From Biological Aging Clocks to Longevity Medicine: An Artificial Intelligence Odyssey
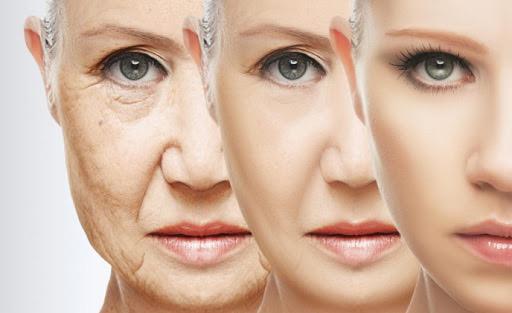
Ramkumar Hariharan
Data Science Engineering Faculty,


Program Director and Senior Scientist
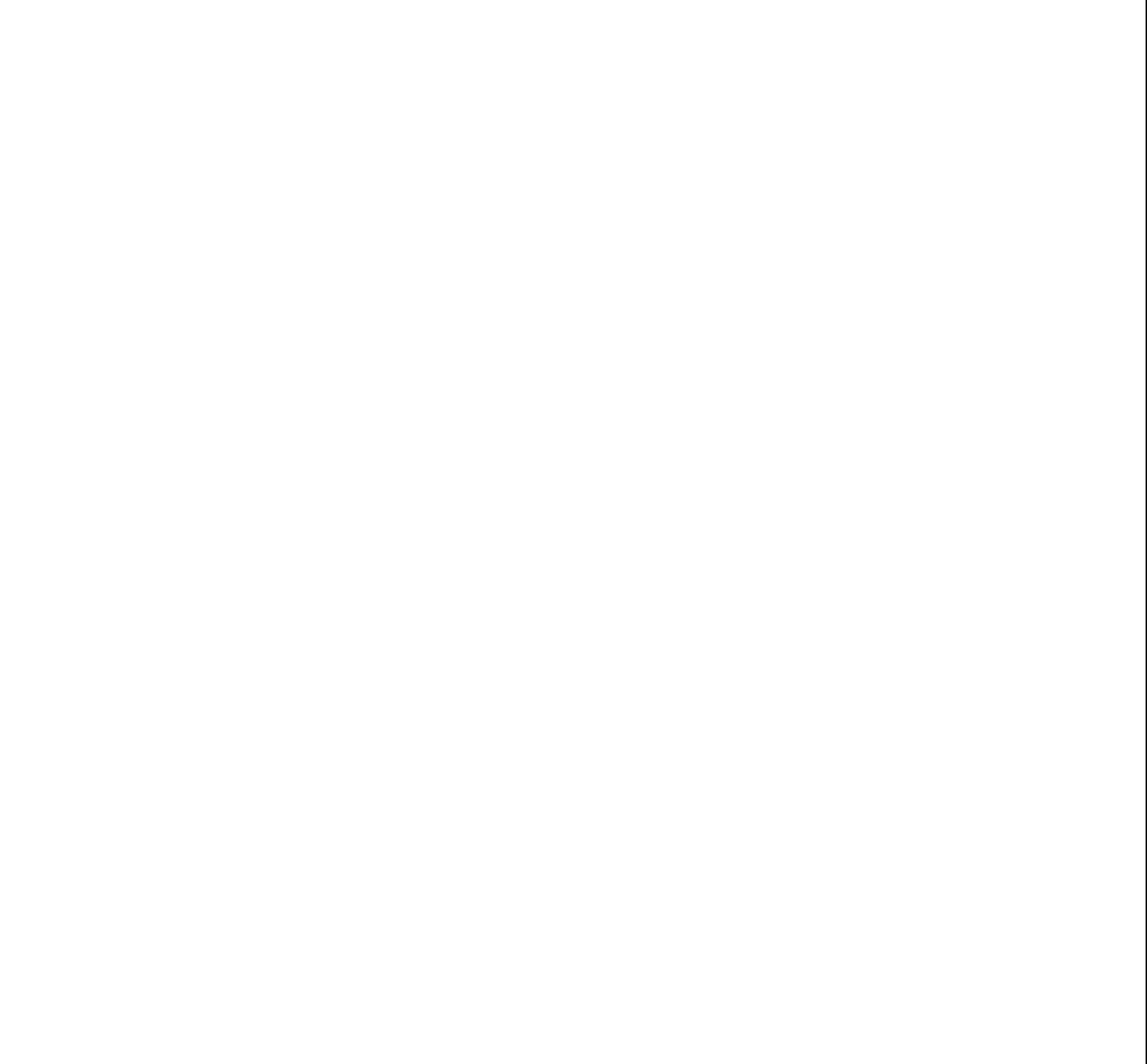
Ramkumar Hariharan
Data Science Engineering Faculty,
Program Director and Senior Scientist
Immune Function
Liver Function
Kidney Function
Cognition
Cancer
AD
CVD
Infections
Source : Seals DR & Melov S, 2014, Aging
What will it take to live longer (No pun intended) ?
Fever
Source: Harvard health, unsplash,Yale medicine,
Cardiovascular health
Lung function, Cardio health
Diabetes
Aging
Epigenome sits on the genome & Directs how information in decoded
Source: cuinnedits.com, frontiersin.org
353 CpG (features)
Elasticnet Regression (Lasso + Ridge) -> ~ 21,000 CpG (features) ->
Error 3.6 yrs
DNAm age Vs. Chrono age corr = 0.97
Total N ~ 8000 from 82
”normal” datasets
• Train = 40, Valid = 30
• 51 cell types & tissues
• Cancers DNAm +
• iPSCs DNAm = 0 • Breast Tissue DNAm +
We
Rooted in biology !!!
need multi-omic, causal clocks
• Carlos López-Otín et al, 2013
Hallmarks of Aging
• Carlos López-Otín et al, 2023
Hallmarks of Aging: An expanding Universe
Source: Carlos López-Otín et al, 2023
Clinical trials for first generation longevity medicine
• Pick one or more gold standard drugs
• Validate them on aging clock
• Build causal aging clocks
Ricardo–Baeza Yates, Professor of the Practice
Usama Fayyad, Executive. Director
Pramod Nagare, Senior Data Engineer
Many others….
Jaren Walker, Director of Partnerships, Seattle
Dave Thurman, Dean and CEO, Seattle
Senior Leadership team @ Boston campus