TheConsistencybetweenCross-Entropyand DistanceMeasuresinFuzzySets
YamengWang,HanYangandKeyunQin*
CollegeofMathematics,SouthwestJiaotongUniversity,Chengdu610031,China; yamengwang@aliyun.com(Y.W.);yanghan@163.net(H.Y.)
* Correspondence:keyunqin@263.net.
Received:14February2019;Accepted:12March2019;Published:16March2019
Abstract: Theprocessingofuncertaininformationisincreasinglybecomingahottopicintheartificial intelligencefield,andtheinformationmeasuresofuncertaintyinformationprocessingarealso becomingofimportance.Intheprocessofdecision-making,decision-makersmakedecisionsmostly accordingtoinformationmeasuressuchassimilarity,distance,entropy,andcross-entropyinorderto choosethebestone.However,wefoundthatmanyresearchersapplycross-entropytomulti-attribute decision-makingaccordingtotheminimumprinciple,whichisinaccordancewiththeprinciple ofdistancemeasures.Thus,amongallthechoices,wefinallychosetheonewiththesmallest cross-entropy(distance)fromtheidealone.However,therelationbetweencross-entropyand distancemeasuresinfuzzysetsorneutrosophicsetshasnotyetbeenverified.Inthispaper,wemainly considertherelationbetweenthediscriminationmeasureoffuzzysetsanddistancemeasures,where wefoundthatthefuzzydiscriminationsatisfiedalltheconditionsofdistancemeasure;thatisto say,thefuzzydiscriminationwasfoundtobeconsistentwithdistancemeasures.Wealsofound thatthecross-entropy,whichimprovedwhenitwasbasedonthefuzzydiscrimination,satisfied alltheconditionsofdistancemeasure,andwefinallyprovedthatcross-entropy,includingfuzzy cross-entropyandneutrosophiccross-entropy,wasalsoadistancemeasure.
Keywords: discrimination;cross-entropy;distancemeasure;consistency
1.Introduction
Intherealworld,thereexistsmuchuncertain,imprecise,andincompleteinformation,meaning thattherearealsomanytoolstosettlethem.Zadeh [1] firstproposedtheconceptofafuzzy set,which,definedbyamembershipfunction,isusedtodepictthemembershipvalueofone objecttoaset.Atanassov [2] proposedanintuitionisticfuzzyset(IFS)describedbytwofunctions, includingamembershipfunctiondepictingthemembershipvalue,andanon-membershipfunction depictingthenon-membershipvalueofoneobjecttotheintuitionisticfuzzyset.Theintuitionistic fuzzysetistheextensionofafuzzysetthroughaddinganon-membershipfunction.Ithas providedamoreflexiblemathematicalframeworktoprocessuncertainty,imprecise,andincomplete information. Smarandache[3] firstlyproposedthenotionofneutrosophyandaneutrosophicsetin 1998.Theneutrosophicsetisdefinedbythetruth-membershipfunction,indeterminacy-membership function,andthefalsity-membershipfunction,anditiscomprisedofamembershipvalue, non-membershipvalue,andindeterminacy-membershipvalue.Theneutrosophicsetstheoryhas beensuccessfullyappliedintheimage-processingfieldbyVlachosandSergiadis [4].Therefore,Wang etal. [5] putforwardthedefinitionofasingle-valueneutrosophicset(SVNS)andsomeoperations forbetterapplicationinrealscientificandengineeringfields.Asinglevalueneutrosophicsetisthe extensionoffuzzyset,andtheSVNStheoryalsoprovidesuswithamoreconvenienttoolforthe uncertaininformationprocessing.Recently,someresearchershavedevotedthemselvestothestudyof
Symmetry 2019, 11,386;doi:10.3390/sym11030386 www.mdpi.com/journal/symmetry
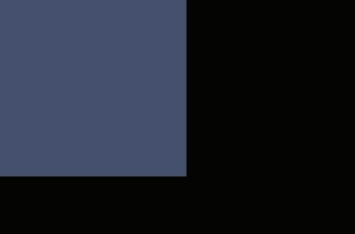
thesingle-valueneutrosophicsettheoryanditsapplications,andachievedsomesuccessfulresults insomefields. Zhangetal.[6–10] didalotofresearchaboutneutrosophicsets,andproposedanew kindofinclusionrelationandnewoperationsinSVNSs;furthermore,theyalsodiscussedthealgebraic structureandsomeapplicationsinalgebraicsystems.
Informationmeasuresareessentialtodecision-makingininformationprocessing,including thesimilarityfunction,distanceordivergencefunction,andentropyandcross-entropy.These informationmeasuresarewidelyapplicableinthingslikeimageprocessing,clustering,andpattern recognition.Liuetal. [11] appliedasingle-valueneutrosophicnumbertotheDecision-Making TrialandEvaluationLaboratoryMethod,andconsequentlypresentedtheSVNN-DEMATEL(single valueneutrosophicnumber-Decision-makingTrialandEvaluationLaboratoryMethod)model. Mukhametzyanovetal.[12] providedananalysisaboutsomemulti-criteriadecision-making(MDM) methodsandthefinalselection,andpresentedaresultconsistencyevaluationmodel. Tuetal.[13] introducedsomesimplifiedneutrosophicsymmetrymeasuresandappliedittotheirdecision-making. Thesimilarityfunctionismainlyusedtomeasurethelevelofsimilaritybetweentwoobjects.Entropy isusuallytodepictthedegreeofuncertaintyofoneobject,andisveryimportantformeasuring uncertaininformation.Cross-entropycandepictthediscriminationdegreeoftwoobjects,andwecan judgetheirrelationfromit.Therefore,cross-entropyhasmanyapplicationsininformationmeasures, decision-making,patternrecognition,andsoon.Zadeh[14]firstlyproposedtheentropyoffuzzy eventsbasedonShannonentropy.Kullback [15] wasconcernedwithaninformationmeasurewhichis knownas“distance”or“divergence”,depictingtherelationshipbetweentwoprobabilitydistributions. Therefore,itcanserveasaninformationmeasurewhichcanindicatethedegreeofdiscrimination. Furthermore,anewkindofinformationmeasurecalledthe“cross-entropydistance”oftwoprobability distributionswasintroducedbyKullback.DeLucaandTermini [16] introducedthenotionoffuzzy entropyandsomeaxiomstoexpressthefuzzinessdegreeofafuzzyset,accordingtoShannon’s function.Then,thefuzzyentropywasgeneralizedtotheinterval-valuedfuzzysetsandintuitionistic fuzzysetsbyBurilloandBustince [17] Szmidt[18]defined intuitionisticfuzzyentropyinanewway. Weietal.[19] proposedinterval-valuedintuitionisticfuzzyentropy.Cross-entropycanbeusedto depictthedegreeofdiscriminationbetweentwoobjects.Therefore,manyresearchershavemodified cross-entropymeasures.Forexample,Lin [20] proposeddivergencebasedonShannonentropy, anditisatypeofmodifiedfuzzycross-entropy.Bhandari [21] introducedfuzzydivergencebetween twofuzzysets. Shangetal.[22] putforwardfuzzycross-entropyandasymmetricdiscrimination measure,whichwasimprovedbyfuzzydivergenceandcanbeusedtodescribethediscrimination degreebetweentwofuzzysets. VlachosandSergiadis[4] presentedintuitionisticfuzzycross-entropy, andalsofoundaconnectionbetweenfuzzyentropyandintuitionisticfuzzyentropyintermsof fuzzinessandintuitionism.Verma[23,24]introducedthedivergencemeasure,whichisaninformation measurethatcandepictthediscriminationdegree.Cross-entropymeasuresweregeneralizedto single-valuedneutrosophicsetsandappliedtomulti-criteriadecision-makingbyYe [25].Sincethen, ¸Shahin[26] hascontinuedtogeneralizethecross-entropymeasuretointervalneutrosophicsets,and introduceditsapplicationinmulti-criteriadecision-making.
Inourstudy,wefoundthatthefuzzydiscriminationproposedbyBhandari[21]andimproved fuzzycross-entropybyShang[22],aswellasneutrosophiccross-entropyintroducedbyYe [25] all havesimilarpropertieswithdistancemeasures,suchasnon-negativity,symmetry,andwhenthe cross-entropy(distance)betweentwofuzzysetsis0,ifandonlyifthetwofuzzysetsarecompletely equal.Furthermore,thedecisionprincipleofcross-entropyanddistanceappliedindecision-making arealsothesame.Thatistosay,intheprocessofdecision-making,amongallthechoices,wefinally chosetheonewiththesmallestcross-entropy(distance)fromtheidealone.Basedontheabove analysis,wetendedtostudytheirrelationshipsbetweencross-entropyanddistancemeasure.There hasbeennopreviousresearchabouttheirrelationshipsbetweencross-entropyanddistance.So, wemainlyprovedthatthefuzzydiscriminationandimprovedfuzzycross-entropyandneutrosophic cross-entropybasedondiscriminationaredistancemeasuresinfact.Wealsopresentalltheproof
aboutfuzzydiscriminationandimprovedfuzzycross-entropyandneutrosophiccross-entropybased ondiscriminationsatisfyingalltheconditionsofdistanceinthepaper.InSection 2,wemainly introducesomerelevantknowledge,andprovideproofthatthefuzzydiscriminationmeasuresatisfies alltheconditionsofadistancemeasure,i.e.,itisactuallyakindofdistancemeasure.InSection 3, wemainlyprovethatthefuzzycross-entropysatisfiesalltheconditionsofadistancemeasure,and thatcross-entropyinsingle-valueneutrosophicsetsisalsoakindofdistance;thatistosay,thatthe cross-entropymeasureisconsistentwithdistancemeasures.
2.FuzzyDiscriminationIsConsistentwithDistanceMeasure
Let X beauniversecourse,andafuzzyset A isdenotedbyamembershipfunction µ A (x) which isusedtoexpressthedegreeofbelongingnessofonepoint x ∈ X totheset A,andforall x ∈ X, µ A (x) ∈ [0,1].When µ A (x)= 0or µ A (x)= 1,then A becomesacrispset. Definition1 ([1]). LetXbeauniversecourse,andfuzzyset AdefinedonXbegivenas: A = { x, µ A (x) | x ∈ X} where µ A : X → [0,1],andeverypointhasamembershipvaluetoexpressthedegreeofbelongingnesstoaset.
Let FS(X) bethesetofallthefuzzysets.Thefollowingaresomepropertiesoffuzzysets: M, N, T ∈ FS(X), (1) if M ⊆ N,then µM (x) ≤ µN (x); (2) if M ⊆ N, N ⊆ M,then M = N; (3) if M ⊆ N, N ⊆ T,then M ⊆ T
Definition2. Bhandari [21] proposedthefuzzydiscriminationmeasurewhichisusedtoexpressa discriminationdegreeinfavorofAagainstB.A, B ∈ FS(X) aredefinedasfollows: I(M, N)= n ∑ i=1 (µM (xi ) ln µM (xi ) µN (xi ) +(1 µM (xi )) ln 1 µM (xi ) 1 µN (xi )
Itisobviousthat I(M, N) ≥ 0 I(M, N)= 0 if,andonlyif M = N.Bhandari [21] alsodefined E(M, N)= I(M, N)+ I(N, M) forsymmetry.
Fromtheabove,thefuzzydiscriminationhassimilarproperties,suchasdistancemeasures (exceptforoneaxiomofdistancemeasure),andtheyalsohaveasimilarprinciplecorrespondingto theprincipleofminimumcross-entropy.Inotherwords,thereexistsaperfectsolution, A,butbecause itisgenerallyunlikelytoexistinarealsituation,weshouldaimtofindasolutionwhichislikelyto existintherealworlddenotedby B, C,...,sothatwecangettheircross-entropyto A.Weendedup choosingthesmallestcross-entropy,andthesolutioncorrespondingtothesmallestcross-entropywas theoptimalsolution.Thedistancemeasurealsohadthesameprincipleasthecross-entropy.
Definition3. Afunction D : FS(X) × FS(X) → [0,1] wasnamedasthedistancemeasureon FS(X) sothat thefollowingconditions[10]couldbesatisfied,andA, B ∈ FS(X),
(1) 0 ≤ D(A, B) ≤ 1;
(2) D(A, B)= 0,ifandonlyifA = B;
(3) D(A, B)= D(B, A);
(4) ifA ⊆ B ⊆ C,thenD(A, C) ≥ D(A, B), D(A, C) ≥ D(B, C)
Weredefinedthefuzzydistancemeasureasfollows,consideringtheinfinityofthediscrimination measure:
Definition4. Afunction FD : FS(X) × FS(X) → [0, ∞) wasnamedasthefuzzydistancemeasuresothat thefollowingconditionsweresatisfied,andA, B ∈ FS(X),
(1) FD(A, B) ≥ 0;
(2) FD(A, B)= 0,ifandonlyifA = B;
(3) FD(A, B)= FD(B, A);
(4) ifA ⊆ B ⊆ C,thenFD(A, C) ≥ FD(A, B), FD(A, C) ≥ FD(B, C)
Itisobviousthatthesymmetryfuzzydiscriminationhassatisfiedthefirstthreeconditionsof fuzzydistance:
(1) E(M, N) ≥ 0
(2) E(M, N)= 0,ifandonlyif M = N;
(3) E(M, N)= E(N, M)
Thus,wejustneededtoverifythatthefuzzydiscriminationsatisfiedcondition(4)ofthefuzzy distancemeasure.
Theorem1. Letm, n, tbethreenumbersin [0,1].Ifm ≤ n ≤ t,thenE(m, t) ≥ E(m, n), E(m, t) ≥ E(n, t).
Proof. Weprovided0ln0 = 0, m 0 = 0.
E(m, n)= I(m, n)+ I(n, m)=(m n) ln( m n )+(n m) ln( 1 m 1 n ) (1)
E(n, t)= I(n, t)+ I(t, n)=(n t) ln( n t )+(t n) ln( 1 n 1 t ) (2)
E(m, t)= I(m, t)+ I(t, m)=(m t) ln( m t )+(t m) ln( 1 m 1 t ) (3)
Firstly,weneededtoprovethat (3) ≥ (1)
(3)= ln( m t )m t + ln( 1 m 1 t )t m = ln[( m t )m t ( 1 m 1 t )t m ]
(1)= ln[( m n )m n ( 1 m 1 n )n m ]
(3) (1)= ln[( m t )m t ( 1 m 1 t )t m ( n m )m n ( 1 n 1 m )n m ]
Let f (t)=( m t )m t ( 1 m 1 t )t m ( n m )m n ( 1 n 1 m )n m ]
A =( n m )m n ( 1 n 1 m )n m , B =( m t )m t , C =( 1 m 1 t )t m
Then, f (t)= A ∗ B ∗ C, f (t)= A ∗ (B ∗ C + B ∗ C ) ∂B ∂t =(e(m t) ln m t ) = e(m t) ln( m t ) ∗ ( ln( m t ) m t t )= B ∗ ( ln( m t ) m t t )
∂C ∂t =(e(t m) ln( 1 m 1 t ) ) = C ∗ (ln( 1 m 1 t )+ t m 1 t )
f (t)= A ∗ B ∗ C ∗ ( ln( m t ) m t t + ln( 1 m 1 t )+ t m 1 t )
Itisobviousthat A, B, C ≥ 0.
Since0 ≤ m ≤ n ≤ t,then m t ≤ 1,ln( m t ) ≤ 0, 1 m 1 t ≥ 1,ln( 1 m 1 t ) ≥ 0.Thatis, f (t) ≥ 0.
When n = t, f (t)= f (n)= 1.When t > n, f (t) > f (n)= 1,thenln f (t) ≥ 0. Thatistosay,theoriginal (3) (1) ≥ 0,i.e., E(m, t) ≥ E(m, n) hasbeenobtained. Fromhere,wecontinuetoprovethat (3) ≥ (2).
(2)= ln[( n t )n t ( 1 n 1 t )t n ]
(3) (2)= ln[( m t )m t ( 1 m 1 t )t m ( t n )n t ( 1 t 1 n )t n ]
Let g(m)=( m t )m t ( 1 m 1 t )t m ( t n )n t ( 1 t 1 n )t n
D =( t n )n t ( 1 t 1 n )t n , B =( m t )m t , C =( 1 m 1 t )t m
Then, g(m)= D ∗ B ∗ C, g (m)= D ∗ (B ∗ C + B ∗ C ) ∂B ∂m =(e(m t) ln( m t ) ) = B ∗ (ln( m t )+ m t t )
∂C ∂m =(e(t m) ln( 1 m 1 t ) ) = E ∗ ( ln( 1 m 1 t )+ m t 1 m ) g (z)= D ∗ B ∗ C ∗ (ln( m t )+ m t t ln( 1 m 1 t )+ m t 1 m )
Itisobviousthat D ≥ 0. Since0 ≤ m ≤ n ≤ t,then m t ≤ 1,ln( m t ) ≤ 0, 1 m 1 t ≥ 1,ln( 1 m 1 t ) ≥ 0.Thatis, g (m) ≤ 0. When m = n, g(m)= g(n)= 1,then m < n,andwhen g(m) > g(n)= 1,thenln g(m) ≥ 0. Thatistosay,theoriginal (3) (2) ≥ 0,i.e., E(m, t) ≥ E(n, t) hasbeenobtained. Finally,weobtainedtheproofofthetheorem.
Theorem2. Let X beauniversecourse, M, N, T ∈ FS(X).If M ⊆ N ⊆ T,then E(M, T) ≥ E(M, N), E(M, T) ≥ E(N, T).
E(M, N)= I(M, N)+ I(N, M)= ∑n i=1(µM (xi ) µN (xi )) ln µM (xi ) µN (xi ) +(µN (xi ) µM (xi )) ln 1 µM (xi ) 1 µN (xi ) (4) E(N, T)= I(N, T)+ I(T, N)= ∑n i=1(µN (xi ) µT (xi )) ln µN (xi ) µT (xi ) +(µT (xi ) µN (xi )) ln 1 µN (xi ) 1 µT (xi ) (5) E(M, T)= I(M, T)+ I(T, M)= ∑n i=1(µM (xi ) µT (xi )) ln µM (xi ) µT (xi ) +(µT (xi ) µM (xi )) ln 1 µM (xi ) 1 µT (xi ) (6)
Fromhere,weneedtoprovethat (6) ≥ (4) and (6) ≥ (5)
Since M ⊆ N ⊆ T,then µM (xi ) ≤ µN (xi ) ≤ µT (xi ),and µ(xi ) ∈ [0,1], ∀xi ∈ X
FromTheorem1,weknowthattheTheoremhasbeensatisfiedtoeverysinglemembershipvalue, meaningthattheproofcanbeeasilyobtainedfromTheorem1.
Example1. Let X beaspaceoftheuniversecourse M, N, T ∈ FS(X),where M = { x,0.5 |x ∈ X}, N = { x,0.7 |x ∈ X}, T = { x,0.9 |x ∈ X}.Clearly, M ⊆ N ⊆ T,andwecanget E(M, N)= 0.1695, E(N, T)= 0.2700, E(M, T)= 0.8789;thatis,E(M, T) ≥ E(M, N), E(M, T) ≥ E(N, T)
Theorem3. Theabove-definedsymmetryfuzzydiscriminationisadistancemeasure.
Finally,theaboveprovesthatthesymmetryfuzzydiscrimination,definedbyDefinition2,is consistentwiththedistancemeasurefromtheabovetheorems.
3.FuzzyCross-EntropyIsConsistentwithDistanceMeasure
BhandariandPalpointedoutthatthefuzzydiscriminationhasadefect—when µB (xi ) approaches 0 or 1,itsvaluewillbeinfinity.Thus,ithasbeenmodifiedonthebasisofdirecteddivergenceproposed byLin[20],andalsomodifiedbyShangetal.[22]asfollows:
Definition5 ([22]). LetM, N ∈ FS(X),wherewecandefineafuzzycross-entropyas:
I2(M, N)= n ∑ i=1 (µM (xi ) ln( µM (xi ) 1/2(µM (xi )+ µN (xi )) )+(1 µM (xi )) ln( 1 µM (xi ) 1 1/2(µM (xi )+ µN (xi )) )
Thisshowsthatitiswell-definedandindependentofeveryvalueof µ(xi ),whichcanexpressthe discriminationdegreeofAfromB.
Italsohasthesamepropertiesastheabovediscriminationmeasures,inthatwhen I2(M, N) ≥ 0, I2(M, N)= 0if,andonlyif M = N. Let E2(M, N)= I2(M, N)+ I2(N, M);then,symmetryissatisfied.Thus,wemainlyconsiderhow theabove-definedfuzzycross-entropy E2(M, N) satisfiesCondition(4)ofthedistancemeasure. E2(M, N)= n ∑ i=1 µM(xi) ln( µM(xi) 1/2(µM(xi)+ µN (xi)) )+(1 µM(xi)) ln( 1 µM(xi) 1 1/2(µM(xi)+ µN (xi)) ) + n ∑ i=1 µN (xi) ln( µN (xi) 1/2(µM(xi)+ µN (xi)) )+(1 µN (xi)) ln( 1 µN (xi) 1 1/2(µM(xi)+ µN (xi)) ) (7)
Theorem4. Let m, n, t bethreenumbersin [0,1].If m ≤ n ≤ t,then E2(m, t) ≥ E2(m, n), E2(m, t) ≥ E2(n, t).
Proof.
E2(m, n)=I2(m, n)+ I2(n, m)= m ln( m 1/2(m + n) )+(1 m) ln( 1 m 1 1/2(m + n) )+ n ln( n 1/2(m + n) )+(1 n) ln( 1 n 1 1/2(m + n) ) (8)
E2(n, t)=I2(n, t)+ I2(t, n)= n ln( n 1/2(n + t) )+(1 n) ln( 1 n 1 1/2(n + t) )+ t ln( t 1/2(n + t) )+(1 t) ln( 1 t 1 1/2(n + t) ) (9)
E2(m, t)=I2(m, t)+ I2(t, m)= m ln( m 1/2(m + t) )+(1 m) ln( 1 m 1 1/2(m + t) )+ t ln( t 1/2(m + t) )+(1 t) ln( 1 t 1 1/2(m + t) )
Wefirstlyprovethat (10) ≥ (8). Weprovided0ln0 = 0, m 0 = 0. (10)= ln( m 1/2(m + t) )m + ln( 1 m 1 1/2(m + t) )1 m + ln( t 1/2(m + t) )t + ln( 1 t 1 1/2(m + t) )1 t = ln[( m 1/2(m + t) )x ( 1 m 1 1/2(m + t) )1 m ( t 1/2(m + t) )t ( 1 t 1 1/2(m + t) )1 t ]
(10)
(8)= ln[( m 1/2(m + n) )x ( 1 m 1 1/2(m + n) )1 m ( n 1/2(m + n) )n ( 1 n 1 1/2(m + n) )1 n ]
(10) (8)= ln[( m + n m + t )m ( 2t m + t )t ( m + n 2n )n ( 1 1/2(m + n) 1 1/2(m + t) )1 m ( 1 t 1 1/2(m + t) )1 t ( 1 1/2(m + n) 1 n )1 n ]
Let f (z)=( m+n m+t )m ( 2t m+t )t ( m+n 2n )n ( 1 1/2(m+n) 1 1/2(m+t) )1 m ( 1 t 1 1/2(m+t) )1 t ( 1 1/2(m+n) 1 n )1 n
A =( m + n 2n )n ( 1 1/2(m + n) 1 n )1 n , B =( m + n m + t )m , C =( 2t m + t )t , D =( 1 1/2(m + n) 1 1/2(m + t) )1 m , E =( 1 t 1 1/2(m + t) )1 t
Then, f (t)= A ∗ B ∗ C ∗ D ∗ E, f (t)= A(B CDE + BC DE + BCD E + BCDE )
∂B ∂t =(em ln( m+n m+t ) ) = e(m t) ln( m t ) ∗ ( m m + t )= B ∗ ( m m + t )
∂C ∂t =(e(t ln( 2t m+t ) ) = C ∗ (ln( 2t m + t )+ m m + t ) ∂D ∂t =(e(1 m) ln( 1 1/2(m+n) 1 1/2(m+t) ) ) = D ∗ ( 1 m 2 (m + t) )
∂E ∂t =(e(1 t) ln( 1 t 1 1/2(m+t) ) ) = E ∗ ( ln( 1 t 1 1/2(m + t) )+ m 1 1 1/2(m + t) )
f (t)= A ∗ B ∗ C ∗ D ∗ E ∗ (ln( 2t m + t ) ln( 1 t 1 1/2(m + t) ))
Itisclearthat A, B, C, D, E ≥ 0.Since 0 ≤ m ≤ n ≤ t,then 2t ≥ m + t, ln( 2t m+t ) ≥ 0,1 t ≥ 1 1/2(m + t), ln( 1 t 1 1/2(m+t) ) ≤ 0.Thatis, f (t) ≥ 0.When t = n, f (t)= f (n)= 1,then t > n. Where f (t) > f (n)= 1,thenln( f (t)) ≥ 0. Thatistosay,theoriginal (10) (8) ≥ 0,meaningthat E(m, t) ≥ E(m, n) hasbeenobtained. Fromhere,wecontinuetoprovethat (10) ≥ (8)
(9)= ln[( n 1/2(n + t) )n ( 1 n 1 1/2(n + t) )1 n ( t 1/2(n + t) )t ( 1 t 1 1/2(n + t) )1 t ]
(10) (9)= ln[( n + t m + t )t ( 2m m + t )m ( n + t 2n )n ( 1 m 1 1/2(m + t) )1 m ( 1 1/2(n + t) 1 1/2(m + t) )1 t ( 1 1/2(n + t) 1 n )1 n ]
Let g(m)=( n+t m+t )t ( 2m m+t )m ( n+t 2n )n ( 1 m 1 1/2(m+t) )1 m ( 1 1/2(n+t) 1 1/2(m+t) )1 t ( 1 1/2(n+t) 1 n )1 n ] M =( 1 1/2(n + t) 1 n )1 n ( n + t 2n )n , N =( 2m m + t )m , P =( n + t m + t )t , Q =( 1 m 1 1/2(m + t) )1 m , S =( 1 1/2(n + t) 1 1/2(m + t) )1 t ,
Then, g(m)= M ∗ N ∗ P ∗ Q ∗ S, g (m)= M(N PQS + NP QS + NPQ S + NPQS ) ∂ N ∂m = N ∗ (ln 2m m + t + t m + t ) ∂P ∂m = P ∗ ( t m + t )
∂Q ∂m = Q ∗ ( ln 1 m 1 1/2(m + t) + t 1 2 (m + t) )
∂S ∂m = S ∗ ( 1 t 2 (m + t) ) g (x)= M ∗ N ∗ P ∗ Q ∗ S ∗ (ln( 2m m + t ) ln( 1 m 1 1/2(m + t) ))
Itisobviousthat M, N, P, Q, S ≥ 0,since 0 ≤ m ≤ n ≤ t,then 2m ≤ m + t, ln 2m m+t ≤ 0,1 m ≥ 1 1/2(m + t), ln 1 m 1 1/2(m+t) ≥ 0;thatis, g (m) ≤ 0.When m = n, g(m)= g(n)= 1,then m < n, andwhen g(m) > f (n)= 1,then ln g(m) ≥ 0.Thatistosay,theoriginal (10) (9) ≥ 0,meaning that E2(m, t) ≥ E2(n, t) hasbeenobtained.
Finally,weobtaintheproofofthetheorem.
Theorem5. Let X beauniversecourse, M, N, T ∈ FS(X).If M ⊆ N ⊆ T,then E2(M, T) ≥ E2(M, N), E2(M, T) ≥ E2(N, T).
E2(M, N)=I2(M, N)+ I2(N, M)
= n ∑ i=1 µM (xi ) ln( µM (xi ) 1/2(µM (xi )+ µN (xi )) )+(1 µM (xi )) ln( 1 µM (xi ) 1 1/2(µM (xi )+ µN (xi )) ) + n ∑ i=1 µN (xi ) ln( µN (xi ) 1/2(µM (xi )+ µN (xi )) )+(1 µN (xi )) ln( 1 µN (xi ) 1 1/2(µM (xi )+ µN (xi )) )
E2(N, T)=I2(N, T)+ I2(T, N) = n ∑ i=1 µN (xi ) ln( µN (xi ) 1/2(µN (xi )+ µT (xi )) )+(1 µN (xi )) ln( 1 µN (xi ) 1 1/2(µN (xi )+ µT (xi )) ) + n ∑ i=1 µT (xi ) ln( µT (xi ) 1/2(µN (xi )+ µT (xi )) )+(1 µT (xi )) ln( 1 µT (xi ) 1 1/2(µN (xi )+ µT (xi )) )
E2(M, T)=I2(M, T)+ I2(T, M)
= n ∑ i=1 µM (xi ) ln( µM (xi ) 1/2(µM (xi )+ µT (xi )) )+(1 µM (xi )) ln( 1 µM (xi ) 1 1/2(µM (xi )+ µT (xi )) ) + n ∑ i=1 µT (xi ) ln( µT (xi ) 1/2(µM (xi )+ µT (xi )) )+(1 µT (xi )) ln( 1 µT (xi ) 1 1/2(µM (xi )+ µT (xi )) )
(11)
(12)
(13) WecaneasilyobtaintheprooffromTheorem3. Example2. Let X beaspaceofuniversecourse, M, N, T ∈ FS(X),inwhich M = { x,0.5 |x ∈ X}, N = { x,0.7 |x ∈ X}, T = { x,0.9 |x ∈ X} Clearly, M ⊆ N ⊆ T,andwecanget E2(M, N)= 0.042, E2(N, T)= 0.0648, E2(M, T)= 0.2035;thatis,E2(M, T) ≥ E2(M, N), E2(M, T) ≥ E2(N, T). Theorem6. Theabove-definedsymmetryfuzzycross-entropyisakindofdistancemeasure.
4.NeutrosophicCross-EntropyIsaDistanceMeasure
Smarandache[3,27]firstlyproposedthedefinitionofaneutrosophicset,whichisanextensionof anintuitionisticfuzzyset(IFS)andaninterval-valuedintuitionisticfuzzyset,asfollows: Definition6 ([3]). Let X beauniversecourse,whereaneutrosophicset A in X iscomprisedofthe truth-membershipfunction TA (x),indeterminacy-membershipfunction IA (x),andfalsity-membershipfunction
FA (x),inwhichTA (x), IA (x), FA (x) : X →]0 ,1+ [. Thereisnorestrictiononthesumof TA(x), IA(x), FA(x),so 0 ≤ supTA(x)+ supIA(x)+ supFA(x) ≤ 3+ .
Wangetal. [5] introducedthedefinitionofsinglevalueneutrosophicset(SVNS)forbetter applicationintheengineeringfield.SVNSisanextensionoftheIFS,andalsoprovidesanotherwayin whichtoexpressandprocessuncertainty,incomplete,andinconsistentinformationintherealworld. Definition7 ([5]) Let X beaspaceofpoints,whereasingle-valuenetrosophicset A in X iscomprisedofthe truth-membershipfunction TA (x),indeterminacy-membershipfunction IA (x),andfalsity-membershipfunction FA (x).ForeachpointxinX,TA (x), IA (x), FA (x) ∈ [0,1].Therefore,aSVNSAcanbedenotedby: A =(x, TA (x), IA (x), FA (x)) | x ∈ X Thereisnorestrictiononthesumof TA(x), IA(x), FA(x),so 0 ≤ supTA(x)+ supIA(x)+ supFA(x) ≤ 3
ThefollowingaresomepropertiesaboutSVNSs M and N: Let X beauniversecourse, SVNS(X) bethesetofallthesingle-valueneutrosophicsets,and M, N, T ∈ SVNS(X): (1) M ⊆ N if,andonlyif TM (x) ≤ TN (x), IM (x) ≥ IN (x), FM (x) ≥ FN (x) forevery x in X [5]; (2) M = N if,andonlyif A ⊆ B and B ⊆ A [5]; (3) If M ⊆ N, N ⊆ T,then M ⊆ T
Then,Ye[25]firstgeneralizedthefuzzycross-entropymeasuretotheSVNSs.Theinformation measureofneutrosophicsetsarecomposedoftheinformationmeasureofthetruth-membership, indeterminacy-membership,andfalsity-membershipinSVNSs.Let M, N ∈ SVNS(X),whereYe introducedthediscriminationinformationof TM (xi ) from TN (xi ) for (i = 1,2,..., n) onthebasisofthe definitionoffuzzycross-entropy I2(M, N) asthefollowing;
I T 2 (M, N)= n ∑ i=1 (TM (xi ) ln( TM (xi ) 1/2(TM (xi )+ TN (xi )) )+(1 TM (xi )) ln( 1 TM (xi ) 1 1/2(TM (xi )+ TN (xi )) )
Wecandefinethefollowinginformationintermsoftheindeterminacy-membershipfunctionand thefalsity-membershipfunctioninthesameway:
I I 2 (M, N)= n ∑ i=1 (IM (xi ) ln( IM (xi ) 1/2(IM (xi )+ IN (xi )) )+(1 IM (xi )) ln( 1 IM (xi ) 1 1/2(IM (xi )+ IN (xi )) )
I F 2 (M, N)= n ∑ i=1 (FM (xi ) ln( FM (xi ) 1/2(FM (xi )+ FN (xi )) )+(1 FM (xi )) ln( 1 FM (xi ) 1 1/2(FM (xi )+ FN (xi )) )
Definition8 ([25]). Thesingle-valueneutrosophiccross-entropyabout M and N where M, N ∈ SVNS(X) canbedefinedasfollows: I3(M, N)= I T 2 (M, N)+ I I 2 (M, N)+ I F 2 (M, N)
Itcanalsobeusedtoexpressthedegreeofdifferencesof M from N.AccordingtoShannon’s inequality,itisclearthat I3(M, N) ≥ 0,and I3(M, N)= 0 if,andonlyif M = N.Thatis, TM (xi )= TN (xi ), IM (xi )= IN (xi ), FM (xi )= FN (xi ) forany x ∈ X.Then,theneutrosophiccross-entropycanbe modifiedas E3(M, N)= I3(M, N)+ I3(N, M) forthesymmetry.
Theorem7. Let X beaspaceoftheuniversecourse, M, N, T ∈ SVNS(X).If M ⊆ N ⊆ T,then E3(M, T) ≥ E3(M, N),andE3(M, T) ≥ E3(N, T)
AccordingtotheproofofTheorem4,wecaneasilyfindthat ET 2 (M, T) ≥ ET 2 (M, N),and ET 2 (M, T) ≥ ET 2 (N, T).Inasimilarway, EI 2(M, T) ≥ EI 2(M, N),and EI 2(M, T) ≥ EI 2(N, T), EF 2 (M, T) ≥ E2 2 (M, N),and EF 2 (M, T) ≥ EF 2 (N, T),meaningthat E3(M, T) ≥ E3(M, N), E3(M, T) ≥ E3(N, T). Thus,conclusively,wewereabletoeasilyobtaintheproof.
Example3. Let X beaspaceoftheuniversecourse, M, N, T ∈ SVNS(X),where M = {(x,0.5,0.3,0.7) | x ∈ X}, N = {(x,0.7,0.2,0.5) | x ∈ X}, T = {(x,0.8,0.1,0.1) | x ∈ X}
ItisclearthatM ⊆ N ⊆ T,andwecanobtain:
ET 2 (M, N)= 0.0420, ET 2 (N, T)= 0.0134, ET 2 (M, T)= 0.1013; thatis,ET 2 (M, T) ≥ ET 2 (M, N), ET 2 (M, T) ≥ ET 2 (N, T)
EI 2(M, N)= 0.0134, EI 2(N, T)= 0.0199, EI 2(M, T)= 0.0648; thatis,EI 2(M, T) ≥ EI 2(M, N), EI 2(M, T) ≥ EI 2(N, T) EF 2 (M, N)= 0.0420, EF 2 (N, T)= 0.2035, EF 2 (M, T)= 0.4101; thatis,EF 2 (M, T) ≥ EF 2 (M, N), EF 2 (M, T) ≥ EF 2 (N, T) E3(M, T)= ET 2 (M, T)+ EI 2(M, T)+ EF 2 (M, T)= 0.5762, E3(M, N)= 0.0974, E3(N, T)= 0.2368. Thus,EF 3 (M, T) ≥ EF 3 (M, N), EF 3 (M, T) ≥ EF 3 (N, T) Theorem8. Theabove-definedsymmetryneutrosophiccross-entropyisadistancemeasure.
5.Conclusions
Onaccountofthesesimilarpropertiesbetweendistancemeasureandcross-entropy(suchas non-negativityandsymmetry),andwhenthecross-entropy(distance)betweentwofuzzysetsis 0 if, andonlyifthetwosetscoincide.Wealsofoundthatthedecisionprincipleofcross-entropyisconsistent withdecisionprincipleofdistancemeasureindecision-making.Thatdecisionprincipleis,amongall thechoices,wefinallychosetheonewiththesmallestcross-entropy(distance)fromtheidealsolution. Basedontheaboveanalysis,wetendtostudiedtheirrelationships.Inthispaper,wemainlyproved thatthefuzzydiscriminationandimprovedfuzzycross-entropyandneutrosophiccross-entropybased onfuzzydiscriminationweredistancemeasuresinfact.Thatistosay,thesymmetrycross-entropy mentionedinthispaperisconsistentwiththedistancemeasure.Inthefuture,wewilltrytosimplify theformulaandproposeanimprovementtoit.Itishowthecross-entropyformulasarecomposedof logarithmicfunctionswhichiswhatmakesthecalculationsocomplicated.
AuthorContributions: Allauthorshavecontributedequallytothispaper.
Funding: ThisworkhasbeensupportedbytheNationalNaturalScienceFoundationofChina(GrantNo. 61473239).
ConflictsofInterest: Theauthorsdeclarenoconflictofinterest.
References
1. Zadeh,L.A.Fuzzysets. Inf.Control 1965, 8,338–353.
2. Atanassov,K.Intuitionisticfuzzyset. FuzzySetsSyst. 1986, 20,87–96.
3. Smarandache,F. Neutrosophy:NeutrosophicProbability,Set,andLogic;AmericanResearchPress:Rehoboth, DE,USA,1998.
4. Vlachos,I.K.;Sergiadis,G.D.Intuitionisticfuzzyinformation-applicationstopatternrecognition. PatternRecognit.Lett. 2007, 28,197–206.
5. Wang,H.;Smarandache,F.;Zhang,Y.Q.;Sunderraman,R.SingleValuedNeutrosophicSets. Multispace Multistructure 2010, 4,410–413.
Symmetry 2019, 11,386 11of11
6.
Zhang,X.H.Fuzzyanti-groupedfiltersandfuzzynormalfiltersinpseudo-BCIalgebras. J.Intell.FuzzySyst. 2017, 33,1767–1774.
7. Zhang,X.H.;Ma,Y.C.;Smarandache,F.;Dai,J.H.Neutrosophicregularfiltersandfuzzyregularfiltersin pseudo-BCIalgebras. NeutrosophicSetsSyst. 2017, 17,10–15.
8.
9.
Zhang,X.H.;Bo,C.X.;Smarandache,F.;Dai,J.H.Newinclusionrelationofneutrosophicsetswith applicationsandrelatedlatticestructure. Int.J.Mach.Learn.Cybern. 2018, 9,1753–1763, doi:10.1007/s13042-018-0817-6.
Zhang,X.H.;Bo,C.X.;Smarandache,F.;Park,C.Newoperationsoftotallydependent-neutrosophicsetsand totallydependent-neutrosophicsoftsets. Symmetry 2018, 10,187.
10. Zhang,X.H.;Yu,P.;Smarandache,F.;Park,C.RedefinedneutrosophicfiltersinBE-algebras. Ital.J.Pure Appl.Math. 2019,inpress.
11. Liu,F.;Guan,A.W.;Lukovac,V.;Vuki´c,M.Amulticriteriamodelfortheselectionofthetransportservice provider:AsinglevaluedneutrosophicDEMATELmulticriteriamodel. Decis.Mak.Appl.Manag.Eng. 2018, 1,121–130.
12. Mukhametzyanov,I.;Pamuˇcar,D.AsensitivityanalysisinMCDMproblems:Astatisticalapproach. Decis.Mak.Appl.Manag.Eng. 2018, 1,51–80,doi:10.3390/sym10060215.
13. Tu,A.;Ye,J.;Wang,B.SymmetryMeasuresofSimplifiedNeutrosophicSetsforMultipleAttribute Decision-MakingProblems. Symmetry 2018, 10,144,doi:10.3390/sym10050144.
14. Zadeh,L.A.Probabilitymeasuresoffuzzyevents. J.Math.Anal.Appl. 1968, 23,421–427.
15. Kullback,S. InformationTheoryandStatistics;DoverPublications:NewYork,NY,USA,1997.
16. DeLuca,A.S.;Termini,S.Adefinitionofnonprobabilisticentropyinthesettingoffuzzysetstheory. Inf.Control 1972, 20,301–312.
17. Burillo,P.;Bustince,H.Entropyonintuitionisticfuzztsetsandoninterval-valuedfuzzysets. FuzzySetsSyst. 1996, 78,305–316.
18. Szmidt,E.;Kacprzyk,J.Entropyforintuitionisticfuzzysets. FuzzySetsSyst. 2001, 118,467–477.
19. Wei,C.P.;Wang,P.;Zhang,Y.Z.Entropy,similaritymeasureofinterval-valuedintuitionisticfuzzysetsand theirapplications. Inf.Sci. 2011, 181,4273–4286.
20. Lin,J.DivergencemeasuresbasedonShannonentropy. IEEETrans.Inf.Theory 1991, 37,145–151.
21. Bhandari,D.;Pal,N.R.Somenewinformationmeasuresforfuzzysets. Inf.Sci. 1993, 67,209–228.
22. Shang,X.G.;Jiang,W.S.Anoteonfuzzyinformationmeasures. PatternRecognit.Lett. 1997, 18,425–432.
23. Verma,R.Ongeneralizedfuzzydivergencemeasureandtheirapplicationtomulticriteriadecision-making. J.Comb.Inf.Syst.Sci. 2014 39,191–213.
24. Verma,R.;Sharma,B.D.Ongeneralizedintuitionisticfuzzyrelativeinformationandtheirproperties. J.UncertainSyst. 2012, 6,308–320.
25. Ye,J.Singlevaluedneutrosophiccross-entropyformulticriteriadecisionmakingproblems. Appl.Math. Model. 2014, 38,1170–1175.
26. ¸Shahin,R.Cross-entropymeasureonintervalneutrosophicsetsanditsapplicationsinmulticriteriadecision making. NeuralComput.Appl. 2017, 28,1177–1187,doi:10.1007/s00521-015-2131-5.
27. Smarandache,F. AUnifyingFieldinLogics.Neutrosophy:NeutrosophicProbability,SetandLogic;American ResearchPress:Rehoboth,DE,USA,1999.
c 2019bytheauthors.LicenseeMDPI,Basel,Switzerland.Thisarticleisanopenaccess articledistributedunderthetermsandconditionsoftheCreativeCommonsAttribution (CCBY)license(http://creativecommons.org/licenses/by/4.0/).
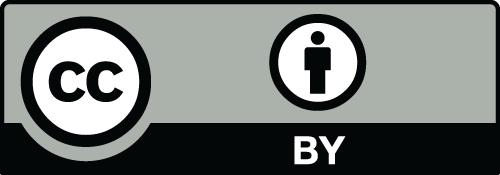