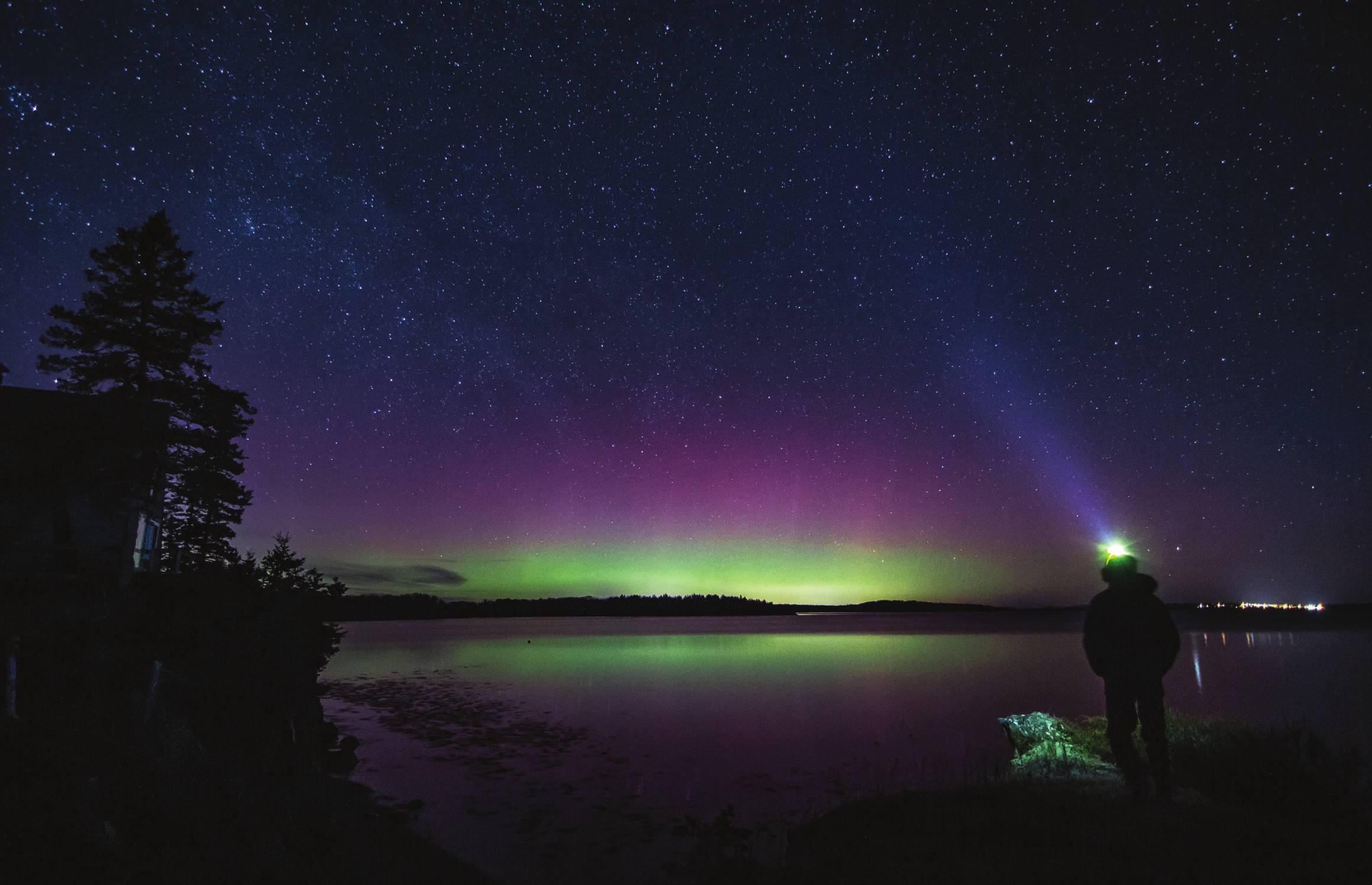
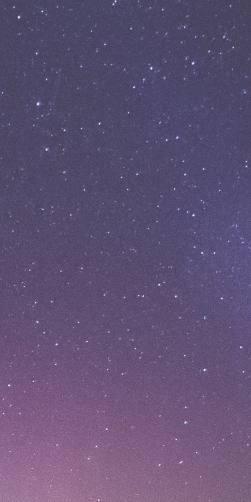

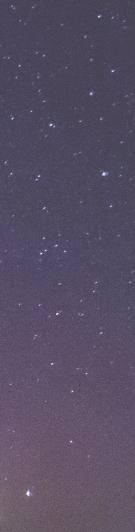

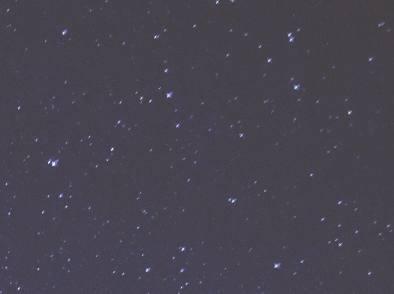
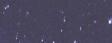
Banks possess such a wealth of customer information that McKinsey referred to data as the “fundamental raw material” of the industr y leaders in Maine are generally interested in exploring how AI could add value to their businesses, they are also exercising caution. Trust is central to a bank’s relationship with customers, so executives are sensitive to concerns about regulator y oversight, fairness, and transparency. “The opportunities are abundant, so we have put rules in place to ensure we invest in AI only in the ways that bring more value to our customers while creating business
Cosby, senior vice president and director of business intelligence at Bangor Savings Bank.
“The dat a it self is so power f ul ,” she s ays, “but we’ve got to do it in the right way and not do harm ” Caution about A I is not unique to Maine. In a recent global sur vey of I T executive s in the banking indus tr y, mos t repor ted that the complexit y and risk s of A I project s out weighed the bene -
Leading consultant s have encouraged banks to become “A I First ” institutions so that they can successfully compete and retain customers Other wise, they risk becoming over taken by competition, espe-
space. Faced with this pressure but al so navigating the A I-powered digital era with caution, banks have sought low-risk ways to optimize their internal processes, such as automating repetitive tasks
At N o r way S av in g s B a n k , t h e b u s in e s s inte lli ge n c e te a m h a s ado pte d Ro b ot ic Pro c e s s Au to m at io n (R PA ) a s a n atu -
r u le s- b ase d to o l s w hich e m u l ate h um a n ac t io n s inter ac t in g w it h d ig it a l s y ste m s m ay b e e spe cia lly we ll- s u ite d fo r -
t i ate s b et we e n “ t r u e A I ” a n d R PA to o l s t h at f o llow a p re ci s e s cript b ui lt by t h e te a m , w h e re t h e re i s n o le a rn in g invo lve d o r a l go rit h m s u s i n g hi s t o ri c a l d a t a fo r d e c i s i o n m a k i n g “ It ’s g o i n g t o d o e x a c t l y w h a t I ’ v e t o l d i t i n t h e s c ri p t t h a t I ’ v e b ui l t , ” e x p l a i n s C hri s t i n e M c C a n n , a s s i st a n t v i c e p r e s i d e n t o f N o r w a y S a v i n g s B a n k . “ It ’s n o t g o i n g t o d o a ny t hi n g m o r e o r a ny th i n g l e s s ”
Nor way Savings uses a vendor RPA tool to per form redundant tasks such as updating many records at a time. “If you were to watch it run, you would actually see the [cursor] moving acros s the page and the keyboard entering the data. It looks just like there’s an invisible person
explains McCann Employees have welcomed the technolog y because it removes monotonous work and frees up their time, a theme we’ve seen echoed acros s other industries in this repor t . McMann sees it as a way to let employees use their brain power. “ We really aren’t taking work away from them We’re freeing them up to focus on the things we want a trained mind to be able to focus on.”
many employee s working in and around Por tland, is a leader in turn-key insurance solutions. When it come s to dis abilit y insurance, the company is similarly
and simple t ask s, allowing employee s to operate in what David Me s singer, director and as sociate actuar y, c all s “ambiguous space s ” There are myriad oppor tunitie s to use A I for ex tracting information from document s or speeding up the claims approval proce s s. “Freeing up and automating away the unambiguous t ask s that highly trained profe s sional s are doing ” is the be s t oppor tunit y for A I in the insurance indus tr y, he s ays “Too many highly-trained profe s sional s spend too long doing thing s that are unambiguous.”
Full scopeR M S, like Nor way Saving s B ank, is intere s ted in exploring ways to automate redundant t ask s to a point , but Me s singer s ays a bot will never f ully replace a claims analys t From a regulator y st andpoint , any decision could theoretic ally be c alled up in front of a
machine told me to’ is simply not good enough,” he s ays.
Similarly, Nor way Saving s is not willing to adopt an A I tool that lack s transparency “ We’re ver y mindf ul about what we let in our house, so to speak,” explains McCann
Even at A I-centered star tups, human analyst s continue to play a critical role. Water ville-based Prospector, which is in star tup accelerator Dirigo Labs’ inaugural class, relies on NLP to extract tabular
document s that mining companies are obligated to release. Their plat form ser ves as a research tool for anyone interested in investing in the mining industr y. According to Jonathan Godbout, Prospector’s chief
machine learning (ML) and natural language processing (NLP) to augment the manual work of an analyst. Allowing employees to focus on reviewing rather than extracting means Prospector, which is interested in moving into other ver tical s beyond mining, can per form content analysis at a previously unimaginable scale, having already analyzed t wo million public disclosure document s.
But even with NLP, there are still clear challenges with text extraction from unstructured document s A s Godbout explains, the industr y has not matured to a
the wheel” and trust a model to synthesize all relevant information without having an analyst review it
embracing A I to suppor t loan or claims pro -
actual decision making. For example, the
and insurance provider Unum built an A I model that has the potential to reduce the approval time for claims When the company s aw an increase in claims during the
over time, the model made a subst antial
vice pre sident of digit al s trateg y and trans formations at Unum Sekharan’s team zeroed in on high-trans action, high-volume, high-velocit y claims (See Four Tips for Succe s s f ul A I Deployment on page 5 0).
Within the same categor y of automating and streamlining redundant internal tasks, both banking and insurance require extensive document s to be received and logged so that customer-facing decisions whether for loans or claims approval s can be made A I can do much of the busy work of compiling, consolidating, and indexing document s. Nor way Savings is in the process of implementing an orchestration system
information accordingly The system can automatically spot risks or trigger a request for a credit check or home appraisal. The tool will aid in the administrative process of gathering and sor ting document s, but as vice president for business intelligence Vick y Libby notes, it will not be used for automatic decision making
G lo b a lly, b an k s ro ut in e ly u s e A I to q u a li f y a p p lic a nt s fo r loa n s u s in g s y s te m at ize d , r u le - b ase d m o d e l s , w h ich f ac to r in cre d it s co re s a n d ot h e r p ub lic ly avai l a b le info rm at io n Po r t l a n d- b ase d p ay me nt s
lever a g in g A I fo r cre d it , f r a ud , a n d key d e ci s io n s acros s W E X , ac co rd in g to R ya n Tay lo r, s e n io r v ic e p re s id e nt of e nte rp ri s e d at a a n d a n a ly t ic s .
been developing complex algorithms that factor in a broader range of unstructured data about a person, including social network activit y How invasive these model s might become has been a source of concern, but regulators have not been sit ting idle Because federal law requires that applicant s who are denied credit be told why, a compliant A I-based credit decision making model must have a cer tain level of transparency and be able to provide an explanation The Consumer Financial Protection Bureau released a memo in early 2022 to remind lending institutions of -
nation if their application for credit was denied ” Lenders cannot blame noncompliance on the fact that their technolog y “is too complicated, too opaque in it s decision-making, or too new,” the agency notedtions like WE X implement explainable A I and formal model governance processes that enable transparency in decisions, explains Taylor.
Ad vo c ate s fo r u s in g A I fo r cre d it d e ci s io n s p o int o u t t h at le n d in g h a s hi s to r ic a lly be e n ri f e w it h di s cr im in at io n
d e s i gn e d to b e m o re e q u it a b le
1: Star t with a targeted use case or proof of concept (POC) to build excitement .
When selecting an initial A I use c ase, dat a teams might choose the low-hanging fruit the easie s t project that provide s the greate s t reward. A logic al place to st ar t might be in high- dat a areas of the company such as the marketing team, which may already have a good repre sent ation of cus tomer dat a and be relatively more sophis tic ated in it s use of analy tic s
Preetha Sekharan, Unum’s head of digit al s trateg y, and her team
to intelligently transcribe document s during the insurance quoting proce s s “Generating excitement
around that POC and the POC
needed,” she explains. “Then we were able to obt ain f unding to st ar t working on sc ale.”
Still , the barriers to A I adoption may be more cultural than technic al . “The technic al barriers have dropped dramatic ally,” explains R yan Taylor, senior vice pre sident of enterprise dat a and analy tic s at WE X “For those that have the c apit al to spend on it , A I c an be built into solutions in a variet y of acce s sible ways.” When thinking about sc aling , advoc ate s of A I mus t appeal to individual s and teams who may not recognize how
winning the heart s and minds of the busine s s teams is to underst and the critic al problems and help demons trate how dat a and A I c an help addre s s those problems
2: Involve all stakeholders in the ‘ test and learn’ process.
To ga in b roa d e m p loye e ac c e pt a n c e of a n ew A I to o l , u s e r s mu s t b e invo lve d f ro m d ay o n e. “ We h a d to en g in e e r h ow we d id thi s p roje c t to m a ke s ure pe o p le we re co mfo r t a b le w it h it , ” s ay s S e k h a r a n U n um a s ke d a n a ly s t s to s p e n d t h re e m o nt h s s tu d y in g t h e o ut p u t of t h e A I to o l a n d thi s a llowe d t h e m
p ro d u c e d h ig h - q u a lit y re s u lt s .
There is a clear challenge here to overcome what David Messinger, director and associate actuar y at Full scopeRMS, call s the “not my day job problem.” How do you involve employees in innovation around A I while allowing time for them to accomplish their day-to-day tasks?
“It ’s incredibly impor tant that the stakeholders are not just a point of contact, but are really integrated through the whole arc of the project,” he says “That ’s a huge time commitment on a speculative project that is, in realit y, not their day job. But nothing that I’ve seen has been more crucial to success.”
Th e B u s in e s s Inte lli ge nc e te a m at
B a n go r S av in g s B an k wo rke d w it h
t h e In s t itu te fo r E x p e rie nt ia l A I a n d
t h e Ro u x In s t itu te at N o r t h e a s te r n U n i ve r s it y to b ui ld a m ach in e le a rnin g m o d e l t h at h e lps t h e b a n k ’s
i s like ly to b e inte re s te d in . Th ey t h e n c a n u s e t h at k n ow le d ge to -
s at io n w it h t h e goa l of be com in g a t ru s te d a dv i s o r D urin g t h e m o d e l d eve lo p me nt , B a n go r S av in g s B an k ’s a n a ly t ic s , en g in e e rin g , a n d b u s in e s s te a m s m et f re qu e nt ly w it h
t h e Ro u x te a m to p rov id e g u id a n c e a ro un d t h e m o d e l’s in p u t s a n d fe a -
ture s Th ey a l s o c o n d u c te d f o cu s -
st a n d t h e co ntex t in w h ich t h e to o l
wo u ld b e u se d a n d get f ee db ack
f ro m u s e r s o n w h ich fe ature s wo u ld
b e m os t h e lpf u l .
Involving st akeholders in the model build al so means it is more likely the model will be integrated into the
current infras tructure, as opposed to operating in a silo. However brilliant an A I solution, if de signed to operate out side an organization’s primar y s ys tems and inter f ace, chance s are it will not be used.
3: Nur ture computational subject mat ter exper t s.
“The word ‘comput ational’ is now in front of ever y subject ,” explains Venkat Srinivas an, founder and managing director of Innospark Venture Capit al . In the f uture, he explains, “ there will be no special purpose A I modelers– dat a scientis t s as we c all them today Ever y subject mat ter exper t will al so be a dat a scientis t ” Today, however, that may be easier s aid than done.
talent with that optimal mix of domain and data skill s remains a missing piece: “You need people who understand data science ver y closely. But they al so really need to understand the business problems and the regulator y environment we’re operating in ” The proliferation of out-of-the-box A I tool s means that
subject mat ter expert s, whether in can leverage their knowledge to deliver more valuable data outcomes compared to a data scientist without industr y experience.
“The inve s tment in the groundwork is s till well behind,” Me s singer note s.
“For many insurance companie s, jus t s aying , ‘ We’ve digitized all our policy contract s into PDFs feel s like a victor y ”
Indeed, technolog y s ys tems at are built for s t abilit y They perform core operations well but al so require ex tensive maintenance
with “legacy c apabilit y ” in mind, and dat a might be siloed acros s multiple busine s s unit s.
C o n so lid at in g d at a b et we e n p rev iou s ly s i loe d te a m s into cros s- o r ga niz at io n a l d at a s y ste m s i s o n e co m m o n a pp ro ach to ach iev in g A I re a d in e s s Th e s e c e nt r a lize d m a n a gem e nt s y s te m s fo rm a “d at a b ack b o n e , ” p rov id in g ac c e s s to d at a in a t im e ly, se cure , a n d ro le - a pp ro p ri ate way.