent at executing what you ask, but not necessarily what you intended. Learning how to prompt effectively is a skill that improves with consistent use.
Using AI involves more than a one-off training session: organisations need to allocate the time for employees to learn and integrate AI into their roles.
Should organisations have a chief AI officer or an equivalent role? And why is it important?
Absolutely. You need a chief transformation officer or a similar dedicated role; someone with the skills to drive change. Managing transformation involves specific competencies, like milestone tracking and prioritisation, which are distinct from standard management skills.
Many companies, including Microsoft, have introduced roles like the chief AI transformation leader. We did this three months ago because the skills required for AI transformation are unique. It’s a significant investment – these roles demand highly skilled individuals, and they don’t come cheap – but if you want the job done well, you need the best people. Having someone with expertise ensures the transformation happens efficiently and stays within budget.
This role should also report to IT, specifically to the chief information officer (CIO). It’s similar to how cybersecurity typically reports to the CIO through a chief information security officer (CISO). AI transformation is a technology-driven process, and IT is best equipped to oversee it.
Additionally, AI implementations require crossfunctional collaboration. For example, integrating AI involves data management, but it also needs to align with cybersecurity measures. These teams need to work together seamlessly, and it’s the responsibility of the transformation leader to facilitate that collaboration.
Does Microsoft have one chief AI transformation officer in a specific region, or are there multiple transformation leaders globally?
About eight years ago we implemented the Chief Transformation Office, starting with one global chief transformation officer. Each country then had its own chief transformation lead, supported by a few program managers. This structure was designed to oversee the overall digital transformation, which was a major focus at the time.
That transformation was significant, but now I think a better term is evolution. We’ve already digitally transformed, and now we’re evolving into the age of AI. Rather than having a dedicated chief AI transformation lead for each market, we’ve created a global organisation to drive the programmatic approach. This structure breaks things down and works directly with regional sales segment leads, often collaborating with roles like sales excellence managers and chiefs of staff in each market.
Using AI involves more than a one-off training session: organisations need to allocate the time for employees to learn and integrate AI into their roles.
When it comes to piloting AI solutions in large companies, who should be involved?
There are two critical aspects to consider: testing groups and governance boards.
Before you even start piloting, I always recommend establishing a governance board. This is essential because, once you begin, issues will inevitably arise. For instance, employees might feel disadvantaged, or unexpected challenges may emerge. A governance board is there to address these situations.
The board should include representatives from IT, HR, the works council (if applicable), your data privacy lead, and possibly your legal department. Often, the data privacy officer reports to the legal department, so they might serve as the representative. It’s important to have clear communication channels so employees know how to reach the board with concerns or questions. This ensures there’s a structured process for handling unforeseen issues that arise during implementation.
Once the governance board is in place, you can move on to testing. At Microsoft, we decided that the people who would eventually sell the product should be heavily involved in the testing phase. Since they work with it daily, their feedback is invaluable in identifying what works and what doesn’t. Alongside them, we included IT, HR, engineering, and the works council.
For countries with works councils (there are around 13 in Europe), it’s especially important to involve them early. Works councils represent employees’
ANNA KOPP
interests in labour relations and play a significant role in determining whether the technology can be implemented.
This is particularly crucial if the technology wasn’t developed in Europe. If you’re adopting software from the United States, China, or India for example, the engineers building it may not fully understand the specific regulations in various European countries. Early feedback from works councils helps ensure the technology or its configurations comply with local regulations, and can be adapted if necessary.
AI is a tool, like a hammer or screwdriver, that supports your work. But it’s still humans who decide how to use it.
If AI has the potential to be capable of replacing human workers, would organisations like works councils ensure that this won’t happen?
Works councils are there to protect employees and their jobs. But displacement isn’t going to happen overnight. Instead, it will evolve gradually, and specific types of jobs – particularly those that are highly data-driven –may be more susceptible.
Take translators, for example. That’s a role where AI has made incredible strides, and it’s now capable of handling translations very quickly and accurately. So it might not be a career someone wants to focus on today. But it’s not about all jobs disappearing; it’s about slowly replacing certain tasks or roles.
At the same time, we’re facing a paradox in the market. On one hand, HR departments say there’s a talent shortage; they can’t find the skills they need to run their organisations. On the other hand, there’s fearmongering about AI being a job killer. The reality lies somewhere in the middle.
To address this paradox, companies need to focus on two things simultaneously: automating what can be easily automated and upskilling or cross-skilling their workforce. These two efforts go hand in hand. You automate repetitive or routine tasks, and then you redeploy your workforce to focus on areas where human input is essential.
So far, we’re still seeing more jobs being created with AI than eliminated. Someone still has to engineer, develop, manage projects, interact with customers, and truly understand their needs. Even with sophisticated AI, there’s an ongoing need for a human in the loop: someone to take accountability and make final decisions. AI is a tool, like a hammer or screwdriver, that supports your work. But it’s still humans who decide how to use it.
Works councils play a key role in this evolution. They’re involved to ensure these principles are upheld:
protecting jobs while also supporting the company’s success. For a company to safeguard jobs in the long term, it needs to remain efficient. If there aren’t enough people to fill critical roles, the company has to turn to automation. It’s a complex balance, but works councils are part of ensuring that the process is fair and sustainable.
What does a well-functioning setup for AI adoption look like?
The first step is to get the basics right, and give employees time to engage with the material. There are a few key components to a good learning path:
First, if there are jobs that require AI-specific skills you need to establish an academy – a series of structured, sequential trainings. I usually describe it as a pyramid. At the base of the pyramid are foundational skills for all employees. These are broad competencies that everyone needs, regardless of their role.
The next layer is role-specific training. For example, what does a salesperson or marketer need to know to integrate AI into their specific job? This layer focuses on the skills and knowledge tied directly to roles within the organisation.
At the top of the pyramid, you have ad hoc, situation-specific training. These are small, snacksized trainings designed to address immediate needs. For example, someone might encounter a situation and wonder, ‘How can AI help me with this?’ or ‘How do I achieve a specific task using AI?’ This is where having a searchable, on-demand database of resources is crucial. Employees need quick and accessible answers without spending days in a classroom.
At Microsoft, we allocate ten learning days a year for training. These include mandatory sessions, as well as AI-specific learning days where employees can dive deeper into topics like prompt engineering.
Finally, businesses should supplement their internal training programs with external industryspecific content.
In summary, a strong learning path starts with foundational training for all employees, builds rolespecific competencies, and provides on-demand resources for immediate challenges. It’s a layered approach that evolves as employees learn and grow.
As issues arise during AI adoption, how does Microsoft ensure clarity on what employees should do in those situations?
This is where HR and IT collaboration becomes essential. There are certain things employees shouldn’t do with AI – guardrails that need to be established. These rules of engagement can sometimes be built into the product itself. For example, you could design the system so that if someone tries to do something inappropriate, the functionality is simply greyed out with a clear ‘no-go’ message.
ANNA KOPP
However, overly restricting the technology can reduce its value. Striking the right balance is critical, especially in regulated industries like finance, where compliance with data privacy laws is a priority. Companies need to develop clear guidelines tailored to their specific processes and culture. While universal principles for responsible AI exist, every organisation must create rules that reflect its unique needs and values.
This requires assembling a team to define these guidelines. It should also be clear what happens if someone violates them. For example, if a manager uses AI inappropriately by tracking an employee unfairly and giving them a lower bonus. Employees need to know exactly where to escalate the issue. This is where the governance board I mentioned earlier comes in. It provides an independent review to ensure fairness, holds people accountable, and determines consequences.
Establishing this kind of structure ensures employees feel protected while enabling responsible AI usage. Of course, it’s a complex challenge that varies from company to company.
What are some of the tactical elements Microsoft has implemented to support co-pilots and ensure adoption?
One of the tools we use is the Work Trend Index (WTI), which you can find on the Microsoft website. It’s a research initiative that gathers statistics on what employees and managers expect from AI. The top expectation from employees is that AI should handle the ‘boring stuff’; tasks like taking meeting notes, transcribing discussions and other repetitive administrative work.
We’ve all been in meetings where someone asks, ‘Who’s going to take notes?’ and everyone avoids eye contact. AI can take over those unenjoyable tasks, helping employees focus on more meaningful work. Beyond that, people want AI to help them keep order
amidst the chaos – organising information and making it easy to find what they need.
To meet these needs, we’ve created unified landing pages where everything related to AI is centralised.
These pages include:
● Training resources: Where to find training materials and courses.
● AI champions: A list of experts employees can reach out to for help.
● Office hours: Opportunities to ask questions and get guidance.
● Reference materials: Links to videos, white papers, and detailed guides on how AI works.
● Re sponsible AI guidelines: Clear recommendations and policies for ethical AI use.
● HR resources: Connections to HR guidelines and support.
Having all this information in one place ensures employees always know where to go for support. It’s about making engagement with AI as easy and intuitive as possible. Accessibility and simplicity are key to driving adoption.
We also have an organisation called Worldwide Learning at Microsoft which strategically develops training programs, because learning doesn’t just happen on its own; it has to be planned. If someone needs to be certified for a task, that requires structured training. Certification programs must be integrated into daily work routines, alongside other activities like dealing with customers.
This learning organisation sets the overall strategy, organises learning days, and designs the various types of training: academies for in-depth skill-building, snack-sized ad hoc training, videos, or searchable databases. These resources support ongoing learning.
The way we learn today is different from 30 years ago. Back then, you might attend a three-day classroom training for a new ERP system and then use that tool for 10–15 years. Today, with agile methodologies and rapid software updates (often new releases every two weeks) you need to continuously adapt.
ANNA KOPP
What criteria should companies use to pinpoint which business functions will benefit most from AI? We start by identifying roles or areas with the most specialised, content-heavy tasks. For example, sales teams working with CRM tools that handle large catalogues of products or SKUs – they need to deeply understand the products. Similarly, operational teams like HR, procurement, finance, and legal often benefit significantly.
Take legal tasks, for instance. Something as straightforward as drafting a non-disclosure agreement, checking when the last one expired, and creating a new one can be done quickly with AI. These are timeintensive, repetitive tasks – mostly involving copypasting and searching – that AI can handle efficiently.
The second recommendation is to start small. Find one specific problem and solve it. Don’t aim for a massive, all-encompassing platform right away. I discussed this in a TED Talk I gave about the Vasa ship – a Swedish warship that sank because too many big ideas were crammed into one project without proper planning. The same principle applies here: if you try to build a massive AI solution over several years, employees will lose patience and resort to quick fixes for their smaller problems.
Analyse your organisation and identify areas where the most time is wasted or where repetitive tasks occur frequently. Start with one problem and one solution. Invest just enough to see if it works. If it does, build on it. If it doesn’t, scrap it and move on to the next issue. By narrowing the focus to specific use cases, you can create a clear path to success. Solve small problems first, and over time, you’ll build a suite of effective, smaller solutions before tackling a larger platform. Additionally, leverage the tools and products already available. There are robust suites, like Microsoft’s, that help with the overall office environment. Instead of reinventing the wheel, focus on your line-ofbusiness applications and industry-specific tools. Also, consider how AI can enhance your service-level agreements (SLAs) and responsiveness to customers.
What are some of the key lessons you’ve learned from driving AI adoption in a multinational company like Microsoft?
Localisation is a big one. First, there’s language localisation, but it goes beyond just translating words and grammar. It’s about understanding cultural nuances. For example, in Germany, when addressing customers, we often use formal titles – like Herr Müller or Frau Müller – and we use formal speech, which we call Siezen . It’s hard to fully explain in English, but it’s about speaking formally versus informally. In contrast, in the U.S.,
For most employees, that’s the key: reducing the overwhelming workload so they can focus on what they enjoy and the work that made them choose their career in the first place
it’s much more casual: ‘Hey, John, how’s it going?’ You can’t just translate these cultural differences directly, and it’s important to account for them when you’re working across different markets.
Another important aspect is how AI can help with time zone challenges in global companies. For example, AI can summarise or catch you up on meetings or decisions made overnight, reducing the need for late-night or early-morning calls. While these basic functions – note-taking, recordings, transcripts, and summaries – might sound mundane, they’re incredibly impactful. If you can remove those repetitive tasks, it frees people up to focus on more innovative and strategic work.
Interestingly, the features people use the most are often the simplest ones because they save so much time and effort. I always say, when in doubt, start with the boring things. There’s a lot of them, and they often deliver the quickest wins.
We’re all so stretched thin, especially since COVID. We once had boundaries, like commuting time, to separate work and personal life. Now, with remote work, we start earlier, finish later, and the workload has only increased.
AI can help lighten that load. For most employees, that’s the key: reducing the overwhelming workload so they can focus on what they enjoy and the work that made them choose their career in the first place.
MAHA REINECKE is a seasoned professional in digital transformation, product development, and data analytics with over a decade of global experience in both startups and multinational corporations. She is currently the Executive Director of Data, Analytics and AI at Reinsurance Group of America, where she champions and accelerates the adoption of enterprise-wide data strategies and data platforms to drive efficiencies across the business, and the development and delivery of data products. Additionally, Maha spearheads the implementation of a data academy to enable RGA’s teams to enhance their data analysis skills, and supports the development of RGA’s global data strategy. She previously held positions at UBS Group AG and Logex Healthcare Analytics, where she focused on the development and implementation of data analytics products. In her native Singapore, Maha has extensive experience in digital transformation and healthcare analytics in the public and private healthcare industry.
Could you share some key milestones in your career that led you to your current role as Director of Data Analytics and AI at RGA?
My journey began with my first job, where I worked with a development team in a public hospital in Singapore. We were tasked with building a digitally integrated hospital by implementing electronic health record systems.
I was involved in defining requirements and collaborating with stakeholders, while considering the kind of data we needed to collect to analyse hospital operations and support decision-making. This was before the term ‘data-driven’ became widely used. Over time, I transitioned from planning and implementation to analysing data, identifying trends, and providing insights that helped streamline hospital processes.
That experience made me realise the potential of data in driving efficiency and innovation. I saw that data analytics would play an increasingly critical role in the future, which led me to change my career path towards data & analytics – both through on-the-job learning and formal courses – and that led me to where I am today.
Were there any significant events that served as stepping stones to your current leadership position?
After leaving Singapore and moving to London, I started engaging with startups. It was a new market and environment for me, so I focused on understanding how companies operated in this region. Connecting with founders and startup teams helped me appreciate their approach to problem-solving and innovation, and I started to collaborate on small projects, assisting with data analysis to understand market trends better. Coming from a healthcare background, working for a data product company in the healthcare space was a logical and natural choice. My domain knowledge and experience in implementing solutions positioned me well to contribute effectively.
This exposure to the entrepreneurial and startup ecosystem was eye-opening. In Singapore, I hadn’t seen innovation at this scale, and moving to London broadened my perspective on application development and data-driven decision-making.
What inspired you to move from a startup environment to a corporate one?
I’ve always worked in small businesses. Even in the hospital setting, whether public or private, it was a localised environment. I transitioned from public to private and then to a product company. By the time I made that shift, I had spent about ten years in healthcare. I wanted to test a hypothesis: Are data skills truly industry-neutral? Can I pivot to another field, apply my skills, and pick up subject matter knowledge on the job? The answer turned out to be yes.
Additionally, having always worked in smaller companies, I felt I was missing the exposure to a corporate culture. That led me to financial services. My long-term vision is to work towards a COO role because I see a strong alignment between operations, data, and management. My career path hasn’t been linear; I didn’t graduate with a data science degree. Ten years ago, such degrees didn’t even exist. Everything I learned was through real-world business contexts and data applications. That background shaped my decision to shift to corporate.
At the same time, I still value and apply the agility and innovative mindset I developed in startups and small businesses. I think that perspective is appreciated in corporate environments, where large-scale projects can sometimes become bogged down in bureaucracy or long-established but ultimately outdated processes.
How have the challenges you’ve faced shaped your leadership style?
I’ve always found myself in greenfield opportunities – every company and project I’ve worked on involved building and implementing something new or testing new ideas. That experience has made me resilient but also enhanced my solutions-oriented mindset and the need to collaborate and convince stakeholders.
I’m also not afraid of failure. My approach has always been to find solutions using the best possible resources available. If something doesn’t work, I pivot. Change doesn’t feel disruptive to me because I see it as part of the process of innovation and problem-solving.
Could you give us an overview of RGA’s journey in digital transformation, within what is a fairly mature sector?
RGA is a reinsurance company, meaning we provide insurance to insurance companies. We’re a relatively young company in the insurance industry – only 51 years old – but we have grown significantly in the last five years. Our business volumes have expanded rapidly, and it’s important for our internal operations, including in data management, to keep pace.
During this growth phase, we realised that we successfully managed the expanding workload, and identified areas where we could optimise data processes. We saw potential to reduce fragmentation, streamline workflows, and establish clear ownership structures. It was as if we had all the right ingredients and were now ready to refine our recipe. This realisation inspired us to evolve our approach, focusing on increased coordination and strategic methodology to better serve our growing business needs.
As an insurance company, risk is our business. We want our time to be spent on deriving insights rather than managing upstream data processes. Currently, the reality in data is that 80% of the effort goes into
collection, storage, and transformation, with only 20% spent on insights. Our goal is to flip that ratio, or at least reduce the time spent on backend processes.
The key objectives of our data strategy are to standardise our data frameworks and address challenges related to people, processes, and technology with the goal to enable the business to spend more time generating insights rather than processing data.
We’ve invested heavily in technology and data governance, and it’s an ongoing journey. We continually reassess how to improve our organisational structure, attract more talent to work with data across the company, and ensure our approach caters to different business needs.
What have been the most significant advancements at RGA in terms of that journey?
After the enterprise data strategy was conceived and communicated, we began building the enterprise data platform. This platform is designed to enable self-service analytics, distributed ownership, and federated governance.
The platform is constantly evolving, which is a significant achievement. We’re moving away from data sitting in silos within individual business units. Instead, we’re enabling cross-domain analysis, reducing duplicative and repetitive processes. As a result, the number of data products we utilise is growing, with each product having a dedicated owner who ensures proper documentation and governance. And more and more business units are getting on board, eager to create and share data assets with their consumers.
We’re also strengthening our data governance practices, including developing an enterprise data catalogue and improving data quality management. And while we have developed a framework for distributed ownership, it is now about ensuring the business units adopt it effectively. For example, what does it mean to be a data product owner, a data steward? Since this role is in addition to a person’s day job, we need to define responsibilities clearly and support those taking on these governance roles.
We want our time to be spent on deriving insights rather than managing upstream data processes.
Do you have different classes of data products at RGA?
There’s a lot of debate around what constitutes a data product but we haven’t imposed strict definitions. Currently, most data products are tables or data assets published in our marketplace or access repositories, allowing consumers to request access.
Beyond that, these data assets can be leveraged for analysis, and dashboards or reports built from these
assets can also be considered data products. We focus on providing the foundational building blocks that enable various forms of data utilisation.
Some users are data-savvy and require direct access to datasets, provided the right governance and security controls are in place to ensure appropriate data access. Others need automated pipelines where data flows seamlessly into dashboards, allowing them to monitor changes without handling raw data themselves. Since every business unit has different needs, our goal is to support all these use cases effectively.
How has your experience as a product manager influenced your approach to data and AI at RGA? I always say I am a data analyst first, who then gained exposure to product management. The way the industry has evolved has allowed me to merge both skill sets. My background in chemistry involved analysing large datasets, and that analytical mindset carries over to my work in data and AI. Product management, on the other hand, is a methodology I’ve learned that helps me apply structured thinking to my work.
One key principle I follow is not relying on a single perspective. Whether it’s interpreting data, designing an architecture, or structuring a workflow, I always compare multiple approaches before deciding on the best path forward.
Another important rule I follow is to never act impulsively. When people come with urgent requests, it’s easy to react immediately, but I’ve learned to apply reasoning first. Impulsive actions often stem from inadequate knowledge, so it’s crucial to take a step back and assess the full context before making decisions.
The concept of a data product also influences my approach. Unlike a project, which has a defined endpoint, a product must continuously deliver value at scale. Whether I’m creating a dashboard, sharing data assets, or designing tables, I always think about its role within the broader business and data ecosystem. Understanding the end users and their needs is critical – rather than just executing tasks, I focus on what they’re ultimately trying to achieve.
Effective data and AI strategy isn’t just about technology and tools – it’s also about streamlining processes. Technology provides the necessary uplift, but true value comes from the intersection of technical expertise, domain understanding, and user empathy. That overlap is where real impact happens.
Are there any specific methods or frameworks from product management that you’ve found effective in your current role?
Prioritising and breaking down the problem is key. One framework I frequently use is the ‘five whys’ methodology, originally developed by Sakichi Toyoda, the founder of Toyota Industries. Asking ‘why’ five
times helps uncover the root cause of a problem. Often, when people present multiple issues, they all stem from a single underlying cause – such as an incorrect data model or poorly structured data.
By addressing the root problem, I can implement a single solution rather than creating multiple workarounds. Always ask ‘why’ multiple times to determine what is truly important versus simply lifting and shifting an existing process.
User empathy is also crucial: who are the consumers, what are their needs, and how will they use the product? Will it effectively serve multiple users rather than just one? The goal is to develop and deliver at scale while reducing variation.
Often, when people say they want to move to the cloud, they see it as a project. But from a product management perspective, moving to the cloud is just a step, not the goal itself. My focus is always on the underlying problem – are we aiming for better compute and storage, or is the goal improved data accessibility and performance efficiency?
Another key approach is reviewing, refining, and iterating. Work in increments rather than committing to large-scale, long-term projects. Traditional financial institutions often plan three-to-five-year initiatives, whereas I normally work within a six-month roadmap. This approach allows me to be agile and adapt to new developments and innovations in the industry.
How has your product management background influenced how you prioritise projects within RGA’s initiatives?
This is where business context becomes crucial –aligning projects with business KPIs. It’s not about how many products I’ve published or how many requests I’ve serviced, but whether I’ve delivered a product that moves a business metric.
For example, if a team’s main challenge is inefficient data processing – spending too much time gathering, transforming, and storing data – then improving that workflow becomes a priority. Rather than focusing on arbitrary output metrics, I align my work with broader organisational goals.
Last year, RGA introduced a refreshed strategy that includes key elements such as an enterprise-first mindset, adaptability to change, and enabling worldclass functions (HR, administration, operations, data, and technology), which resonates deeply with me. My work directly supports these objectives.
Can you share an example where your product management approach directly contributed to the success of a data or AI project at RGA?
One project involves addressing fragmented data sources and redundant processing. We found that multiple teams were using the same data source but
generating several outputs. That inefficiency prompted me to investigate. Who are the consumers, what do they truly need, and why are so many versions necessary?
By analysing these issues, we realised that the data model itself could be simplified. Instead of running separate queries for each request, we are working on exposing data at a more granular level with proper governance and access controls. This way, users can generate their own outputs dynamically rather than relying on predefined reports.
This shift aligns with our broader goal of self-service analytics – enabling users to query and interpret data independently rather than submitting repeated service desk requests. The aim is to reduce manual ad hoc reports and streamline operations, freeing up the development team from handling routine data dumps and requests.
This project’s shaping to be a major step towards making data more accessible while optimising internal workloads.
How does your team handle the translation of data insights into strategic business decisions? Has your product management background influenced this process?
One key principle I follow is ensuring that my development team produces an outcome that allows the end user to enhance their decision-making ability. We do not want to build something simply because it was requested or has been used in the past, but we engage with the business domain experts and subject matter specialists early on to ensure we understand what they are trying to achieve. You could call it ‘beginning with the end’.
Using agile practices, we ensure that the solutions we develop remain modular and can easily adapt to changing business needs. Since business requirements evolve, having this iterative process helps us refine solutions within a short timeframe. Ultimately, we aim for the end user to be in a better position to gain data insights that will then translate into business decisions.
As part of RGA’s transformation efforts, you set up an internal Data Skills Academy. What motivated that initiative?
When I joined RGA, we were already a few years into our enterprise data strategy. Significant investments had been made in tools and technology, but we needed to increase awareness and adoption across the company. Many employees were unfamiliar with the tools and felt intimidated by them.
Having previously developed a similar program in another organisation, I saw the need to create a structured approach to upskilling employees. Without proper training and support, these investments would remain underutilised.
Some employees showed a strong interest in learning how to use these tools but lacked the guidance to do so effectively. Many found traditional upskilling methods – such as one-day workshops – too disconnected from their actual work. To bridge this gap, we designed a program that aligns directly with their job functions, providing hands-on learning opportunities.
By building the Data Skills Academy, we aim to help employees adopt and integrate these tools into their workflows, ultimately driving the success of our enterprise data strategy. This initiative ensures that our workforce is empowered to leverage data effectively, rather than relying on fragmented or siloed approaches.
Was the Academy aimed at certain roles or business areas?
The Academy was developed for everyone. Using my product management approach, I engaged with users across different business functions – leaders, middle management, and analysts – to understand their challenges, experiences with tools and technology, and learning preferences.
Through these conversations, I identified different user personas within RGA, recognising that each business unit has unique needs. This led to the development of an operating model that aligned with our enterprise strategy, which emphasises a winning mindset and the need to embrace change.
The core pillars of the operating model focused on curating tailored learning pathways based on user personas and job roles. The goal was not simply to have a certain number of employees learn Python by 2026. Instead, the focus was on providing content that aligned with their daily work and RGA’s technology stack.
For example, if someone primarily used Excel, the objective was not to push them towards Python but to enhance their skills with Power Pivot and Power Query to automate repetitive tasks. Many users were unaware of these built-in Excel features, which could significantly improve their efficiency.
For those with some coding knowledge, the focus was on advancing their skills – learning CI/CD, source control, and GitHub, ensuring they could leverage existing tools effectively.
Additionally, many actuaries, particularly those in the process of qualification, had backgrounds in mathematics, computer science, or econometrics, giving them some exposure to programming. The Academy allowed them to apply their academic experience at a practical level within their job roles.
Ensuring that our content and training providers catered to these varied personas was a crucial element of the Academy’s design.
What impact has the Academy had on the transformation journey as a whole, and have you been able to measure that impact?
Because it’s early days since we have implemented the Data Academy in RGA, most of the data we are collecting is related to user behaviour such as active user, time spent, courses completed, etc. That gives us some base level KPIs, which will help us improve the content and program as a whole. In addition, we collect qualitative data in the form of one-on-one feedback sessions, surveys, etc. to gain real user insights into how the platform is helping RGA colleagues.
For example, someone mentioned taking an Alteryx course on DataCamp and using that knowledge to refine a workflow. What previously took a full day of manual manipulation in Excel now takes just a few minutes in Alteryx. That’s a tangible success story.
I’ve also received messages from employees who appreciate the tools and training. Many have realised that learning about data isn’t just about coding; it’s also improved their ability to write better prompts for our internal generative AI tool. This has helped them get more accurate and useful responses to their enquiries.
We’re still early in this journey. Last year’s small pilot programs demonstrated strong demand and clear benefits, so this year we’re expanding the program enterprise-wide, with a focus on scaling the initiative and formally measuring its impact. Our goal is to align the Academy’s success with the broader enterprise data strategy and quantify the value of investing in data upskilling.
Looking back, what advice would you give to your younger self at the start of your career?
I would say to put myself out there more – beyond just my day job. Engaging with the professional community in my field would have been beneficial.
Also, I’d tell myself to take more risks and seek out opportunities earlier. I used to think I had to achieve certain milestones – another qualification, more experience, or a specific project – before pursuing what I really wanted.
Instead of letting these self-imposed barriers hold me back, I should have trusted my ability to navigate challenges. Because when faced with difficulties, I’ve always found a way to overcome them through diligence, persistence and perseverance.
By building the Data Skills Academy, we aim to help employees adopt and integrate these tools into their workflows, ultimately driving the success of our enterprise data strategy.
STEFANIE BABKA is a marketer with a second diploma in public relations, who is fascinated by the interface between people and technology. She has over 20 years’ experience in digital marketing, public relations and digital transformation in different industries (automotive, FMCG, science & technology), and for the last 10 years she’s led teams of different sizes.
Stefanie has published two books on social media, and as a pivotal employee of major corporations, she’s organised and supported countless big events, including IAA, Stars & Cars, Autosalon Geneva and Fashion Week. She’s launched more than 50 digital products, apps and websites, and ran campaigns and projects for more than 60 brands such as Mercedes, Smart, Dodge, Saab, Kit Kat, Wagner Pizza, Maggi, Beba, Purina, Perrier and Vittel.
At Merck, she led various big transformation projects, including the introduction of the digital workplace EVA (consolidating 160 intranets into one) and the change and comms stream of the Group Data Strategy. She’s also supported rebranding and the integration of newly acquired companies.
As the Global Head of Data Culture, Stefanie is responsible for communication, change management and upskilling programs on data and AI, including the Merck Data & Digital Academy and their enterprise-wide data & digital community. Stefanie is passionate about bringing together artificial intelligence and human intelligence to drive meaningful insights and data-driven decision-making.
STEFANIE BABKA
Stefanie, you have an interesting title. Tell us about yourself, how you stepped into this role and what you do as ‘Global Head of Data Culture’.
When we first established an enterprise-wide data strategy at Merck I led the Change & Communication workstream, and this is how I grew into this topic. As a result of this strategy, the Merck Data & AI Organisation – including a role for data culture – was established. And I was happy to take that role. This was, and still is, a perfect mission for me: I have a background in digital marketing and my data love comes from customer data. I have seen the power data can unlock if you target someone in the perfect perception moment with the perfectly tailored message.
Today, my team and I run the group-wide data & AI upskilling and change management initiatives at Merck to enhance the data culture in our company.
Merck is recognised as a leader in establishing a datadriven culture within a large corporation. Could you elaborate on the strategies used to develop a strong data culture within your organisation?
To build a strong data culture I always say we need to win the head, the heart, and the herd.
For winning the head, we focus on fostering the right skillset among our employees. This can be done by upskilling, reskilling or hiring. For upskilling we have two main approaches. The first is what we call ‘curiosity-sparked learning.’ This approach allows employees to find a starting point in a moment of curiosity and begin their learning journey. They can select their background, skill level, time budget, and topic of interest to find suitable training options in our comprehensive training hub. This flexibility empowers employees to take control of their learning at their own pace.
For those who prefer a more structured learning path, we offer guided learning programs. These range from simple data & AI literacy paths to our flagship program, Fast Track Upskilling for Data and Digital. This intensive program offers up to 10 weeks of full-time training, enabling participants to become what we call ‘citizen data scientists’. The beauty of this program lies in its practical approach – participants bring in their own projects, which allows them to tackle real business challenges or opportunities using real data. Additionally, each participant is paired with a mentor to ensure that by the end of the training, they not only gain knowledge and skills but also develop their own data or AI solution.
Winning the heart involves engaging our employees emotionally. We do this by creating a data appetite through storytelling and fun activities such as our Data Escape Room. These initiatives make learning enjoyable and memorable, helping employees see the value of data in a relatable way.
Lastly, for winning the herd, we emphasise the importance of community. We foster communities of practice to enhance exchange and learning across sectors and functions. This approach ensures that data culture is deeply rooted in our organisational practices, promoting collaboration and continuous improvement. By addressing these three areas – head, heart, and herd – we create a comprehensive strategy that embeds data culture throughout the organisation.
Can you share examples of successful initiatives that have improved data-driven decision-making at Merck? Certainly! One of our standout initiatives is our internal everyday AI companion, which we call myGPT Suite It is available to all employees to foster generative AI literacy at scale. It has quickly become one of the most used tools at Merck, allowing employees to save significant time in their daily tasks. These initiatives exemplify how data and AI are transforming our decision-making processes at Merck, allowing us to deliver innovative solutions more efficiently.
How do you measure the effectiveness of data literacy programs across different teams?
We use a combination of qualitative and quantitative metrics to measure effectiveness. Qualitatively, we gather insights from employee engagement surveys focused on winning the head (Do you feel you have the right skills?), heart (Are you looking forward to working with data & AI more?), and herd (Are you applying data-driven ways of working in your team?), along with training evaluations and feedback surveys.
Quantitatively, we rely on system data, such as the number of use cases in production within Foundry and the monthly user statistics for our everyday AI companion myGPT Suite. Notably, myGPT Suite ranks among the top 10 most used tools at Merck, even without including any mandatory services, indicating its widespread adoption and utility.
By addressing these three areas –head, heart, and herd – we create a comprehensive strategy that embeds data culture throughout the organisation.
STEFANIE BABKA
How do you ensure that employees at all levels understand the importance of data in their roles?
We’ve developed a comprehensive Data Culture Concept that targets five distinct groups within the organisation: executives, managers, data & AI practitioners, everyone at Merck, and physical operations (production and lab personnel) . This plan utilises different angles to spread ‘data love’ across the company. Executives create top-down excitement, while practitioners and enthusiasts are equipped with materials, tools, and arguments to foster a bottom-up data culture. By addressing the specific needs of each group, we ensure that every employee understands the
pivotal role data plays in their work and in the broader success of Merck.
How do you stay updated on the latest trends and technologies in data and AI?
Staying updated is crucial in our fast-evolving field, and I engage in a variety of activities to ensure our training remains up to date. This includes reading industry news, listening to relevant podcasts, participating in training sessions, and collaborating with our tech teams. This multifaceted approach allows me to keep abreast of the latest developments and ensures that we continuously integrate cutting-edge innovations into our data culture strategy.
If you could build precise and explainable AI models without risk of mistakes, what would you build? Rainbird solves the limitations of generative AI for high-stakes applications.
TAMANNA HAQUE
Lead data scientist at Jaguar Land Rover TAMANNA HAQUE talks about her latest challenge: motherhood. As a powerhouse in AI, itself an ever-progressive field, Tamanna reveals how she maintains a dynamic mode to stay ahead at work and the new road at home.
Tamanna’s journey with JLR started long before she was employed by them. Since childhood, she’s been passionate about the Jaguar brand, writing about it, attending previews, going to experience days and more. Jaguars turned her head, with their attractive, luxurious and powerful presence. She was fortunate to be a fan-turned-customer at 22, later becoming an employee at 25.
When Tamanna joined JLR in 2019, it was
I am in ‘Jaguar’ mode all the time!
Were there any tools, technologies, or strategies that helped you stay organised and connected during your maternity leave?
‘Keeping in touch’ time was valuable in helping me maintain contact with work and ease the transition back.
A lot of routine was gone initially, so I had to be smart and effective about the little ‘keeping in touch’ time I wanted to give my work life. A lot of preplanning and deciding what was worth that small fraction of time helped me to stay on top of things. Otherwise, nearly all of my time was spent on growing my baby… it is quite something getting to grips with parenting for the first time! My daughter is my top priority and I also made sure to focus on myself and my recovery.
To give a clearer picture of what I had to plan for and organise around, I’ll provide some insight into my role. I am constantly balancing line management, idea generation, stakeholder buyin, project to product delivery, technical advisory and more for my AI work, with a passionate,
as an original team member of the Vehicle Connected Data Science team. She enjoyed the unexpected challenge of shaping a new AI team (now nearly 40-strong across multiple countries) and jumping into the world of the connected vehicle, which creates interesting data and futuristic data science work.
Tamanna was soon promoted to lead data scientist, leading a cross-country team called ‘AI-PACE’ who are delivering customer-facing AI products at pace for the end-to-end vehicle experience. She is constantly engaging in a wealth of activities outside her role which ‘give back’ whilst helping her to find new limits to serve her clients better; she feels personally committed to them, having originated as one.
customer-first focus. I am in ‘Jaguar’ mode all the time! I often have a multitude of projects on the go and enjoy the pace.
Before leaving for maternity, I agreed roadmaps with my key stakeholders and had work planned to a fine detail. I occasionally checked in to field new ideas or step in if there were any impediments to delivery. For my team, I created an idea bank, reserved for them to explore in case their bandwidth allowed it. The roadmaps, explorations and skill-building tasks were carefully considered and tied into their development whilst adding value to the business.
There were personal activities (outside of my role, some outside my capacity at JLR) which I lined up to pick up, such as the Women in AI network (which I founded and chair at JLR), guest lecturing, patent filings, events I’d be speaking at, and more. The world of AI is more fast-paced than ever, and I kept up with this and with my industry (automotive), to keep on the ball creatively. These activities kept my soft skills, network and technical knowledge fresh, and I’m now much more selective about events I support or learning I embark on. Time is precious!
Staying connected in a very lightweight but purposeful manner worked for me, but I appreciated having the choice to totally switch off (as this can be less of a choice for others).
What was the most rewarding aspect of navigating motherhood alongside your career?
The most rewarding aspect is that each side enriches the other! As
TAMANNA HAQUE
a new mum I have picked up a lot of new skills which help me to be a better data science leader. For instance, handling new or unexpected situations at home has made me more adaptable –much like the essence of machine learning. I’m constantly improving, thinking more critically and faster on my feet in any setting.
Things have been quite full-on at times, especially in the initial weeks. Unpredictability and added responsibilities meant I needed to refine my time management and prioritisation skills, which reflects at work where I’m much more value-driven and efficient. Something has to be great to get my attention! The AI products we’re delivering are more innovative, appealing and valuable for the business and for our clients than ever before. Back to my personal life, I’m adopting the use of more AI to increase productivity; a simple example is voice dictation of messages. Generally, AI has helped me to capitalise on my time, and better keep up to date with my family, friends and network.
I also have a perspective about our luxurious vehicles as a parent now! I always stay up to date with our products, as this gives me a baseline on the vehicle experiences we offer today, and feeds into inspiration for how we can use AI to offer even better experiences tomorrow. With my personal perspective now broadened, I’m seeing some of our cars a bit differently and I’m loving some of them more than ever. My daughter and I are appreciating the Defender at the minute: we’re getting around with lots of space and capability from the iconic 4x4.
My previous car, the Jaguar F-TYPE, was a two-seater sports car. Very different, and enjoyed during a different phase of my life. The more product and personal perspectives I gain myself – or learn from our customers – helps me to create AI with ‘customer love’ and ultimately better their experiences.
I couldn’t have navigated motherhood and my career together without my amazing husband and family. I had lots of valuable support (which felt like training at times!) and daily personal time which helped me to show up as my best self in every setting, despite being a new mum.
Did you discover anything about yourself during this time that surprised you, either as a mother or as a data science leader?
Yes, the fact there are parallels in my approach to being a mum and being a data science lead. I now see how my approach to people and leadership probably touched on some natural maternal instincts I had already – the high attention I put on my team’s development, and skills such as teaching, emotional intelligence, compassion, time management, adaptability, planning and more. All of these qualities are exaggerated during parenting and I’m really enjoying growing a mini-me!
Has becoming a mother changed the way you lead your team?
Yes, it’s reinforced my beliefs about modern ways of working and also made me a better leader.
I lead with a great deal of energy, commitment and passion for our products, customers and business, always thinking about new ways
The more product and personal perspectives I gain myself – or learn from our customers – helps me to create AI with ‘customer love’ and ultimately better their experiences.
I can add value to the business, keeping up with trends in AI to support. This maintains a great feeling of pace and purpose in my squad and I strive to provide a working environment that I would have wanted for myself, serving up motivation to deliver quality results just like our SVs (Special Vehicles)!
Data science is quite demanding, so I support working in a way which optimises for what really matters – real, tangible value-add. Working in and now leading a cross-country team in tech and data science, I promote flexiblity, trust and productivity-led working to get lots of great things done! We are constantly delivering and creating use cases alongside building up our capabilities to deliver cutting-edge AI. Our working style therefore needs to be just as dynamic, helping to promote more success with our AI products, as well as more diversity and inclusion. Meetings or facetime are scheduled meaningfully. This way I’m saving
TAMANNA HAQUE
energy which is channelled back into my brain and I’m more healthy, happy and productive than ever.
I’m saving time which I can give to my daughter. Flexibility helps both sides, since some of my best thoughts have come up after-hours. Overall, I have the best work-life balance I’ve ever had, doing the best work I’ve ever done, and I’m obviously going to keep pursuing this to support myself and others around me.
What advice would you give to women planning their maternity leave in similarly fast-paced industries?
It’s really important to plan thoroughly before you leave for maternity; every baby, birth and postpartum experience is unique, and these are obviously your top priorities, so it’s hard to predict your ability or desire to ‘keep in touch’.
I would plan as if you were hoping to completely step away
during your maternity leave. A roadmap with project goals and agreements, key contacts, areas of support needed etc., will help your team and stakeholders to stay on track with deliverables. Providing personalised guidance to each team member about what you want them to learn over the period – as well as deliver – means you can help their development to keep progressing. Centralising all of this information is useful, so that relevant colleagues are empowered to know what you’re thinking and expecting, even when you’re not present!
There are ways to keep up with people and movements without opening your laptop and it’s also great to catch up socially sometimes.
Remember, there’s no need to stay connected with anything other than your baby, so plan really well to give yourself reassurance and to keep things moving (and moving the way you want them to). It’s true you won’t get the time back!
DIDEM UN ATES
is a renowned AI and Responsible AI executive with over 25 years of experience in management consulting (Capgemini, EY, Accenture) and industry roles (Motorola, Microsoft, Schneider Electric). As the founder of LotusAI Ltd, she advises private equity firms, hedge funds and financial institutions, including Goldman Sachs Asset Management and its portfolio companies, on AI/GenAI strategies, responsible AI implementation, and talent transformation.
Didem serves as Advisor and member of the AI/ Generative AI Council for Goldman Sachs Value Accelerator, sits on the Boards of the Edge AI Foundation and Wharton AI Studio, and is a certified AI Algorithm Auditor and Executive Coach. Her industry contributions have earned her recognition such as the Tech Women 100 Champion and Trailblazer 50 awards. In her tenure as Vice President of AI Strategy & Innovation at Schneider Electric, she led the development of the company’s AI/GenAI strategy and forged strategic partnerships with startups, VCs/PEs, and academia. At Microsoft, she was pivotal in launching Business AI Solutions at Microsoft Research and spearheaded diversity-focused programs, including Alice Envisions the Future / Girls in AI hackathons. In her role as head of Applied Strategy, Data & AI in Microsoft’s Chief Data Office, she oversaw Microsoft’s largest AI engagements, contributing to the OpenAI partnership.
Didem has led the operationalisation of Responsible AI at Microsoft, Accenture, and Schneider Electric, advising C-suite leaders on talent strategy adaptations and promoting responsible AI practices.
Can you tell us about your early experiences in the AI sector and how they shaped your career path, particularly during your time at Microsoft?
I’ve spent over 26 years in the technology sector, focusing mainly on disruptive technologies. For the past 13 years, I’ve been deeply involved in AI.
I witnessed the rise of traditional AI while I was working with Microsoft Ventures and Accelerators. Across accelerators worldwide, 80–90% of the highestgrowth startups were AI-focused. That’s when I realised AI was where I wanted to be. Steven Guggenheimer, a VP at Microsoft at that time, sent an email about forming a Business AI team. I offered to volunteer for competitive benchmarking and startup ecosystem projects. That volunteer role turned into my first official position in AI.
I was part of Microsoft’s first Business AI team, which originated within Microsoft Research. Our team had about 100 members, and I was the only person based outside the U.S. – in fact, outside of Redmond, the headquarters. I was also the only Turkish woman in a predominantly male team, consisting mostly of Chinese, French, and Canadian men. I highlight this because, although I was accustomed to limited diversity in tech, the lack of diversity in AI felt even more pronounced. It motivated me to take intentional action, and that’s how the initiative Alice Envisions the Future / Girls in AI started.
At Microsoft Research, we developed some of the first enterprise AI algorithms for customer service, sales, and marketing. When these proved successful, our team transitioned to the AI Engineering division to launch them as official products. This effort led to the creation of Microsoft’s first AI SaaS platform: Power Platform. I call it the ‘grandparent’ of the Co-
Pilot suite, because it shares a similar architecture and logic, later enhanced with generative AI.
Fast-forward 12 years at Microsoft, and my final role involved working with the Chief Data Officer on large-scale AI engagements with Fortune 500 clients. One such collaboration was with Schneider Electric, who later invited me to implement their AI strategy.
Now, through my own company, LotusAI, I help organisations like Goldman Sachs and private equity firms, hedge funds, and other financial institutions with AI transformation, responsible AI strategies, and talent development. It’s been an incredible journey, combining proactive volunteering, seizing opportunities, and pursuing cutting-edge technology – not because it’s trendy, but because it has a massive power to drive positive societal change at scale.
Can you share pivotal moments from your career that shaped your approach to AI strategy and leadership?
incredible to see.
Another defining moment was attending my first offsite meeting with our team of approximately 100 members. I was the only woman and the only person based outside the U.S.
I hadn’t set out to be a leader in diversity and inclusion, but I knew someone had to address the issue. Diversity isn’t just a social responsibility; it’s essential for business success, especially when developing complex products like AI. Alice Envisions the Future / Girls in AI , which eventually became a global program, has connected over 8,000 girls worldwide through a Facebook group. High school girls in cities like Seattle, Warsaw, London, Athens, and Istanbul began hosting hackathons, sometimes even independently at their schools, and Microsoft partners such as Accenture and KPMG supported these events.
Diversity isn’t just a social responsibility; it’s essential for business success, especially when developing complex products like AI.
One pivotal moment was when I brought Microsoft its fourth paying AI customer. Today, we talk about AI generating trillions in market value, but back then, AI was still in its early days. With that customer, we made a bold decision: instead of selling the AI product as a managed service – where we could only serve a few large customers per year –we decided to package it as a SaaS AI solution.
This required us to pivot our entire business model just six months before the product’s general availability, and ultimately led to the creation of Microsoft’s Power Platform as a SaaS offering. It wasn’t just pivotal for me personally but also for Microsoft and the broader tech industry. Today, millions of developers build on that platform, which is
It’s one of the most meaningful parts of my career – doing something positive for society while scaling AI in my day job. Many of these young women stay in touch with me, and I continue supporting them with recommendation letters for their university applications. It might be a small step, but it means a lot to me.
A third pivotal moment happened recently, after I left Schneider Electric in mid-November. Goldman Sachs’ asset management team had invited me to join their new Generative AI Council of seven or eight experts from around the world. It was intended as a board-like role, requiring just a few hours a month. But two days later they reached out again. They’d seen my LinkedIn post announcing my departure from
Schneider Electric and asked if I would consider a broader role. They had invested in hundreds of portfolio companies, many of which needed AI support, and they wanted help at the value accelerator level.
So, while waiting for a friend who was late to lunch, I officially launched my AI advisory business, LotusAI, on December 13, 2023. It was a spontaneous start, but it’s turned into the most fulfilling phase of my professional life, and I’m now helping many clients with their AI journeys. As much as I enjoyed my time at Microsoft and other companies, this is the best time of my career. I always wanted to be an entrepreneur, and everything just aligned perfectly: clients were ready, product (AI) was in demand, and the timing was right.
What are some common misconceptions enterprise organisations have about implementing generative AI?
The first, and perhaps the most important, relates to culture and mindset. From an enterprise transformation perspective –especially with generative AI – things move so fast that the real starting point is leadership. There needs to be a leader at the top with vision, courage, and an investment mindset.
This investment isn’t just financial or resource-based; it’s also about people and time. Leaders must commit to the journey, knowing it won’t be easy. They must accept that there will be failures, but these are part of the learning process. Ultimately, there’s no turning back – generative AI isn’t just a tool for survival but for thriving in the future.
Another major misconception is that many organisations start with a small proof of concept (POC) – a use case that’s tangential to their core business. They do this because they either lack the leadership sponsorship to take a holistic approach, or fear the complexity of full-scale adoption.
While a POC might work, the biggest pitfall is that these isolated experiments rarely scale. If AI initiatives aren’t integrated into the core business strategy and work flows from the start, they remain stuck in the lab, failing to generate real impact. Later, organisations conclude that AI doesn’t work or doesn’t scale, but the reality is that the approach wasn’t designed for success in the first place.
Generative AI requires a holistic approach – an end-toend transformation that includes talent development, responsible AI practices, and integration across every function, from HR to finance. It doesn’t all have to happen simultaneously, but the vision must be comprehensive.
Another misconception revolves around data. With advancements like synthetic data, automation, and new methodologies, many data challenges can now be overcome. Organisations shouldn’t let data concerns hold them back from exploring generative AI solutions.
Lastly and most importantly, there’s the issue of talent and execution. Many companies hire consultants for time-boxed projects – eight, twelve, or sixteen weeks –to develop a strategy or implement a few use cases. These projects often end with hundreds of slides or complex Excel models. But once the consultants leave, internal teams are left catching up on their day jobs they had to put on hold for weeks, and the AI strategy sits idle because there’s no internal capability to execute it.
To avoid this, organisations need a hybrid approach: a balance of in-house expertise, strategic
partnerships, and external advisors. Most importantly, there should be a ‘chaperone’ figure – someone who can walk the journey with the organisation at its own pace, ensuring the transformation is sustainable and scalable. Such a figure is also helpful in terms of having an objective outsider who is not part of internal politics and can candidly point to the North Star –sometimes playing the ‘good cop’ and other times the ‘bad cop.’
In your opinion, is a chief AI officer needed to ensure AI initiatives will scale and have the desired impact? Many AI initiatives sit within the CIO or CTO organisation. The core mission of these departments is often to ‘keep the lights on.’
As a result, AI projects become low priority. This is a key reason why these projects don’t scale or deliver impact.
On top of that, when AI initiatives are placed in these departments, employees often don’t even have access to generative AI tools because relevant websites are blocked. I’ve seen employees transfer company data to their personal PCs so they can work with AI tools like ChatGPT at home. This is a nightmare scenario for CIOs and CTOs.
I do believe the chief AI officer role is essential, but it must operate closely with other C-level leaders. If the role ends up merely coordinating AI efforts in a hub-and-spoke model, it becomes ineffective.
If you already have a chief digital officer, a CTO, and a CIO, adding a chief AI officer can cause overlaps and conflicts. Therefore, this role needs to be clearly defined and strategically positioned. The visionary leader driving AI doesn’t need to understand all the technical details, but they must believe in AI’s transformative potential and communicate that belief effectively.
Another critical misconception lies in language, communication, and change management. How
leaders frame AI adoption matters immensely. For example, in one of the largest German organisations I worked with, a new CEO wanted to make an impact. At major global conferences, he repeatedly said, ‘I’m going to save X billion dollars with AI,’ framing AI purely as a cost-cutting tool.
When developing an end-to-end AI transformation roadmap, the projected business impact is often quoted between 20–40%. This could affect top-line revenue, bottom-line profit, EBITDA, or a combination of these.
The visionary leader driving AI doesn’t need to understand all the technical details, but they must believe in AI’s transformative potential and communicate that belief effectively.
I gave feedback that this messaging was counterproductive. If employees hear that AI is primarily about cost-cutting, they will resist it with everything they have. Instead, leaders should present AI as an opportunity for growth and empowerment.
The conversation should be: ‘This is the future. There’s no turning back. But we’ll work with you. We’ll co-create talent transformation maps, personal development plans, and team growth strategies. How can you imagine your job being augmented by AI? How can AI help you add more value? We’ll upskill and reskill you. And in the process, you might save enough time to finally take your evenings or weekends off.’
In industries like financial services, this is already becoming a reality. In R&D organisations, tools like GitHub Copilot accelerate time to value for research projects. Ultimately, how we articulate the opportunity AI presents – how we inspire and address employees’ concerns – will determine whether AI strategies succeed or fail.
What are the most critical factors that determine success or failure when implementing an enterprisewide AI strategy?
The most critical factor, without a doubt, is talent transformation.
Naturally, this excites CFOs and board members. However, I always caution them: this doesn’t mean we can simply cut costs by 30%. Even if we wanted to, there isn’t enough skilled talent available to do the transformative work AI requires. The only viable path forward is upskilling and reskilling the workforce, which accounts for 80–90% of the solution.
Talent transformation involves both technical and cultural training. It’s about shifting mindsets, fostering an AI-ready culture, and ensuring employees have foundational AI knowledge. Alongside talent transformation, responsible AI is another crucial success factor. Consider a midsize bank I’m advising; they currently operate with 500 AI systems. This situation is like having 100 different ERP systems. Many organisations have implemented AI solutions without conducting proper impact and risk assessments or establishing guardrails.
This is where responsible AI becomes essential. Without embedding responsible AI principles from the design stage throughout the product or solution lifecycle, problems are inevitable. Irresponsible AI systems are like cybersecurity vulnerabilities –ticking time bombs. Eventually, they will lead to scandals or catastrophic failures.
Having worked closely with the Office of Responsible AI at Microsoft, I’ve seen firsthand how essential it is to prioritise responsibility from the outset.
Whether or not governments enforce AI regulations, these issues will resurface. Why wait for a crisis when it can be addressed proactively?
Finally, I must emphasise the role of visionary leadership. Leaders at the top must be committed to AI transformation. They need to provide the necessary time, resources, and investment. And, just as importantly, they must understand that AI adoption won’t be perfect from day one. AI algorithms start ‘dumb’ and become smarter over time. Leaders must grasp at least this much about the technology to champion it effectively.
Ultimately, success comes down to talent transformation, responsible AI, and visionary leadership – all working in harmony.
What other aspects of responsible AI are important for organisations to consider?
Responsible AI starts with core principles, and globally, these principles are remarkably consistent across industries. I recently conducted a global review of AI regulations and responsible AI guidelines for a client, and the same key principles came up repeatedly: transparency, accountability, fairness, privacy, reliability, and security.
However, knowing these principles isn’t enough. The critical factor is executive sponsorship.
Leadership at the highest level must understand that AI technologies carry significant risks. To protect the business – its finances, legal standing, and reputation – responsible AI practices must
be adopted proactively, not just because regulations demand it.
For example, I’m working with a client in a country without any AI regulations. Yet, they’re still performing gap analyses and impact assessments because they recognise the risks. The potential consequences are enormous. There are countless cases of AI-related scandals resulting in billions of dollars in lawsuits. While many of these incidents are quietly handled behind the scenes to honour data privacy of the individuals involved and to avoid reputational damage, the risks are real and significant.
What advice would you give to organisations that are just beginning to develop a generative AI strategy?
My key recommendation for organisations starting their generative AI journey is to find a guide or ‘chaperone.’
This could be an advisor, an external organisation, or someone who can support the company at the right pace. AI transformation isn’t something you can inject overnight or complete in an eightweek sprint. It needs to be infused at a pace the organisation can absorb, adopt, and grow with.
Sometimes, an organisation
might be ready to sprint for a few weeks or months, but then may need to slow down to focus on training and absorbing the benefits of the technology. Every industry and organisation has its own rhythm, and it’s crucial to respect that.
Having external guidance is especially valuable. AI is an exciting, ‘shiny’ object, and internally, this can create friction and power struggles. An external advisor can serve as a neutral mediator – someone who isn’t caught up in internal politics. Sometimes, I play the ‘bad cop,’ telling clients they need to invest more or that progress will take longer. Other times, I’m the ‘good cop,’ encouraging them by reminding them about the North Star of their AI transformation and showing achievable milestones.
After 26 years in corporate life, I understand how challenging internal dynamics can be. AI transformation is deeply humancentric and culture-centric. If people don’t want to share their data, they won’t. You can have the best tools and automation, but resistance to change will derail any effort. That’s why having an inspiring, external catalyst can make all the difference.
BY NICOLE JANEWAY BILLS
NICOLE JANEWAY BILLS is the founder & CEO of Data Strategy Professionals. She has four years of experience providing training for data-related exams and offers a proven track record of applying data strategy and related disciplines to solve clients’ most pressing challenges. She has worked as a data scientist and project manager for federal and commercial consulting teams. Her business experience includes natural language processing, cloud computing, statistical testing, pricing analysis, ETL processes, and web and application development. She attained recognition from DAMA for a Master Level pass of the CDMP Fundamentals Exam.
In an era when algorithms make decisions about hiring, healthcare, and so much more, it’s refreshing to see a wave of influential books written by women who are deeply involved in shaping these technologies. Each author featured here highlights a distinct aspect of data and AI, from uncovering bias and advocating for inclusive design to translating complex technical challenges into practical guidance for organisations. These reads offer more than technical insights: they reveal how AI and analytics collide with social values, cultural contexts, and human needs. Whether you’re a data practitioner seeking fresh perspectives or simply curious about the intersection of technology and ethics, the voices captured in these five titles will broaden your understanding of what it means to create responsible, forward-looking solutions.
Authors: Sol Rashidi
Time to read: 7 hrs 28 mins (224 pages)
Rating: 4.7/5 (63 total ratings)
As an early adopter who helped launch IBM Watson in 2011, Sol Rashidi brings hands-on experience to implementing AI in business environments. She presents a practical approach that cuts through the hype, offering guidance for non-technical leaders who need to understand and deploy AI effectively.
The book stands out for its use of real-world examples across multiple industries – from retail to healthcare, energy to agriculture. Through these case studies, Rashidi demonstrates how different organisations have navigated the complexities of AI implementation, sharing both successes and setbacks. Her insider perspective on working with leading AI companies provides valuable context for understanding what’s possible and what’s premature.
What sets this book apart is its focus on the human side of AI deployment. Rashidi addresses the common obstacles that arise when introducing AI into established organisational processes, from securing executive buy-in to preparing teams for change. She provides frameworks for identifying suitable AI applications and building support among stakeholders who may be sceptical or hesitant.
The book gives special attention to the gap between
A GUIDE FOR THINKING HUMANS
Author: Melanie Mitchell
Time to read: 11 hrs 12 mins (336 pages)
Rating: 4.6/5 (904 total ratings)
Melanie Mitchell, a computer science professor and veteran AI researcher, clarifies the complex world of artificial intelligence. She begins by charting AI’s origins in fields such as computer vision and neural networks before connecting these ideas to modern-day machine learning breakthroughs. Rather than claim that AI is poised to eclipse human intelligence tomorrow, she points to the gaps in current algorithms and shows how easily they can be led astray by ordinary inputs. This viewpoint reveals where human judgment still trumps computation. Her approach is refreshingly straightforward. For instance, Mitchell distinguishes between ‘narrow’ AI – systems tuned for specific tasks – and the broader, more flexible intelligence we exhibit in everyday life. She touches on successes, such as high-level game-playing systems, but reminds us that real-world problems rarely have perfect rules or neat boundaries. Drawing on her academic background, she explains key topics such as natural language processing without veering into overly dense theory.
A key strength of this book lies in its reflection on
1AI’s potential and its practical implementation. Rashidi offers specific techniques for evaluating AI opportunities and managing expectations, which is particularly valuable for organisations taking their first steps with this technology. Her emphasis on starting small and scaling gradually provides a realistic roadmap for businesses at any stage of AI adoption.
Throughout the book, Rashidi’s conversational tone helps ensure that readers outside the technical realm remain engaged. She avoids heavy jargon, choosing to prioritise clarity over arcane explanations of algorithms or code. Her anecdotes emphasise the importance of strong project ownership, proper resource allocation, and setting realistic timelines. In doing so, she dispels the myth that AI initiatives are always highstakes moonshots. Instead, she shows how organisations can reach meaningful results by focusing on tangible benefits and maintaining flexible structures.
TL;DR: A practical guide for organisational leaders implementing AI based on real-world experience rather than theoretical possibilities. Focuses on actionable strategies and common pitfalls to avoid.
2the relationship between AI and human cognition. Mitchell brings up how biases in training data mirror societal issues and how interpretability remains a challenge, especially for large models. She also addresses pressing ethical questions around automation, emphasising that humans should remain active decision-makers in how AI evolves. By weaving in her own experiences and careful analysis of well-known experiments, she presents AI as a rapidly developing field still in need of critical thinking and ongoing research.
Mitchell is a master at exploring how AI systems actually work, what they can and cannot do, and what these limitations mean for their role in our future. This perspective helps readers move beyond both excessive hype and unwarranted fears about AI technology.
TL;DR: A clear-eyed examination of artificial intelligence’s current capabilities and limitations, written by an expert who knows how to make complex concepts accessible without losing technical accuracy.
Authors: Joy Buolamwini
Time to read: 11 hrs 12 mins (336 pages)
Rating: 4.6/5 (159 total ratings)
In her latest book, Joy Buolamwini shares her journey from tinkering with robotics as a high-school student in Memphis to her work at MIT’s Media Lab, where she uncovered bias in widely used facial recognition technologies. These systems often perform poorly on individuals from certain racial or gender groups, and her evidence shook the tech community. In describing this ‘coded gaze,’ she reveals how unexamined assumptions can seep into AI and produce harmful outcomes at scale.
What distinguishes this work is its focus on practical solutions. Rather than simply critiquing AI’s problems, Buolamwini outlines specific steps for building more equitable systems. She examines how intersecting forms of bias – including racism, sexism, and ableism – create compounding effects in AI applications, affecting everything from healthcare access to criminal justice.
The book shows that algorithmic errors are more than technical glitches; they can amplify inequality. Buolamwini recounts how these flaws motivated her to found the Algorithmic Justice League, an initiative that strives for
Author: Kirsten Martin
Time to read: 11 hrs 40 mins (352 pages)
Rating: 5/5 (2 total ratings)
Kirsten Martin examines the ethical dimensions of data analytics through a practical lens in her latest book. Whether an organisation is collecting user information for targeted marketing or applying algorithmic risk assessments for employee evaluations, Martin makes it clear that these choices carry serious consequences. She offers a range of ethical frameworks to guide readers in assessing the impact of analytics at every step, reminding us that being ‘datadriven’ does not necessarily mean being just or fair.
The book is structured around three core themes. First, it explores how data analytics programs inherently embed values that affect moral outcomes. Second, it emphasises the critical role of design in shaping these outcomes. Third, it examines how data analytics and AI systems distribute power, investigating who benefits and who faces marginalisation. Unlike many texts that search for universal answers, Martin takes a more nuanced approach. She neither condemns technological progress outright nor does she blindly endorse it. Instead, she presents a balanced view that invites critical thinking about transparency and responsibility. Through examples, she highlights how organisations can violate privacy or reinforce harmful biases
accountability and ethical guidelines in AI development. While her research focuses on facial analysis tools, her broader aim is to caution that any poorly audited AI can reinforce stereotypes and perpetuate injustices.
Buolamwini encourages readers to look beyond shortterm commercial goals and instead examine how new technologies shape civic life. She calls upon policymakers, developers, and the public to demand transparency in automated systems. By drawing on examples from her own investigations and ongoing advocacy, she highlights how public pressure can spur companies to improve their products and avert discriminatory outcomes.
TL;DR: A researcher’s firsthand account of uncovering bias in AI systems, combined with a practical roadmap for building more equitable technology. Explains complex technical concepts while keeping focus on their human impact.
when they don’t question the algorithms they build and deploy.
Martin pays particular attention to questions of accountability, privacy, and surveillance. She demonstrates how seemingly technical decisions about data collection and analysis carry substantial moral weight, affecting individual rights and dignity. The book shows how standard business decisions in analytics create ripple effects that extend far beyond immediate business objectives. Her background as a technology ethics professor underpins her arguments, grounding them in both real-world practicality and academic rigour. Ultimately, the reader is encouraged to view data analytics not just as a path to efficiency or profit but as a domain where human dignity and rights can be protected or eroded by design.
TL;DR: A practical examination of ethical decisionmaking in data analytics, focusing on how technical choices affect human rights and dignity. Uses real cases to demonstrate how different ethical frameworks can guide better system design.
NICOLE JANEWAY BILLS
Authors: Caroline Criado Perez
Time to read: 14 hrs 32 mins (436 pages)
Rating: 4.6/5 (12,076 total ratings)
Caroline Criado Perez’s meticulous research underscores how our data-driven world often overlooks half its population. By treating men’s experiences as the baseline, products and policies inadvertently fail to address women’s specific needs. Through a range of cases – from medical research to urban planning – Perez shows how everyday decisions can disadvantage women, often in surprising ways. She references data gaps in crash-test dummies, for example, that can lead to higher injury rates for female drivers. These gaps emerge in places as routine as office temperature standards or smartphone sizing.
The book goes beyond exposing shortcomings; it addresses the tangible consequences for women’s health, productivity, and economic advancement. At the same time, Perez offers evidence-based steps toward more inclusive practices, calling for better representation in data collection and a broader effort to question existing assumptions. Her
5approach aims to shift mindsets rather than simply point out inequities: if designers and policymakers ask more inclusive questions, they might arrive at solutions that serve everyone more effectively.
While the subject matter can be unsettling, Perez’s writing remains direct and informative, letting realworld examples speak for themselves. By spotlighting the hidden costs of treating men’s data as the default, she illustrates how more inclusive data practices can improve outcomes across all demographics.
TL;DR: A data-driven examination of how the failure to collect and analyse gender-specific information has created a world that systematically overlooks women’s needs, with consequences ranging from daily inconvenience to substantial harm.
The women behind these works bring clarity, expertise, and personal experience to a field that too often glosses over its deeper implications. They remind us that data is never just data – it carries the weight of human decisions, beliefs, and potential biases. By looking at AI through their eyes, we gain a more nuanced picture of how these systems are built, deployed, and regulated. We hope these recommendations spark fresh ideas and greater awareness of the human stakes in AI and data analytics. Whether you’re managing AI projects, debating the ethics of automation, or simply looking to better understand the world of technology, these authors show the importance of questioning existing assumptions. In so doing, they also shine a light on new pathways for growth, innovation, and respect for the people who engage with these systems every day.
SONJA STUCHTEY
What inspired you to enter the environmental, social, and governance (ESG) field?
I’ve been in this field for 30 years, though it wasn’t called ESG at the time. My focus has always been on addressing the most pressing challenges businesses and societies face. My journey began with my second startup in the social space, which aimed to scale and improve early STEM education.
From there, I moved into
SONJA STUCHTEY is an accomplished professional with a rich background in business economics and broad experience in entrepreneurship and technology. She started her career as a business consultant at Booz Allen and shifted her focus towards her own business ventures.
Sonja has founded SCIENCE LAB, an education platform, and Alliance for Europe, a civil tech company. Sonja acted as a director in the business consultancy AlixPartners and – focusing on enterprise improvement and innovation – she’s part of the Capitals Coalition supervisory Board and the HeadBox Advisory Board.
Sonja received her PhD from the Technical University of Munich for empirical education research. Her most recent business endeavour is the naturefintech company The Landbanking Group, which strives to put nature on the balance sheet and fosters the idea of land stewardship.
Sonja is a prolific author, with multiple publications spanning nature tech, economic development, and science education.
governance – the ‘G’ in ESG – by founding a civic tech startup focused on social listening and monitoring misinformation on social media. More recently, I’ve entered the environmental sector, the ‘E’ in ESG, through our work at The Landbanking Group.
There are many urgent challenges, but one in particular caught my attention: leveraging
technology to drive land transformation toward more regenerative practices. With the right technology, we can make informed, data-driven decisions about land use management. This ensures long-term sustainability –and ensures prosperity – benefiting both the environment and future generations.
Regenerative agricultural practices are essential for restoring soil health and ensuring food security.
Looking at your specific transition into the environmental sector, what were the initial challenges you faced?
Ecology – especially when you consider entire ecosystems rather than just human-driven interactions with nature – is incredibly fascinating. Our goal is to establish nature as an asset class by creating transactional contracts based on ecological health. However, investors often found this very ambitious and asked us to narrow our focus to a single metric, such as carbon measurement. We explained that nature’s health is interdependent; we must consider not only carbon but also water, biodiversity, and soil health.
To make biophysical improvements investable, we needed to integrate this approach into financial markets in a way that investors could appreciate. Hence, we have to connect two disparate fields: ecosystems that obey the laws of nature and thrive in balance – and finance which is set up for continuous growth. Building a bridge between the two is our challenge. It requires us to embrace both in their entirety.
dismissed environmental issues, attempting to revert to outdated economic practices reminiscent of the 1960s. This demonstrates a failure to acknowledge that environmental sustainability is fundamental to economic stability rather than a passing trend.
What industry challenge drove you to develop your platform?
The main challenge is shifting the perception of environmental sustainability from a marketing trend to an essential infrastructure. This perspective has driven the development of our technology over the past three years. Thriving ecosystems enable business continuity and prosperity, so we must understand, measure, and monitor them effectively.
Once we grasp the risks and opportunities associated with ecosystems, we can mitigate those risks and invest in opportunities.
The main challenge is shifting the perception of environmental sustainability from a marketing trend to an essential infrastructure.
What are the most significant trends you’ve observed in the environmental sector since you started?
We’re experiencing a rollback in this sector. Unfortunately, we have yet to fully recognise the natural environment and ecosystems – on which our prosperity depends – as critical infrastructure. Instead, environmental concerns have often been treated as trends that come and go – like in a hype cycle.
About two years ago, environmental discussions were at a peak, but we’re now in a deep trough. The current U.S. administration has largely
This understanding led us to create Landler: a technology solution and management platform that facilitates both comprehension and investment transactions at scale. Our approach is unique in its ability to analyse plot-level data, leveraging AI, advances in remote sensing and increased monitoring frequency.
Thanks to these technological advancements, we can now assess ecological and ecosystem health at the hectare level over time. This enables businesses to manage natural assets just as they would any other critical component of their operations.
Can you elaborate on the key ecological problems your company is trying to solve?
One of the most pressing ecological issues is the overuse and depletion of ecosystems. We’re
increasingly hearing about the loss of biodiversity and the lack of water; there is less focus on soil. For decades, we’ve used pesticides and fertilisers to maximise yields, but this has come at the cost of degrading soil health. The delicate balance within soil ecosystems has been disrupted, leading to predictions that up to 95% of the planet’s soil could become infertile by 2050. If this happens, largescale food production will become impossible, pushing us toward a global food crisis.
Surprisingly, despite the alarming findings of recent studies, the issue has not received the urgent attention it deserves. People remain focused on other concerns while an impending food crisis looms. If we don’t change our approach to land use, we risk longterm agricultural collapse.
To address this, we must allow soil to regenerate. Soil is more than a medium for plant growth; it provides essential nutrients and sustains biological processes critical for fertility. Regenerative agricultural practices are essential for restoring soil health and ensuring food security.
This shift is crucial for businesses as well. Any company that depends on land-based resources must transition from exploitative practices to regenerative ones. Our solution, Landler, facilitates this shift by leveraging remote sensing and high-frequency monitoring. With hectare-level insights, we can track ecological health over time, allowing businesses to manage soil, water, biodiversity as a vital asset for their long-term success.
How does your company plan to address the ecological crisis of soil degradation?
One of the biggest barriers to ecological transition is the lack of funding. Many land users, especially smallholder farmers, can’t afford to shift to regenerative practices. Around two-thirds of
their costs go toward fertilisers and pesticides, ensuring short-term yields but degrading long-term soil health. Transitioning to sustainable methods would eventually reduce costs, but initially leads to lower yields for several years as the soil recovers.
With approximately 600 million smallholder farmers worldwide, most lack the financial means to make this transition. The same issue applies to forestry, particularly in restoring biodiverse forests rather than maintaining monocultures.
Our solution facilitates a contract between willing investors and farmers based on measurable ecological outcomes. A farmer commits to regenerative practices, resulting in improved carbon storage, water retention, and biodiversity. Investors can track these improvements over time using Landler’s platform. As the farmer successfully transitions their land to sustainable practices, investors receive measurable ecological assets and gain access to healthier, more resilient harvests in the future.
This approach allows investors to secure the future health and resilience of their supply chains. By investing in ecosystem regeneration, they’re not just financing sustainability – they’re ensuring the long-term viability of the very resources their businesses depend on.
Can you share an example of how your clients have benefited from using the Landler platform?
One example comes from the construction sector. A company building a new industrial site for cement production used Landler to assess the natural capital of its location and surrounding landscape. They discovered that while their site had minimal flood risk, rising temperatures due to climate change would make summer production increasingly
difficult within the next ten to twenty-five years.
Recognising this long-term risk, they began reconsidering their site design. Instead of conventional approaches that prioritise quick water drainage, they implemented measures to retain water on-site, leveraging its natural cooling effect. They also integrated more biomass around the facility to reduce surface temperatures.
Additionally, they identified a neighbouring forest as a valuable asset for cooling during the summer. Rather than purchasing the land outright, they invested in its preservation by compensating local land stewards to maintain the forest. This provided a tangible benefit: reduced heat exposure, leading to lower cooling costs and improved working conditions.
This case illustrates how Landler enables companies to make datadriven decisions that enhance both environmental sustainability and business resilience. By managing natural assets strategically, businesses can mitigate risks, lower operational costs, and ensure longterm viability.
Have any recent technical breakthroughs aided your approach?
A key breakthrough has been the advancements in remote sensing and satellite data. The
frequency, resolution, and variety of data we now receive allow us to construct a detailed history of a site. Additionally, progress in AI enables us to process vast amounts of information, triangulating in-situ data to make sense of site-specific ecological changes at a granular level. We use machine learning and deep learning models to analyse bioacoustics, images, remote sensing data, thermal data, and radar data.
AI also helps us integrate multiple data layers, allowing us to understand the complex interdependencies between biological, atmospheric, and soilrelated factors that influence land integrity.
Beyond commercial success, what broader impact do you hope your technology will have on society? Our mission is to redefine nature as critical infrastructure and a source of wealth. If we succeed, we can fundamentally change how we value and interact with the natural world. Instead of viewing nature as an external cost, we must recognise it as our most essential value driver – one that appreciates as we invest in it. The greatest potential impact is fostering a new relationship with nature that prioritises regeneration and long-term sustainability.
By investing in ecosystem regeneration, [investors] are not just financing sustainability – they’re ensuring the long-term viability of the very resources their businesses depend on.
I want to emphasise that we are in a groundbreaking space, tackling a well-known problem in an unprecedented way. We need the best minds – visionary technologists, AI experts, and problem-solvers – to help build this solution.
I’m eager to hear from people with ideas, passion, and expertise who want to contribute innovative solutions, or join us on this journey.
I’ve heard you use the phrase ‘putting nature on the balance sheet.’ How do you see that unfolding in the coming years?
Historically, investing in nature was seen as an external cost recorded in P&L statements – something to be minimised. This approach is fundamentally flawed because it fails to acknowledge that natural resources are critical assets. We have been cutting down the very tree we are sitting on.
We need to shift our perspective and recognise natural capital as an investment. Our approach has enabled companies to capitalise on their investments in nature as intangible assets. We already have examples of companies successfully recording these investments on their balance sheets.
This shift is crucial, particularly for industries dependent on long-term environmental health. In sectors like coffee, cocoa, and citrus, declining ecosystem health threatens future harvests. Recognising nature as an asset ensures sustainable production and long-term economic viability.
How do you see the ESG landscape evolving over the next three to five years?
Unfortunately, we’re witnessing accelerating climate change, increasing ecological depletion, and worsening food security. This will have profound effects on urban environments and
industrial activities. I foresee a shift from a compliance-driven market to one focused on risk and opportunity management.
Discussions around ESG have often centred on CO2 certificates and carbon footprints, focusing on guilt and compliance. However, I believe the conversation will pivot towards assessing risks and seizing opportunities. Companies that take the lead in this transition will be best positioned to navigate the inevitable wave of asset devaluation and impairment in the coming years.
What experiences from earlier in your career have shaped your leadership philosophy?
At the Landbanking Group, we take a different approach compared to many tech companies. While we use agile methods, we have deliberately moved away from traditional topdown organisational structures. Instead, we operate in specialised circles that cover key areas such as tech, science, marketing, sales, and projects.
Rather than relying on a strict hierarchy, we encourage shared responsibility for the company’s success. It’s not about making every decision democratically, but ensuring team members take full ownership of their roles, while contributing beyond their immediate responsibilities.
Managing these interconnected circles can be challenging due to the rapid pace of development, but the benefits are immense. This structure fosters an incredible density of ideas and skills, allowing each team member to leverage their expertise while engaging in broader company objectives.
I faced resistance – particularly from more senior colleagues who were accustomed to traditional hierarchies. However, given the complexity of the challenges we address, I’m grateful that we
pushed forward with this model. We can’t afford to rely on just a few decision-makers when solving such multifaceted problems. Every mind in our team plays a crucial role in driving solutions forward.
How do these circles differ from traditional teams or business functions?
The key difference is that we don’t have traditional department heads. Instead, we distribute responsibilities based on skills rather than rank or tenure.
For example, in our science circle, one team member might oversee the overarching scientific strategy, while another manages budget details. Instead of assuming that the most senior scientist should handle all aspects, we assign tasks based on who’s best suited for each role.
This approach ensures that expertise is leveraged effectively while maintaining flexibility. Rather than adhering to rigid structures, we allow team members to contribute in ways that align with their strengths, leading to a more dynamic and adaptive organisation.
As a founder, is there anything you wish you’d known earlier that could have helped you now?
There were actually no major surprises. Maybe because this is my fourth startup, I knew it would be challenging. You have to be a little crazy and fully committed to your technology and business model.
Many of the initial hurdles we faced have faded as the world evolved in the direction we anticipated. I wouldn’t have done anything differently. Our team is fantastic: dedicated, innovative, and a joy to work with. We also have an incredible group of investors who are fully committed for the long term, which makes a huge difference.
To hire the best person available for your job you need to search the entire candidate pool – not just rely on the 20% who are responding to job adverts.
Data Science Talent recruits the top 5% of Data, AI & Cloud professionals.
Fast, effective recruiting – our 80:20 hiring system delivers contractors within 3 days & permanent hires within 21 days.
Pre-assessed candidates – technical profiling via our proprietary DST Profiler.
Access to talent you won’t find elsewhere – employer branding via our magazine and superior digital marketing campaigns.
Then why not have a hiring improvement consultation with one of our senior experts? Our expert will review your hiring process to identify issues preventing you hiring the best people.
Every consultation takes just 30 minutes. There’s no pressure, no sales pitch and zero obligation.
ROSANNE WERNER
ROSANNE WERNER is an internationally recognised data and AI culture change leader, known for her innovative approach to data and digital transformation. Her specialty lies in integrating behavioural science principles with data and digital initiatives, creating a unique methodology that addresses both the technical and human aspects of organisational change.
She led the Coca-Cola Europacific Partners’ Data in Action programme – a global data and AI culture and mindset initiative reaching over 2,000 participants across multiterritories. This programme’s innovative approach and measurable impact earned industry-wide recognition by DataIQ in the Best Data Skills
Development Programme Category, and by IDC Foundry’s CIO 100 Awards.
Having established an influential legacy during her tenure at Coca-Cola, she has continued to shape the field on a global scale.
Rosanne’s work incorporates insights from cognitive psychology, organisational behaviour, and change management initiatives to improve the adoption and impact of data and AI technologies. This approach recognises that successful digital transformation isn’t just about implementing new tools or technologies, but also about changing habits, mindsets, and organisational culture.
Rosanne is this year’s British Data Award Finalist - Data Leader of the Year (Supplier).
You previously led the Data and AI Mindset and Culture Programme at Coca-Cola Europacific Partners. Can you share with us your experience and what made the programme so successful?
During my 10-year tenure at Coca-Cola Europacific Partners, I had the privilege of leading the Data in Action programme – a multi-award recognised Data and AI Mindset and Culture initiative. This programme employed a multi-touch approach that empowered participants to turn data into insights for action.
Coca-Cola Europacific Partners wants to be the best at using data: adopting new data products, asking the right questions, telling stories with data. It’s set an
The biggest mistake organisations make is treating the human element as an afterthought.
ambition to become a data-guided company. Crucial to that is to have the right mindset, one that knows how to use data.
The programme reached over 2,000 data catalysts across different functions and territories around the globe. This vibrant and engaged data catalyst community was a key cornerstone of the programme’s success. By equipping the data catalysts with a unified data strategy vision – essentially giving them the same ‘hymn sheet’ – we leveraged their diverse backgrounds and extensive organisation reach. This approach helped raise the awareness of our cohesive data strategy and drive large-scale mindset and cultural transformation.
Most significantly, these data catalysts generated a powerful ‘pull’ effect throughout Coca-Cola. They challenged the status quo and championed the desired data habits, behaviours and mindset. They led change daily by inspiring their teams to build data fluency and adopt data-first decision-making.
The Data in Action programme was recognised in the DataIQ Best Data Academy or Skills Development Programme Category, as well as IDC Foundry’s CIO 100 Awards.
What made you decide to leave the legacy behind at Coca-Cola, and start XcelerateIQ Limited?
Leaving behind the legacy built at Coca-Cola was not an easy decision, but it was one driven by the inner desire to create an even greater impact and support more organisations on their transformation journey. My time at Coca-Cola provided invaluable experience in data-driven strategies, innovation, and large-scale business transformation.
However, throughout my journey, I recognised a critical gap that many organisations face – bridging the divide between technology adoption and real cultural and mindset change. To o often, businesses invest in data and technology solutions without embedding them into their organisational DNA, leading to missed opportunities and unrealised potential.
XcelerateIQ Limited was born from this realisation. I wanted to take my expertise and apply it across multiple industries, helping businesses unlock opportunities through data and decision intelligence, digital transformation, and strategic insights.
We focus on embedding data-driven decision-making into mindsets, processes, and company culture. By integrating the technical what with the human how , we ensure that investments in data and technology deliver real, sustainable value.
On a personal level, this transition also meant embracing entrepreneurship – stepping outside the comfort of a corporate structure and taking the risk to build something that can accelerate meaningful change on a larger scale – empowering teams, fostering data cultures, and ensuring that transformation efforts lead to sustainable, measurable impact.
What are some of the biggest challenges organisations face when trying to bridge this gap?
Through years in the transformation space, I’ve observed a consistent pattern: while organisations invest heavily in data and technology, they often struggle to realise the full value of these investments.
The critical human element is often overlooked. This creates a two-fold challenge: teams struggle to use new technical capabilities while simultaneously unable to embed these tools into their daily workflows.
This leads to strong cultural resistance that runs deeper than simple change aversion. The main challenge isn’t primarily about tools or technology – it’s about fundamentally changing how people work, think, and act.
The usual data literacy programmes tend to fall short as it’s not just about one-off learning modules or workshops. It’s about building confidence in handling and interpreting data, developing fluency through regular practice and application, changing habits and behaviours to embed data into daily routines, and creating environments where data-driven decisions are expected and valued.
You often reference Martec’s Law in your work. How does this concept shape your approach to data and digital transformation?
Martec’s Law perfectly captures the central challenge in data and digital transformation. It illustrates how technology advances exponentially while organisations can only change logarithmically. This creates a widening gap that many companies struggle with.
Martec’s Law highlights the critical need for organisations to develop strategies that enable faster adaptation to technological change while recognising the inherent limitations of organisational transformation speeds – particularly the time required for shifts in behaviour, culture, and processes.
This requires a balanced approach – one that integrates technological advancements with cultural and operational readiness. Rather than pushing technology faster – which often leads to resistance and failed initiatives – it means creating strategies that work with human nature rather than against it, helping organisations absorb change more effectively.
For example, when implementing new data tools, we break down adoption into manageable steps, integrating it with existing workflows, ensuring educational resources are always accessible and establishing peersupported systems. This approach helps organisations navigate change at a sustainable pace while maximising the value of their technology investments.
Many companies invest in data literacy programmes but struggle with long-term adoption. What’s your approach to ensuring sustainable change?
Traditional one-off training rarely leads to lasting behavioural change. My approach focuses on three key elements that align with how our brains naturally learn and adapt:
LEARNINGS THAT STICK
First, we create ‘Learnings that Stick’ through microlearning approaches. These bite-sized, interactive sessions provide immediate feedback and regular reinforcement through practical application. We incorporate gamification elements like quizzes, polls, leaderboard points and badges to make learning more engaging and memorable.
LEARNING BY DOING
Second, we emphasise ‘Learning by Doing’ through hands-on activities that integrate data and AI tools into daily work routines. This practical application helps embed new skills and behaviours through repeated use.
LEARNING WITH OTHERS
Third, we facilitate ‘Learning with Others’ through peer-to-peer activities across functions and territories. This creates a supportive community around data usage, encouraging the sharing of insights and best practices. The community aspect helps sustain long-term change in organisational behaviour.
You’re known for integrating behavioural science with data and digital initiatives. How does behavioural science enhance the effectiveness of data and AI programmes, and what role does it play in overcoming resistance to change?
People are complex beings shaped by their experiences, habits, and existing ways of working.
Our approach starts with understanding current data and AI behaviours within teams – how they interact with data, their decision-making patterns, and any underlying resistance or cultural factors. We also consider previous experiences with technology changes, areas of concern or scepticism, and the overall level of trust in data and systems.
ROSANNE WERNER
We examine how teams currently collect, store, and share data, their ability to interpret and use data, and the frequency and depth of data usage in daily operations. We look at key decision points in core business processes, the balance between intuition and data-led decisions, and the speed and effectiveness of decision processes. This understanding allows us to identify both barriers and enablers, helping us prioritise initiatives and tailor solutions that leverage existing positive practices and attitudes.
By recognising underlying resistance, cultural backgrounds, and past experiences with technology, we can design interventions that address concerns directly and build trust in data-driven approaches.
This ensures that change initiatives work with natural human tendencies rather than against them, fostering adoption and utilisation of data and digital investments. By embedding data and AI into everyday workflows
in a way that aligns with how people naturally work, organisations can realise the full potential of their data and digital investments – enabling better-informed decisions, fostering innovation, improving performance, and ensuring a sustainable long-term impact.
With 70-95% of data and digital transformations failing, how do you address the human element when leading large-scale digital initiatives?
The biggest mistake organisations make is treating the human element as an afterthought. People are at the heart of any transformation, and they can’t change overnight. The people engagement needs to run in parallel with technical implementation – not as a separate stream that comes later.
Organisational attitudes, behaviours and culture are listed high on the greatest challenges in the CD(A)O role per the latest AWS CDO Agenda Report:
AWS CDO Agenda 2024: Navigating Data and Generative AI Frontiers
Drawing from my experience leading global transformations, particularly at Coca-Cola Europacific Partners, there are three key elements that have been critical for large-scale transformation success:
Every aspect of the transformation should prioritise user experience:
● Design intuitive interfaces that minimise friction or obstacles to utilisation
● Conduct regular user research to understand pain points and needs
● Create feedback loops for continuous improvement
● Maintain collaborative spaces where teams can share learnings and wins
● Test and iterate based on real user experiences
Achieving meaningful change requires building a strong community of catalysts who:
● Drive transformation within their areas of influence
● Act as role models for new behaviours and mindsets
● Create organic momentum through peer-to-peer influence
● Embed incremental changes that build lasting habits
Leaders play a crucial role in:
● Articulating a clear vision that resonates across all levels
● Demonstrating visible commitment to the transformation
● Connecting changes to individual career growth opportunities
● Building team capabilities through structured support
● Celebrating progress and sharing success stories
ROSANNE WERNER
By incorporating the human element from day one, you create an environment where change feels natural rather than forced. This approach has consistently delivered better adoption rates and more sustainable outcomes in my experience.
Successful transformation isn’t about pushing people to change – it’s about creating conditions where change becomes the path of least resistance. When people see the benefits, have the right support, and feel part of the journey, they become active participants rather than passive recipients of change.
What are the most critical challenges organisations face in AI integration, and how can they be overcome? AI integration presents enormous potential, but many organisations underestimate the challenges that come with it. It’s more than just implementing the latest capabilities – it’s a fundamental shift that requires the right foundations and sustainable strategies. Organisations must take a structured approach, ensuring their data, people, and strategy are aligned to ensure effective adoption and utilisation that brings real value in return.
AI implementation must solve real business problems rather than chasing the latest shiny tools. Organisations need clarity on value creation – identifying specific business challenges AI will address, defining how success will be measured, and calculating expected returns on investment.
The implementation strategy must be equally clear. Organisations should assess whether AI is truly the most effective solution, what resources and timelines are required, and how progress will be tracked and adjusted. This prevents organisations from pursuing AI simply because it’s trendy or because competitors are doing it.
Many organisations dive into AI without a proper data infrastructure, leading to the classic ‘garbage in, garbage out’ scenario. There needs to be a solid foundation in both data quality and governance. This means establishing clear ownership and accountability across the organisation, implementing robust quality control measures, and creating accessible, well-documented data systems that build trust.
The infrastructure must support these ambitions. Organisations need to assess their current data architecture, ensure scalable storage and processing capabilities, implement proper security measures, and create seamless data integration workflows. Without this groundwork, even the most sophisticated AI solutions will struggle to deliver value.
Organisations need a comprehensive people strategy, starting with strong leadership alignment. Senior leaders must actively champion the change, clearly communicate the AI vision and benefits, and demonstrate visible commitment to supporting the transition through regular team engagement.
Cultural transformation requires building AI fluency across all levels. Addressing fears and misconceptions early, creating engaged and active communities of practice, and celebrating early wins all contribute to sustainable adoption. Success stories should be shared widely to build momentum and demonstrate practical and relevant real-world impact.
Organisations that tackle these challenges systematically are far more likely to succeed. They create sustainable AI transformations that deliver measurable business value rather than just implementing technology for technology’s sake.
Effective AI integration requires careful preparation, continuous adjustment, and unwavering focus on both technical and human elements
How do you recommend organisations balance increasing AI adoption with cultivating essential human skills?
With AI developing at such a fast rate, many are experiencing ‘AI fatigue’ – overwhelmed by the constant influx of new tools, ever-increasing capabilities, and the pressure to keep up. But the key to navigating this shift isn’t about resisting AI or blindly adopting it; it’s about developing an AI Mindset – one that sees AI as an enhancer of human potential rather than a replacement.
Companies must be intentional about integrating AI in ways that elevate human capabilities rather than erode or diminish them.
One key strategy is mapping the AI impact and how this redefines roles. AI should take on data-heavy tasks like processing, pattern recognition, and automation, while humans focus on areas where we excel: strategic thinking, emotional intelligence, and complex problem-solving.
Complementary workflows that incorporate AI for efficiency while maintaining human oversight ensure an effective partnership between technology and human expertise. AI can generate insights and options, but human judgment must guide decisions.
We should celebrate the unique human elements that AI complements but cannot replicate – our creativity, curiosity, critical thinking, adaptability, collaboration, and communication must be prioritised as core competencies.
ROSANNE WERNER
Organisations should encourage and nurture a culture of experimentation, encourage cross-functional collaboration, and invest in developing these skills through training and leadership initiatives. Recognising and rewarding these abilities reinforces their value alongside AI capabilities. These are the skills that AI can support but never replicate, and they will define the next generation of high-impact, AI-empowered organisations.
Driving people change is one of the greatest challenges in any data and digital transformation. While technology can be implemented relatively quickly, shifting mindsets, behaviours, and ways of working takes time and deliberate effort. We need to approach this with the right mindset – one that places people before technology. Ensuring employees feel supported and empowered as new tools are introduced is critical. Without this, even the most advanced solutions will struggle to deliver meaningful impact due to lack of adoption and utilisation.
Sustainable change is built through small, consistent actionable steps. Embedding transformation into daily routines, reinforcing new skills, habits and behaviours through micro-learning moments, and fostering strong peer networks all help to accelerate adoption at scale. Regular check-in feedback loops, open and safe communication channels, and celebrating progress and achievements – no matter how small – help maintain momentum and engagement.
Measuring progress effectively is just as important as setting ambitious goals. Organisations should track both technical advancements and shifts in behaviour to gain a holistic view of success. Gathering continuous feedback ensures that strategies remain relevant and adaptive, while celebrating incremental wins keeps teams engaged and motivated.
Ultimately, data and digital transformation is not a one-off initiative but an ongoing journey. We need to remember to place people at the heart of the journey, commit to continuous learning, and measure what truly matters to ensure that data and technology empower and serve people.
By integrating the technical what with the human how , we ensure that investments in data and technology deliver real, sustainable value.
AGATA NOWICKA is the Managing Partner at Visionaries – an AI incubator launched in partnership with Google, focused on accelerating the growth of the most ambitious female entrepreneurs in Europe with AI. With 13 years of experience as a VC investor and founder across the UK, US, and Hong Kong, Agata is also the founder of Female Foundry, a network of 7,000+ VC investors and founders in Europe, and the author of the Female Innovation Index – Europe’s largest report mapping the funnel of innovation driven by female entrepreneurs.
Can you tell us about your background?
Over the past 13 years, I’ve been part of the venture ecosystem in different ways; first as a founder and later as a venture capital investor and an advisor to startups. As a founder, I’ve built two companies. My first startup was a data analytics platform in Hong Kong, which I eventually sold. My second venture, which is still running, is a securitisation platform for investment banks.
What have been the most significant challenges you’ve faced on your entrepreneurial journey? It is part of an entrepreneur’s journey to be facing and solving challenges on a daily basis. I faced some of the toughest challenges in my second venture, where our potential clients were toptier investment banks. When working with large enterprises, there’s a lack of transparency and, consequently, it is very difficult to influence the outcome because of limited information and power imbalance. How can you influence the outcome if you don’t even know who is involved in the decision-making process? Even with a superior product and an innovative approach that could lead to significant revenue generation and cost savings, navigating this lack of clarity – especially when interactions are limited to short calls – was a difficult hurdle to overcome.
What inspired you to start Female Foundry?
About five years ago, I decided to move to the venture capital side. I realised I had accumulated a wealth of experience as a founder and wanted to be part of other startups’ journeys, rather than focusing solely on a B2B capital markets company, which was moving slowly at the time. I was also looking for more variety in my work, as I had been laser-focused on my startup for so long. After joining a
VC fund, I very soon noticed that I was one of very few investors with founder experience. I looked into the statistics and found some eye-opening data. According to PitchBook data from 2022, only 7% of VC investors in Europe had built or attempted to build a company. That’s a shockingly low number. Meanwhile, in the U.S. the percentage is 45%. In Europe, you have to search very hard to find an investor who truly understands what it takes to build a company. And so, I realised that by bringing both my founder and investment experience, I had a lot to offer to founders who are trying to navigate their company building and fundraising processes.
Can you explain why there is such a disparity in the number of VC investors with founder experience between the U.S. and Europe? I think it relates to the origins of these two ecosystems. In the U.S., the venture capital industry as we know it today was originated by Stanford and MIT professors and successful exited founders of the dot com era like PayPal, Yahoo and many others who ended up either directly or indirectly investing in other startups – whether through syndicates, angel investing, or eventually by setting up their own funds. In Europe, venture capital emerged mainly from the private equity space. It was essentially an early extension of private equity, hedge funds and banking, which meant it attracted two types of investors: first, those who had inherited capital and sought to diversify their exposure by investing in startups. Second, those focused on private equity investments just with higherrisk. Many of these funds still exist today and tend to concentrate on series A and laterstage investments.
availability of high risk capital in Europe, institutional investors like the European Investment Fund serve as LPs in numerous venture capital funds. However, their risk appetite is significantly lower than that of an exited founder who wants to reinvest in new startups. Institutionalising capital often leads to a more conservative investment approach. That cycle of giving back to the venture industry is not as prevalent in Europe. I’m part of an exited founders’ group in London, and out of 35 members, only two – including myself – have returned to the venture space. Many have been burned in the sale process and, due to the institutionalised nature of the ecosystem, don’t feel that it’s founder-friendly space. As a result, they often choose to invest their time and capital elsewhere.
If the journey is more difficult for European founders, how does that impact them, and what should they be aware of?
VCs with founder experiences have a better idea of what it takes to turn a vision into reality. However, investors without entrepreneurial experience often rely too much on early-stage metrics to build their conviction. I actually actively seek out founders with big vision because I can understand what it takes to make it happen. I’m particularly excited about bold, tenacious, adaptable and problemsolution obsessed founders.
VCs with founder experiences have a better idea of what it takes to turn a vision into reality.
Because of the limited
Was this realisation the reason why you started Female Foundry? Yes! I saw that as a vast majority of VC investors had no prior founder experience, I had so much more to offer to the most ambitious entrepreneurs. I had built two companies successfully, and as a woman in the venture ecosystem,
I firmly believe that if you build a strong business, capital will find you.
I noticed even fewer women had both founded companies and were actively investing. During COVID, when everything went online, I started hosting discussions on the Clubhouse app where I would share my growth-hacking tips with founders, from both an investor and entrepreneur perspective. These discussions gained significant traction: at one point, we had around 200 people in a single session. That’s when I realised there was a real need for this kind of support. Also, having transitioned to the investor side, I saw that women founders were underrepresented in venture and I decided to focus on that niche. If there are only a few women in the venture space, we need to ensure those who are there, succeed. Initially, I did this alongside my full-time VC role, but about a year and a half ago, I decided to fully focus on Female Foundry.
What’s the vision for Female Foundry?
Unlike my previous ventures, my mission for Female Foundry has
not been profit-driven. If I had approached it purely as a business, I might have taken a different route by now. But since it’s deeply personal, its future is somewhat open-ended and tied to where I see myself in my career. Over the past three and a half years I’ve accomplished a lot, and I now see immense potential in accelerating growth of female-founded startups with AI.
The challenge, however, is the number of female founded startups. This might be an unpopular opinion, but I have a fairly good understanding of the European, particularly UK, ecosystem, the issue is that there simply aren’t as many exceptional female founders as I would like there to be. To be clear, this isn’t about ability or talent, but about numbers. There aren’t as many female founders to begin with, and within that smaller pool, only a fraction stand out as exceptional for various reasons. If I want to remain true to my investment principles and focus on high-potential founders, the challenge is that the pipeline isn’t
as large as we’d like to believe. Proportionally, and the same goes for both female or male founders, in general I’d say only one in ten startups I speak to is interesting, and when the overall numbers of female co-founded companies are small, that results in very few exceptional candidates to back.
What percentage of all founders are women, roughly?
Based on the Female Innovation Index 2025, only about 15% of all startups in Europe are female (co) founded and so, saying ‘only 2% of total venture capital deployed in startups goes to female founders’ is very misleading. The commonly quoted 2% figure actually refers to female-only founding teams. If we’re talking about diversity, we need to consider a broader metric. When factoring in mixed-gender teams, the funding figure improves to about 12%, and when we take into account how many (co)founded female startups there are in the first place, the fundraising success rates between female (co)founded and gender agnostic startups are actually similar!
How did your partnership with Google Cloud come to be, and how has it helped shape the development of Female Foundry?
Google approached me last year to build an accelerator, leveraging the network I had built through Female Foundry and my founder, investor and advisor experience and I saw it as a great opportunity to accelerate adoption of something I had already been passionate about: AI. This is one of the most exciting times to build a company because the amount of capital needed to develop an MVP and prove a value proposition has never been lower. AI has significantly reduced the cost for founders. That’s why value propositions matter more than ever. I had been mapping out the AI adoption curve, and when Google proposed the partnership, it made perfect sense. By combining my business and advisory experience with over 300 startups with Google’s leadership in AI, we created a truly impactful program.
What specific support do you offer to female tech entrepreneurs?
Having been a mentor at multiple accelerators, I didn’t want to create just another accelerator. There are around 500 accelerators in Europe, and I wasn’t interested in duplicating existing models. Unlike most accelerators that focus on fundraising, pitch decks, and investor introductions, we focus on company-building and growth acceleration. I firmly believe that if you build a strong business, capital will find you. We also keep our cohorts small: only six to eight companies are invited to each program. Many accelerators accept 20, 30, or even 100 startups, but that dilutes the tailored support each founder receives. With a small group, we ensure customised mentorship based on their specific needs, opportunities and challenges.
Additionally, we prioritise companies that are already
leveraging AI as a core part of their value proposition, or are science-driven.
What stage of company creation do you focus on?
Female Foundry encompasses a broad range of initiatives, such as the Female Innovation Index, which covers businesses at all stages, from the earliest startups to IPOs. However, the Visionaries AI incubator with Google focuses specifically on companies that have at least built an MVP, are potentially generating revenue and now are looking to accelerate their growth. These companies are typically post-pre-seed, having raised some capital, and now face more pressure from investors. To give you an idea, the companies that have joined Visionaries so far have raised anywhere between €600K and €15 million. The goal is to support founders in reaching their key value inflection point, whether that’s Series A or beyond.
The Visionaries program helps founders navigate this phase, filling a gap where many investors are less involved. Investors focus on capital deployment and scouting new opportunities, so founders often lack ongoing hands-on support. During the program, we provide access to AI engineers, data experts, and decision-making advice that aren’t easily accessible. By combining these resources, we empower founders to make high-quality decisions, scale effectively, and maximise their business impact.
Is Visionaries the core offering of Female Foundry?
While Visionaries is our flagship program, we also have the Female Innovation Index. Additionally, I write the Female Foundry newsletter, which has been running for three and a half years and is read weekly by over 7,000 VCs and female entrepreneurs across Europe. So, Female Foundry is more of an ecosystem player.
You’ve mentioned the Female Innovation Index. What is it and how was it created?
When I moved to the venture capital side, I kept hearing the statistic that only 2% of VC capital goes to female entrepreneurs, but I quickly realised that there was no other data on female innovation and entrepreneurship in Europe. There was no way to track the full innovation funnel from aspiring founders to those who launch companies, secure funding, and progress through the investment stages. That’s why I founded the Female Innovation Index, to provide granular data of the funnel of innovation and funding driven by female entrepreneurs. Nothing like this existed in Europe, and for what I know, in the world and so, I had to build the Index from scratch: I partnered with data providers, created the survey, and engaged about 35 private equity and VC associations across 20 European countries to get it to the point where it is today. Our data partner, Dealroom, for example, had never previously analysed gender data. As a result of our collaboration, they now offer gender-based filtering on their platform.
In the process, we analysed around 650,000 European companies to build this dataset. It was a massive undertaking, requiring the creation of a 200-question framework to determine what data points should be included. It took about a year and a half to develop the first edition of the Index and luckily over one hundred insights that we delivered proved to be very valuable! For example, we discovered that the top three industries female founders build businesses in are health, finance, and food. There’s a common misconception that female founders primarily go into stereotypically ‘female-oriented’ industries like fashion, and our
data broke that stereotype.
We also, for example, analysed the 50 largest fundraising rounds in Europe raised by female-founded companies in 2023, and learned that 95% of all female-founded companies on the list had scientific backgrounds. This insight showed me a real opportunity – supporting scientifically-driven female founders and accelerating their growth made perfect sense for Visionaries.
Investors and ecosystem players are now much more proactive in looking for investment opportunities and support structures for female entrepreneurs.
We launched the second 2025 edition of the Index in early March this year, with over 350 founders and investors joining the official launch. We had founders who collectively raised over €1.6B in one room – it was exhilarating! The Female Innovation Index is now Europe’s largest report on female innovation and funding driven by female entrepreneurs.
What were some of the key findings for this year?
Total investment fluctuates due to macroeconomic forces, so it’s important to analyse trends over time. I would encourage everyone to visit femaleinnovationindex.com to view all 100+ insights. What I can say to keep it short: 2024 was a particularly strong year for female entrepreneurs. AI adoption and the total amount of funding, IPOs, the number of unicorns, near-unicorns, and M&A transactions have all been on the rise.
What impact have Female Foundry’s initiatives had on the broader venture ecosystem so far?
When I started Female Foundry almost four years ago, no one was specifically focusing on female entrepreneurs, and at the time it was even seen as bold
to put ‘female’ in the centre of our name. Now, there are hundreds of initiatives dedicated to supporting female founders, which is fantastic progress. I’m not saying we caused this shift, but we’ve certainly contributed to the broader conversation. Investors and ecosystem players are now much more proactive in looking for investment opportunities and support structures for female entrepreneurs.
Our approach has been to frame this as a positive opportunity rather than an obligation or charity. That perspective has resonated strongly with the venture ecosystem. About 30% of our newsletter readers are men, and around 40% are VC investors.
In terms of tangible impact, the Female Innovation Index has provided transparent, granular never-seen-before data. It has helped local venture ecosystems to leverage the funding insights to drive their own local initiatives. Many have leveraged our dataset for lobbying efforts to secure more government funding. And that was just from the first edition.
With Visionaries, even though we only invite six to eight companies per cohort, we aim to build a consistent pipeline of high
potential founders. The program is still in its early stages, but the feedback from participants has been overwhelmingly positive, particularly regarding how it has influenced their growth trajectory. Ultimately, Female Foundry reflects my experience as both a founder and an investor. The way we talk about these issues has set a new precedent, making the conversation about female entrepreneurship one of opportunity and success.
How do you help founders – who are still figuring out what it takes to run a business – gain the skills and confidence to deliver at a high level to investors?
As mentioned, we live in unprecedented times where visionary, adaptive, and obsessively curious founders have the opportunity to build multi-billiondollar businesses very quickly! Investors are only a small part of the story – and always will be! What matters is your ambition to solve important problems and leveraging what you have: the latest tech, sharp advisors, and your endless creativity to get you there. Everything else is trivial. If you truly care about the opportunity you see, then start building!
ŁUKASZ GĄTAREK
Logistic regression is by far the most widely used machine learning model for binary classification datasets. The model is relatively simple and is based on a key assumption: the existence of a linear decision boundary (a line or a surface in a higher-dimensional feature space) that can separate the classes of the target variable y based on the features in the model.
In a nutshell, the decision boundary can be interpreted as a threshold at which the model assigns a data point to one class or the other, conditional on the predicted likelihood of belonging to a class.
The figure below presents a schematic representation of the decision boundary that separates the target variable into two classes. In this case the model is based on a set of two features (x1 and x2). The target variable can be clearly separated into two classes based on the values of the features.
ŁUKASZ TEOFIL GĄTAREK earned his PhD in Bayesian econometrics from the Tinbergen Institute in the Netherlands. His research focuses on time series analysis, with a particular interest in exploring machine learning concepts for sequential data analysis. His recent publications have appeared in several prestigious journals, including the Journal of Forecasting , Journal of Risk , and the Oxford Bulletin of Economics and Statistics . For more than a decade, Łukasz has been advising corporations and startups on statistical data modelling and machine learning. He currently serves as a data governance adviser for key global companies that are market leaders in their field.
However, in your daily modelling activities, the situation might look rather similar to the figure below.
Again, there are two features in this model. However the estimated decision boundary does not separate the data based on the features. This is one of the biggest issues related to the logistic model, whose impact is often highly underestimated among data scientists. The problem arises from significant overlap between the classes y=0 and y=1 in the feature space, making the decision boundary unable to cleanly separate them. This situation is commonly observed in imbalanced datasets, which can result in skewed decision boundaries.
In such cases, logistic regression struggles because it assumes linear separability. The decision boundary will not cleanly divide the classes. A major consequence of the problem concerns feature selection. During feature engineering, the features without strong separability may be deemed unimportant or insignificant by the model. The typical recommendation for logistic regression feature selection, which can be found in many books and articles, points at recursive feature elimination. This
is however pointless due to a simple fact: when some variables get removed from the model, the estimated parameters for the remaining variables will change accordingly, see:
Integrating Feature Selection into the Model Estimation
Combining mixture of normal regressions with in-built feature selection into powerful modeling tool towardsdatascience.com
The relationships between predictors and the target variable are often interconnected. The coefficients in the reduced model will no longer reflect their values from the full model, what in the end effect might be leading to biased interpretations of the model parameters or predictions. Moreover, under the scenario of weak separability by the decision boundary, the recursive feature elimination or even regularisation will not help
What is the remedy for this problem?
With nearly 20 years of experience in modelling categorical data, I would suggest that the most effective solution to this issue is to perform feature engineering using probit regression rather than logistic regression (logit model). This approach has proven to be highly effective, and I have tested it many times in practice!
Similarly to the logistic regression, the probit model can be used to categorical data modelling. Instead of logistic function
it uses normal cumulative distribution function
The models are quite similar, although training a probit model is often preferred over a logit model in terms of the decision boundary because the probit model assumes a normal cumulative distribution, which can lead to a more natural and smoother boundary. In contrast, the logit model’s logistic function may produce decision boundaries that are less flexible and more prone to overshooting or underfitting, especially when the data is not well-suited for a logistic transformation.
As a result, the probit model can better capture the nuances in the data, leading to more accurate and meaningful decision boundaries even for nonlinear and non normal data.
Let us consider the following latent variable representation of the probit model
where the value of the binary variable y_i is observed, as are the values of the explanatory variables x_i. The latent data z_i, however, are unobserved.
The model training can be performed by drawing sequentially from two distributions
where
and with the notation TN for a truncated normal.
The implementation shows how well the feature selection mechanism in-built in the model is able to identify the features irrespective of a decision boundary which is not separating the classes in the feature domain.
In the code, the set of features is created by sampling from a normal distribution. Then, a mask vector is created to simulate the situation where only a subset of the features are relevant for the data-generating process. In this case, features 1, 3, and 7 are selected. The latent variable z is simulated using the selected features based on the mask and their corresponding coefficients from the vector. The observed variable y is derived from the latent variable z. A threshold of 0 is used, such that if z is greater than 0, y is set to 1 (class 1), and if z is less than or equal to 0, yyy is set to 0 (class 0). The result is a binary outcome (0 or 1), which is typical for a categorical model, where the outcome is determined by whether the latent variable crosses a threshold.
We make it difficult for the model to identify the relevant features within the larger set of variables by using features with unclear separability, as shown in the figure below. Boxplots show highly overlapping classes for x1 and x3.
Boxplots of Selected Features by Class
Similar conclusions can be made based on density and scatter plots.
Density Plots of Selected Features by Class
As explained above, the code simulates data for a binary classification problem using a subset of explanatory variables and tests whether the model selection procedure can identify the relevant features. The feature engineering procedure based on stochastic search has been previously described in case of linear regression model and then successfully applied in context of an advanced model of mixture of regressions
In this case, the results are just as good as before!
The model is able to identify the proper features among the broader dataset, as shown in the figure below. The inclusion probability MCMC sampling trajectory for x1, x3, and x7 is either exactly 1, or mostly 1, whereas for all the other features is rather 0. (The individual probabilities computed over the full trajectory comprising of 10000 MCMC draws are given by 1.0000 0.1624 0.9752 0.1269 0.2757 0.1839 1.0000 0.1220).
Scatterplot Matrix for Selected Features
The results have been achieved despite weak separability for x1 and x3. As shown in the figure below, all of the parameters beyond the truly selected features, oscillate around 0 indicating no impact of those variables on our target!
The full code can be viewed here
Unless otherwise noted, all images are by the author.
REVOLUTIONISING BIOPHARMACEUTICAL DATA INTEGRATION AND DECISI ON-MA KING FOR BOEHRINGER INGELHEIM
BETTINA KNAPP studied bioinformatics and earned a doctorate in computer science. In 2015, she started work at Boehringer Ingelheim, initially as a team leader in biostatistics in Biopharmaceutical Manufacturing and later as a principal statistician, supporting and advancing portfolio projects in Biopharmaceutical Development. Since 2018, Bettina has been leading a laboratory in cell culture development, and in February 2024 she took over the role of Product Owner in a scrum team to develop Biobase. Thus, Bettina’s experience combines three worlds: 1. laboratory and the business side, 2. data analysis and machine learning, and 3. software development of an internally developed IT project.
BETTINA KNAPP
Boehringer Ingelheim is a family-owned pharmaceutical company founded in 1885 in Ingelheim, Germany. We focus on human pharma and animal health, and have research and development as well as commercial manufacturing sites all over the world.
Biobase is a Boehringer Ingelheim internallydeveloped web application for use within biopharmaceutical development and production. It provides access to a mapped and harmonised dataset of biopharmaceutical development and manufacturing data. The application was developed in an agile scrum team and uses commercial software parts to run the pipeline and store the data, mainly a Boehringer Ingelheim environment, which runs with tools on the AWS (Amazon Web Services) cloud. The Biobase team consists of five developers, one scrum master, four subject matter experts (SMEs) and one product owner. The stakeholders and users of Biobase come from different departments and areas within Biopharma of Boehringer Ingelheim itself, and from other sources around the world.
The mission of Biobase is the integration of biologics development and manufacturing data to create a high-quality FAIR data landscape. Biobase aims to enable fast data and model-driven decision-making and digital innovation for biologicals development and manufacturing.
The training material for Biobase users consists of short walkthrough videos of around 5-10 minutes, documentation, and in-person training sessions given by the Biobase team.
Benefits of the Biobase approach include enhanced data quality and consistency, as well as a facilitated and automated data visualisation and analysis.
The motivation for the development in Biobase is sourced in the following use cases:
● Data-sharing: Data is shared and discussed with peers directly within Biobase and there is no need for email, download or Excel spreadsheet sharing.
● Reporting and visualisation: The Biobase user interface allows users to select, filter, and display the data needed for reporting. An export in PDF, XLSX or CSV format is possible. Besides the user interface, Biobase allows us to link the data with other visualisation tools (e.g. Spotfire) which gives the user more flexibility to build up specific reporting, visualisation and data modelling requirements.
● Data first: The harmonised data within Biobase enables data-driven experimental planning by using data from previous projects for scientific decisionmaking. It is the basis for any kind of cross-project data analysis such as modelling.
● Faster decisions: Biobase allows users to back up data-driven project decisions with cross-project and cross-scale data. Decisions can thereby be made much faster.
● Troubleshooting: Biobase can, for example, display the connection of different process steps within biopharmaceutical development, or easily show the information of used media. This enables fast and easy troubleshooting and root-cause analysis in case of problems. Direct correlations across the processes are easily done in Biobase.
● Transfer of a process: Data coming from different manufacturing sites is directly available and harmonised in Biobase. This saves time in data collection and reporting.
● Submission and filing of a proce ss: Biobase supports rapid submission and filing due to facilitated data collection and reporting options.
● Automated modelling: Being able to access and programmatically ( Python/R/SQL /etc.) process Biobase data without the need to export it, allows us to easily embed the data in modelling workflows. Due to the harmonisation of the data, an upscaling of workflows – for example from one project to many projects – is really easy with Biobase. Thereby sophisticated analyses are possible and support the transition to in-silico development. Further, the possibility of leveraging cloud computing resources is available.
The value proposition of Biobase spans various aspects of the biopharmaceutical development process. Firstly, it accelerates pipeline impact, affecting each biopharmaceutical product from the start of development through to submission to health authorities, and even during the commercial production phase of a product. Specific use cases, especially at interfaces during transfers between different sites, have the most significant impact. In instances of troubleshooting, the ability to instantly access data is crucial, making Biobase integral to different concepts and necessary for fast drug development.
Secondly, Biobase contributes to cost efficiency and savings. Almost every workflow requires data for reporting, team discussions, and much more. In terms of operational excellence, Biobase ensures that all workflows use a single source of truth for data. The workflow requirements are designed and implemented by SMEs and key users, enhancing customer centricity.
From a security and compliance perspective, Biobase operates at a non-GxP (GxP = good practice quality guidelines and regulation within pharmaceutical and food industry) compliance level, with full technical implementation of data integrity measures. Data
security and compliance are ensured by utilising Boehringer’s infrastructure and technology.
In the realm of sustainability, environmental, and social considerations, Biobase’s ability to find and analyse historical data avoids the need for repeating similar experiments, fostering a data-first approach. This also aids in shifting mindsets by leveraging synergies across different departments.
Finally, for business continuity, it is imperative to establish data roles and responsibilities for data standards to ensure a continuous run mode for Biobase. As more and more workflows rely on Biobase data, the need for business continuity measures built into the data pipeline becomes increasingly important.
BIGGEST CHALLENGES AND HURDLES
The biggest challenges within the development of Biobase are:
● Heterogeneous landscape of data sources ranging from pure Excel tables to diverse kinds of databases.
● Different ‘vocabulary’ of experts as well as often undefined naming conventions along the process chain in bioprocess development and along the bioprocess itself.
● Cultural barriers: digitalisation should not be seen as a different discipline but should be part of everyone’s work on all levels. It is the basis of every model generation and data interpretation, but is often tedious and needs an investment.
How to overcome these challenges is discussed in the following chapters.
TECHNICAL BACKGROUND OF BIOBASE TO COMBINE DIFFERENT DATA SOURCES
The data coming from the very heterogeneous landscape of data sources is collected within Biobase. Several automated steps of data pre-processing and data harmonisation exist, as well as data linkage tools to ensure consistent data reporting and scientifically sound representation of data categories (see Figure 1).
The data can be shown as given in the source systems to reproduce the original presentation, or in a harmonised manner which allows an immediate reporting and analysis of data coming from different sources.
During the data processing, Biobase ensures documentation and traceability of the process by using the following tools and features:
Technical tools:
● Bitbucket: Version control, track changes in configurations
● Jira/Confluence: Critical Biobase tables and columns are well-documented in confluence pages, showcasing the concept and specifications, contributing to the credibility of the data
● Backend tools: Automated pipeline monitoring, data drift alerts
● CI/CD: E2E function testing on unit features, data quality gates
Procedures:
● Microsoft Teams: bug reporting and user feedback
● SME community: collaboration for data inspection and verification
Biobase employs a data lineage interactive notebook to represent the flow of data, simplifying the understanding of complex data journeys (see Figure 2). Data quality checks are performed during harmonisation and then reported in so-called data drift reports. In more detail, the basic principle of ensuring data lineage is to apply a unique hash value to each ingested raw data point that will always be kept throughout the data journey. As a result, it is technically possible to trace each data value in the results tables and graphs back to its original raw data point that was originally ingested.
2
Data journey. Raw data tables are transferred to intermediate tables where rigorous data validation checks and quality assessments are performed and reported (data drift reports). Final tables can be tracked back to the source system via hash values.
To overcome the challenge of having different vocabulary within biologicals development, Biobase harmonises the data coming from different data sources. Biobase uses a harmonisation tool which provides a clear and complete view of mapping rules defined by the SMEs, further enhancing transparency and traceability throughout the data transformation process. The developers of Biobase do have a specific account type that allows them to influence the harmonisation rules for the respective data pool they are working on. The release and the changes are documented. The users of the Biobase productive system do not have access to the harmonisation rules as such. The standard user cannot change the data pipeline and cannot change any data in the source
systems. The Biobase team does not change any raw data value as the data is ingested from a data warehouse only. An audit trail records data transformation, source details, and any changes made during integration. Version control is implemented to track modifications and ensure transparency.
With the se measures, the segregation of duties is assured (see Table 1) and the workflow of the data harmonisation is separated into the two Biobase environments, i.e. development (Dev) and productive (Prod) environment:
Biobase Dev Environment – Harmonisation rules are settled, defined and verified Biobase Dev Environment – Data testing by SMEs Biobase Prod Environment – Documented implementation and release
Standard userRead-only, no access to harmonisation rules
Biobase SME Harmonisation and verification of harmonisation rule s
Developer Generation of harmonisation rules in (Biobase Dev)
Administrator Releases new features and harmonisation rules into (Biobase Prod)
Table 1
Segregation of duties
The following data-sharing terms are given in Biobase:
● Purpos e of Data-Sharing: The purpose of datasharing is to have a combined platform for data access across different source systems and is purely non-GxP, independent of the GxP status of the source systems.
● Data Ownership: The data is owned by the user of Biobase and he takes full responsibility for data correctness and completeness.
● Data Privacy and Security: The data is not protected or private, i.e. a user of Biobase can see all data but cannot change the data.
● Data Access: All employees from Boehringer Ingelheim could in theory have access after successfully accomplishing the necessary training.
● Data Usage: The data shall be used solely for nonGxP purposes.
● Data Retention: The data is available in Biobase until the next update from the source as it reflects the status of the source systems (updates are usually done within 24 hours).
● Data Quality and Inte grity: The data is shared as given in the source systems or in a harmonised manner.
Biobase adheres to the application of the diligence measures of ALCOA principles, in the source systems and in good scientific practice:
● Attributable: Biobase can trace back each data point to the original data entry into the respective source system.
● Le gible: The data represented in Biobase is sorted according to the same criteria as in the source systems and enriched with process context, which should improve the legibility according to the scientific standards applied.
● Contemporaneous: The pipeline of Biobase runs once per night automatically. Time and date stamps of the original raw data are copied and not altered by this process. However, Biobase provides additional control over changes in the source system, as a comparison of the same hashtag data is possible day by day. A data drift or change therefore can be automatically detected (data drift detection). As a side note: data drift detection also helps to identify potential errors in the data pipeline and serves as an additional safety feature.
● Original: There is an additional safety margin for originality built into the Biobase system. The need to generate uncontrolled copies of the data, e.g. in Excel spreadsheets, is minimised, as you always
have access to the original data. Despite Biobase being a copy of the raw data, in fact the introduction of hash values for each data point makes it always possible to assign the data to the right raw data value.
● Accurate: As far as the scientific accuracy is concerned, the numerical value of the processed data is not at all altered in Biobase. There is only a harmonisation taking place to make sure that all the units and method descriptions are properly used, and in this respect, the data could be equipped with additional metadata. In case of the unit harmonisation and yield calculation, there is always the option to go back to the original value with a potentially different unit used in the raw data. Accuracy is often also defined as using the right number of digits and rounding principles. Therefore, rounding and truncation of the data is only performed on the copy (e.g. for better representation of the diagram). In the background, behind the respective hash value, there is always the value with the original number of digits unaltered.
In Biobase, all data is processed, as there is no pre-selection of data occurring. Biobase resides in an IT-controlled environment with backup and lifetime concept, so the data in Biobase will be protected from any loss or copy-paste error which can arise in manual data processing.
Finally, the availability and shareability of Biobase analysis makes a peer-to-peer review of findings much more transparent, and one can always refer to the dataset used in the analysis directly on Biobase.
Digitalisation is not a different discipline (such as IT) but should be accessible to everyone on all hierarchical levels. It is well known that a datapoint without the necessary metadata is more or less useless in modelling approaches. Thus, not only the amount of data matters, but also the description of the data, to overcome the inconsistency problem which often occurs in data analysis. Biobase has proven in many use cases to be the solution to this problem: having good, documented, harmonised data is worth the investment! Besides the allocation of budget, efficient planning of resources and prioritisation of different tasks is most important to achieve cross-functional and global stakeholder management during the Biobase development. A constant recruitment of new users and use cases is essential to meet and align to the needs of the whole biologicals organisation.
BETTINA KNAPP
Biobase is an internally developed web application by Boehringer Ingelheim that provides access to a harmonised dataset of biological development and manufacturing data. The application, developed by a team of developers, SMEs, a scrum master, and a product owner, aims to integrate biologics data to create a high-quality FAIR data landscape.
The application serves various departments within Biopharma of Boehringer Ingelheim worldwide, and offers benefits such as enhanced data quality, consistency, and credibility. Major use cases include data-sharing, reporting and visualisation, data-driven experimental planning, faster decision-making, troubleshooting, data transfer, submission and filing, and automated modelling.
Despite the heterogeneous landscape of data sources and the cultural concerns related to digitalisation, Biobase manages to combine different data sources through a series of automated
data pre-processing, harmonisation, and linkage steps. The application ensures data lineage and transparency through robust methods and welldocumented specifications.
Biobase harmonises data to create a joint data ‘vocabulary’ and maintains segregation of duties to ensure data integrity. The data-sharing terms are designed to ensure data ownership, privacy, security, access, usage, retention, quality, and integrity.
The application adheres to the ALCOA principles, ensuring that each data point can be traced back to the original data entry, is legible, contemporaneous, original, and accurate. Biobase emphasises that digitalisation should be part of everyone's work, not a separate discipline and that the value of data lies not only in its quantity but also in its description. With that, we are very pleased to pave the way for any data analysis tools such as AI or machine learning to be used by everyone within Boehringer Ingelheim Biopharma in an easy and user-friendly manner.
HENRIEKE MAX is a data science manager at Delivery Hero, a German multinational e-commerce company based in Berlin. She works with analysts, data scientists, machine learning engineers and product managers to optimise the assortment and content of Delivery Hero’s Quick Commerce business. Prior to entering the tech industry and leading full-stack data teams, she held positions in policy research in the humanitarian aid sector as well as in management consulting with a focus on the public sector and big data. Henrieke’s personal passion lies in using her learnings from the non-profit, public and private sector and her mixed background in quantitative methods, engineering, social sciences and psychology to contribute to industry debates on enabling purpose-driven AI.
Summer 2023, it’s quarterly planning time for my org at Delivery Hero. I massage my temples in an effort not to drift off mentally to the upcoming vacation, and use what brain juices I have left to absorb the discussion. ‘But are we doing something with AI?’, a product director enquires. I feel an inner chuckle. Even a tech-native company such as ours with a mature product org is not immune to AI-for-the-sake-of-AI conversations.
Years before my career in tech and data science began, I was working in the Policy team of the European headquarters of a global humanitarian aid organisation. In the simplest terms, my job was to advocate for greater adoption of cash delivery, rather than goods delivery, to people hit by conflict, war and natural disasters. The evidence that providing cash (rather than material forms of aid) was more effective was already robust then. Yet, screening and targeting eligible individuals or communities to receive cash was time-consuming, leading to delays in delivering aid in situations where time is of the essence. Much effort was
invested to deliver cash faster, more securely and more cost-efficiently using technology such as blockchain. Leveraging algorithmic tools to determine eligibility was still in its infancy, however. Fast forward a decade, the non-profit organisation GiveDirectly has creatively combined different types of data, from mobile phone usage patterns to satellite imagery and socioeconomic household surveys, to more swiftly identify eligible cash clients [1,2,3]. Using intelligent targeting, they delivered 300 million USD to vulnerable clients in 11 countries in the COVID-19 pandemic, achieving a 30% reduction in administrative costs [4]. During a 2022 US hurricane, the same organisation served over 3.3 million USD to around 4.7k affected households within hours and days, rather than weeks and months [5]. Yet despite obvious benefits, the adoption of machine learning for cash aid targeting across the public and non-governmental sectors has been cautious and slow.
Transitioning from the non-profit to the corporate sector has shaped my view of the AI hype of recent years. The corporate sector is leading the way in investing in AI, with a 25.2 billion USD investment in generative AI in 2023 alone, this being but a quarter of the total AI private sector investment [6] Arguably, returns are lagging behind investment. This is sometimes attributed to the so-called ‘AI skills gap’, referring to the discrepancy between the current capabilities of a workforce and the advanced technical know-how required to extract value from AI. While that can be part of the equation, I argue that headway in regard to core technical skills has been made, but we continue to face an AI product management skill gap. Many companies become so focused on proving the technical feasibility of AI that they lose sight of the purpose and the intended audience, resulting in significant resource waste. Irrespective of sector, a dedication to what consumers, users, actually need, as well as a deep understanding of the market conditions in which users operate, makes AI-powered products successful. In the non-profit world, strong value alignment, the scarcity of resources and pressure of public accountability and limited access to technical expertise act as organic safeguards from something-with-AI. This does lead to slow adoption and underutilisation of AI, but the inclination to focus on societal impact improves the odds for developing usercentric solutions when resources allow. The corporate world, on the other hand, is at risk of malinvesting or overinvesting, losing sight of genuine user needs and a problem-driven approach. Companies need to pay more attention to this risk in systematic ways. My goal is to illustrate why, particularly in the for-profit world, the
biggest AI gap lies in AI product management. We need conscious investment in data and machine learning product management capabilities. These have been largely neglected as corporates upskill or make strategic engineering and data hires. I’ll deep dive into this argument with three concrete steps on what filling the gap could look like:
1. Build a wareness for the full spectrum of reasons AI-based products might fail
2. Double-down on upskilling for AI product management
3. Make Product the glue of your AI strategy
AI-powered products have more potential points of failure than products not relying on models. Especially in the tech industry, technical or business failures are more likely to be anticipated than those relating to human nature.
Business: Products leaning heavily on AI components, hereafter referred to as AI products, require high upfront investments. These could relate to data sourcing and curation, time to reach a good-enough starter model and procuring the tools needed to productionise and maintain models at scale. Such products risk failing if they cannot deliver incremental value from early on or even lack the ability to demonstrate satisfactory results in later stages, losing leadership faith and support. A recent example is the discontinuation of the IBM Watson-McDonald’s partnership on AI-enabled drive-thru orders in summer 2024. Reports of mishaps, such as adding uncalled for quantities, confusing distinct user orders or unsolicitedly adding unconventional extras had been amassing. Three years following the start of the work on automatic order taking (AOT), the quality of user experience had not reached adequate levels to reduce labour costs while keeping the user experience consistent. The automated voice-ordering was fully halted for the foreseeable future[7]
Technical: AI models can behave in unpredictable ways. They may perform excellently during testing, then fail to reproduce the same performance once live on the product. How users will interact with AI-generated outputs is highly uncertain, and can influence the model performance. A much-cited example is Microsoft’s chatbot Tay, which groups of Twitter users deliberately targeted with racist and misogynist comments, leading the bot to produce more than 95k tweets of the same nature [8,9]. Engineering teams must be prepared to monitor systems closely, implement
[1] Aiken & Blumenstock, 2022 [6] Stanford Institute for Human-Centered Artificial Intelligence, Stanford University, 2024
[2] Aiken et al., 2022 [7] Sanders, 2024
[3] Byrnes, 2024 [8] Olavsrud, 2024
[4] Hart International, 2024 [9] Lee, 2016
[5] Keen, 2023
pre-cautious content filtering, and promptly make adjustments, such as adding additional safeguards, once a model has gone live. Inability to respond quickly can become a deterrent to user adoption or erode organisational faith that the product can work.
Human: Understanding the user context, preferences and subjective benchmarks is critical. AI products may be launched or discarded prematurely when judged solely by standard model metrics or by whether they objectively solve a problem.
● First, the performance of a model is usually measured in mathematical terms, with wellestablished machine learning (ML) and deep learning (DL) success metrics. However, model performance is both subjective and relative. In many use cases, users determine how good is good enough, using their own experience as a benchmark. A mediocre model could be sufficient if users perceive it as a relative improvement to what they knew to date, a flawless model can fail if concerns around safety, transparency, social stigma, cultural norms or other personal or social factors impede adoption. One example of this is the story of Zume Pizza. With a hefty 450 million USD in venture funding, Zume aspired to automate pizza making and delivery using robotics [10]. Their core value proposition did not resonate with pizza consumers, as the end product was not tastier, more affordable, delivered faster or in any other way better than traditional pizza prepared by humans. Had Zume focused on automating pizza making for restaurant partners or other upstream users in the supply chain who may have benchmarked the cost and speed of human-made pizza against robot-made pizza as the primary success metric, their product may have been evaluated more favourably. Zume is also a prime example of how an abundance of funding can deter genuine problem-solving and result in overindexing for AI.
● Second, not every task that can be simplified, automated, or enhanced by AI should be, particularly if it replaces the labour of human users. Data scientists (DS) and machine learning engineers (MLE) may have an inclination to replace effort with a model wherever it is technically feasible (i.e. the data is available, of good enough quality, the deployment infrastructure permits it). And yet, technical feasibility does not trump user desirability. As humans, having a sense of mastery and agency gives us purpose. Convenience or ease can be a selling point in some contexts, in others it can deter
adoption. Products that limit (human) users’ agency or autonomy can feel disempowering. Some AI products may attempt to facilitate users’ life to the extent that they challenge users’ expertise, reduce transparency, or cause them to lose touch with their ‘gut feeling’. Even if an AI product addresses an important problem, its acceptance hinges on whether users have sufficient trust and decide that the gains of the AI solution are greater than its trade-offs. This has been heavily misjudged by many, including FAANG [11] companies. A fitting illustration is facial emotion recognition (FER) technology. In 2017, Meta, then Facebook, patented a technology able to watch users through device cameras to identify their emotions based on their facial expressions as they browse social network content [12]. Applications are manyfold, and include leveraging users’ emotional states to determine which content and ads to display to them, or back-inferring the nature of existing content-based users’ emotions. While the business use cases are clear, users have spoken out against the usage of their, literally speaking, emotional data, by Meta and other tech giants. The dominant user sentiment was that this poses a violation of their rights as data subjects. There does not seem to be a user demand for this extent of personalisation, and Meta has since shelved not only its FER efforts, but even ceased features using facial recognition from photos and videos [13]
[14] based on concepts from Parasuraman & Riley, 1997 User trust and engagement for sustainable AI products
user rejects or avoids product despite benefits due to skepticism toward or lack of understanding of its capabilities
abilities are enhanced without over-reliance on product
ABUSE user does not use product as the product is overly complex, poorly matched to needs or undermining user capabilites MISUSE user complacency leads to errors/incidents when product fails to perform as expect
[14]
A key message from Parasumaran and Riley’s 1997 paper on ‘Humans and Automation’ is transferable to AI product management today: AI products must support, not supplant users’ abilities. PMs need to foster an appropriate level of both trust and critical engagement in users, so they make use of the AI product, yet understand its limitations.
Software engineering (SWE), ML and DL are increasingly interwoven, yet distinct crafts. Traditional SWE applications without AI components come down to providing instructions in the shape of fixed, hard-coded rules of behaviour. AI-applications fundamentally differ in that they learn from the past and may continue the learning to improve or remain relevant to the evolving needs of the user. AI products are therefore a ‘living thing’, requiring not just continuous integration and continuous development (CI/CD), but continuous model (re-)training and monitoring. This distinction between the static versus ever-changing nature of a product is pivotal to the user experience. Traditional SWE has been the formative force of the Product profession, but it is crucial for AI product managers to be versed at additional skills to lead the creation, launch and management of ML and DL products.
Estimating return on investment (ROI) of AI investments: Measuring ROI is arguably a key responsibility of all product managers (PMs). However, an AI PM needs to be even more attuned to the costs of technologies, including ML infrastructure, cloud resources, data acquisition and annotation, and time investment of engineering teams. These can explode quickly when building AI products. Delivery life cycles are often longer, particularly in the first iteration, meaning the lags of returns may be greater. Moreover, the cost of maintaining a high-quality AI product post-launch could likewise be higher than for products without algorithmic components. AI PMs need the ability to manage expectations and communicate around probabilistic outcomes to non-technical audiences. They need to know techniques to slice the data science product lifecycle to deliver incremental value, fostering an iterative and experimental development style. That also entails grasping the principles of machine learning operations (MLOps) and short-cuts for prototyping, such as working with pre-trained models whenever feasible. When it comes to returns, PMs need to distil which return or key performance indicator improvement is expected, i.e. a user facing one such as improving user experience through personalisation, or an internal one, such as saving money or time. Determining how returns can be measured is a difficult, but essential skill. The variable and context dependent behaviour of AI models can call for knowledge of more advanced experimentation tactics than simple A/B testing. Quantifying returns may require more complex attribution methods than launching products or features not involving AI.
[15] Hern, 2020
[16] Clarke, 2023
[17] Hanrahan, 2017; Vieira, 2017
Mapping user problems and needs to AI capabilities: An AI PM needs to grasp the possibilities and boundaries of ML and DL to have initial hypotheses about whether a user problem is solvable by AI. If it is, they must know how to work with full-stack data and engineers teams to distil which methods might be most appropriate. That requires a conceptual understanding of the types of data that exist, foundational statistics, types of learning (supervised, unsupervised, reinforcement) and the most common algorithms used within them. It also involves the ability to judge what data would be required and support creatively sourcing and validating its quality. Not least, an AI PM ought to know which model performance monitoring is apt for the solution being built. Monitoring is not always straightforward or fully automatable, and it’s a popular stage of the ML/DL lifecycle to neglect or skip altogether. An AI PM understands why observing model or data drift is vital to uphold the relevance of a model, and therefore product, to the user.
Integration of ethical, data privacy and compliance considerations: When optimising for user experience, particularly in a competitive, profit-oriented context, ethical, data protection and regulatory aspects may fall off the radar. For instance, X (then Twitter) had to admit to racial bias after users reported that its automatic photo cropping feature systematically favours the inclusion of white faces over Black ones in photo preview mode [15]. The facial recognition platform Clearview AI scraped photos without user consent [16] , violating laws such as the EU’s GDPR and resulting in lawsuits and regulatory bans. Uber’s autonomous vehicle program faced scrutiny for its non-compliance with safety regulations and testing protocols [17]. The risks of damaging user trust and violating laws are greater when leveraging AI. The likelihood of regulatory scrutiny or user callouts of product issues related to data privacy, discrimination and bias is high. AI PMs must hold and maintain up-to-date expertise on these topics and be able to translate them into product requirements. This entails protecting and enabling agency over user data, guaranteeing the rights of user data subjects for data storage, historical traceability and deletion. PMs must develop a solid understanding of AI-specific guidelines and laws (GDPR, AI Act) and of approaches for auditing models for bias and ensuring systems for AI explainability are in place. Standard monitoring solutions focus solely on model performance, and are insufficient to observe model bias or transparency about model rationale. AI PMs need to understand the nuances of monitoring and advise on product components operating in the backend that
users may not realise they need, but that protect them. For software and AI PMs alike, the main tenets of product management are market research, user needs, and strategy. Yet, PMs specialising in AI must learn
these additional skills to guide on executing the sweet spot of what makes a viable business, what engineering can do, what is in the users’ interest, and what is ethical, particularly when these areas are not clearly aligned.
Filling the AI product management gap to connect the dots
is it solvable with ethical, fair, compliant means? should it be solved by AI? user desirability can it be solved by AI? technological possibilities & engineering capabilities what is the user problem/need?
can it become a viable business (ROI)?
A better organisational awareness for what makes AI products less prone to failure and targeted investment in AI PM skill building can make single AI products successful. As a function owning checks and balances around the motivations, needs and expectations held around AI, Product also needs a strong voice in corporate AI strategies. Being conscious of business, tech and end users alike, AI product management is in a position to identify the macro needs for the pursuit of the AI roadmap. Concretely, it can help answer questions such as:
What type of infrastructure do we need mid-long term? This includes ensuring the tech stack matches the data storage and compute power needs to run the product at scale as its user base grows, planning for major tech migrations or code refactorings. It also includes ensuring the product’s AI ecosystem supports the desired deployment strategies to expose users to
new models (such as standard A/B testing, canary or shadow deployment) and duplication of efforts amongst data science teams are minimised via collaborative tools, algo libraries, and feature stores.
What level of agency and customisation over our product do we require? Product is well-placed to chime in on decisions around investments in engineering capabilities vs. infrastructure-as-a-service/managed services for flexible scaling and general guidance on build-vs-buy principles, as these depend highly on the value proposition of the product and user requirements.
What measures, tools or systems need to be in place to comply with brand, user and legal expectations for relevance, transparency, data privacy, fairness and bias prevention? These must be appropriate to the specific risk level of exposing users to AI within the industry and use case. Product management must define overarching risk categories and risk mitigation strategies
for each. For AI products, ensuring sensitive user data is safe, bias monitoring is being adapted to evolving needs, and intuitive explainability tools are accessible is key. Product strategising means thinking of these as core features of the product, planning for potentially crossproduct, scalable solutions and creating accountability mechanisms for ethical product development that are ingrained in the product life cycle.
How will the product remain relevant over time? This covers many aspects, from standards for model retraining, procuring fresh, clean and current data to ensuring the engineering teams and product functions are able to keep up to speed with AI developments and engage in continuous learning from academic and industry advances.
balance the training dataset (resampling, adjusting weights, modifying class labels)
remove discriminatory correlations between features and sensitive attributes
algo adjustments: impose fairness restrains on model’s objective functions through regularisation and restrictions
adjust train classifier to satisfy fairness constraints, e.g. modify decision thresholds for balanced outcomes; add filters
control model accuracy-fairness trade-off
The AI gap is shifting from tech to product: The 2023 State of AI Product Management report cites ‘Data privacy concerns’ and ‘Lack of understanding about AI among stakeholders’ as the top two challenges faced by PMs in the context of working on AI products. ‘Technical limitations’, on the other hand, are cited as the second to last challenge. When participating Product professionals were asked whether lack of access to ML and DS talent was hindering their AI product progress, 68% had affirmed in 2021, but only 43% responded affirmatively in 2023. The same report shows that 71% of surveyed organisations did not have a dedicated AI PM role in 2023 [19]
AI failures in the private sector, often in spite of massive investment, continue to be numerous. These are not limited to the average small to mid-sized enterprise.
add guardrails to preempt harmful outputs
Top tier tech companies have had to pause or scrap some of their signature AI undertakings. Particularly for the tech giants, a lack of data and engineering talent does not account for why some AI products fell through initially, or were even ultimately abandoned. It is time, I have argued, to shift focus from the AI gap in technical skills and capabilities to the AI product management gap. In the triangle of business, tech and user experience, the product profession is best placed to ensure user experience is not only considered, but comes first. In AI product management, user experience encompasses more than is visible to the users’ immediate perception. Model-fuelled products are moving targets, both influencing and influenced by users. The ‘human factor’ is much more far-reaching compared to products based on software engineering alone: be it understanding the individual psychological
and socio-cultural user considerations influencing whether AI-products get adopted or fail, the ability to assess if AI is truly needed to solve a user problem and which manifestation of it is most suitable and economically viable, or having a grasp of how data protection, legal and ethical requirements translate into technical product requirements. Beyond the singular AI-supported feature or product, companies must evolve to productise their AI strategy as such. Scale of infrastructure, agency over customisation of algorithms, systems for fairness, data protection and explainability are all critical product components directly or indirectly affecting the users’ needs and interests.
Closing the AI product gap boils down to training AI PM talent that is equipped for the many facets of the job. The upskilling effort should be no less rigorous than that for engineering and data science. Engineering and statistics skills of sufficient depth and ROI measurement are non-negotiable AI PM assets. But the AI PM’s core expertise lies in building sensible intelligent user experiences. And although the public
and non-profit sectors globally may not have nearly as much to show for when it comes to productionised AI in action, there are lessons to learn for the corporate sector as far as a focus on societal impact and accountability in the use of artificial intelligence is concerned. There are now countless frameworks, technical and methodological guidelines around the responsible, people-focused application of AI, such as by the World Economic Forum (PRISM) [20], advocacy organisations like the Algorithmic Justice League [21] and academic research outlets such as the Harvard Data Science Review [22]. Upskilling product functions for AI can leverage these resources, but will also need to involve more foundational teachings from psychology, social sciences, philosophy and legal studies.
For now, we are left with the irony that increasing tech know-how has enabled companies to do something-with-AI, commonly producing anythingwith-AI products. What they need now is rigorous AI product upskilling to create products that users actually need, and that can and should be AI-driven.
Author’s Note
Tara Dezhdar, Anela Hoti, and Alexia Vazoura offered ideas and constructive feedback on this article, drawing on their extensive experience in data science and Product. I thank them for generously sharing their expertise.
[20] Nowack et al., 2024
[21] Algorithmic Justice League, n.d.
[22] Luciano & Cowls, 2019
PHILIPP DIESINGER
By DR PHILIPP DIESINGER
DR PHILIPP DIESINGER
is a seasoned data scientist and AI expert with a PhD in theoretical physics from Heidelberg University. His career began with a postdoctoral position at the Massachusetts Institute of Technology (MIT). He has since held significant roles, including Head of Global Data Science at Boehringer Ingelheim and serving in a partner role at BCG.X. Currently, Philipp serves as a senior partner at Rewire, leading expert teams across the healthcare sector and beyond.
Artificial intelligence (AI) is increasingly shaping drug discovery, clinical trials, and healthcare decision-making. However, when AI models are trained on biased data, they can reinforce or even worsen existing healthcare disparities. Bias in healthcare data refers to systematic errors or skewed representations and it can lead to unfair or inaccurate outcomes for certain groups of patients. If left undetected, such biases can exacerbate existing health disparities and undermine trust in medical systems [1]. Global health authorities emphasise the importance of identifying and addressing bias in health data to ensure that advances like AI benefit all populations equitably [1]. Biases can enter at many stages – from how data are collected (e.g. which populations are included) to how algorithms interpret that data – making detection a critical step toward safer, more effective healthcare interventions.
In statistical terms, bias arises when a dataset is not representative of the true population, causing analyses or models built on that data to systematically deviate from reality [2]. In healthcare, this often means certain demographic groups (defined by race, gender, age, socioeconomic status, etc.) are underrepresented or misrepresented in the data. Social biases and systemic inequities can thus become encoded in healthcare datasets and algorithms, leading to worse outcomes for marginalised groups [2]. For example, an AI model trained mostly on data from one group may perform poorly on others, simply because it learned patterns that don’t generalise.
Detecting these biases involves scrutinising both the data and the outcomes of any algorithms using the data to ensure no group is being inadvertently harmed or overlooked.
Common sources of bias in healthcare data include the following:
1 | Sample Bias (Underrepresentation): Certain groups may be underrepresented in clinical trials, electronic health records, or genomic databases. For instance, over 90% of participants in genome-wide association studies have been of European descent, which limits the applicability of genetic findings to other ethnic groups [3]. Such sampling bias means medical knowledge and tools might be less effective for underrepresented populations.
2 | Measurement Bias: The way health data are obtained can introduce bias. A notable example is the pulse oximeter, a routine device that estimates blood oxygen. Studies found that pulse oximeters overestimate oxygen saturation in patients with darker skin, resulting in Black patients being nearly three times more likely than white patients to have low oxygen levels go undetected [4]. This bias in a common medical device illustrates how flawed data can directly impact care decisions.
3 | Algorithmic Bias or Feature Bias: Bias can also arise when algorithms use proxies in data that reflect societal inequities. A landmark investigation revealed that a widely used health risk prediction algorithm systematically underestimated the risk for Black patients compared to equally sick white patients because it used healthcare spending as a proxy for health. Due to unequal access to care (and thus lower healthcare spending for Black patients), the algorithm falsely judged Black patients to be healthier, resulting in fewer referrals for advanced care until the bias was detected and addressed [5] .
4 | Observer/Recording Bias: Human biases by clinicians or data recorders can creep into healthcare data. For example, if pain levels of certain patients are consistently underestimated due to implicit bias, those biases become part of the recorded data. Similarly, missing or inconsistent data (such as incomplete recording of a patient’s ethnicity or gender identity) can mask true patterns and make it harder to detect when outcomes differ among groups. Undetected bias in healthcare data can lead to inequitable care and poorer outcomes for certain groups. As the above examples show, biased data or algorithms might mean a serious condition goes unrecognised in
a minority patient, or resources are allocated unfairly. These issues compound existing health disparities. For instance, biases in diagnostic tools or decision support systems can further disadvantage populations already facing barriers to care. Research has documented that AI systems, if unchecked, may amplify societal biases – one study warned that biased AI could misdiagnose or mismanage care for underrepresented groups, potentially leading to higher error rates or even fatal outcomes [2]. Detecting and correcting bias is thus essential to ensure patient safety and fairness. Moreover, from an ethics standpoint, leading organisations like the World Health Organization (WHO) stress ‘inclusiveness and equity’ as a core principle for health AI – meaning technologies should be designed and evaluated so they work well for all segments of the population [1] Detecting bias is a prerequisite to building trust in data-driven healthcare innovations and ensuring they improve health for everyone, not just a subset.
Detecting bias requires a systematic and proactive approach to analysing both data and model outcomes. Key methods include:
Analysis: Examine the composition of healthcare datasets to check whether key demographic groups are adequately represented. This involves comparing dataset demographics (e.g. race, gender, age distribution) against the relevant patient population. Any major imbalance or gap (such as a lack of data on a certain group) is a red flag for potential bias. For example, auditing a national health record database might reveal that ethnicity data is missing for 10% of patients [6]. Such gaps need to be identified, as they can hide disparities or make certain groups ‘invisible’ in analyses. Proper dataset documentation can help record these characteristics and alert researchers to possible biases in the data.
When developing predictive models or AI in healthcare, it is crucial to evaluate their performance separately for different patient subgroups. Metrics like accuracy, error rates, sensitivity, or treatment recommendation rates should be compared across categories such as race, sex, or age. A significant disparity, for instance, an algorithm that has a much higher false-negative rate for women than for men, would indicate bias. In practice, the racial bias in the health risk algorithm [5] was detected by observing that Black patients with the same predicted risk score had worse actual health outcomes than white patients, prompting investigators to dig deeper. Similarly, checking a diagnostic AI on diverse test images might uncover that it performs
poorly on images from older machines or from certain hospitals – pointing to bias from differing data sources or quality [7] . Regularly reporting model performance by subgroup is now considered a minimum requirement in best practices for medical AI [7]
C | Statistical Fairness Metrics: Researchers can apply formal fairness metrics to quantify bias in healthcare models. These metrics (borrowed from the field of machine learning fairness) include tests for ‘disparate impact’ (i.e. does a model’s predictions affect one group disproportionately?), ‘equalised odds’ (are error rates equal across groups?), or ‘calibration’ (are risk scores equally meaningful for each group?). For example, one might calculate whether a diagnostic test has the same sensitivity for minority patients as for others. If not, bias is present. Statistical tests, like chi-square or z-tests for differences in proportions, can flag when differences between groups are unlikely to be due to chance. Using such metrics provides a more objective way to identify bias beyond just anecdotal observations.
D | Reviewing Proxy Variables and Model Inputs: Detecting bias also means scrutinising which variables (or features) are used in algorithms and whether they could be acting as proxies for protected characteristics. In the case of the biased risk algorithm, the use of ‘healthcare cost’ as a proxy for health status was the culprit [5] . By reviewing model features, analysts can sometimes spot features that correlate strongly with race, gender, or other sensitive attributes. If a feature is contributing to unequal outcomes, it may need adjustment or removal. Feature importance analyses and sensitivity tests (evaluating model output after toggling certain inputs) are useful techniques to uncover proxy bias.
E | Human-in-the-Loop Evaluation: Finally, involving domain experts and stakeholders can aid bias detection. Clinicians, for example, might recognise when an AI’s recommendations consistently underserve a group of patients, triggering a closer look at the
data. Patient advocacy groups can also provide insight into whether a model’s behaviour aligns with real-world experiences of different communities. This qualitative feedback can guide quantitative checks and vice-versa, creating a more robust bias detection process.
Identifying bias is only the first step – once detected, steps can be taken to correct or mitigate it. Approaches like collecting more diverse data, rebalancing datasets, or adjusting algorithms can help make healthcare data and tools fairer. For instance, if a dataset audit finds underrepresentation, targeted efforts can be made to include more data from the missing groups, such as launching studies in under-served communities or updating data collection practices. If performance disparities are found, developers might retrain models with bias mitigation techniques or introduce calibration factors to even out the outcomes. There is a growing movement in healthcare informatics to establish standards for fairness. An international consortium of researchers and clinicians recently published consensus recommendations on improving transparency and diversity in health datasets to combat algorithmic bias [8]. They call for measures like documenting dataset demographics, evaluating AI tools for bias before deployment, and involving diverse stakeholders in development [8]. Such guidelines echo the WHO’s principles and provide concrete steps for organisations to follow.
It is also worth noting that regulatory bodies and journals are increasingly urging bias evaluations as part of clinical AI validation [7]. The field is moving toward a future where claims of algorithm performance must be accompanied by evidence that the model was tested for bias and is safe for all patient groups. By integrating bias detection into the standard workflow – from data gathering to model training to deployment –healthcare providers can catch problems early and avoid propagating injustices. Detecting bias in healthcare data is essential to ensure equitable and effective care. By understanding the sources of bias and diligently auditing data and algorithms, healthcare researchers and professionals can uncover hidden disparities.
REFERENCES
[1] World Health Organisation (WHO). Ethics and governance of artificial intelligence for health: WHO guidance. Geneva: WHO; 2021. iris.who.int
[2] Norori N, Hu Q, Aellen FM, Faraci FD, Tzovara A. Addressing bias in big data and AI for health care: a call for open science. Patterns (NY ). 2021;2(10):100347. doi:10.1016/j.patter.2021.100347. pubmed.ncbi. nlm.nih.gov
[3] Bustamante CD, Burchard EG, De la Vega FM. Genomics for the world. Nature. 2011;475(7355):163-165. doi:10.1038/475163a. nature.co
[4] Sjoding MW, Dickson RP, Iwashyna TJ, Gay SE, Valley TS. Racial bias in pulse oximetry measurement. N Engl J Med . 2020;383(25):2477-2478. doi:10.1056NEJMc2029240. nejm.org
[5] Obermeyer Z, Powers B, Vogeli C, Mullainathan S. Dissecting racial bias in an algorithm used to manage the health of populations. Science. 2019;366(6464):447- 453. doi:10.1126/science.aax2342. science.org
[6] Pineda-Moncusí, M., Allery, F., Delmestri, A. et al . Ethnicity data resource in population-wide health records: completeness, coverage and granularity of diversity. Sci Data 11, 221 (2024). doi.org
[7] Chen, R.J., Wang, J.J., Williamson, D.F.K. et al. Algorithmic fairness in artificial intelligence for medicine and healthcare. Nat. Biomed. Eng 7, 719–742 (2023). doi.org
[8] Alderman JE, Palmer J, Laws E, et al. Tackling algorithmic bias and promoting transparency in health datasets: the STANDING Together consensus recommendations. Lancet Digital Health . 2024; (in press). doi:10.1016/S2589-7500(24)00224-3. thelancet.com
Ah, the tech industry. The same industry that once worshiped programmers now treats them like relics from an ancient civilisation, like scribes who refuse to accept the printing press. Companies are convinced AI is the answer to everything, and programmers? Well, they’re just expensive, opinionated, and worst of all, human. But here’s the thing – if you think cutting programmers in favour of AI is a genius move, you might want to remember the last time a company fired all its engineers: it ended in lawsuits, product failures, and a desperate rehiring spree. But sure, go ahead. Lay them off. You’ll regret it faster than you can say ‘syntax error.’
Let’s break this down properly. Three things are about to happen, and none of them are good for companies that think AI will replace programmers:
Once upon a time, aspiring programmers cut their teeth on real problems – fixing code, breaking systems, and learning from grizzled veterans who’d been through a thousand production crises. They learned how to optimise performance, deal with weird hardware bugs, and – most importantly – how to think like an engineer, not just type words into a compiler.
But with the AI craze, companies aren’t investing in junior developers. Why train people when you can have a model spit out boilerplate? Why mentor young engineers when AI promises to handle everything?
Spoiler alert: this is a terrible idea.
The next generation of programmers will grow up expecting
FRANCESCO GADALETA is a seasoned professional in the field of technology, AI and data science. He is the founder of Amethix Technologies, a firm specialising in advanced data and robotics solutions. Francesco also shares his insights and knowledge as the host of the podcast Data Science at Home
His illustrious career includes a significant tenure as the Chief Data Officer at Abe AI, which was later acquired by Envestnet Yodlee Inc. Francesco was a pivotal member of the Advanced Analytics Team at Johnson & Johnson. His professional interests are diverse, spanning applied mathematics, advanced machine learning, computer programming, robotics, and the study of decentralised and distributed systems. Francesco’s expertise spans domains including healthcare, defence, pharma, energy, and finance.
AI to do the hard parts for them. They won’t know why an algorithm is slow, they won’t be able to debug cryptic race conditions (provided they are familiar with the concept), and they certainly won’t know how to build resilient systems that survive real-world chaos. It’s like teaching kids to drive but only letting them use Teslas on autopilot – one day, the software will fail, and they’ll have no idea how to handle it.
The result? We’ll have a whole wave of programmers who are more like AI operators than real engineers. And when companies realise AI isn’t magic, being just a bunch of tokenised words in line (prove me wrong on that), they’ll scramble to find actual programmers who know what they’re doing. Too bad they spent years not hiring them.
FRANCESCO GADALETA
Imagine a company that fires its software engineers, replaces them with AI-generated code, and then sits back, expecting everything to just work. This is like firing your entire fire department because you installed more smoke detectors. It’s fine until the first real fire happens.
Let’s say you’re a big fintech company. You fired half your dev team because ‘AI can write code .’ Now, six months later, you realise that your AI-generated software is riddled with security holes. Whoops! Your database is leaking private financial data like a sieve, regulators are breathing down your neck, and customers are fleeing faster than rats from a sinking ship. The AI that wrote your software? It doesn’t care. It doesn’t fix bugs. It doesn’t ‘own’ the problem. It just generates more broken code, like a toddler smashing LEGO bricks together and calling it a house. What do you do? You try to rehire the programmers you laid off. But guess what? They’ve moved on. The good ones are at startups or working on their own projects. Some are consulting for obscene rates. And now your company is left with AI-generated spaghetti code and no one to fix it.
Now, let’s talk about the real winners in all this: the programmers who saw the chaos coming and refused to play along. The ones who didn’t take FAANG jobs but instead went deep into systems programming, AI interpretability, or high-performance computing. These are the people who actually understand technology at a level no AI can replicate.
And guess what? They’re about to become very expensive. Companies will soon realise that AI can’t replace experienced engineers. But by then, there will be fewer of them. Many will have started their own businesses, some will be deeply entrenched in niche fields, and others will simply be too busy (or too rich) to care about your failing software department.
Want to hire them back? Hope you have deep pockets and a good amount of luck. The few serious programmers left will charge rates that make executives cry. And even if you do manage to hire them, they won’t stick around to play corporate politics or deal with useless middle managers. They’ll fix your broken systems, invoice you an eye-watering amount, and walk away.
The tech industry is making a massive mistake. By believing AI can replace programmers, it’s killing the very ecosystem that keeps innovation alive. We’re about to enter a world where:
● Junior programmers will be undertrained and over-reliant on AI.
● Companie s that fired engineers will be scrambling to fix the mess AI-generated code leaves behind.
● The be st programmers will be so rare (and so expensive) that only the wealthiest firms will afford them.
But hey, if tech companies really want to dig their own grave, who are we to stop them? The rest of us will be watching from the sidelines, popcorn in hand, as they desperately try to hire back the programmers they so carelessly discarded.
Good luck, tech industry. You’re going to need it.