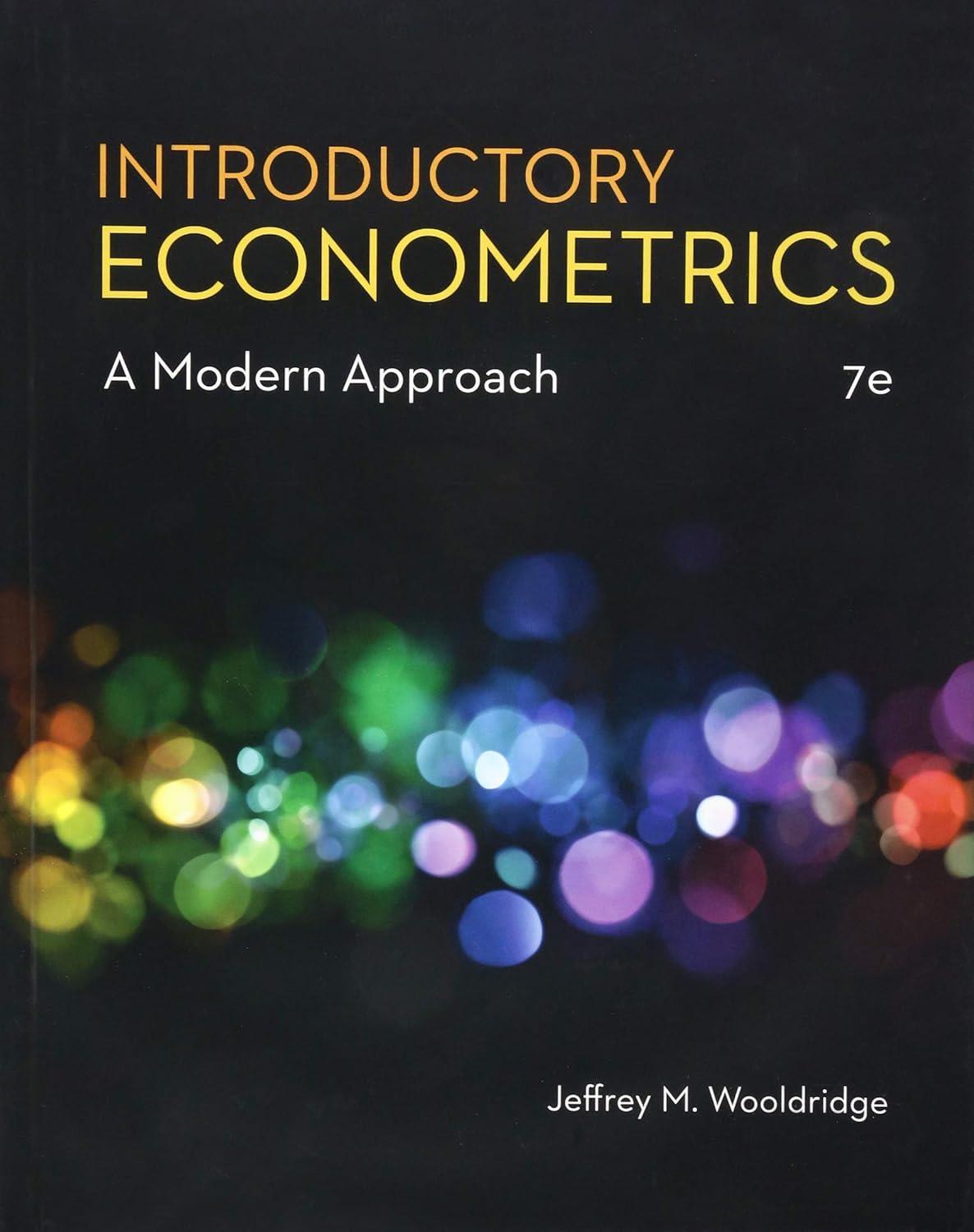
In-Depth Dive into Introductory Econometrics: A Modern Approach (7th Edition) by
Jeffrey M. Wooldridge
Wooldridge's "Introductory Econometrics: A Modern Approach" (7th edition) isn't just another econometrics textbook. It's a comprehensive guide that equips you with the knowledge and tools to analyze economic data and answer critical questions that impact real-world scenarios. Here's a detailed breakdown of the key areas the book explores:
Part 1: Foundations
• Chapter 1: Introduction: This chapter sets the stage by introducing the field of econometrics and its role in economic analysis. It highlights the importance of causal inference and the distinction between correlation and causation, a fundamental concept in econometrics.
• Chapter
2: Review of Probability and Statistics:
This chapter refreshes your memory or introduces you to core statistical concepts like probability distributions, hypothesis testing, and confidence intervals. These tools form the foundation for drawing inferences from econometric models.
• Chapter 3: Asymptotic Theory: This chapter delves into a more advanced concept – asymptotic theory. It explores how statistical properties of estimators (like the sample mean) behave as the sample size increases. Understanding asymptotic theory helps interpret the reliability of results obtained from econometric analysis.
Part 2: The Linear Regression Model
• Chapter 4: The Simple Linear Regression Model: This core chapter introduces the workhorse of econometrics: the simple linear regression model. Here, you'll learn how to estimate the slope and
intercept of a line representing the relationship between a dependent variable (the variable you're trying to explain) and an independent variable (the variable you believe is influencing the dependent variable). You'll also explore how to assess the model's goodness-of-fit and diagnose potential problems like multicollinearity (when independent variables are highly correlated).
• Chapter 5: Multiple Regression Analysis:
Estimation: This chapter builds on the foundation of simple regression and introduces multiple regression analysis. Here, you'll learn how to estimate the relationship between a dependent variable and multiple independent variables, considering the influence of each independent variable while controlling for the effects of others.
• Chapter 6: Multiple Regression Analysis:
Inference: Once you've estimated a multiple
regression model, this chapter equips you with the tools to assess its statistical significance. You'll learn how to perform hypothesis tests on the estimated coefficients, interpret p-values, and construct confidence intervals to gauge the precision of your estimates.
Part 3: Specification and Testing
• Chapter 7: Specification Error: This chapter delves into a critical aspect of econometrics: specification error. It explores what happens when the model you choose doesn't perfectly capture the true relationship between the variables. You'll learn about omitted variable bias, a common problem that arises when important explanatory variables are left out of the model, and how it can lead to misleading results.
• Chapter 8: Heteroskedasticity: This chapter introduces heteroskedasticity, a situation where the variance of the error term in a regression model is not
constant across all observations. You'll learn how to detect heteroskedasticity and explore methods to address it, ensuring the validity of your statistical inferences.
• Chapter 9: Functional Form: This chapter explores the concept of functional form, which refers to the specific mathematical relationship between the dependent and independent variables in your model. You'll learn how to test for different functional forms and choose the most appropriate one for your analysis.
Part 4: Special Topics in Linear Regression
• Chapter 10: Measurement Error: No data is perfect, and this chapter addresses the issue of measurement error, where the observed values of variables might not perfectly reflect their true values. You'll learn how measurement error can affect your estimates and explore techniques to mitigate its impact.
• Chapter 11: Qualitative Information and Limited
Dependent Variables: This chapter expands your toolkit by exploring how to incorporate qualitative information (like categorical variables) into your econometric models. It also introduces models for analyzing data where the dependent variable is limited in its range, such as binary choice models used to predict probabilities (e.g., the probability of a customer purchasing a product).