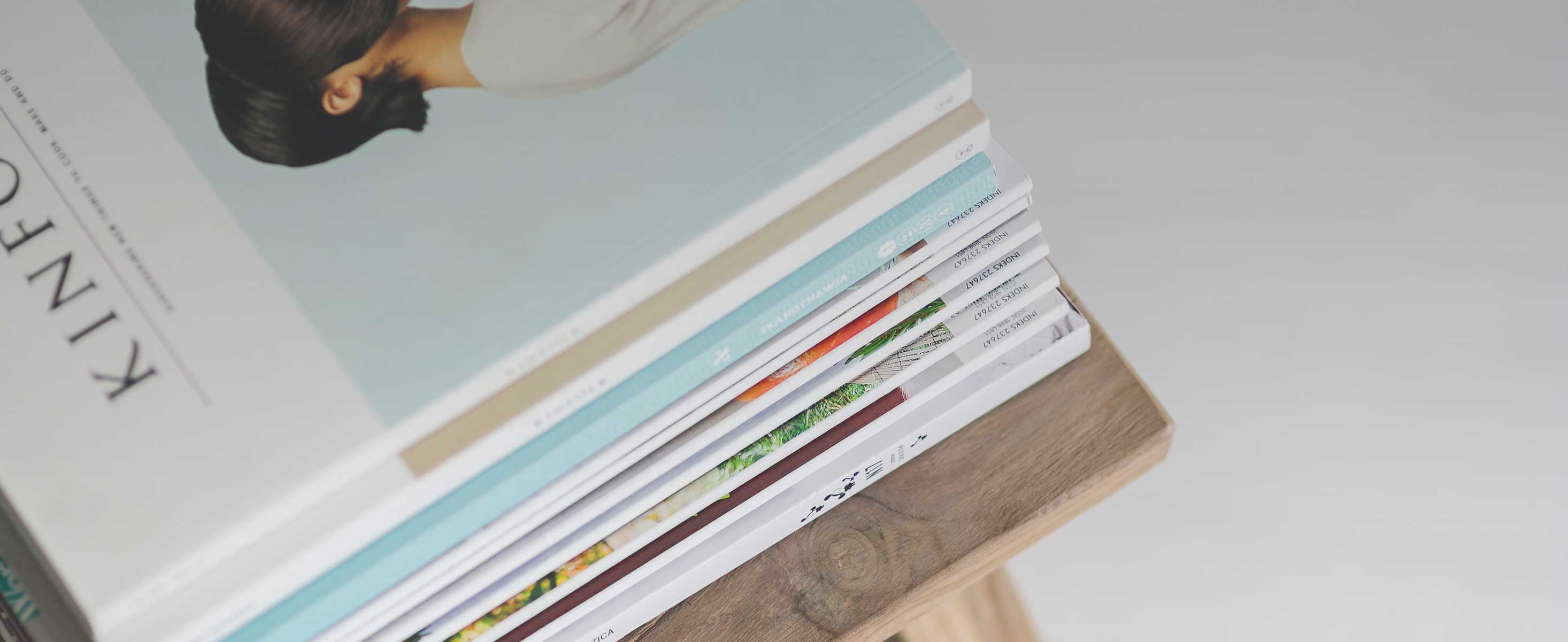
8 minute read
6.3 Illustration of PMT Weights for Selected Variables
How to Harness the Power of Data and Inference | 393
example, 2, 5, 10, 15, 20, and 30 percent) to estimate the model’s predictive power.51
Once the modeling is complete, the scoring formula can be easily read by analysts and policy makers. Table 6.3 shows that predicted welfare increases for households whose heads have secondary or higher education and households with piped water inside the dwelling, electricity or solar panels for lighting, and gas/liquefied petroleum gas for cooking fuel.
Updating the Model and the Social Registry
Once it is calibrated, the model will continue to estimate the level of welfare with the same level of precision if the (partial) correlations between the variables selected in the scoring formula do not change, but of course they eventually will. Therefore, a good practice is to revisit the modeling approach, revisit the cutoff points, and retest the precision of the formula after two or three years, whenever a new representative household survey becomes available, or whenever the social registry or data system
Table 6.3 Illustration of PMT Weights for Selected Variables
Caretaker characteristics
Age
Educational level
Mark one of the codes below regarding your highest school grade attained PMT weights
[ ] in years 0.0003 0. No education 0
1. Primary 0
2. Secondary or higher 0.0881
What is household’s main source water over the past month?
Piped water inside dwelling
[ ]
Rain, unprotected dug well/spring, river, lake, pond, or similar
[ ]
Other
[ ]
What is household’s main source of lighting fuel?
Firewood
[ ]
Electricity, solar
Other What is household’s main source of cooking fuel?
Firewood
Gas/LPG
Other
Source: Original compilation for this publication. Note: LPG = liquefied petroleum gas; PMT = proxy means test. 0.4471
0
0
0 0.1178 0
0 0.5607 0
394 | Revisiting Targeting in Social Assistance
recertification cycle is completed (in static systems), or whenever a major program expansion occurs. The following examples illustrate these cases: • Georgia assesses the performance of its main antipoverty program, the
Georgia Targeted Social Assistance Program (TSA), every year (thanks to the regular production of representative household surveys that include information on receipt of TSA). It updates the PMT scoring every four to five years (see box 6.6, which illustrates the type of analytical work and the political economy of updating the formula). The TSA program started in 2006, and the formula was updated in 2010, 2015, and 2020. • In Colombia, revisions to the PMT formula are made with each new survey sweep that updates the SISBEN social registry.52 With each new cycle, based on academic studies, the National Planning Department changes the modeling technique, the number and delineation of regional models, and the variables used. SISBEN I used one national model using principal components; SISBEN II used different OLS models for urban and rural areas; SISBEN III used three OLS models—one for the 14 cities, one for other urban areas, and one for rural areas; and SISBEN IV uses multiple machine learning–PMT models differentiated by department and rural/urban areas. • The Palestinian Authority has reviewed its PMT formula as new data have become available. The Cash Transfer Program was created in 2010.
The PMT used for its targeting was based on the then most recent available household survey, from 2007. The formula used regional cutoff points (one for Jerusalem, one for the West Bank, and one for Gaza).
During 2011, the Palestine Expenditure and Consumption Survey 2009 was made available, and the formula was reassessed with the new data.
The improvements in targeting accuracy that could be obtained by simply updating the formula were considered not enough to warrant a change; instead, attention was given to improving the delivery system.53
A new round of the survey was fielded in 2017, data were made available in 2019, and since then, the Palestinian Authority has carried out the analytical work to update the formula, modify the regulatory framework, and adjust the delivery system. The Ministry of Social Development is now starting the process to recertify beneficiaries and apply the new formula as part of strengthening the Ministry of Social Development’s social protection system. • In Tanzania, revisions to the formula follow the availability of new data but with some lags related to when the data are available and when a new phase of program intake is due. The government piloted a community-based conditional cash transfer program during 2008–12,54 which was subsequently scaled up into the Productive Safety Net
Program. The process evaluation for the pilot suggested that the
How to Harness the Power of Data and Inference | 395
implementation of the two-phase PMT it had used was problematic for logistical reasons. Thus, before the phase I scale-up in 2011, a new
PMT model was estimated, still on the basis of the 2006 Household
Budget Survey. The next round of major revisions to the formula was triggered by the next phase (II) of scale-up in 2015 and the availability of data from the 2011 Household Budget Survey. The formula was revised again in 2020 using the 2018 Household Budget Survey, again in time for a new phase of scale-up to full national coverage. All three rounds of revisions made in 2011, 2015, and 2020 included reweighting and adding/dropping a few variables.
As discussed in papers on poverty and inequality decomposition,55 household welfare changes over time can be understood mostly in terms of changes in observed characteristics of the population and the returns to those characteristics. Changes in observed characteristics mean that the average characteristics of the poor have changed over time. For example, 10–15 years ago, possession of a cell phone or having connectivity to water or sanitation services could be associated with not being poor. Nowadays, cell phone coverage is extensive in most countries and large sanitation programs may have improved accessibility to potable water and sanitation in poor areas, so those variables are no longer close correlates of poverty in many countries (although a smartphone may be). Changes in returns to those characteristics mean that the value of a given characteristic has changed. For example, the value of a high school diploma relative to not completing high school may change as more people complete high school, or it may depend on the skills sought as technology changes or as trade and competitiveness policies, prices for major commodities, and the like influence the demand for different skills in the labor market.
Accounting for these potential determinants of changes in the distribution of welfare, periodic updating of a model should consider its various features, that is, whether (1) characteristics or returns to characteristics have changed, (2) adjustments are needed for use of the model by different programs, (3) new poverty lines/thresholds must be estimated, and (4) modeling errors due to past modeling limitations and low-quality data would improve if new data were used.
Poverty decomposition models such as the Oaxaca-Blinder decomposition can be used to assess the need for updating the traditional PMT model using the new data. The decomposition consists of estimating the difference in returns to characteristics over time by comparing the coefficients of the selected variables in the model for year t and year s and estimating the differences in the characteristics themselves by comparing the matrix of characteristics used in the model for both years.56 The process is as follows:
396 | Revisiting Targeting in Social Assistance
(1) Estimate the same model used for estimating the PMT in year t on the new household data for years to run a chi-squared statistic test to compare the equality of both vectors of PMT weights (price effect). (2) Run a chi-squared statistic test to compare the equality of the matrixes of covariates used for the PMT model for years t and s (characteristic effect).
In practice, the assessment will generate two stylized situations: (1) There is a price effect, for example, the vectors of PMT weights are different. The solution is to use the same PMT model but estimated on year s and adjusting the poverty line/threshold as the price effect implies economic growth and inflation or economic contraction with no changes in the determinants of poverty. (2) There is a characteristic effect, for example, the distributions of the explanatory variables are different (or there can be both price and characteristic effects). The solution is to consider a general model change by adding or removing explanatory variables and adjusting the poverty line/threshold using year s data, as the determinants of poverty and the macroeconomic environment have changed.
Recent poverty assessments and other studies would indicate whether the poverty and inequality determinants may have changed. In this case, the test presented above will corroborate the findings of the poverty assessment, while the reports will guide the data analyst to identify new variables that could be added to the model.
Changing the model to add new variables has implications for the delivery system processes of social programs and the political economy. First, adding new variables may mean revising the full intake questionnaire, which involves changes in communication, outreach, software, training of staff, new intake and registration process, and so forth. Second, a new set of PMT weights means having new scores for each person and maybe new thresholds, so some current beneficiaries may lose benefits, while others may need to be included. Hence, there are winners and losers due to the new model, which requires new processes for onboarding, communications of rules and responsibilities, and so forth. Box 6.6 describes how this was handled in Georgia.
Another issue is that PMT models are based on household socioeconomic characteristics, and transfers can have a direct impact on those characteristics. A poor beneficiary can use the transfers to improve their human capital and productivity, buy an asset, repair housing, and so forth. Such improvements caused by the transfers affect the characteristics that initially characterized the household as poor. Applying the updated PMT for a current beneficiary household would lead to a better score simply