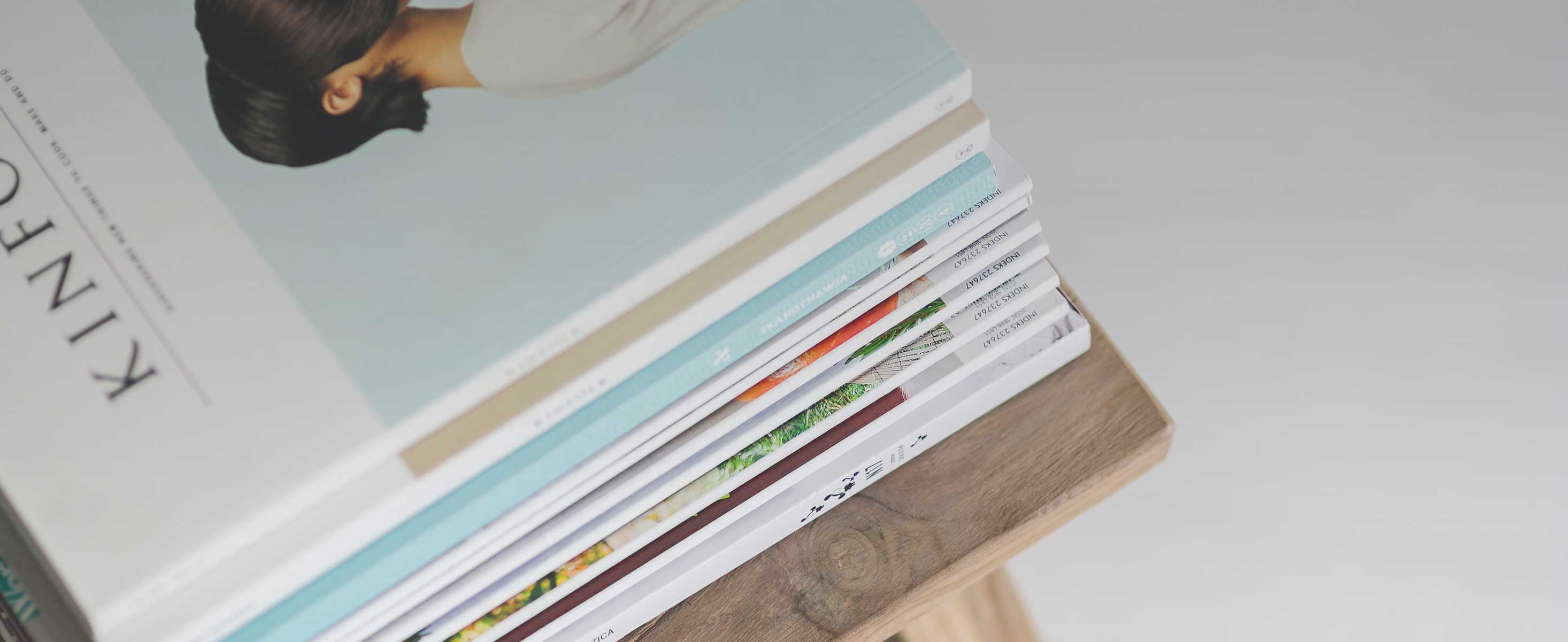
4 minute read
7.3 Inclusion and Exclusion Errors in a 10-Person Economy
474 | Revisiting Targeting in Social Assistance
reduce poverty. In this economy (table 7.3), the poverty headcount is estimated at 40 percent, and the government has allocated $30 for a transfer program. The observed total income gap is $40, which means that the current budget allocated would not suffice to eradicate poverty. As the budget is smaller than the needs, policy administrators decided that it would be better to use this budget to reach the poorest 20 percent (the relatively poorest for sure, perhaps labeled the extreme poor) instead of all the poor. They would like to compare such a program with a universal program that might avoid social tensions and promote solidarity across the population. Four scenarios are presented as follows: • Scenario 1 depicts a process that has perfect inclusion of the poorest two individuals. • Scenario 2 depicts a process with all benefits going to poor individuals but to only one of the two extreme poor individuals. • Scenario 3 depicts a process with all benefits going to poor individuals but missing the two poorest individuals. • Scenario 4 reaches all individuals.
Table 7.3 illustrates the economy and presents the two most commonly used metrics for measuring performance: exclusion and inclusion errors (see the formulae in annex 7A). First, the errors are estimated against two benchmarks: the real poverty line and a relative poverty line that was used as the eligibility threshold (the poorest 20 percent).
Table 7.3 Inclusion and Exclusion Errors in a 10-Person Economy
Person 1 2 3 4 5 6 7 8 9 10 Total Observed income ($) 6 8 11 15 21 25 30 40 50 100 306 Income gap ($) 14 12 9 5 0 0 0 0 0 0 40 Poor 1 1 1 1 0 0 0 0 0 0 4 Poorest 20% 1 1 0 0 0 0 0 0 0 0 2
PL: EE: 50%; IE: 0%
Scenario 1 1 1 0 0 0 0 0 0 0 0 2 P20: EE: 0%; IE: 0%
PL: EE: 50%; IE: 0%
Scenario 2 0 1 1 0 0 0 0 0 0 0 2 P20: EE: 50%; IE: 50%
PL: EE: 50%; IE: 0%
Scenario 3 0 0 1 1 0 0 0 0 0 0 2 P20: EE: 100%; IE: 100%
PL: EE: 0%; IE: 60%
Scenario 4 1 1 1 1 1 1 1 1 1 1 10 P20: EE: 0%; IE: 80%
Source: Original compilation for this publication. Note: EE = exclusion error; IE = inclusion error; PL = poverty line; P20 = relative poverty line (the poorest 20 percent).
Measuring the Performance of Targeting Methods | 475
Although there is a rationale for each of the benchmarks, the numbers and their interpretations are different. Using the benchmark of the 40 percent poverty line finds very high exclusion rates that may be read as poor performance of the method when the problem is that the size of the program is smaller than the size of the population of interest. A program for 20 percent of the population when 40 percent of the population is below the poverty line will have at least 50 percent exclusion errors, as it can only cover one-half of the poor population even if all those included are poor. Conversely, it is easier for small programs to avoid inclusion error. In this economy, measured against the poverty line, the three small program scenarios have no or smaller inclusion errors despite the 50 percent exclusion error as program size for the first three scenarios is smaller than the poor population. At the same time, looking only for exclusion error hides the significant inclusion error in scenario 4, which is 60 percent.
Mistakenly looking at the poverty line as benchmarking, the first three scenarios have similar errors. Looking at the actual targeted population, that is, the bottom 20 percent, reveals nuances in performance. Scenario 1 has a perfect outcome with 0 percent errors as designed, and scenario 3 has the worst outcome with 100 percent error. Therefore, the example illustrates that exclusion and inclusion errors cannot be analyzed in absence of program size, and results vary depending on the benchmark set.
2. Even in the binary world of measuring method performance, there are measures preferable to simple errors of inclusion and exclusion.
Combining three indicators—coverage and inclusion, and exclusion errors—helps to normalize the errors accounting for the program size and measure performance according to Ravallion (2007) and Wiesmann et al. (2009). Ravallion (2007) shows that the targeting differential (TD) is a metric that is easy to interpret and brings the coverage of the intended population into the equation. When only the intended group gets help from the social program and all of them are covered, the TD equals 1, which is the measure’s upper bound; when only the unintended group gets the program and all of them do, the TD equals −1, the lower bound. Hence, the TD ranges between −1 (low performance) and 1 (high performance) (table 7.4). Detailed formulae are provided in annex 7A.
As the TD is estimated as the difference between the coverage of the eligible population (100 − exclusion error) and the inclusion error, it can be estimated for the four scenarios and for both the real poverty line and the poorest 20 percent benchmark. Scenario 1, which reaches the poorest two individuals, would have a TD of 50 percent for the full poverty line and 100 percent for the poorest 20 percent, implying perfect inclusion for the