What is AI Powered Product Recommendation Engine and How Does It Works?
Personalization is now becoming a trending and attractive feature to get users' attention and engage them better with the platform.
Well, most of us can relate with this personalization under numerous circumstances, such as while surfing Netflix, the platform amazes with making accurate recommendations as per viewers expectation.
The platform knows very well what next the user would like to explore and spend their few spare hours on the Netflix app
All such recommendations usually come as a surprise for viewers, but let me clear the air, all this becomes possible with Artificial Intelligence.
Yes, almost every medium to large scale on-demand service provider is working on AI-powered recommendation engine to serve their customers or users with utter precision. The recommendation engine has induced a new energy in businesses. And it gets reflected into increased customer reach, conversation rate, user satisfaction, good ROI, and many more.
The AI powered recommendation engine is playing a vital role in almost every industry. If you are curious to understand what exactly the recommendation engine is, how it works, or what are its benefits for businesses- then let’s answer all your queries ahead.
Continue reading and get to know how businesses work nowadays while tapping human psychology.
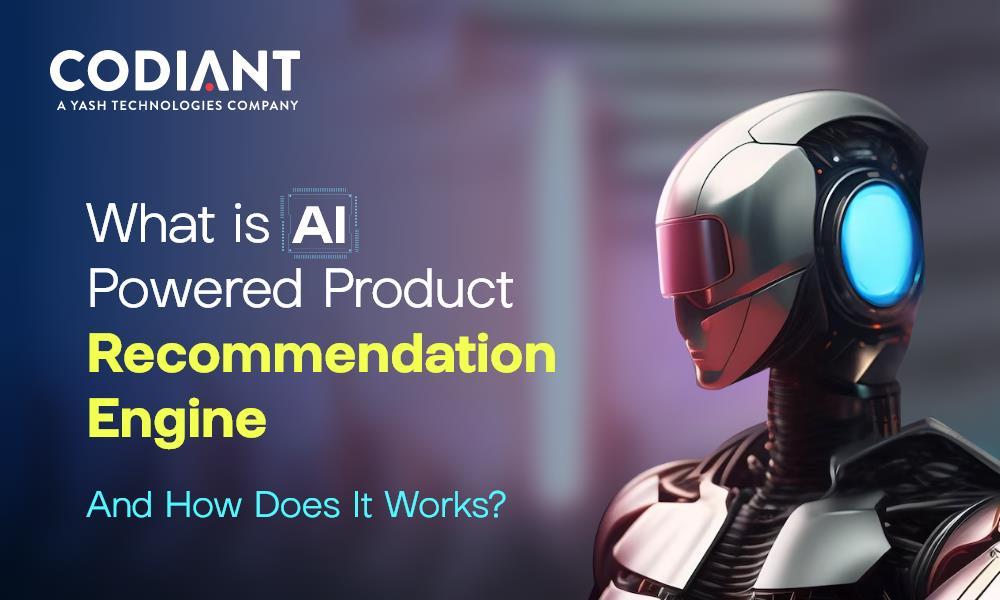
What is Product Recommendation Engine?
A Product Recommendation Engine is a well-formulated and intelligently designed solution that follows an algorithm. The algorithm ensures to display relevant product suggestions to every individual user.
In general words, product recommendation engine is an AI-enabled data filtering tool, which follows an algorithm to suggest the most relevant items to every customer. The algorithm follows a pattern while analyzing user behavior data.
Not only Artificial Intelligence, but also Machine Learning, Big Data, Natural Language Processing technologies, enables recommendation algorithm to suggest products in such a way that under higher mathematical probability the user likes the specific suggestions or recommendations made on the particular platform.
The AI recommendation systems are rapidly disrupting every industry. They have become an easy formulae for interacting with the users and habituating them to the online e-commerce by extracting their behavior, preferences, interests, and experiences.
Understanding Two Different Modes of Product Recommendation Engine
Hard-Coded Product Recommendation Engine
Hard-coded model is a traditional recommendation system that works on a trigger and rule basis. Under this, the user performs a definite action and the platform sends the offer.
The action could be product view, review or feedback, adding the product in the cart, or purchasing it, etc.All these activities demonstrate user’s interest in the particular product, category, price range, etc.
As the name suggests, the traditional system is quite outdated now and possesses multiple drawbacks which generated the demand for a new and automated recommendation system. Some drawbacks of hard-coded recommendation engine are;
• It fails to work effectively during daytime and pays no heed to the changes in seasonal trends and consumer behavior.
• It requires manual changes to track updates.
• It is least effective for big organizations having a huge number of product options.
AI-Powered Product Recommendation Engine
AI powered product recommendation engine provides the solution to all the drawbacks/issues hard-coded product recommendation engine experiences. It is a step ahead tool in terms of data analysis and capabilities.
Some of the benefits of AI-powered product recommendation engine over traditional recommendation system are as follows:
• It can effectively recommend the right product to the customer in real-time.
• It can manage a huge database to carry out single sales activity instantly.
• Customer data analysis becomes quite easier to understand features and benefits of products. This helps to recommend the right product to right customer based on their past and ongoing activities.
Overall, such on-point product recommendation supports companies from every angle. Right from enhancing their sales by analyzing the customer’s past and current browsing behavior to increased customer satisfaction, it changes the overall shopping dynamics.
How does AI Powered Product Recommendation Engine Work?
The AI powered recommendation engine usually goes through four phases in data processing, namely;
• Collecting
• Storing
• Analyzing
• Filtering
1. Collecting
To setup a product recommendation engine, the first step is collection of explicit and implicit data. Explicit Data, is user activity data such as comment, share, like, feedback, rating, etc. Implicit Data, is details about cart activities, browsing history, page views, return history, etc.
All such data collection requires time but is quite an easy process, as you have to track activity data from your website/application only.
In addition to that, the best thing about data collection is that you don’t have to seek any assistance or actions from the users.
The recommendation engine becomes smarter in its job, as it fed with the data. Evidently, as the quantity of data collection grows, the recommendation engine can analyze it better and yield more precise product suggestions.
2. Storing
In a period of time, the collection of data will expand gradually and the intelligent recommendation system can deliver more precise suggestions. Hence, to store such a rapidly increasing data size, you need a database to store it.
The type of data the recommendation engine is fed with, will determine the storage type your business database requires. So, the available database options could be standard SQL, NoSQL, object storage, and many more. The factors that determine the choice of right storage type are;
• Portability
• Easy implementation
• Easy integration with the environment
• Data capacity to manage the storage
3. Analyzing
To find similar user engagement data which can help the recommendation engine to work more accurately requires data filtering. This works through different data analysis approaches. Some of the most suitable data analysis methods are;
1. Real-Time Analysis
Real-time system processes data instantly as it is created, with the help of tools to process and analyze a series of events. Thus, to provide a real-time product recommendation system you require real-time data analysis system.
1. Near Real-Time Analysis
In near real-time analysis the data is collected instantly. But to capture the latest data details, it requires refreshing the analytics frequently. It is a suitable analytics system to recommend products during browsing sessions. Thus to provide a real-time product recommendation engine.
• Batch Analysis
In batch analysis the data is processed at regular intervals or requires an ample amount of data to yield results through analytics. Daily sales volume data can be a suitable example of this.
4. Filtering
Filtering relevant data for user recommendation is the final step of the AI-powered product recommendation engine. The filtering of data can be done through different types of algorithms that we discussed above, namely;
1. Collaborative Filtering Recommendation Engine
2. Content-based Filtering Recommendation Engine
• Network Generation Recommendation Engine
1. Knowledge-Based Recommendation System
How AI Recommendation Algorithm Works in E-Commerce?
A majority of large-scale e-commerce marketplaces use AI-enabled recommendation algorithm, such as Flipkart, Amazon, eBay, Alibaba.
These businesses use all these tactics to analyze customer behavior and preference to suggest the most suitable products in which the customer might be interested.
The product recommendation algorithms are quite a guided business booster for e-commerce services. Their platforms can promote upselling and cross selling of products in an appropriate manner.
It also increases customer engagement and average time spent on the platform. In addition to that, the recommendation engines support reducing cart abandonment frequency and is also an easy way to minimize the bounce rate.
According to Amazon data, it is generating up to 35% of revenue of online stores with its highly effective product recommendation engine. With its effective implementation, Amazon witnessed 5 times increase in recommended products reactions as compared to its previous solutions.
Overall, the AI powered recommendation engine is an intelligent tool that drives revenue growth and increases the customer satisfaction rate.
Conclusion
Looking for a simple and highly effective way to boost your business revenue and customer satisfaction? Adopt AI-enabled product recommendation engine without any second thought.
E-commerce website solutions are integrating an AI-enabled recommendation engine for matching right product and services with the right customer. This is improving shopper’s engagement and conversions tremendously.