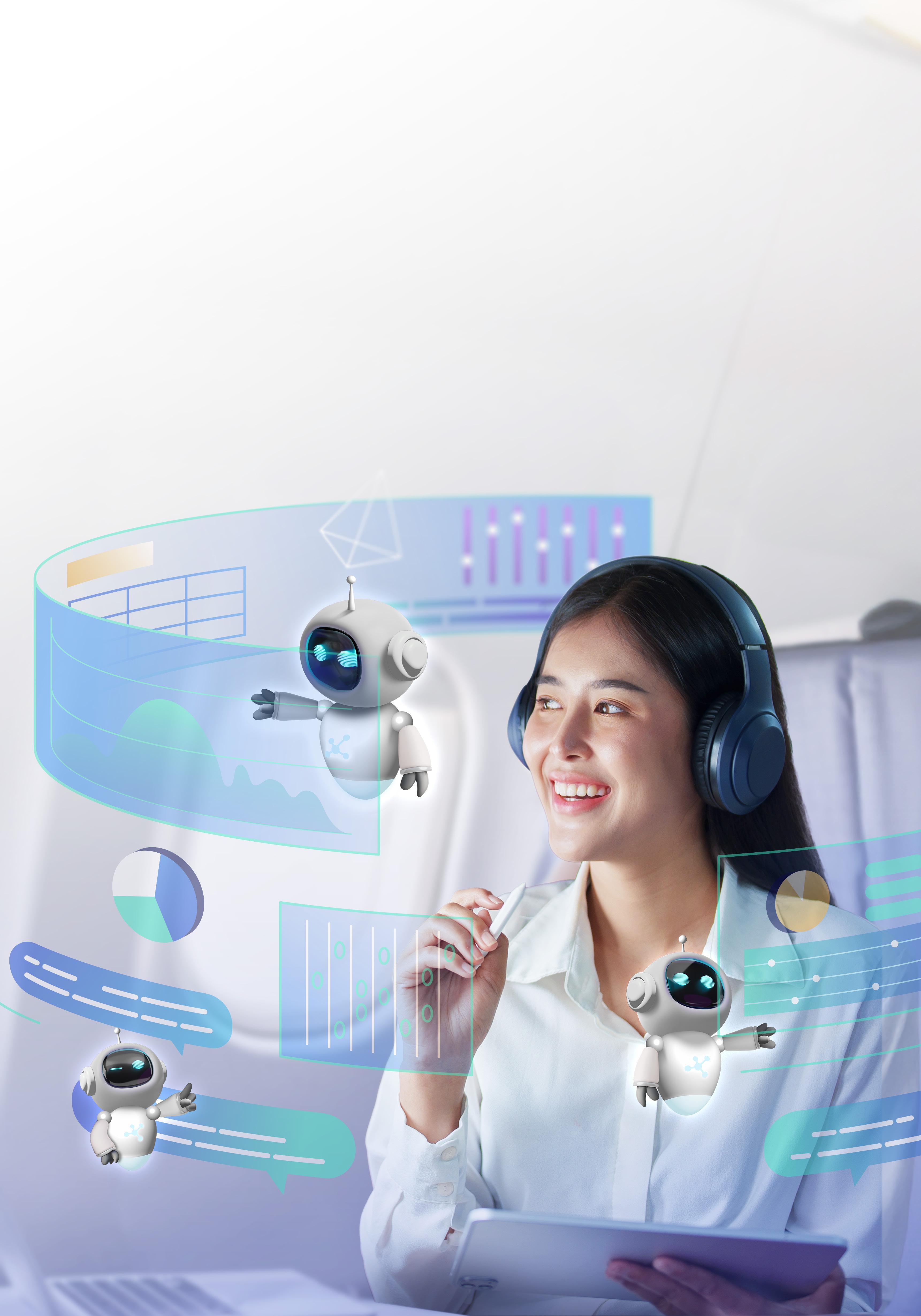
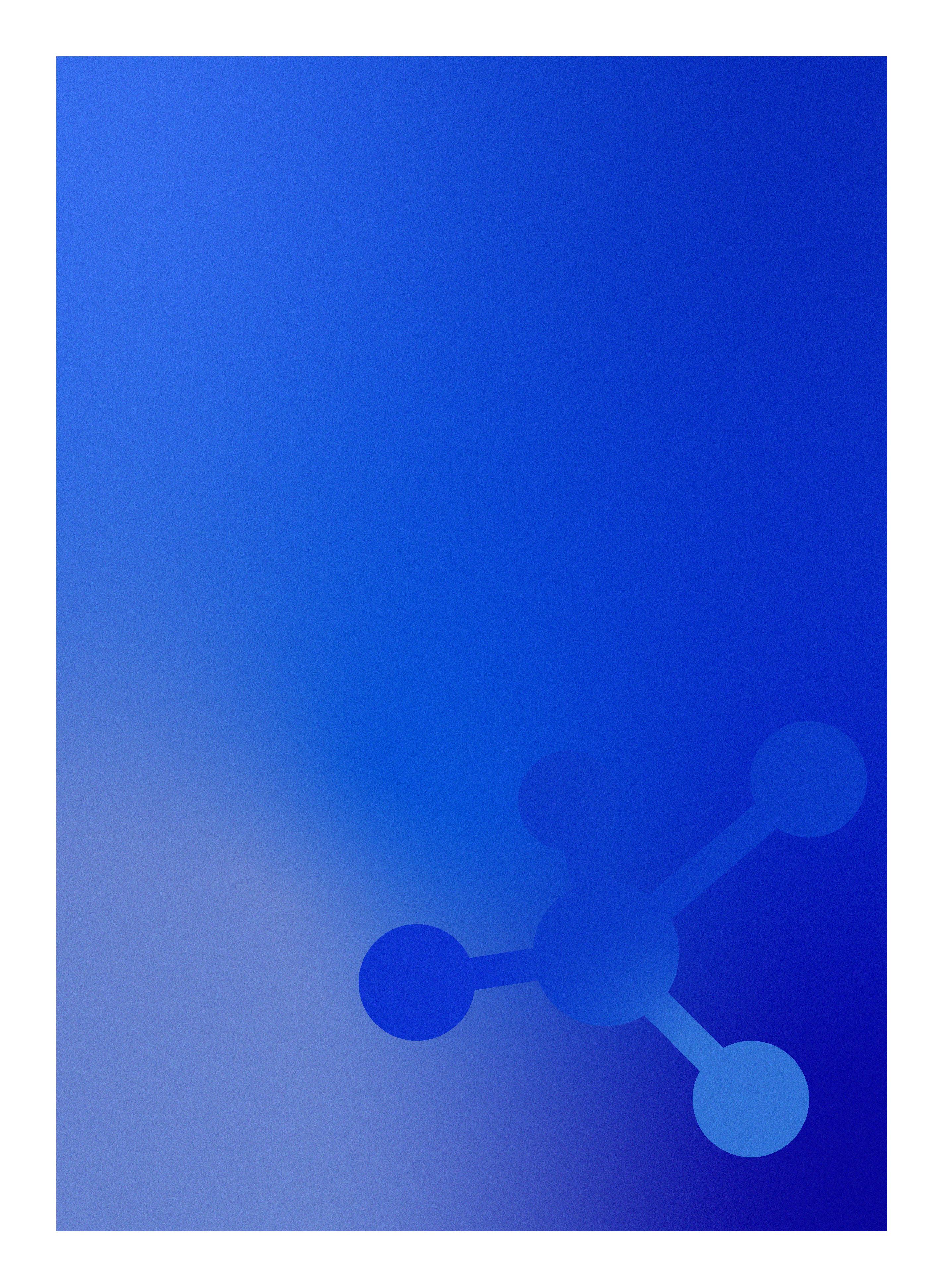
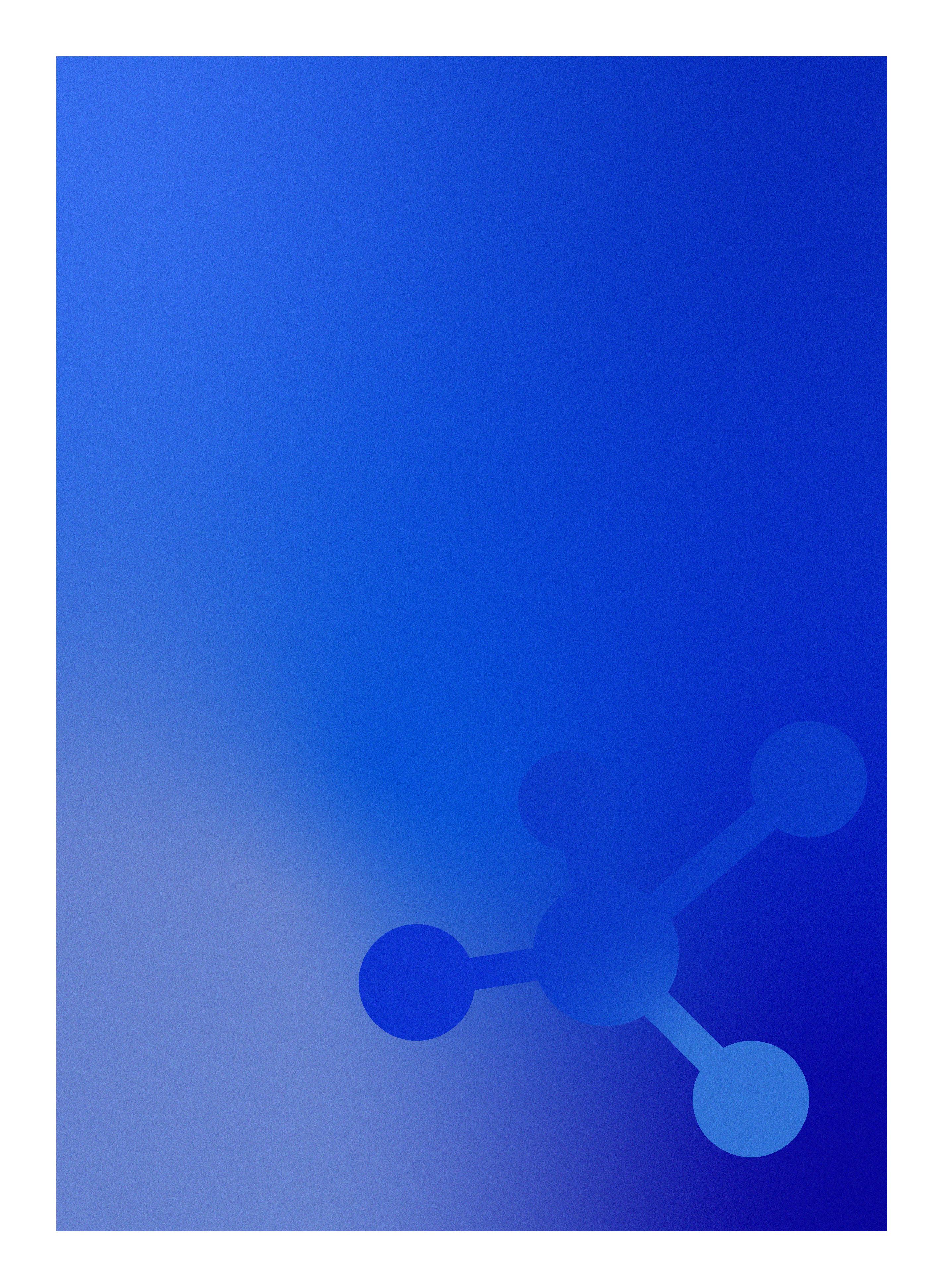
The year 2020 witnessed the blossoming of generative AI, a subset of artificial intelligence (AI) that can generate new content such as text, video, and audio based on user prompts, with popular applications like ChatGPT, Google Gemini, and Dall-E (European Parliament, 2023 & McKinsey, 2024). Generative AI is predicted to contribute an annual value of 4.4 billion USD to the global economy and revolutionize productivity in every organization (Chui et al., 2023). J.P. Morgan reveals that generative AI is believed to be the most prevalent in marketing, with 28% votes, followed by legal services and insurance, media, data analytics, and consumer technologies (2024). The evolution of generative AI has significantly changed how humans and machines interact, especially with the development of AI agent systems. Emergen Research (2024) estimates that the global market of AI agent systems will reach 30.89 billion USD with a compound annual growth rate (CAGR) of 31.68% in the period from 2024 to 2033. The BCG analysis (n.d.) further articulates that AI agents are picking up steam across a vast array of business applications with a CAGR of 45% in the next five years.
AI Agents Are Becoming More Prolific Across Tech Applications, Growing with a 45% CAGR Over Five Years
Acknowledging the potential of AI agents, a survey conducted by Khmel indicates that 82% of businesses plan to deploy these systems into their organizations in the next three years (2024). By the end of 2025, there will be approximately 50 to 100 billion AI agents integrated into various types of businesses, providing human workers with the "necessary tools, data, and capabilities" to increase e ciency and bring about a new form of human-machine collaboration, generating immense values in all business sectors (Amazon, n.d.; Gomez, 2024b). These agent systems will deliver improved productivity by automating tasks and streamlining processes at high speed. AI agents can also redefine the next frontier of service quality through 24/7 support, personalized interactions, and seamless engagement. Additionally, they can enable e cient data-driven decisions with powerful predictive analytics. Beyond operational benefits, these self-learning AI agents will help organizations drive innovation and create a sustainable competitive edge. Motivated by fast-growing market trends and high application potential, this white paper aims to provide an outlook on AI agents in action for business leaders and explore how businesses can take advantage of AI agents to unlock unprecedented e ciency, scalability, and innovation.
In terms of theoretical research, the concept of AI agents is not new. They have previously provided the foundation for numerous computational models and applications. According to IBM, an AI agent is “a system or program that is capable of autonomously performing tasks on behalf of a user or another system by designing its workflow and utilizing available tools” (Gutowska, 2024a).
On a technical aspect, an agent system consists of four key components to serve user requests, resembling how a human works: the core (brain), memory, planning capabilities, and tools (Han et al., 2024; Leeway Hertz, n.d.; & SuperAnnotate, 2024b).
Figure 2: The main components of an AI agent resembling how a human works
The core, or the brain, of the agent system is made up of large language models with advanced language understanding capabilities and adaptive features such as persona configuration (SuperAnnotate, 2024b; Zhang et al., 2024). This is also why an AI agent is referred to as an LLM agent (Bozorg, 2024).
Next is memory, including short-term and long-term memory, to help the system improve and handle more complicated tasks based on past interactions (Kumar, 2024; SuperAnnotate, 2024b).
Short-term memory helps the agent stay within the context of the current conversation and provide relevant responses. However, this memory is temporary, so it will be cleared out once the agent system completes the assigned task. Long-term memory stores information from previous conversations, allowing the agent system to identify patterns, learn from experience, and recall such insights to perform better in similar situations in the future.
Planning capabilities allow the agent system to reason, decompose tasks into more manageable parts, and make specific plans to perform them (IBM, 2024; SuperAnnotate, 2024b). Additionally, an agent system is capable of adjusting the plans according to real-world circumstances (SuperAnnotate, 2024b).
Finally, tools allow the agent system to connect with external environments (i.e., business databases) to complete assigned tasks (Gutowska, 2024a; SuperAnnotate, 2024b). The external sources vary depending on the nature of the tasks, like extracting, querying, coding, and generating content.
There are two forms of AI agent systems available for businesses depending on the use cases: single-agent systems or multi-agent systems. In a single-agent system, there is only one AI agent to engage with the surrounding environment and attain pre-defined goals. According to Figure 3, a single-agent system typically includes the interactions between the environment and the agent model itself through actions and feedback (Stone & Veloso, 2000; SuperAnnotate, 2024b). Each agent will have a specific goal, a defined course of action, and domain knowledge to support it in completing a task, such as searching for product information for customers.
Figure 3: A general framework of a single-agent system
A multi-agent system (MAS) “consists of multiple artificial intelligence (AI) agents working collectively to perform tasks on behalf of a user or another system” (Gutowska, 2024b). Each agent model possesses di erent goals, actions, and domain knowledge.
In terms of system components, multi-agent systems also leverage large language models in their core, along with memory, planning capabilities, and tools, similar to single-agent systems. Nevertheless, multi-agent systems often require more advanced planning capabilities to enable e cient collaboration among AI agents and more tools to complete subtasks and fulfill multi-intent user requests (Gutowska, 2024b; Stone & Veloso, 2000; SuperAnnotate, 2024b). Since multi-agent systems have such collaborative capabilities, they can fulfill more functionalities, including but not limited to natural language processing, decision-making, problem-solving, and so on (Bozorg, 2024).
Figure 4: A general framework of a multi-agent system
Both single-agent systems and multi-agent systems provide distinct advantages and limitations depending on the complexity and scale of the tasks involved. Generally, multi-agent systems outperform single-agent systems by leveraging collaborations among agents and their specialized abilities (Han et al., 2024; Gutowska, 2024b).
Table 1: Comparison of single-agent and multi-agent systems (Bozorg, 2024; Gutowska, 2024b; Leeway Hertz, n.d.)
Complexity
Coordination
Scalability
Use cases
Decision making
Resource allocation
Adaptability
Single-agent system
Generally simpler, with fewer interactions.
Not required, as there is only one agent.
Limited to the capabilities of a single agent.
Simple, straightforward tasks, single-user applications.
Decisions are taken by a single agent, based on its own goals.
Lower resource use, managed by a single agent.
Limited to the capabilities of one agent.
Multi-agent
More complex, involving interactions between multiple agents.
Required to manage interactions and conflicts among agents.
More scalable as additional agents can be added to handle complex tasks.
Complex tasks, collaborative work, distributed systems.
Distributed among multiple agents, which may have di erent goals.
Higher computational requirements to enable coordination among agents.
Can adapt to dynamic environments through the collective behavior of agents.
Single-agent systems, such as basic virtual assistants, are ideal for straightforward, repetitive tasks that require minimal decision-making, such as providing information about opening hours, tracking orders, or answering frequently asked questions (FAQs) (Ignise & Vahi, 2024; Pum, 2022). For example, a single-agent system could be used in an e-commerce website to guide users through the checkout process or assist with password recovery. Nevertheless, their limited adaptability becomes a drawback when the scope of customer inquiries expands or when the system encounters an unfamiliar issue, leading to a bottleneck in service delivery. If this single agent fails (e.g., a system crash), it often necessitates human intervention when customer issues exceed the system's functional capabilities, causing delays and customer dissatisfaction.
In contrast, multi-agent systems are ideal for dynamic, complex tasks (Han et al., 2024; Ignise & Vahi, 2024). This collaborative approach magnifies scalability, as additional agents can be deployed to manage higher customer volumes during peak hours. Moreover, multi-agent systems are more robust in the sense that if one agent fails (e.g., the payment processing agent), other functions like product consultation and order processing agents can continue operating, minimizing disruptions. For instance, in e-commerce, MAS can integrate the following specialized agents to deliver comprehensive customer support with e ciency and accuracy:
Query Agent:
Handle initial customer inquiries and route them to the human agents appropriately;
General Inquiry Agent:
Address primary questions, identify if a product return is eligible, and escalate to the relevant agent;
Recommendation Agent:
Product Return Agent:
Process return requests and transfer them to the Return Processing Agent;
Return Processing Agent:
Finalize the return procedure, update order status, and provide confirmation to the customer;
Analyze customer preferences and purchase history to suggest suitable products and services.
As an innovative consumer finance company experienced a surge in customer engagement across multiple channels, the demand for quicker, more e cient service was growing. Automating customer services became essential to reduce wait times and improve response e ciency, which would not only amplify productivity but also enhance customer satisfaction, ensuring that the company can meet the expectations of a rapidly expanding customer base.
Solution: AI agents are deployed across multiple digital channels, such as the call center, website, and social media platforms, to automate 100+ customer service tasks.
Inbound calls: FAQ (cards, loans, others), Self-service (card lock/activation), Search (card information, contract/order information), Support (complaint, feedback, issue report)
Outbound calls: Customer service (welcome call, CSAT), Collection (pre-due/overdue collection), Telesales (card, loan)
Message-based conversations: Customer service (card deactivation, inquiry of loan information, inquiry of card information), Service-to-sales
Implementation Results:
12,000,000 automated calls per month
90%+ customer inquiries resolved
60% operating expenses saved
Leveraging a strong, growing customer base, a leading consumer finance company in Vietnam needed a scalable solution to strengthen telesales e ciency without the high costs of hiring additional sta . Traditional methods were time-consuming, making it di cult to connect with potential customers in a quick and e cient manner. As a result, the company sought a tech-driven solution to automate outreach, personalize o ers for customers, and increase conversion rates.
Solution: AI agents are integrated into the call center to automate outbound calls, qualify loan leads for new customers, and cross-sell to existing clients. Applying state-of-the-art technologies, these AI agents can personalize interactions, refine customer engagement, and boost sales growth.
Implementation Results:
1,000,000 - 1,500,000 automated calls/month
700,000 - 1,000,000 customers reached/ month
15–20% conversion rate for hot leads
With more than 1,000 stores scattered across Vietnam, the fashion retail chain needed a technology solution to provide professional, high-quality learning experiences for its sales employees. By implementing a comprehensive training platform, the company targeted to ensure organization-wide compliance, build up employee skills, and build a competent workforce, strengthening performance and maintaining its consistent service reputation for excellence across all locations.
Solution
AI agents are embedded into the business system to provide an intelligent training platform for the sales workforce. Every sta can receive daily, personalized assessments with up-to-date content generated from the business database and access knowledge anytime, anywhere. Furthermore, the agent system has holistic dashboards for both employees and managers to track progress and obtain recommendations for adequate learning programs and career paths for each personnel.
100% employees participated per month
55% employee competency increased
80% training e orts and resources saved
With thousands of sales agents operating nationwide, the support workloads for sales agents were heavy and time-consuming. Thus, the insurance company realized the imperative to streamline internal support, providing real-time access to sales process guidance, product insights, and IT helpdesk assistance through innovation.
Solution: AI agents are integrated into the internal website and call center to automatically handle simple to complex issues about product updates, sales procedures, and internal processes.
▪ Assist IT Helpdesk
▪ Search information during online sales sessions with the customer
Implementation Results 98% 38%
▪ Resolve inquiries about promotions, services, and products
▪ Resolve FAQs related to the onboarding process and tax issues
▪ Request the sales agent to provide additional documents during the onboarding process
Implementation Results:
task success rate productivity improved
From an AI agent that detects and flags fraudulent transactions in real time to a multi-agent system that automates customer interactions across all business channels, AI agents are the next transformative force for businesses. So, how can enterprises leverage these intelligent systems to boost compliance monitoring, optimize workflows, and enhance customer service?
This is where di erent frameworks come in.
IT architectures are shifting from traditional application-focused models to multi-agent systems, where businesses manage large numbers of AI agents that work together and communicate with various systems to achieve common goals (See Figure 5).
McKinsey specifies that there are three main ways to deploy AI agents, namely Super Platform, AI Wrappers, and Custom AI Agents (Kaplan et al., 2024).
▪ Super Platforms: These next generations of third-party applications integrate AI agents that perform tasks. For example, an upgraded CRM platform allows users to automatically generate sales reports and interact with analytics tools through a conversational interface. They are pre-packed based on specific industry standards and can be quickly put into use. However, this approach has low scalability to expand use cases when necessary.
▪ AI Wrappers: These intermediary platforms allow enterprise services to connect and collaborate with third-party services via APIs while protecting proprietary data. For instance, a bank might use an AI wrapper to interact with an external service for card activation/deactivation or password recovery without exposing sensitive customer data. This model enables businesses to not only rapidly adopt innovation but also e ciently maintain a high level of agility and security.
▪ Custom AI Agents: These agent systems are developed internally by using pre-trained large language models (LLMs) or retrieval-augmented generation (RAG) with company-specific data. For example, a custom AI agent could assist call center sta by using internal data like customer information and company policies to answer queries. While this method helps to preserve the highest level of security, it can be time-consuming and resource-intensive to manage a full cycle of development, implementation, and maintenance.
Figure 5: The evolution of architectures to implement AI agents (Kaplan et al., 2024)
SaaS, packaged apps Third-party super platforms
▪ AI-enabled via 3rd-party platforms
▪ Best for rapid deployment of industry-standard capabilities (e.g., CRM, ERP)
Platform-based apps
Customer built in-house
AI wrappers + Third party
▪ Enterprise creates its own AI wrapper to enable API access for 3rd parties to interact with the enterprise’s platforms
▪ Fit for accessing latest innovation while preserving flexibility and protecting proprietary data
▪ Full stack of AI agents built via shaping a previously trained large language model
▪ Fit for protecting proprietary data and creating di erentiating intellectual property
Despite outstanding advantages, the deployment of AI agents entails notable challenges in terms of system integration, performance, security, and resource allocation (Gutowska, 2024b; Han et al., 2024; Leeway Hertz, n.d.; Singh et al., 2014).
1. Complexity: Determine whether a single-agent or multi-agent system is required based on the complexity of tasks. Multi-agent systems may involve interactions between di erent agents, with human intervention in certain cases. customers.
2. Deployment Approach: Choose a deployment approach that matches your organizational capabilities. AI agents present huge challenges related to time and cost, requiring significant computation and infrastructure investments, coupled with a high level of expertise for in-house system design and maintenance.
3. Ease of Use: Choose a framework that matches the skill level of your development team. Beginner-friendly frameworks o er no-code interfaces, while more advanced options provide deeper customization and control.
4. Data Privacy and Security: Ensure the framework prioritizes security through encryption, access controls, and secure handling of sensitive information.
5. Seamless Integration: Evaluate how well the framework integrates with your existing tech stack, data sources, and deployment environment (on-premises or cloud).
6. Performance and Scalability: Assess response times, latency, and the ability of the framework to handle large data volumes or concurrent requests. Also, businesses should consider its scalability to accommodate business growth.
7. Human Resistance: Invest in AI training and education, helping employees see how AI can enhance their work rather than replace them. Businesses should be transparent about their AI implementation, clearly communicating its purpose and benefits to both employees and customers.
The FPT AI Agents platform enables e ortless development and operations of multi-lingual AI agents, utilizing powerful generative AI technologies to cultivate the future of human-AI collaboration. Businesses can easily manage FPT AI Agents using natural language without the need for technical expertise. Currently, FPT AI Agents can process many languages, such as Vietnamese, English, Indonesian, and Japanese.
▪ Dynamic engagement: Make natural, flexible engagement with users on various messaging and call channels, fueled by advanced large language models and enterprise knowledge.
▪ Amplify productivity: Execute tasks with excellence with deep integration with the business system to optimize task processing capabilities and enterprise data, increasing productivity by 67%.
▪ Rapid implementation: Deploy, fine-tune, and train AI agents e ortlessly and quickly with active learning capabilities and natural language interactions.
Figure 6: Implementation process for FPT AI Agents
Define use cases
Initiate an AI agent
Set the targets; update knowledge and business descriptions
Conduct testing
Launch
Identify constraints if necessary
Adjust to improve user experience
Operating on a robust, agile cloud infrastructure of FPT AI Factory, FPT AI Agents can adapt to any business need and ensure seamless performance and scalability while maintaining high e ciency. In 2024, as a preferred partner of NVIDIA, FPT launched FPT AI Factory – a comprehensive suite for the end-to-end AI development cycle, including three main components. FPT AI Factory is further integrated with 20+ ready-to-use AI products for rapid AI adoption and instant results in elevating customer experience, achieving operational excellence, transforming the human workforce, and optimizing operating expenses. Powered by NVIDIA H100/H200 Tensor Core GPUs, FPT AI Factory will empower businesses to create AI agents that serve various purposes in minutes or hours.
The shift to AI agents marks a paradigm change in how businesses approach customer service and complex operational challenges. As AI agents continue to evolve, they are becoming indispensable tools for businesses aiming to optimize processes, elevate customer experiences, and sustain a competitive advantage in an ever-changing marketplace. Nonetheless, the implementation of AI agents implies many issues, such as system complexity and resource allocation, calling for robust frameworks, high technical expertise, and heavy infrastructure investments. Tackling these hurdles through continued research and innovation will be critical in unlocking the full potential of multi-agent systems in diverse domains.
Leveraging years of research, development, and industry expertise, FPT has launched FPT AI Agents to revolutionize human-AI collaboration. By enabling e ortless deployment, seamless integration, and dynamic multi-lingual interactions, FPT AI Agents will pave the way for businesses to adopt the latest AI technologies with speed and e ciency. As businesses navigate the evolving digital landscape, FPT is ready to be your trusted partner in this transformative AI journey, helping you achieve new levels of innovation and growth.
FPT Smart Cloud – the 8th subsidiary of FPT Corporation, is a technology company that provides a world-class Artificial Intelligence (AI) platform, Cloud Computing services, and Data Platform. FPT Smart Cloud was established to transform businesses into digital businesses by innovating technologies and products.
As a leading AI provider in the region, FPT Smart Cloud established FPT.AI to o er comprehensive products and solutions, striving to drive operational excellence, elevate customer experience, transform the workforce, and accelerate innovation. FPT.AI is trusted by more than 200 large enterprises in Vietnam and across the world in various sectors, namely Banking, Finance, Insurance, e-commerce, Healthcare, and Logistics, serving millions of end-users every year.
For more information, please contact us at
Website: https://fpt.ai/ Hotline: 1900 638399 Email: support@fpt.ai
Amazon (n.d.). What are AI Agents?. Retrieved on 4 January 2024 at https://aws.amazon.com/what-is/ai-agents/#:~:text=Improved%20customer%20experience,engagement%2C%20conversion %2C%20and%20loyalty.
BCG analysis (2024). AI Agents. Boston Consulting Group. https://www.bcg.com/capabilities/artificial-intelligence/ai-agents Bozorg, J. (2024, December 19). What is agentic architecture?. IBM. https://www.ibm.com/think/topics/agentic-architecture Chui, M. et al. (2023, July 14). The economic potential of generative AI: The next productivity frontier. McKinsey & Co. https://www.mckinsey.com/capabilities/mckinsey-digital/our-insights/the-economic-potential-of-generative-ai-the-next-pr oductivity-frontier#introduction
Dwivedi, D. (2024, June 15). Single-Agent vs. Multi-Agent Generative AI: Comparative Insights and Industry Applications. Medium.
https://dwivedy.medium.com/single-agent-vs-multi-agent-generative-ai-comparative-insights-and-industry-applications-c 48f1e505d9d
Emergen Research. (2024). Agentic Artificial Intelligence (AI) Market, By Component (Hardware, Software, Services), By Technology (Machine Learning (ML), Natural Language Processing (NLP), Computer Vision, Robotics), By Industry Vertical (Healthcare, Finance, Retail, Manufacturing), By Deployment Model (On-Premise, Cloud-Based, Hybrid), and By Region Forecast to 2033.
https://www.emergenresearch.com/industry-report/agentic-artificial-intelligence-market/research-methodology European Parliament (2023, December 13). Generative AI and watermarking. https://www.europarl.europa.eu/thinktank/de/document/EPRS_BRI(2023)757583 Gomez, K. (2024a, July 17). Multi-Agent vs Single Agent. Medium. https://medium.com/@kyeg/multi-agent-vs-single-agent-a72713812b68 Gomez, K. (2024b, August 30). The Agentic Economy: How Billions of AI Agents Will Transform Our World. Medium. https://medium.com/@kyeg/the-agentic-economy-is-coming-ecf789a370f2 Gutowska, A. (2024a, July 3). What are AI agents?. IBM. https://www.ibm.com/think/topics/ai-agents Gutowska, A. (2024b, August 6). What is a multi-agent system?. IBM. https://www.ibm.com/think/topics/multiagent-system Han, S., Zhang, Q., Yao, Y., Jin, W., Xu, Z., & He, C. (2024). LLM multi-agent systems: Challenges and open problems. arXiv preprint arXiv:2402.03578.
Ignise, A., & Vahi, Y. (2024). Applications of Multi-Agent Systems. J.P.Morgan. (2024 February 14). Is generative AI a game changer?. https://www.jpmorgan.com/insights/global-research/artificial-intelligence/generative-ai Kaplan, J., Gu, M., & Sinha, M. (2024, December 2). Enterprise technology’s next chapter: Four gen AI shifts that will reshape business technology. McKinsey.
https://www.mckinsey.com/capabilities/mckinsey-digital/our-insights/enterprise-technologys-next-chapter-four-gen-ai-shif ts-that-will-reshape-business-technology
Khmel, M. (2024, September 29). AI Agents in action. How are they shaping our world today?. Medium. https://medium.com/@mariakhmel/ai-agents-in-action-how-are-they-shaping-our-world-today-30f8b0541e2e Kumar, V. (2024, November 27). Short-term vs. Long-term Memory in AI Agents. The Association of Data Scientists. https://adasci.org/short-term-vs-long-term-memory-in-ai-agents/ Leeway Hertz (n.d.). Multi-agent system: Types, working, applications, and benefits. Retrieved on 7 January 2024 at https://www.leewayhertz.com/multi-agent-system/ McKinsey (2024, April 2). What is Generative AI?
https://www.mckinsey.com/featured-insights/mckinsey-explainers/what-is-generative-ai Pum, Mengkorn. (2022). Multi-Agent Conversational Systems. https://www.researchgate.net/publication/387669935_Multi-Agent_Conversational_Systems Singh, A. J., Dalapati, P., & Dutta, A. (2014). Multi-agent based dynamic task allocation. In Agent and Multi-Agent Systems: Technologies and Applications: Proceedings of the 8th International Conference KES-AMSTA 2014 Chania, Greece, June 2014 (pp. 171-182). Springer International Publishing. Stone, P., & Veloso, M. (2000). Multiagent systems: A survey from a machine learning perspective. Autonomous Robots, 8, 345-383. https://doi.org/10.1023/A:1008942012299 SuperAnnotate. (2024a, September 9). Multi-agent LLMs in 2024 [+frameworks]. https://www.superannotate.com/blog/multi-agent-llms SuperAnnotate. (2024b, October 22). LLM agents: The ultimate guide. https://www.superannotate.com/blog/llm-agents#what-can-llm-agents-do Zhang, C., He, S., Qian, J., Li, B., Li, L., Qin, S., ... & Zhang, Q. (2024). Large language model-brained GUI agents: A survey. arXiv preprint arXiv:2411.18279.