6 minute read
Building a greener future
MACHINE LEARNING
Building a greener future
In light of the current global political situation, the rapidly changing market environment, and the worldwide efforts to decarbonize industrial production, there is no question that our industry’s ability to transform into a reliable supplier of green steel quickly is crucial to our future viability. This article will reveal how firms can achieve that sustainable goal without setting aside – rather, actually increasing–profitability. By Berk Birand*
IT’S no secret that there has been extreme uncertainty and volatility present in the manufacturing sector over the past three years. In 2021, most manufacturers had tremendous success. Demand was high, boosted by low interest rates, higher savings rates, and the promise of government spending like the infrastructure bill. As a result, almost every sector achieved incredibly high profit margins. We saw steel companies’ margins go up to 25%, compared to typical margins of 7%. Most manufacturers knew this kind of success was never going to last, so they made the most of it while they could.
My hope is that the high profitability achieved last year will help manufacturers survive the potential rough road ahead. An economic downturn could present several challenges, including rises in commodity prices and raw material costs, decreased demand, and the need to downsize workforces to maintain profitability.
As the regulatory environment tightens, involving increased scrutiny of CO2 emissions, producers that are not able to keep up with this change will, sooner or later, no longer have a market. Sales may not be recovered by the competition, but will simply disappear. As a result, entire business models are at stake.
The road to true green steel is still long and highly complex. However, enormous potential is already lying fallow today. By optimizing the existing process to reduce raw material consumption and avoid scrap, we can create new paths of profitability and move closer to achieving sustainability goals at the same time.
Optimizing raw material consumption is one way to achieve the twin goals of profitability and sustainability. Traditionally, steel engineers tend to overestimate the quantity of alloys they will need to ensure a given heat of steel ends up within quality specs, and will add these materials generously in order to avoid ending up in the undesirable situation of needing to scrap the heat. However, with machine learning algorithms, we can transform this process to minimize the amount of costly new alloys added during production.
At Fero Labs, we make factory optimization software that enables manufacturers to stay ahead of everchanging production conditions. Fero’s white-box ML software adapts to real-time fluctuations in raw material composition, significantly reducing the likelihood of scrap in the production process. This saves both raw materials and energy.
Making supply chains more sustainable can be a challenge, because the work is so siloed. Typically you will see different teams working on different objectives. Some are optimizing quality, while others are trying to cut costs. As a result, sustainability often ends up falling into the bucket of lower-tier priorities.
That’s where digital twins change the game. Using historical data, a digital twin can be built that creates a virtual copy of an existing steel plant. Engineers can then use this tool in conjunction with machine learning models to create a more efficient supply chain that optimizes for every goal at the same time, from cutting costs to reducing greenhouse gas emissions.
Saving 9% on raw materials
Together with our partner Gerdau, we are working on influencing the variance in the production process in real-time by optimizing the use of raw materials. As a result of using Fero software, the steel manufacturer is achieving 9% yearly savings with an average production volume.
Gerdau initially approached us because
*CEO, Fero Labs
MACHINE LEARNING
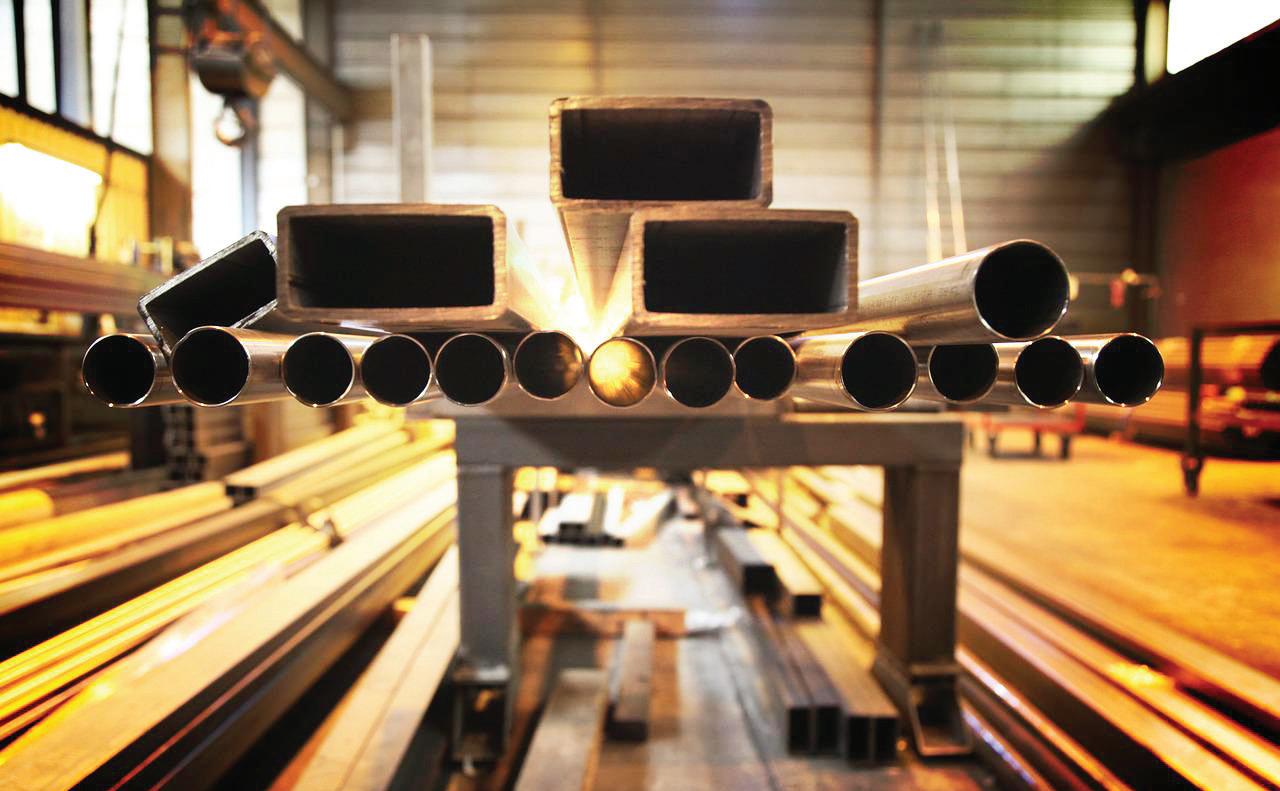
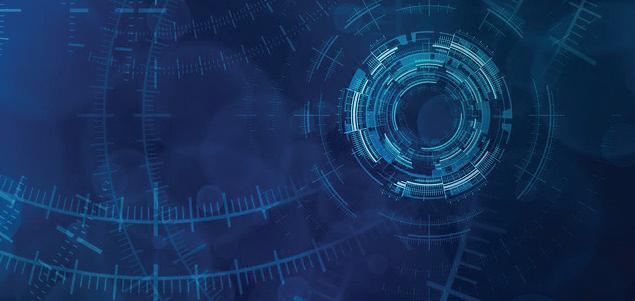
they wanted to lower production costs, while maintaining the same quality steel. Traditional tools were unable to make real-time adjustments or evaluate multiple non-linear relationships between data points. With Fero software, they were able to take various data points, including production temperatures and the final steel product thickness, and analyze them to see how they could optimize different aspects of the process.
Once they had understood much of their data through insights that had previously been unavailable to them, the steel manufacturer focused on optimizing the raw material process. For this they tapped into Fero’s software, which learns from historical data to recommend the minimum amount of additional new material (if any) that needs to be added to a particular batch of molten recycled steel, thereby reducing the amount of freshly mined materials used. As recommendations are provided in real time, this approach also decreases the amount of time that the steel must be maintained in a molten state, reducing overall energy usage during production.
Fero analyzed each heat in real time and told operators how much alloy to use so that they would meet quality specifications. Within six months of deployment, the average plant saw alloy reduction corresponding to $1-$6 per ton in raw material savings. Scaled across five or more plants, the results were even more dramatic, highlighting the vast amounts steel manufacturers could potentially save by deploying Fero across a wider number of grades.
The project proves that machine learning can significantly reduce waste and provide users with a clear competitive advantage. Machine learning tells Gerdau operators the exact amount of alloys they need to produce each heat, thus reducing the waste created by overdesign and adding money to the plant’s bottom line. This method also helps the manufacturer use fewer of the mined resources required to produce these ingredients–making their steel greener and better for the planet.
Steel production accounts for more than a fifth of greenhouse gas emissions from manufacturing, making it a prime target for emissions reduction. Fero software reduces the use of mined ingredients in steel production by up to 34%, an approach that can be scaled to eliminate millions of tons of CO2 emissions annually, according to a recent report by the Global Partnership on Artificial Intelligence.
When employed by steel plants, Fero’s white-box ML-driven optimization software reduces alloy usage by up to a third. Avoiding mining, smelting, and transporting these alloys has so far prevented an estimated 450kt of CO2 emissions per year. If scaled to the rest of steel production in the US, this approach could prevent 11.9 Mt of emissions per year — equivalent to a quarter of New York City’s yearly CO2 emissions.
Tackling climate change is more crucial than ever. For manufacturers reluctant to jeopardize production speed or quality to comply with environmental regulations, Fero provides a solution to lower costs and hit sustainability goals. The next 12 months will be transformative for manufacturers. They will have to start focusing on improving margins and profitability in light of continued supply chain issues. In the end, the most resilient manufacturers will emerge from this recession stronger than before.
It’s key to remember that machine learning is not magic. This is a statistical discipline that can be used in a very predictable way to reduce emissions and cut costs while maintaining quality. By allowing customers to fully exploit the possibilities of automated data processing in production, we can achieve a greener future for the steel industry. �