AI, IoT, and Machine Learning Fueling Predictive Maintenance Market Growth
The global predictive maintenance market is set to grow from US$ 12.3 billion in 2025 to US$ 50.3 billion by 2032, expanding at a CAGR of 22.2%. This growth is fueled by the increasing adoption of AI, machine learning, and IoT technologies to optimize asset utilization and reduce downtime. Key drivers include the integration of digital twin technology, real-time equipment monitoring, and advancements in sensor and analytics software. North America is predicted to dominate the market, with large enterprises leading the way due to their vast asset portfolios and operational capacity.
The Asia Pacific region is also expected to see rapid growth, driven by industrialization in emerging markets. With the rise of Industry 4.0 and smart manufacturing, predictive maintenance solutions are becoming more accessible, offering significant cost savings and efficiency improvements across sectors such as manufacturing, energy, and aviation.
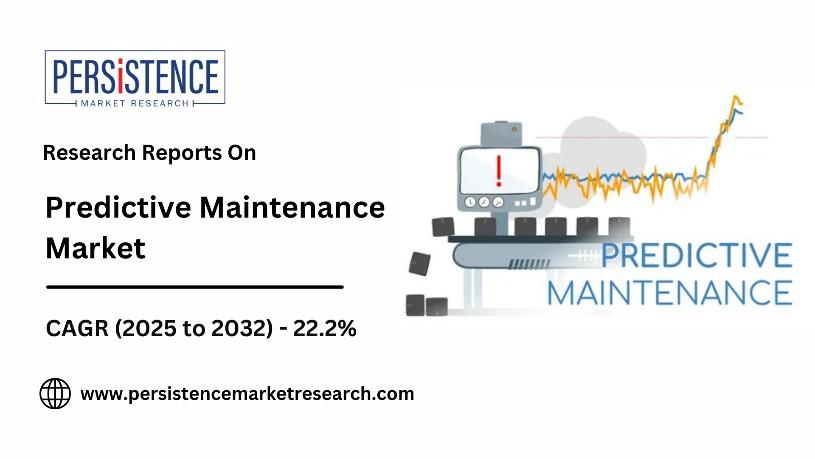
The integration of Artificial Intelligence (AI), the Internet of Things (IoT), and Machine Learning (ML) is transforming industries globally. These technologies are revolutionizing predictive maintenance, enhancing operational efficiency, reducing downtime, and improving safety across various sectors. Predictive maintenance is fast becoming a cornerstone of modern industrial practices, empowering businesses to predict and address equipment failures before they happen, minimizing costs, and optimizing the lifespan of machinery. The market for predictive maintenance is growing rapidly, driven by technological advancements and the increasing demand for cost-effective solutions. This article explores the factors driving this growth, the benefits of predictive maintenance, and how AI, IoT, and ML are playing a crucial role in shaping the future of this industry.
The Role of AI in Predictive Maintenance
AI has significantly contributed to the predictive maintenance market by enabling automated, realtime decision-making and advanced analytics. The integration of AI allows systems to learn from past maintenance data, identify patterns, and predict potential failures more accurately than traditional methods. AI algorithms are designed to handle large volumes of sensor data and use it to recognize subtle changes in operational behavior. For example, AI-powered systems can analyze temperature fluctuations, vibration patterns, and other sensor data from machinery to detect anomalies that may signal an impending failure.
AI is not only enhancing the precision of predictive maintenance but also automating decisionmaking processes that would otherwise require human intervention. Predictive algorithms analyze data faster, making it easier to pinpoint specific issues and provide actionable insights. The
automation provided by AI also reduces the reliance on human expertise, resulting in greater efficiency and accuracy in predicting maintenance needs.
How IoT is Revolutionizing Predictive Maintenance
The Internet of Things (IoT) is another key driver of growth in the predictive maintenance market. IoT connects machines, devices, and sensors to a central network, enabling real-time data collection and monitoring of equipment performance. With IoT-enabled devices, companies can continuously monitor equipment health and detect potential issues as they arise. This constant flow of data is invaluable for predictive maintenance systems, as it provides up-to-date information on machinery status, which can be analyzed for patterns indicating wear and tear.
IoT devices provide the raw data needed to train AI and machine learning models for predictive maintenance. They can track various parameters like temperature, pressure, humidity, vibration, and sound to detect early warning signs of failure. IoT networks allow businesses to remotely monitor equipment and intervene before failures occur, reducing costly downtime and emergency repairs. The synergy between IoT and predictive maintenance technologies offers a proactive approach to managing equipment and preventing unnecessary disruptions in operations.
The Role of Machine Learning in Predictive Maintenance
Machine Learning (ML), a subset of AI, plays a vital role in predictive maintenance by improving the accuracy and effectiveness of predictive models. Machine learning algorithms are designed to learn from historical data, including equipment performance data, failure records, and maintenance logs. Over time, these algorithms improve their predictive capabilities, recognizing patterns that might not be immediately obvious to human operators.
The use of machine learning in predictive maintenance enables continuous improvement of predictive models as new data is fed into the system. As more data is collected, machine learning models evolve and become better at forecasting equipment failures with greater precision. This data-driven approach reduces human error and minimizes the risk of unexpected breakdowns. For example, ML models can predict when a specific part or component of machinery is likely to fail, enabling operators to schedule maintenance proactively and prevent unplanned downtime.
Cost Reduction and Operational Efficiency
One of the most significant benefits of predictive maintenance is cost reduction. Traditional maintenance strategies, such as reactive maintenance (fixing things after they break) or scheduled maintenance (replacing parts at fixed intervals), can be costly and inefficient. Predictive maintenance, however, helps businesses minimize unnecessary repairs and avoid the high costs associated with equipment failure.
By forecasting potential failures before they happen, predictive maintenance allows companies to optimize their maintenance schedules, reducing the frequency of unnecessary interventions. Businesses can also avoid the cost of emergency repairs, which can be significantly more expensive than planned maintenance. Furthermore, predictive maintenance helps extend the lifespan of equipment by identifying problems early and addressing them before they cause major damage. This prolongs the life of machinery, improving the overall return on investment.
The integration of AI, IoT, and machine learning also boosts operational efficiency by enabling continuous monitoring and analysis of equipment performance. Real-time data collection ensures that businesses can react quickly to any issues, ensuring that operations run smoothly. The reduced
downtime associated with predictive maintenance also contributes to higher productivity, as equipment is more reliable and less prone to sudden failures.
Enhancing Safety and Risk Management
Predictive maintenance also plays a crucial role in improving workplace safety. In industries where machinery failure can lead to hazardous situations, such as manufacturing, oil and gas, and mining, predictive maintenance systems help mitigate risks by preventing sudden breakdowns. By identifying potential issues early, predictive maintenance allows businesses to take corrective actions before equipment failures compromise safety.
For instance, in the energy sector, predictive maintenance can be used to monitor critical components of power plants, such as turbines and generators. The ability to predict when these components might fail can help prevent catastrophic events, such as explosions or fires, which can result in significant loss of life and property. In addition, predictive maintenance helps organizations comply with safety regulations and reduce the likelihood of costly accidents or insurance claims.
Industry Applications of Predictive Maintenance
Predictive maintenance has applications across a variety of industries. In manufacturing, for example, it is used to monitor assembly lines, robotics, and automated systems to ensure they operate efficiently and prevent costly downtime. In transportation, predictive maintenance is used to monitor the health of vehicles, airplanes, and trains, allowing companies to schedule maintenance based on the condition of the equipment rather than a set time interval.
In the energy sector, predictive maintenance is critical for ensuring the reliability of power grids, turbines, and generators. In healthcare, medical equipment such as MRI machines, ventilators, and infusion pumps benefit from predictive maintenance, reducing the risk of malfunctions that could affect patient care. Similarly, in the aviation industry, airlines use predictive maintenance to monitor aircraft components and ensure safe, timely operations.
The Future of Predictive Maintenance: Trends and Innovations
The future of predictive maintenance is bright, as advancements in AI, IoT, and machine learning continue to drive growth in the market. As technology evolves, predictive maintenance systems will become even more accurate, autonomous, and integrated across industries. One of the key trends to watch is the increasing use of edge computing, which involves processing data closer to the source (such as on the machinery itself) to improve real-time decision-making.
Additionally, the integration of digital twins (virtual replicas of physical assets) with predictive maintenance systems will allow businesses to simulate and test various scenarios, providing even more accurate predictions and insights. With the continued growth of 5G networks, predictive maintenance solutions will become more scalable and accessible, especially for industries that require real-time data processing.
The increasing adoption of AI, IoT, and machine learning in predictive maintenance will also lead to the development of more sophisticated tools and platforms. These innovations will further streamline operations, enhance safety, and reduce costs, contributing to the overall growth of the market.
Conclusion:
A Growing Market with Vast Potential
AI, IoT, and machine learning are undoubtedly transforming predictive maintenance and propelling market growth. As industries continue to adopt these technologies, the demand for advanced predictive maintenance solutions will only increase. Companies that leverage these innovations will benefit from reduced downtime, lower operational costs, and improved safety. With the continued evolution of AI and IoT, predictive maintenance is poised to become an integral part of the future industrial landscape.