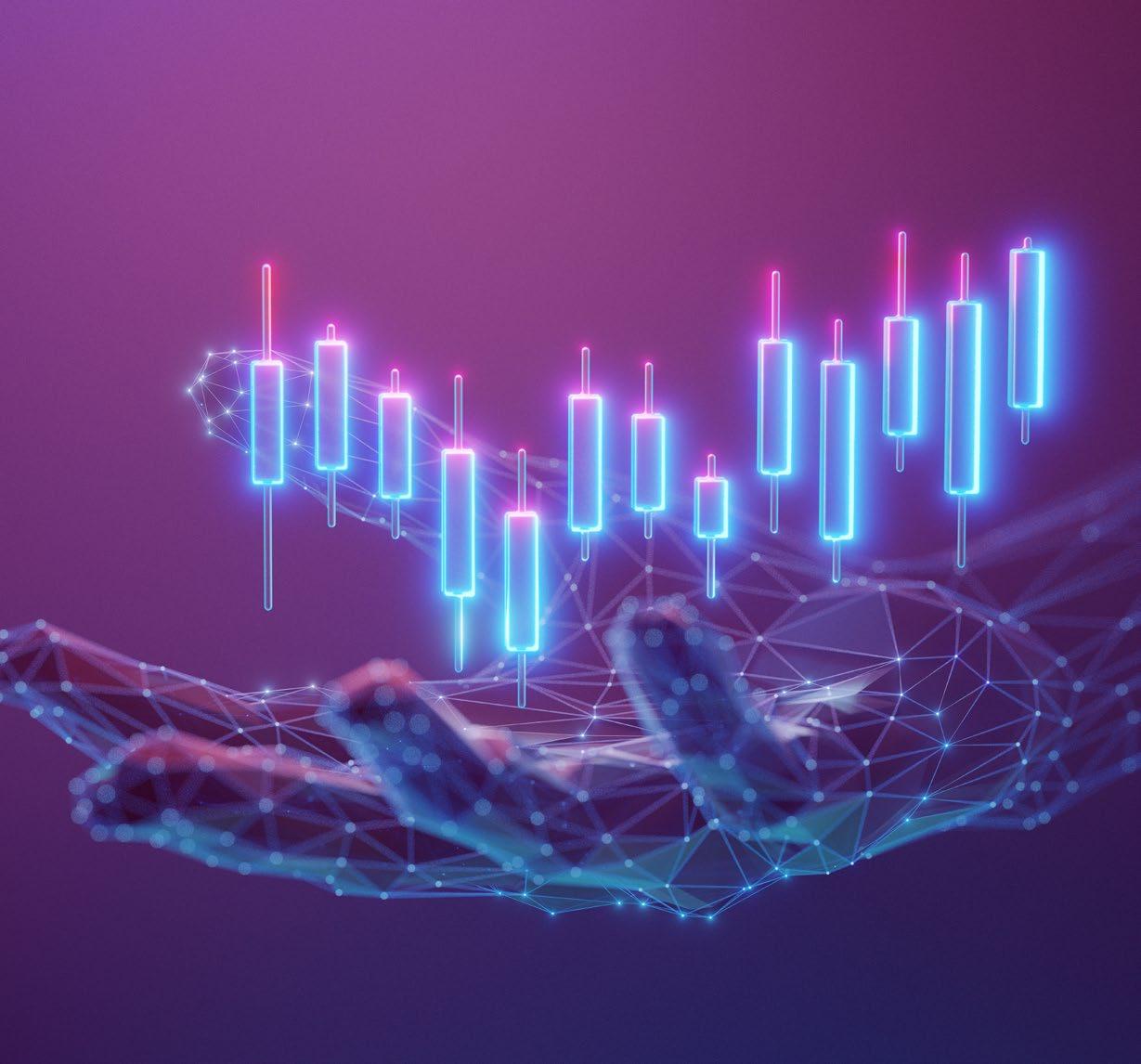
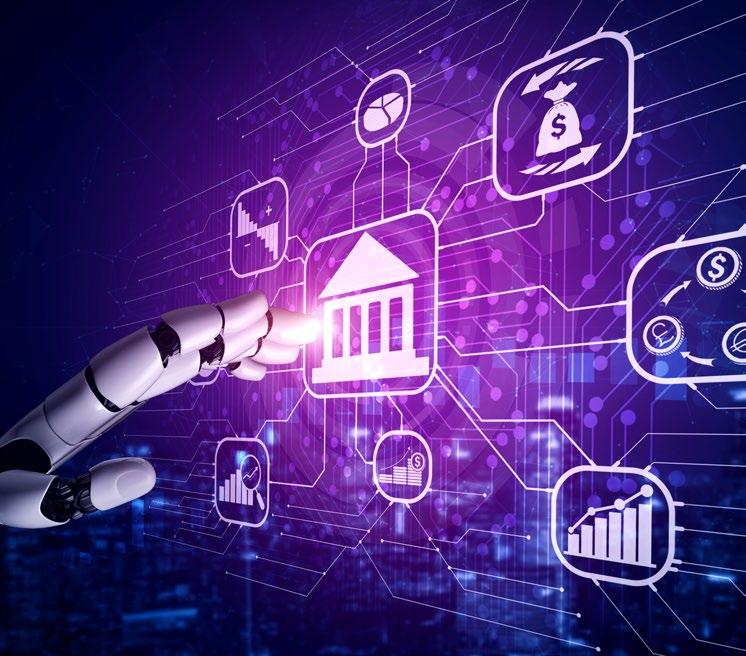
Devin Shanthikumar, associate dean of undergraduate programs at the UC Irvine Paul Merage School of Business, is at the forefront of AI-driven financial research. Her work focuses on two key areas: using AI to track extreme sentiment on social media, and examining how that sentiment affects financial markets. She is also researching how AI can help improve the accuracy of financial analyst forecasts. Together, these projects highlight the powerful impact AI is having—and will continue to have—on financial research and decision-making.
Shanthikumar’s groundbreaking working paper, “Sentiment Extremeness on Social Media: Evidence from Seeking Alpha Comments,” is coauthored with Qiao Annie Wang, Montana State University, and Shijia Wu, The Chinese University of Hong Kong, Shenzhen, both PhD graduates from the Merage School. This research examines how financial social media platforms like Seeking Alpha, a widely used financial platform where investors share their views on stocks
and market trends, affect user sentiment and extremism.
“Social media can drive us to become more extreme, but it could also have the opposite effect— helping us learn from each other and meet closer in the middle,” Shanthikumar says. Her research aims to determine how these phenomena play out in financial contexts. She and her team analyzed vast comment datasets from Seeking Alpha, using AI to measure whether users’ sentiments were positive or negative and how extreme those sentiments were.
The results were surprising: Participation on Seeking Alpha tended to moderate users’ opinions over time, making them less extreme rather than more. This moderation effect challenges the common assumption that social media inherently amplifies extremism. “When someone comments early on—then they comment again and again—they become more and
more moderate as they discuss the stock,” says Shanthikumar. This effect is particularly strong when users aren’t anonymous, suggesting platform design and transparency play a crucial role in shaping user behavior.
Their research implications are broad. Platforms like Seeking Alpha, which expose users to a variety of perspectives, can reduce echo chambers and encourage more balanced discussions. This, in turn, leads to a decrease in volatility, as more moderate sentiment tends to result in less erratic trading behavior. “When you have more extreme sentiment, you get more trading volume, more volatility. But as extremeness decreases, that excess trading volume goes away,” Shanthikumar says.
She stresses how integral the AI language tool was to their research. “One of the key takeaways from our paper is that you can use large language models very well
to measure the type of free-form, regular conversational text that most people use. For example, the AI seemed to catch when people were being sarcastic or using words in a nontraditional way. It was very good at capturing true sentiment.” As she ponders ways to teach AI use to her students, this is an important takeaway, she says.
Shanthikumar also explores how AI affects the productivity and accuracy of financial analysts’ forecasts. A new working paper, “Artificial Intelligence and Analyst Productivity,” coauthored with Il Sun Yoo, Shidler College of Business, University of Hawai‘i at Mānoa, another Merage School PhD graduate, addresses three key questions:
1. Does AI improve the quantity and quality of forecasts?
2. Does it complement or replace human analysts?
3. How does AI investment affect employment in the financial sector?
Their research reveals that AI
significantly increases the quantity of forecasts financial analysts produce. More importantly, the quality of these forecasts also improves, especially for more volatile stocks that are harder to predict. “The AI allows the analysts to do what they do best and to do it better,” she says. “It’s basically complementing the analyst’s skills.”
This is a crucial finding because it demonstrates AI can enhance human performance in complex tasks rather than simply automating them. However, AI’s effect on employment is more nuanced. While analysts’ productivity increases, according to the research, investment banks that employ AI decreased hiring of new analysts.
One of the other interesting aspects of the research is how different types of AI are used. Shanthikumar distinguishes between broad AI systems that analyze complex financial data and more accessible tools like ChatGPT. While ChatGPT can increase the quantity of work, it does not improve the quality of forecasts as broadly. “ChatGPT might help us write emails and reports, but it will not necessarily help with complex numerical
analysis for forecasting earnings,” she says. This highlights the need for specialized AI systems tailored to specific financial tasks.
Shanthikumar’s AI research offers valuable insights for investors and the financial industry. On social media platforms like Seeking Alpha, AI reveals that certain key factors influence the moderation of sentiment to create a more balanced and informed investing environment. Investors should be aware that platforms designed to expose users to diverse opinions, rather than reinforce existing beliefs, can provide a more accurate picture of market sentiment and reduce the risk of making decisions based on extreme or emotionally charged views. In terms of AI, the research shows that AI can be used to analyze investor sentiment in a systematic way.
“They didn’t lay anyone off, but they reduced subsequent hiring,” Shanthikumar says. “It’s important to note that this is the shortterm effect on employment. The existing analysts are doing more, and they’re doing it better, so the firm doesn’t need more analysts. But it remains to be seen if firms then figure out new and better ways to use analysts and subsequently return to hiring more.”
With regard to financial analysis, Shanthikumar’s work shows AI is not only a tool for automation but a powerful complement to human expertise. Investors can take comfort in the fact that AI-backed forecasts are likely to be of higher quality, especially for uncertain or volatile firms. This suggests banks and financial institutions with significant AI investments may offer more reliable forecasts, which is something investors should pay attention to.
For financial institutions, Shanthikumar’s findings underscore the importance of thoughtful AI integration. While AI can improve productivity and forecast quality, firms need to be mindful of its effect on employment and ensure it supplements, rather than replaces, human analysts. “Our research shows that AI is currently most useful to complement what humans do best, not to replace us. Let’s not try to automate knowledge-based jobs,” she says. “Instead, let’s think about how we can take advantage of AI to let people do what they do well.”
As AI continues to evolve, its role in both financial analysis and investor behavior will grow. Shanthikumar’s research is the beginning of what promises to be a transformative period for the financial industry. In the future, she plans to explore how
AI affects other aspects of analysts’ work, such as their written reports and participation in conference calls. Moreover, the socioeconomic implications of AI adoption in finance cannot be ignored. While AI-driven productivity gains are beneficial, the potential for reduced hiring in knowledge-based industries raises important questions about AI’s effect on the future of white-collar work in finance and beyond. “We’re seeing a little bit less new hiring, even in highly educated fields. That does raise questions going forward,” she says.
Shanthikumar’s dual approach to AI in financial research exploring both its effect on analyst productivity and its role in assessing user sentiment online—offers a comprehensive view of how AI is reshaping the financial landscape.
Her findings suggest that while AI can enhance human performance, careful consideration must be given to its broader implications, from employment to market behavior.
As AI technology continues to advance, its integration into financial markets will require a balanced approach, one that leverages its strengths while safeguarding the human element that remains critical to interpreting and navigating the complexities of financial forecasting. //
Devin Shanthikumar is the Associate Dean for Undergraduate Programs at Merage and an Associate Professor in the Accounting group. She teaches undergraduate, MBA, executive-level and PhD courses, covering topics such as Managerial Accounting, Driving Profitability, and Research Presentations. Prior to joining the Merage School, she served on the faculty at the Harvard Business School (HBS), where she taught both Financial Accounting and Managerial Accounting for MBAs, and wrote cases which are used in accounting courses across the country.
Founded in 1975 by John Bogle, The Vanguard Group pioneered passive investing, an innovative way to reduce the cost of the investment process and simplify it for everyday Americans. Since then, passive investing, led by index funds and exchange-traded funds, has exploded. According to Statista, passively managed index funds accounted for 19% of the total assets investment companies managed in the United States in 2010. As of 2023, that number had skyrocketed to 48%.
such as their written reports and participation in conference calls.
Moreover, the socioeconomic implications of AI adoption in finance
advance, its integration into financial markets will require a balanced approach, one that leverages its strengths while safeguarding the
But even Bogle began to wonder about the unintended consequences of too much passive investing. Shortly before he passed away in 2019, he predicted it wouldn’t be long until index funds would own half of all U.S. stock, which he didn’t consider a good thing: “I do not believe that such concentration would serve the national interest.”
What happens, then, if passive investing becomes too successful for its own good? This is the question Lu Zheng, professor of finance at UCI’s Paul Merage School of Business, and her coresearchers, Hao Jiang of Michigan State University and Dimitri Vayanos of the London School of Economics, set out to answer in their awardwinning paper: “Passive Investing and the Rise of Mega-Firms.”
“When I started my doctoral research years ago, the mutual fund sector was just starting to flourish,” Zheng says. “Empirical evidence
shows that, on average, index funds performed better than actively managed funds after fees. But now that we’ve seen dramatic growth in passive investing, people are starting to wonder about its longterm market impact.” she believes.
Based on her research, one of the primary effects of passive investing is the largest firms in the S&P 500 experience the highest returns and increases in volatility following flows into that index. In this way, passive investing biases the stock market toward overvaluation.
“Basically, what we see happening is large firms keep getting bigger, and their stock prices keep going higher,” Zheng says. “One day there might be a reversal of this trend, but for now, our study shows a rise of megafirms in large concentrations.”.
The reason for this has much to do with the strategies behind passive investing. A passive investing strategy usually tracks a valueweighted index, which involves putting more money into large cap stocks. As a result, the economy’s largest firms receive more flow, thereby increasing in price. If a large firm was overvalued, the flow from passive funds would exacerbate overvaluation.The now overpriced stocks are riskier for active investors who would otherwise offset the flow’s effect on price.
In other words, these flows into passive funds disproportionately increase the stock prices of the market’s largest firms, and especially those large firms that the market overvalues. The effect on a grand scale is a rise in the aggregate
market, which happens even when a flow is entirely the result of investors switching from active to passive strategies.
This finding was somewhat surprising to Zheng and her coresearchers. They anticipated new money flowing into the mega-firms would lead to overvaluation, but they did not anticipate switching also causes overvaluation.
“The reason for this has to do with proportions,” says Zheng. “As people switch to passive investing, there are fewer active investors in play. The additional demand for the mega-firm’s stock then leaves these few active players more exposed to risk, so the stock price must rise to convince them to accept that risk. We end up with a loop of rising prices.”
Part of what makes this research valuable enough to win awards is its combination of theory and empirical evidence. Using a mathematical model, the team was able to manipulate different variables. This allowed them to see cause and effect more clearly.
“In the real world, it’s hard to disentangle all the effects of passive investing, but the modeling we did here gave us good calibration,” Zheng says. “It helped us see each individual element more clearly. Then, when the empirical data corroborated the model’s results, we saw that as confirmation.”
Without this extensive modeling, the complexity of the empirical
data would limit the research. It wouldn’t be possible to unpack the theoretical motivation—the “why ”—behind the market phenomenon in question. Being able to capture the “what” and the “why” is what Zheng believes is the most valuable contribution of this research.
“We’ve documented some of the consequences of indexing that have yet to be documented up to this point,” says Zheng. “This information is important for market participants and regulators to know. To make informed choices, they need to understand how the market adjusts itself and where it could head from here.”
How big can indexing get? And what are other unintended
consequences of it? These are the questions Zheng wants to answer next. In fact, she and Jiang have recently collaborated with another group of coresearchers to explore how passive investing affects diversification. According to their study, the rise of indexing corresponds with an increase in market-level volatility, which limits the power of diversification and the advantages of passive investing itself.
“Diversification has been a strategy investors use to diversify away firmspecific risk and reduce portfolio risk, but index funds tend to buy and sell together, which increases the co-movement among stocks,” Zheng says. “The more stocks comove, the less benefit we get from diversification, so indexing can lead to more co-movement—and thus increased market risk.”
“For example, when Tesla entered the S&P 500, it was a significant event, but people knew about it beforehand.,” Zheng says. “Index funds had to buy Tesla, and the market participants were aware of this–some would front–run and drive up the price. The question is how index funds can time their purchases to serve the best interests of their investors while minimizing the impact of these inflated prices, despite their lack of discretion in trading strategies.” //
From here, Zheng wishes to investigate other consequences of passive investing, particularly within the realm of mutual funds. She also wants to look into how passive investing affects the information efficiency of the market during crises—that is, how the market absorbs shocking news when its investments are mostly passive.
Lu Zheng is a professor of finance at UCI’s Paul Merage School of Business. She obtained her PhD in finance from Yale University and received her bachelor’s degree from Agnes Scott College. Prior to joining UC Irvine, she served on the faculty of the University of Michigan. Zheng’s research covers many aspects of investments, including mutual funds, hedge funds, equity markets, investor behavior and expectations, and institutional trading.
Nearly everyone wants to talk about the importance of human capital these days, but so far, publicly traded companies in the United States are not legally required to disclose human capital-related details. In 2020 the Securities and Exchange Commission (SEC) mandated that companies disclose certain human capital-related information. The requirement is only principle-based, leaving companies with ample discretion about what and how much they disclose to the public. Of the various types of information about human capital, the one that is of particular interest to both regulators and public investors is employee diversity information.
In their article, “Does the Risk Aversion of Accountants Matter?
Female Rank-and-File Accounting Employees and Internal Control Quality,” published in the Journal of Accounting and Public Policy, Associate Professor Ben Lourie and Assistant Professor Chuchu Liang of the UCI Paul Merage School of Business look into the question of human capital from the employee diversity side, specifically to use gender to proxy for risk preferences of employees and examine if employee risk aversion affects the company’s information environment, with a focus on internal quality control.
Moreover, Turner sees potential applications in other fields, albeit with some caveats. There are some general principles, he says, but he doesn’t want to go too far to suggest others do exactly what they’ve done. “A lot of work goes into finding the right tweaks that work in a specific environment.”
“People have talked about the importance of the rank-and-file employees,” says Liang, “but we have limited research demonstrating the effect of employees on company outcomes, mostly due to the lack of data.”
The study uses third-party data to show the characteristics of accounting employees, particularly
their risk aversion, which is proxied by the employee’s gender and whether it affects the internal quality control of the firm.
In response to the recent SEC suggestion that firms consider reporting more details about their human capital, Lourie and Liang wanted to provide usable data to policymakers who evaluate the relevance of the information for investors.
Prior studies suggest a firm’s upper management mostly influences accounting performance and internal quality controls. However, Lourie and Liang wanted to know if that
“We know the investors demand such information, but we also know it’s costly to directly mandate that firms disclose these details,” says Liang. “We understand how important it is to collect more evidence to show the potential usefulness versus the cost of disclosure.”
was really the case. “Prior literature in this field tended to look at the entire workforce as a homogeneous unit, instead of looking at the differences across different functions within the workforce,” says Liang. “Our study uses the data at the function level. Since we have the data for accounting employees versus non-accounting employees, we can show how the individual accounting employee matters for the company’s overall risk aversion and for accounting-specific outcomes like internal controls, which are directly linked to the risk aversion of the accounting employees. This allows us to demonstrate that accounting employees, in particular, play a key role in shaping a company’s risk profile and internal control outcomes.”
Lourie and Liang use third-party data to obtain diversity information from employee profiles, as well as online profiles for 3,052 unique firms dating from 2008 to 2018. “We’re using a large data set from a vendor called Revelio Labs,” says Liang. “They extract raw data from employee profiles on LinkedIn and many other
online platforms. Then they use a proprietary algorithm to structure data to make it more comparable across firms.”
After examining the data, Lourie and Liang found that the percentage of female accounting employees is directly associated with the quality of a firm’s internal controls. “When the company has a higher percentage of female accountants, they’re less likely to have internal control weaknesses,” Liang says. “In other words, they’re more likely to have a higher quality of internal control. This
is our baseline result. Additionally, we show that the association holds in the next year. Thus, if we have the information about a company’s female accounting number, we might predict the next year’s internal control quality.”
Public disclosure of human capital information regarding the percentage of male and female employees is an indicator of the firm’s internal control quality. “If all public companies make the data available, investors can understand and even predict the company’s accounting quality,” says Liang. Liang and Lourie are both quick to point out that their findings do
Ben Lourie serves as an Associate Professor of Accounting at the UCI Paul Merage School of Business. His research centers around equity analysts, human capital and household finance. Lourie’s scholarly contributions have been recognized in esteemed academic journals, including The Accounting Review, Journal of Financial Economics, Management Science, Review of Accounting Studies, Contemporary Accounting Research, and Review of Finance.
Chuchu Liang’s current research interests primarily include accounting issues related to intangibles, corporate disclosures, information acquisition and transfers, and financial reporting choices. She is also interested in exploring big data and applying textual analysis in studying the decision making of market participants such as managers, investors and auditors.
not suggest it’s always better to have more women than men on the accounting team. “We’re not trying to say it’s better to have more women or men,” says Lourie.
“We actually found no difference in operating performance at that level, but we do find there are more internal controls and weaknesses when you have more men. It doesn’t necessarily mean it’s good or bad. Sometimes maybe you want to take more risks.”
As the SEC and other policymakers try to decide whether to mandate firms to disclose employee information at this level or not, this study certainly demonstrates the potential usefulness of this data, especially as a way for investors to better understand a company’s accounting environment and quality.
“Overall, we show the usefulness of this gender diversity employee information for investors,” says Liang. “We not only show that employee data at the workforce level are useful but also at the function level because there’s a predictive power for accounting employees versus non-accounting employees. That means if firms are forced to disclose information, it’s better to not only disclose diversity information for the entire workforce but also to disclose by different department levels or functions.” //
It’s no secret that incentives shape individual behaviors, but the challenge is to accurately predict the specific behaviors that may arise from a particular incentive.
Researchers at the UCI Paul Merage School of Business have been exploring how incentives influence financial analysts. What they found raises a few red flags both for investors and for the industry at large.
For Shanthikumar, the goal of the research was to develop a better understanding of how incentives influence the brokerage industry. In the 2000s, she researched conflicts of interest in the investment banking industry during a time of intense regulatory interest in the topic.
The 2003 case of star analyst
“All of this activity is happening inside the brokerage houses, and they don’t want to share all of that information,” she says. “We were able to get data that allows us, with a little creative research design, to figure out how to answer this question.”
Henry Blodget was especially influential in bringing the issue of incentives to the forefront. Blodget, then working as a researcher for Merrill Lynch, was charged by the Securities and Exchange Commission with civil securities fraud after he was found to have publicly issue buy recommendations for certain tech stocks while expressing negative views of those same stocks in private emails. The lawsuit led to significant media attention and rule changes that broke the link between analyst research and investment banking.
The resulting separation of research from brokering removed the incentive structure which tied analyst compensation with investment banking outcomes. However, Shanthikumar says, the new rules only addressed one incentive problem. “Now the brokerage trading incentive will be that much more important to study,” she says.
Shanthikumar has been interested in studying how brokerage trading and incentives influence
analyst behaviors for many years. The challenge has always been accessing the right data. When new data became available, the team recognized they had an opportunity to gain new insights into the link between incentives and analyst behaviors.
Shanthikumar and her colleagues looked at data for each brokerage house and measured how much trading volume they executed in a particular stock. The team compared trade volumes at different brokerage houses while also looking at which analysts issued forecasts, and whether the forecasts were optimistic or not.
This new comparative approach was a huge first step, but the team still needed to work out whether the numbers firmly showed causation in the correlations between trading volumes and analyst reporting. To resolve the question of causation, they looked at how analysts changed over time, including when they moved from one firm to another. “Are analysts learning from what happened last year and updating their behaviors the next year? Using that data and using
both correlations and exploiting data related to analyst changes we were able to better understand what was going on,” Shanthikumar says.
Analysts working in-house at brokerages are paid to produce research and reports. While customers benefit from the insights reports provide, regardless of the analyst’s final recommendation, the concern is that the brokerage as a whole stands to benefit from recommendations that could drive trading volumes.
“It’s a little bit like being in customer service,” Shanthikumar says. “Analysts don’t directly bring in revenue to the company. They’re being paid to help the company by performing a service to investors. That’s one of the reasons why analysts’ incentives can be harder to understand.”
Simply put, analysts want to support the trading business of their brokerage house. The intended effect of incentives is to encourage analysts to provide helpful research data to the investors who are trading with the firm so that they make better trades and everyone wins. But is it really that simple?
“We do find evidence for the intended effects of incentives in our research,” Shanthikumar says. “They drive more volume for the brokerage house. So, volume is sensitive to
the quality of the analyst. If they issue better earnings forecasts, or if they’re more timely, those things really do impact the trade volumes.”
In short, higher quality research leads to more trading volume, providing an incentive for quality –the intended effect.
Shanthikumar and her colleagues were especially interested to see if analyst incentives had any unintended consequences. “We did find evidence for a few unintended effects,” Shanthikumar says. “The incentives lead analysts to issue more positive or optimistic forecasts. The main reason for that is that most of us can buy stock, but you can’t sell a stock unless you own it. If you issue a positive forecast, you generate more trades.”
Another unintended consequence was the analyst’s response to the optimism incentive. We found a stronger response to that optimism incentive than the accuracy incentive,” she says. “At least in the short term. Maybe over the course of their careers, that accuracy and quality incentive is going to help
things, but on the one or two-year horizon what we see is that analysts are becoming more optimistic when they see that it works.”
While the research shows that trading volume responds to analyst quality, volume also responds to optimism. “That is the thing we need to address,” she says. “It can drive the analysts to be too optimistic and it can lead us as investors to buy too much. This is a sign that the finance industry needs to reevaluate their incentives. Europe has already started to change practices by requiring direct payment for analyst research. For us as investors, this means we need to take that very positive research with a little grain of salt.”
One surprising insight from the team’s research came from looking at what happened when an analyst moved from one brokerage house to another. “What we found was that the trading volume followed them,” Shanthikumar says. “If a brokerage house got a high-volume analyst from another firm, they could increase their volume on those
stocks that the analyst covers by as much as twenty percent. That’s a huge effect.”
While they found strong evidence that analysts are strategically updating their behavior in response to these incentives and becoming overly optimistic, Shanthikumar wants to be careful not to paint with a broad brush. “I don’t want it to sound like we’re saying that analysts are just lying to us or that we can’t trust them,” she says. “Analysts are simply responding to the incentives they’re given. They’re also compensated for accuracy, so that can provide some balance to the equation as well.”
Shanthikumar has advice for current investors and for students who want to work in the finance industry. “For people who are going into wealth management or investment management, they need to understand how to use information in a sophisticated way,” she says. “Part of what that means is to adjust for the potential biases created by these types of incentives. We don’t want to ignore analysts. They have a lot of great insight and information that we can all learn from. But in light of our research, we need to make the appropriate adjustments.”
Shanthikumar feels that their report has implications for pretty much
everyone. “I teach MBAs and undergraduates to really be careful about incentives,” she says. “The incentives they design for their employees, the incentives their customers have, that their suppliers have, they all impact our behaviors. As parents, we design incentives for our kids to try to drive their behavior. We know how our incentives at work drive our own behaviors. But we need to be thoughtful about the intended and the unintended effects.”
The bottom line is that the financial industry needs to think about the role they want analysts to play. “What’s happening right now is a lot of experimentation,” Shanthikumar says. “In Europe, these kinds of incentives are no longer allowed. In the U.S. we’re seeing the rise of these independent research firms that aren’t brokerage houses. They’re just doing the research. But how do they sell that research? Some are funded by the people using the research, others are
funded by the companies that want coverage. Each of these is going to come with their own set of incentives and problems.”
Shanthikumar is optimistic about the future. “I don’t think we’re going to see legislation to step in and stop this. I don’t think the problem is that bad at this point,” she says. “We just need to be more sophisticated about how we respond to the research. We need to be aware of these conflicts of interest. What I’m hoping is that people might start to be even more sophisticated about other conflicts of interest that we may not have the research on yet.” //
Devin Shanthikumar is the Associate Dean for Undergraduate Programs at Merage and an Associate Professor in the Accounting group. She teaches undergraduate, MBA, executive-level and PhD courses, covering topics such as Managerial Accounting, Driving Profitability, and Research Presentations. Prior to joining the Merage School, she served on the faculty at the Harvard Business School (HBS), where she taught both Financial Accounting and Managerial Accounting for MBAs, and wrote cases which are used in accounting courses across the country.