Decoding Research Data
Analysis: A Pivotal Tool For Business Decision-Making
Research Data Analysis: the term might seem complex, but its essence is integral to business success. Essentially, it involves inspecting, cleaning, altering, and modeling data to uncover useful insights, draw conclusions, and support decision-making For businesses, it provides a strategic lens to view operations, trends, patterns, and potential market opportunities. Let's delve deeper to understand its significance and the role it plays in business decision-making
● Understanding Research Data Analysis
○ Data analysis is the science of extracting meaningful insights from raw data.
○ It involves various statistical, computational, and analytical methods applied to data sets to reveal patterns, anomalies, and relationships.
○ The ultimate aim is to derive actionable insights that can inform business decisions and strategies.
● Importance Of Research Data Analysis In Business
○ In today's data-driven world, businesses generate and have access to vast amounts of data.
○ However, this data is only as valuable as the valuable insights that can be extracted from it.
○ Research data analysis helps businesses, to -
■ Understand Customer Behavior
● Through analysis, businesses can understand their customer's preferences, buying habits, and needs, enabling them to tailor their offerings accordingly.
■ Improve Operational Efficiency
● Data analysis can identify bottlenecks in business operations and suggest improvements, leading to cost savings and increased productivity
■ Drive Innovation
● By analyzing market trends and consumer needs, businesses can uncover opportunities for new products or services
■ Manage Risk
● Data analysis can help identify potential risks and develop strategies to mitigate them
■ Make Informed Decisions
● Data-backed decisions tend to be more accurate and effective, leading to better business outcomes.
● Role Of Data Analysis In Business Decision Making
○ In business decision-making, data analysis acts as a compass, guiding businesses through the vast sea of information toward their strategic objectives.
○ It helps in -
■ Setting Goals
Data analysis provides evidence-based insights to set realistic and measurable goals
■ Formulating Strategies
By revealing trends, patterns, and relationships, data analysis helps businesses devise effective strategies
Data analysis helps monitor key performance indicators (KPIs), providing a clear view of whether the business is on the right path to meeting its goals.
■ Risk Management
Through predictive analysis, businesses can anticipate potential issues and devise strategies to manage risks.
■ Resource Allocation
Data analysis can help determine the most efficient way to distribute resources, optimizing productivity and profitability.
● The role of research data analysis services in business decision-making is pivotal.
● It turns raw data into valuable insights, guiding strategic decisions and propelling business growth.
● In the modern business landscape, where data is abundant but actionable insights are scarce, mastering data analysis can provide a significant competitive edge
● It isn't solely about collecting data; it's also about making the data work for you
● By effectively analyzing data, businesses can navigate the complexities of their operations and the market landscape, steering their way toward success
Navigating The Diverse Landscape Of Research Data Analysis Types - A Guide For Businesses
● In the realm of business decision-making, research data analysis stands as a compass, guiding businesses through the myriad complexities of data toward valuable insights
● However, to harness its full potential, it is crucial to understand that not all data analysis is the same
● Different types of analysis serve different purposes and yield distinct insights.
● Detailed below are the varied types of research data analysis and their respective value to businesses.
● Descriptive Analysis - The What
○ Descriptive analysis is the simplest form of data analysis. It answers the question "What happened?"
○ By summarizing past data, it provides a clear understanding of the events that have occurred
○ Businesses use descriptive analysis to assess past performance and analyze historical trends.
○ Descriptive analysis includes measures like averages, percentages, and frequency counts.
○ Graphical representation of data such as bar graphs, pie charts, and histograms are common tools in descriptive analysis.
For example, businesses like Ph D thesis writing online providers use data analysis services to gain insight into the market.
● Inferential Analysis - The Why
○ The inferential analysis takes a step beyond the 'what' to explore the 'why'
○ It involves drawing conclusions from the data that extend beyond the immediate dataset
○ In other words, inferential analysis enables businesses to make data-backed assumptions and predictions about larger populations based on a smaller sample.
○ The inferential analysis is particularly useful in market research, where it can provide insights into customer behavior, preferences, and attitudes.
○ For instance, businesses can analyze survey data to draw conclusions about their target market's preferences
● Predictive Analysis - The Future
○ Predictive analysis ventures into the realm of prediction, answering the question of "what could happen?".
○ By leveraging statistical algorithms and machine learning techniques, it extrapolates from existing data to forecast future trends or behaviors.
○ Predictive analysis can help businesses anticipate future sales, customer churn, market trends, and more.
○ This forward-looking approach aids in proactive decision-making and risk mitigation
● Prescriptive Analysis - The Action
○ Prescriptive analysis is the pinnacle of data analysis, addressing the question of "what should we do?".
○ It not only forecasts future outcomes but also suggests actions to benefit from these predictions.
○ Prescriptive analysis is used in various business contexts, such as determining optimal pricing strategies, improving supply chain efficiency, and enhancing customer experience
○ This type of analysis is often powered by complex algorithms, simulation, and machine learning
● Research data analysis is not a one-size-fits-all solution; instead, it encompasses a diverse array of methods, each suited to different business objectives
● From understanding the past with descriptive analysis to shaping the future with prescriptive analysis, each type offers unique value
● In the dynamic business landscape, understanding these different types of research data analysis can empower businesses to extract maximum value from their data.
● By tailoring the type of analysis to their specific needs and objectives, businesses can turn raw data into a potent strategic tool, fueling informed decisions, optimizing operations, and driving growth
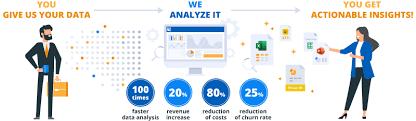
Unfolding The Steps Of Research Data Analysis
For Businesses
- A Comprehensive Guide
Research data analysis plays a significant role in any business operation. It provides a clearer understanding of the business landscape, reveals patterns and trends, and informs strategic decision-making. However, deriving meaningful insights from data is not an instantaneous process; it's a journey that comprises several critical steps Detailed below is an in-depth exploration of these steps, from data collection to interpretation.
● Step 1
Research Data AnalysisData Collection
○ The journey of data analysis begins with data collection
○ It involves gathering relevant information that can answer business questions or support decision-making.
○ Data can be gathered from numerous sources, such as transaction records, customer surveys, social media, or external research reports.
● Step 2
Data Cleaning
○ Once data is gathered, the subsequent step is data cleaning, also known as data preprocessing
○ This step aims to identify and rectify errors and inconsistencies in the data, such as duplicate entries, missing values, or incorrect formatting
○ Clean data is crucial for ensuring the accuracy and reliability of the subsequent analysis
● Step 3
Data Transformation
○ After cleaning, the data is transformed into a suitable format for analysis.
○ This process can include activities like normalization (scaling data to a standard range), discretization (converting continuous variables into discrete ones), or encoding (converting categorical data into numerical format)
● Step 4
Data Analysis
○ This is the core stage where the actual analysis occurs
○ Depending on the business objectives, different analytical techniques may be employed, including -
■ descriptive analysis (understanding what happened),
■ inferential analysis (exploring why something happened),
■ predictive analysis (forecasting future outcomes), and
■ prescriptive analysis (suggesting optimal actions)
● Step 5
Data Interpretation
○ Data interpretation involves making sense of the results of the analysis.
○ This stage is about translating the technical findings into meaningful information that can advise business decisions.
○ For example, an increase in sales might be interpreted as a positive response to a recent marketing campaign.
● Step 6
Data Visualization
○ Data visualization aids in understanding complex data by representing it in a graphical form
○ Charts, graphs, and infographics make it easier to spot trends, compare variables, and comprehend the story that the data is telling.
Reporting & Decision-Making
○ The final step is to communicate the findings and insights to the relevant stakeholders through reports or presentations.
○ The insights derived from data analysis should inform decision-making, guiding the business toward its strategic objectives.
● Research data analysis is a systematic process that demands meticulous execution at each step.
● It involves transforming raw data into actionable insights through a journey of collection, cleaning, transformation, analysis, interpretation, visualization, and reporting
● Businesses that understand and adeptly navigate this process stand to gain a substantial competitive edge by unlocking the potential of data to inform strategies, optimize operations, and drive growth.
● It's not just about having data; it's about understanding it, and that's where the magic of data analysis lies
Harnessing The Power Of Research Data Analysis In Business Operations
In today's dynamic business environment, the difference between success and failure often hinges on the ability to make data-driven decisions. More than ever, businesses need to understand their markets, customers, and internal processes, and research data analysis provides the necessary tools for such understanding This article explores how businesses can utilize data analysis in various aspects of their operations, including marketing, sales, supply chain, and human resources.
● Data Analysis In Marketing
○ Effective marketing begins with understanding the target audience, and data analysis offers invaluable insights into customer behavior, preferences, and trends.
○ By analyzing customer data, businesses can identify successful marketing strategies, segment their audience for targeted campaigns, and predict future marketing trends
○ Moreover, through sentiment analysis, businesses can gauge customer reactions to marketing campaigns and adjust their strategies accordingly
● Data Analysis In Sales
○ Sales data analysis can be a gold mine of insights for a business
○ By scrutinizing sales data, businesses can identify best-selling products, peak sales periods, and the effectiveness of sales strategies
○ Furthermore, predictive analysis can forecast future sales trends, enabling proactive planning and inventory management.
● Data Analysis In Supply Chain Management
○ Supply chains generate a wealth of data, from procurement and production to distribution and customer service
○ Analyzing this data can help businesses optimize their supply chain operations, diminishing costs, and elevating efficiency
○ For instance, data analysis can help identify bottlenecks in the supply chain, predict demand and supply trends, and streamline logistics and inventory management.
● Data Analysis In Human Resources
○ Data analysis is increasingly finding application in human resources (HR) management
○ HR analytics involves analyzing employee data to inform HR policies and strategies
○ For instance, data analysis can provide insights into employee performance, retention, and satisfaction
○ These insights can guide recruitment strategies, employee development programs, and workplace policies, contributing to a more productive and satisfied workforce.
● In summary, research data analysis is a potent tool for businesses, providing insights that inform decision-making across various operational domains.
● Whether it's understanding customer preferences, forecasting sales, optimizing supply chains, or enhancing human resource management, data analysis is key to unlocking operational efficiency and strategic advantage
● In the competitive world of business, data-backed decisions are the currency of success.
● By effectively leveraging research data analysis, businesses can ensure they are making decisions based on facts, not just hunches, positioning them for sustained success in their respective markets
Tackling Challenges In Research Data Analysis - A Strategic Guide For Businesses
Research data analysis has emerged as a cornerstone of successful business decision-making By providing deep insights into market trends, customer behavior, and operational efficiency, research data analysis can give a competitive advantage to companies like United Innovator However, like any powerful tool, its implementation is not without challenges This article sheds light on some of these common hurdles and offers practical solutions for businesses to overcome them
● Data Privacy Concerns
○ With the surge in data collection comes increased concern over privacy
○ Businesses must ensure they are compliant with privacy laws such as GDPR and CCPA when handling personal data.
○ In order to overcome this challenge, businesses can adopt robust data governance practices, anonymize personal data, and ensure transparency about data usage with their customers
● Ensuring Data Quality
○ Poor quality data can lead to inaccurate insights and misguided decisions
○ It's imperative for businesses to ensure the data they analyze is accurate, complete, and up-to-date
○ Regular data cleaning and validation can help maintain data quality
○ Moreover, deploying data quality management tools can automate the process, reducing errors and improving accuracy
● Lack Of Skilled Personnel
○ Data analysis requires specialized skills and knowledge, which many businesses may lack.
○ This shortage can be addressed by investing in training existing employees or hiring skilled data analysts.
○ Collaborating with external data analytics consultants is another viable option, particularly for small businesses
● Managing Large Volumes of Data
○ With the rise of big data, managing the sheer volume of information can be overwhelming
○ However, advancements in data storage solutions, such as cloud computing and data warehousing, can help manage and organize large data sets
○ In addition, tools like Hadoop and Spark can process big data efficiently, making it easier to analyze
● Integrating Data From Various Sources
○ Businesses often collect data from numerous sources, leading to disparate data types and formats
○ Integrating this data for analysis can be challenging.
○ However, data integration tools can help combine data from different sources into a unified view.
○ Implementing a data warehouse can also facilitate the integration process.
● Making Data Analysis Actionable
○ Translating data insights into actionable decisions is a key challenge.
○ It's not enough to collect and analyze data; businesses need to interpret and apply these insights effectively.
○ Creating a culture of data literacy within the organization can help bridge this gap.
○ Also, visualizing data using tools like Tableau or Power BI can make insights more understandable and actionable
● While challenges in research data analysis are undeniable, they are not insurmountable
● With the right strategies, businesses can navigate these obstacles and harness the power of data analysis.
● By addressing data privacy concerns, ensuring data quality, developing skilled personnel, managing large data volumes, integrating various data sources, and making data actionable, businesses can unlock the full potential of data analysis.
● In doing so, they transform these challenges into opportunities, driving their growth and competitive edge in the data-driven business landscape.
Pioneering The Future With Advanced Research Data Analysis Techniques
● Research data analysis is at the forefront of innovation in the business world Companies offering Ph.D. research proposals are using this actively nowadays.
● From understanding customer behaviors to predicting future trends, data analysis has become a crucial tool for business success.
● The evolution of this field is continuously unveiling new techniques and methodologies that offer deeper insights and more precise predictions.
● Explored below are some of the advanced techniques in research data analysis that are shaping the future of businesses
● Machine Learning
○ Machine learning is a subdiscipline of artificial intelligence that makes it possible for systems to learn and improve from experience without explicit programming
○ It is particularly effective in analyzing large and complex datasets.
○ Machine learning algorithms can help businesses predict customer behavior, identify patterns and anomalies, automate decision-making processes, and much more
● Natural Language Processing (NLP)
○ NLP is another AI technique that allows computers to understand, interpret, and generate human language.
○ For businesses, NLP can be used to analyze text data like customer reviews, social media comments, or call center transcripts, providing valuable insights into customer sentiment and preferences
● Deep Learning
○ Deep learning is another aspect of machine learning that apes the functioning of the human brain to process data
○ It's exceptionally useful for tasks like image and speech recognition and can also be employed for complex prediction tasks
○ For instance, deep learning can be used in predictive maintenance, where it can predict equipment failures by analyzing various sensor data
● Network Analysis
○ Network analysis involves studying the relationships between entities
○ In a business context, it can be used to understand relationships between different products, customers, or various business units.
○ By analyzing these networks, businesses can identify influential entities, understand community structures, and optimize communication or distribution paths
● Time Series Analysis
○ Time series analysis involves analyzing data that is collected over time to identify patterns or trends.
○ This method is commonly used for forecasting purposes.
○ For instance, businesses can use time series analysis to forecast sales, stock prices, or product demand.
● Sentiment Analysis
○ Sentiment analysis, also called opinion mining, is a technique used to determine the sentiments expressed in a piece of text
○ Businesses can use sentiment analysis to understand customer opinions and feelings towards products, services, or brands, often using data from social media or customer reviews.
● The advanced techniques of research data analysis open new doors for businesses to understand their environment, optimize their operations, and innovate their strategies
● From machine learning to sentiment analysis, these techniques offer a deeper, more nuanced understanding of data, enabling businesses to make better-informed, data-driven decisions.
● In the evolving world of business, the importance of staying on the cutting edge of data analysis techniques cannot be overstated.
● Businesses that adapt and adopt these advanced methodologies will not only obtain a competitive edge but also pioneer the future of their respective industries