SomeLinguisticNeutrosophicCubicMeanOperators andEntropywithApplicationsinaCorporationto ChooseanAreaSupervisor
MuhammadGulistan 1,*,HafizAbdulWahab 1,FlorentinSmarandache 2 ,SalmaKhan 1 andSayedInayatAliShah 3
1 DepartmentofMathematics,HazaraUniversity,Mansehra21120,Pakistan;wahab@hu.edu.pk(H.A.W.); salmakhan359@gmail.com(S.K.)
2 DepartmentofMathematics,UniversityofNewMexico,Albuquerque,NM87301,USA;smarand@unm.edu
3 DepartmentofMathematics,IslamiaCollegeUniversity,Peshawar25120,Pakistan;inayat64@gmail.com
* Correspondence:gulistanmath@hu.edu.pk;Tel.:+9-299-741-4164
Received:26August2018;Accepted:19September2018;Published:22September2018
Abstract: Inthispaper,wecombinedentropywithlinguistineutrosophiccubicnumbersandusedit indailylifeproblemsrelatedtoacorporationthatisgoingtochooseanareasupervisor,whichisthe maintargetofourproposedmodel.Forthis,wefirstdevelopthetheoryoflinguisticneutrosophic cubicnumbers,whichexplainstheindeterminateandincompleteinformationbytruth,indeterminacy andfalsitylinguisticvariables(LVs)forthepast,present,aswellasforthefuturetime veryeffectively Aftergivingthedefinitions,weinitiatesomebasicoperationsandpropertiesoflinguisticneutrosophic cubicnumbers.WealsodefinethelinguisticneutrosophiccubicHamymeanoperatorandweighted linguisticneutrosophiccubicHamymean(WLNCHM)operatorwithsomeproperties,whichcan handlemulti-inputagentswithrespecttothedifferenttimeframe. Finally,asan application,wegive anumericalexampleinordertotesttheapplicabilityofourproposedmodel.
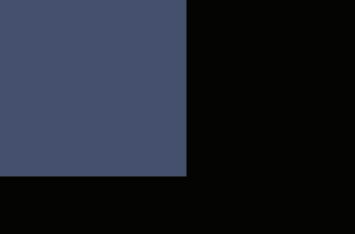
Keywords: neutrosophicset;neutrosophiccubicset;linguisticneutrosophiccubicnumbers; linguisticneutrosophiccubicweightedaveragingoperator;entropyoflinguisticneutrosophic cubicnumbers;application;multipleattributedecisionmakingproblem
1.Introduction
In1965,Zadeh[1]introducedthenotionoffuzzysets,whichbecameasignificanttoolofstudying manyvagueanduncertainconcepts.Ithasalargenumberofapplicationsinsocial,medicineand computersciences.Atanassov[2]generalizedthethemeofafuzzyset(FS)byinitiatingtheideaof intuitionisticfuzzysets(IFS)byintroducingtheideaofnonmembershipofanelementinacertainset. Junetal.[3]initiatedtheideaofcubicsets,inwhichtherearetworepresentations:oneisusedfor themembership/certainvalueandtheotheroneisusedforthenonmembership/uncertainvalue. Themembershipfunctionishandledintheformofaninterval,andthenonmembershipishandled bytheordinaryfuzzyset.Cubicsetshavebeenconsideredbymanyauthorsinotherareasof mathematics,forinstanceKUsubalgebras[4,5], graphtheory[6],leftalmost Γ-semihypergroups[7], LA-semihypergroups[8–11],semigroups[12,13]andHv-LA-semigroups[14,15].Smarandache[16,17] presentedthenewideaoftheneutrosophicset(NS)andneutrosophiclogic,whichthegeneralized fuzzysetandintuitionisticfuzzyset.Theneutrosophicset(NS)isdefinedbytruth,indeterminacy andfalsitymembershipdegrees.Forapplicationsinphysical,technicalanddifferentengineering regions,Wangetal.[18]suggestedtheconceptofasingle-valuedneutrosophicset(SVNS)in 2010.Afterthis,manyresearchersusedneutrosophicsetsindifferentresearchdirectionssuch asDeand Beg[19] andGulistanetal.[20].Junetal.[21,22]extendedtheideaofcubicsetsto
Symmetry 2018, 10,428;doi:10.3390/sym10100428 www.mdpi.com/journal/symmetry
neutrosophiccubicsetsanddefineddifferentpropertiesofexternalandinternalneutrosophiccubicsets. Recently, Gulistanetal.[23] combinedneutrosophiccubicsetswithgraphs.Inmulti-criteriadecision makingproblems,theapplicationofneutrosophiccubicsetswasproposedby Zhanetal.[24].In[25], Hashimetal. usedneutrosophicbipolarfuzzysetsintheHOPEfoundationwithdifferenttypesof similaritymeasures.Fortheaspectsofreal-lifeobjectives,thehumandesireofjudgmentcanbe usedforlinguisticexpressionratherthannumericalexpressiontobettersuitthethinkingofpeople. Therefore,Zadeh[26]introducedtheconceptoflinguisticvariableandappliedittofuzzyreasoning. Theideaofaggregationoperatorswaspresentedbymanyresearchersindecisionmakingproblems;see forexample[27–29].Theconceptoflinguisticintuitionisticfuzzynumbers(LIFN)wasintroducedby Chenetal.[30].Afterthat,someresearchersalsogavetheideaoflinguisticintuitionisticmulti-criteria groupdecision-makingproblems[31].ThethemeofLNNS wasinitiatedby Fangetal.[32].Besides,a multi-criteriadecisionmakingproblemlikethelinguisticintuitionisticmulti-criteriadecision-making problemwasalsointroduced[33].Yein2016presentedtheconceptofanLNNS andalsogavethe ideaofdifferentaggregationoperatorsinmultipleattributegroupdecisionmakingproblems[34]. Then,theconceptofalinguisticneutrosophicnumberwasproposedtosolvemultipleattributegroup decisionmakingproblemsbyLietal.in[35].In[36],Haraetal.proposedsomeinequalitiesforcertain bivariatemeans.Ausefultoolknownasentropyisusedtodeterminetheuncertaintyinsets,like thefuzzyset(FS)andintuitionisticfuzzyset(IFS),whereLNCSisdefinedbymanaginguncertain informationabouttruth,indeterminacyandfalsitymembershipfunctions.In1965,Zadeh[37]first definedtheentropyofFStodeterminetheambiguityinaquantitativemanner.Inthesameway,the non-probabilisticentropywasaxiomatizedby DeLuca-Termini[38].Healsoanalyzedmathematical propertiesofthisfunctionalandgavetheconsiderationsofandapplicabilitytopatternanalysis. AdistanceentropymeasurewasproposedbyKaufmann[39].Anewnon-probabilisticentropy measurewasintroducedby Kosko[40].In[41],MajumdarandSamantaintroducedthenotionof twosingle-valuedneutrosophicsets,theirpropertiesandalsodefinedthedistancebetweenthese twosets.Theyalsoinvestigatedthemeasureofentropyofasingle-valuedneutrosophicset.The entropyofIFSswasintroducedbySzmidtandKacprzyk[42].Thisentropymeasurewasconsistent withtheconsiderationsoffuzzysets.Afterward,themeasurementoffuzzinessintermsofdistance betweenthefuzzysetanditscomplimentwasputforwardby Yager[43];seealso[37,44]formore details.TheentropyintermsofneutrosophicsetswasdiscussedbyPatrascuin[45].Theoflinguistic neutrosophicnumbers(LNNs)andthelinguisticneutrosophicHamymean(HM)(LNHM)operator wasinvestigatedbyLiuetal.,in[46].Yediscussedlinguisticneutrosophiccubicnumbersandtheir multipleattributedecisionmakingmethodin[47].
Thepresentstudyproposesanewnotionoflinguisticneutrosophiccubicnumbers(LNCNs), wheretheundeterminedLNNS agreeswiththetruth,indeterminacyandfalsitymembership.Besides that,wedefinethedifferentoperationsonLNCNs,thelinguisticneutrosophiccubicHamymean operatorandtheweightedlinguisticneutrosophiccubicHamymean(WLNCHM)operatorwithsome propertiesthatcanhandlemulti-inputagentswithrespecttothedifferenttimeframes.Wedefine score,accuracyandcertainfunctionsofLNCNs.Attheend,weusethedevelopedapproachina decisionmakingproblemrelatedtoacorporationchoosinganareasupervisor.
2.Preliminaries
Remark1. [35]Let ˚ A bethesetofLNNS, thenitscomplementisrepresentedby ˚ AC , whichisdenotedas ˚ α ˚ A = ˚ γ ˚ A; ˚ β ˚ A = t ˚ β ˚ A; ˚ γ ˚ A = ˚ α ˚ A.
Definition2. [35]Let ˚ g =( ˚ pα , ˚ pβ , ˚ pγ ), ˚ g1 =( ˚ pα1 , ˚ pβ1 , ˚ pγ1 ), ˚ g2 =( ˚ pα2 , ˚ pβ2 , ˚ pγ2 ) beanyLNNS and λ > 0. Then(i): ˚ g1 ⊕ ˚ g2 =( ˚ p ˚ α1 , ˚ p ˚ β1 , ˚ p ˚ γ1 ) ⊕ ( ˚ p ˚ α2 , ˚ p ˚ β2 , ˚ p ˚ γ2 )= ˚ p ˚ α1+ ˚ α2 ˚ α1 ˚ α2 t , ˚ p ˚ β1 ˚ β2 t , ˚ p ˚ γ1 ˚ γ2 t (1)
(ii):
˚ g1 ⊗ ˚ g2 =( ˚ p ˚ α1 , ˚ p ˚ β1 , ˚ p ˚ γ1 ) ⊗ ( ˚ p ˚ α2 , ˚ p ˚ β2 , ˚ p ˚ γ2 )= ˚ p α1 α2 t , ˚ pβ1+β β1 β2 t , ˚ p ˚ γ1+ ˚ γ2 γ1 γ2 t (2)
(iii):
λ ˚ g = λ( ˚ pα , ˚ pβ , ˚ pγ )= ˚ pt t(1 α t )λ , ˚ pt( β t )λ , ˚ pt( γ t )λ ;(3) (iv)
˚ gλ =( ˚ p ˚ α , ˚ p ˚ β , ˚ p ˚ γ )λ = ˚ pt( ˚ α t )λ , ˚ pt t(1 ˚ β t )λ , ˚ pt t(1 ˚ γ t )λ .(4)
Definition3. [35]Let ˚ g =( ˚ p ˚ α , ˚ p ˚ β , ˚ p ˚ γ ) beanLNN.ThefollowingarethescoreandaccuracyfunctionofLNN, ˆ S( ˚ g)= 2t + ˚ α ˚ β ˚ γ 3t ,(5)
ˆ H( ˚ g)= ˚ α ˚ γ t .(6)
Definition4. [35]Let ˚ g1 =( ˚ p ˚ α1 , ˚ p ˚ β1 , ˚ p ˚ γ1 ), ˚ g2 =( ˚ p ˚ α2 , ˚ p ˚ β2 , ˚ p ˚ γ2 ) beLNNs Then:(1)If ˆ S( ˚ g1) < ˆ S( ˚ g2), then ˚ g1 ≺ ˚ g2 (2)If ˆ S( ˚ g1)= ˆ S( ˚ g2), (a)and ˆ H( ˚ g1) < ˆ H( ˚ g2), then ˚ g1 ≺ ˚ g2, (b)and ˆ H( ˚ g1)= ˆ H( ˚ g2), then ˚ g1 ≈ ˚ g2
Definition5. [36]Suppose uˆ ı (ˆ ı = 1,2, , n) isanassortmentofnon-negativerealnumbersandparameter ˚ k = 1,2,..., n TheHamymean(HM)isdefinedas: HM ˚ k (x1, x2,..., xn )= ∑ 1≤ˆ ı1<... ˆ ı˚ k ≤n
1 k (n ˚ k) (7) where ˆ ı1, ˆ ı2,..., ˆ ık navigateallthek-tuplearrangementsof (1,2,..., n), (n ˚ k) isthe binomialcoefficientand (n k) = n! ˚ k!(n ˚ k)!
˚ k ∏ j=1 uıj
ThefollowingaresomepropertiesofHM: (1) HM( ˚ k) (0,0,...,0)= 0, HM( ˚ k) (u, u,..., u)= u, (2) HM( ˚ k) (u1, u2, ... , un ) ≤ HM( ˚ k) (v1, v2, ... , vn ), ifuˆ ı ≤ vˆ ı forall ˆ ı, (3) min{uˆ ı }≤ HM( ˚ k) (u1, u2,..., un ) ≤ max{uˆ ı }.
Definition6. [17](Neutrosophicset)Let U beanon-emptyset.Aneutrosophicsetin U isastructureofthe form A := {u; ATru (u), Aˆ ınd (u), AFal (u)|u ∈ U}, ischaracterizedbyatruthmembership Tru,indeterminacy membership ˆ ındandfalsitymembershipFal,whereATru, Aˆ ınd, AFal : U → [0,1] Definition7. [21](Neutrosophiccubicset)LetXbeanon-emptyset;anNCSin U isdefinedintheformof apair Ω =( ˚ A, Λ) where ˚ A = {(x; ˚ ATru(u), ˚ AInd(u), ˚ AFal(u) ) | u ∈ U} isanintervalneutrosophicsetin U and Λ = {(u; ΛTru(u), Λınd(u), ΛFal(u) ) | u ∈ U)} isaneutrosophicsetinU
3.LinguisticNeutrosophicCubicNumbersandOperators
Inthissection,wedefinethelinguisticneutrosophiccubicnumbersandalsodiscussdifferent operationsandpropertiesrelatedtolinguisticneutrosophiccubicnumbers.WedefinethecubicHamy meanoperator,LNCHMoperatorandWLNCHMoperatoranddiscusstheirproperties.
Definition8. LNCNs(linguisticneutrosophiccubicnumbers):Let U beauniversalsetand ˚ p =( ˚ p0, ˚ p1,..., ˚ pt ) beaLTS.AnLNCN ˚ A in U isdeterminedbytruthmembershipfunction ( ˜ α ˚ A, ˚ α ˚ A ), anindeterminacymembershipfunction (β ˚ A, ˚ β ˚ A ) andafalsitymembershipfunction (γ ˚ A, ˚ γ ˚ A ), where α ˚ A, β ˚ A, γ ˚ A : U → D[0, t] and ˚ α ˚ A, ˚ β ˚ A, ˚ γA : U → [0, t], ∀ u ∈ U,anditisdenotedby ˚ g = ( ˚ p(αA , ˚ αA )(u), ˚ p(β ˚ A , ˚ β A )(u), ˚ p(γA , ˚ γA )(u) ) ∈ ˚ A.
Remark2. Suppose A isasetofLNCNs,thenitscomplementisrepresentedby Ac anddefinedas {(αA, ˚ αA )c = (γA, ˚ γA ), ( ˜ β A, ˚ β A )c =(t ˜ β A, t ˚ β A ), (γA, ˚ γA )c =(αA, ˚ αA )}
Definition9. Let ˚ g = ˚ p(α, ˚ α), ˚ p(β, ˚ β), ˚ p(γ, ˚ γ) , ˚ g1 = ˚ p(α1, ˚ α1), ˚ p(β1, ˚ β1 ), ˚ p(γ1, ˚ γ1) , ˚ g2 = ˚ p(α2,α2), ˚ p(β2, ˚ β2 ), ˚ p(γ2,γ2) beanyLNCNsand λ > 0. Then,wedefine: (i): ˚ g1 ⊕ ˚ g2 = ˚ p(α1, ˚ α1), ˚ p(β1, ˚ β1 ), ˚ p(γ1, ˚ γ1) ⊕ ˚ p(α2, ˚ α2), ˚ p(β2, ˚ β2 ), ˚ p(γ2, ˚ γ2) (8) = ˚ p(α1+α2,α1+α2) α1 α2 t , α1 α2 t , ˚ p β1 β2 t , β1 β2 t , ˚ p γ1 γ2 t , γ1 γ2 t
;
(ii): ˚ g1 ⊗ ˚ g2 = ˚ p(α1, ˚ α1), ˚ p(β1, ˚ β1 ), ˚ p(γ1, ˚ γ1) ⊗ ˚ p(α2, ˚ α2), ˚ p(β2, ˚ β2 ), ˚ p(γ2, ˚ γ2) (9) = ˚ p α1 α2 t , α1 α2 t , ˚ p(β1+β2, ˚ β1+ ˚ β2 ) β1 β2 t , β1 β2 t , ˚ p(γ1+γ2, ˚ γ1+ ˚ γ2) γ1 γ2 t , γ1 γ2 t
;
(iii): λ ˚ g = λ ˚ p(α1, ˚ α1), ˚ p(β1,β1 ), ˚ p(γ1, ˚ γ1) ⊕ ˚ p(α2, ˚ α2), ˚ p(β2,β2 ), ˚ p(γ2, ˚ γ2) (10) = ˚ pt t(1 α t ,1 ˚ α t )λ , ˚ p t β t , β t λ , ˚ p t γ t , ˚ γ t λ
(iv): ˚ gλ = ˚ p(α1, ˚ α1), ˚ p(β1, ˚ β1 ), ˚ p(γ1, ˚ γ1) ⊕ ˚ p(α2, ˚ α2), ˚ p(β2, ˚ β2 ), ˚ p(γ2, ˚ γ2) λ (11) = ˚ pt( α t , α t )λ , ˚ p t t 1 β t ,1 ˚ β t λ , ˚ p t t 1 γ t ,1 γ t λ
ItisclearthattheseoperationalresultarestillLNCNs.
Definition10. Let ˚ g = ˚ p(α, ˚ α), ˚ p(β,β), ˚ p(γ, ˚ γ) , beanLNCNthatdependson LTS , ˚ p. Then,thescorefunction, accuracyfunctionandcertainfunctionoftheLNCN, ˚ g,aredefinedasfollows:
(i):
ϕ( ˚ g)= ϕ ˚ p(α, ˚ α), ˚ p(β, ˚ β), ˚ p(γ, ˚ γ) = 1 9t [ 4t + ˚ pα ˚ pβ ˚ pγ + 2t + ˚ p ˚ α ˚ p ˚ β ˚ p ˚ γ ], for ϕ( ˚ g) ∈ [0,1] (12) (ii): Φ( ˚ g)= 1 3t [( ˚ pα ˚ pγ ) + ˚ p ˚ α ˚ p ˚ γ ], for Φ( ˚ g) ∈ [ 1,1] (13) (iii): Ψ( ˚ g)= ˚ pα + ˚ p ˚ α 3t for Ψ( ˚ g) ∈ [0,1].(14)
Now,withthehelpoftheabove-definedfunction,weintroducearankingmethodforthese function. Definition11. LettwoLNCNsbe ˚ g1 = ˚ p(α1, ˚ α1), ˚ p(β1, ˚ β1 ), ˚ p(γ1, ˚ γ1) and ˚ g2 = ˚ p(α2, ˚ α2), ˚ p(β2, ˚ β2 ), ˚ p(γ2, ˚ γ2) Then,theirrankingmethodisdefinedas:
1. If ϕ( ˚ g1) > ϕ( ˚ g2),then ˚ g1 ˚ g2,
2. If ϕ( ˚ g1)= ϕ( ˚ g2) and Φ( ˚ g1) > Φ( ˚ g2),then ˚ g1 ˚ g2,
3. If ϕ( ˚ g1)= ϕ( ˚ g2), Φ( ˚ g1)= Φ( ˚ g2) and Ψ( ˚ g1) > Ψ( ˚ g2),then ˚ g1 ˚ g2,
4. If ϕ( ˚ g1)= ϕ( ˚ g2), Φ( ˚ g1)= Φ( ˚ g2) and Ψ( ˚ g1)= Ψ( ˚ g2),then ˚ g1 ∼ ˚ g2
Example1. Let ˚ g1 = ˚ p(α1, ˚ α1), ˚ p(β1, ˚ β1 ), ˚ p(γ1, ˚ γ1) , ˚ g2 = ˚ p( ˚ α2, ˚ α2), ˚ p( ˚ β2, ˚ β2 ), ˚ p( ˚ γ2, ˚ γ2) and ˚ g3 = ˚ p(α3, ˚ α3), ˚ p(β3, ˚ β3 ), ˚ p(γ3, ˚ γ3) bethreeLNCNsinthelinguistictermset ϕ = { ϕ ˚ g | ˚ g ∈ [0,8]} where ˚ g1 = ([0.2,0.3] , [0.4,0.5] , [0.3,0.5] , (0.1,0.2,0.3)) , ˚ g2 = ([0.3,0.4] , [0.4,0.5] , [0.5,0.6] , (0.2,0.4,0.6)) , ˚ g3 = ([0.4,0.5] , [0.4,0.6] , [0.5,0.7] , (0.2,0.3,0.5)) , thenwewillfindthevaluesoftheirscore,accuracy andcertainfunctionasfollows:
(i)Scorefunctions:
ϕ( ˚ g)= 1 9t [ 4t + ˚ pα ˚ pβ ˚ pγ + 2t + ˚ p ˚ α ˚ p ˚ β ˚ p ˚ γ ], for ϕ( ˚ g) ∈ [0,1]
ϕ( ˚ g1)= [32 + 0.2 + 0.3 (0.4 + 0.5 + 0.3 + 0.5)+ 16 + 0.1 (0.2 + 0.3)] 72 = 0.644
ϕ( ˚ g2)= [32 + 0.3 + 0.4 (0.4 + 0.5 + 0.5 + 0.6)+ 16 + 0.2 (0.4 + 0.6)] 72 = 0.6375
ϕ( ˚ g3)= [32 + 0.4 + 0.5 (0.4 + 0.6 + 0.5 + 0.7)+ 16 + 0.2 (0.3 + 0.5)] 72 = 0.638
(ii)Accuracyfunctions:
Φ( ˚ g)= 1 3t [( ˚ pα ˚ pγ ) + ˚ p ˚ α ˚ p ˚ γ ], for Φ( ˚ g) ∈ [ 1,1]
Φ( ˚ g1)= [0.2 + 0.3 (0.3 + 0.5)+ 0.1 0.3] 24 = 0.0208
Φ( ˚ g2)= [0.3 + 0.4 (0.5 + 0.6)+ 0.2 0.6] 24 = 0.0333
Φ( ˚ g3)= [0.4 + 0.5 (0.6 + 0.7)+ 0.3 0.5] 24 = 0.0292
(iii)Certainfunctions:
Ψ( ˚ g)= ˚ pα + ˚ p ˚ α 3t for Ψ( ˚ g) ∈ [0,1]
Ψ( ˚ g1)= [0.2 + 0.3 + 0.1] 24 = 0.025
Ψ( ˚ g2)= [0.3 + 0.4 + 0.2] 24 = 0.0375
Ψ( ˚ g3)= [0.4 + 0.5 + 0.2] 24 = 0.0416
Definition12. Suppose (uˆ ı, uˆ ı ) where ˆ ı = 1,2, , n isanassortmentofnon-negativerealnumbersand parameter ˚ k = 1,2,..., n Then,thecubicHamymean(CHM)isdefinedasfollows:
CHM ˚ k ( ˜ uı, uı )= ∑ 1≤ˆ ı1< ˆ ı˚ k ≤n
˚ k ∏ j=1 uıj ,
˚ k ∏ j=1 uıj
1 k (n ˚ k) (15) where ˆ ı1, ˆ ı2,..., ˆ ık navigateallthek-tuplearrangementsof (1,2,..., n ), (n k) isthebinomialcoefficientand (n k) = n! ˚ k!(n ˚ k)!
Example2. Let ( ˜ uˆ ı, uˆ ı )= (( ˜ u1, u1), ( ˜ u2, u2)) i = 1,2 and k = 1, where u1 = ([0.2,0.4] , (0.6)) , u2 = ([0.3,0.5] , (0.7))
CHM1 ((u1, u1), (u2, u2))
= ∑ (((u11, u11)(u22, u22)))1 (2 1) = (((u11, u11)(u22, u22)))1 + (((u11, u11)(u22, u22)))1 (2 1) = ∑ (([0.2,0.4] , (0.6))([0.2,0.4] , (0.6))) (([0.3,0.5] , (0.7))([0.3,0.5] , (0.7)))
1 (2 1) =
(([0.2,0.4] , (0.6))([0.2,0.4] , (0.6))) (([0.3,0.5] , (0.7))([0.3,0.5] , (0.7)))
1 + (([0.2,0.4] , (0.6))([0.2,0.4] , (0.6))) (([0.3,0.5] , (0.7))([0.3,0.5] , (0.7)))
1 (2 1) =
(([0.04,0.16] , (0.84))([0.09,0.25] , (0.91))) + (([0.04,0.16] , (0.84))([0.09,0.25] , (0.91))) (2 1) =
([0.004,0.04] , (0.98)) + ([0.004,0.04] , (0.98)) (2 1) = ([0.008,0.08] , (0.96)) (2 1) = ([0.004,0.04] , (0.48))
k ∏ j=1
˚ gˆ ıj , k ∏ j=1
Definition13. Suppose (gı, ˚ gı ) where ˆ ı = 1,2, ... , n. isanassortmentoflinguisticneutrosophiccubic numbersandparameter ˚ k = 1,2,..., n. Then,theLNCHMoperatorisdefinedasfollows: LNCHM ˚ k (gˆ ı, ˚ gˆ ı )= ∑ 1≤ı1< ık ≤n
1 ˚ k (n ˚ k) (16) where ˆ ı1, ˆ ı2,..., ˆ ı˚ k navigateallthek-tuplearrangementsof (1,2,..., n ), (n ˚ k) isthebinomialcoefficientand (n ˚ k) = n! ˚ k!(n ˚ k)!
˚ gˆ ıj
Example3. Let (gˆ ı, ˚ gˆ ı )= ((g1, ˚ g2), (g2, ˚ g2)) i = 1,2 and k = 1, where g1 = ([0.2,0.4] , [0.3,0.4] , [0.4,0.6] , (0.6,0.5,0.8)) , g2 = ([0.3,0.5] , [0.4,0.7] , [0.2,0.4] , (0.7,0.8,0.6)) ,
LNCHM1 ((g1, ˚ g2), (g2, ˚ g2)) = ∑ (((g11, ˚ g11), (g22, ˚ g22)))1 (2 1) = (((g11, ˚ g11)(g22, ˚ g22)))1 + (((g11, ˚ g11)(g22, ˚ g22)))1 (2 1)
([0.2,0.4] , [0.3,0.4] , [0.4,0.6] , (0.6,0.5,0.8)) ([0.2,0.4] , [0.3,0.4] , [0.4,0.6] , (0.6,0.5,0.8)) ([0.3,0.5] , [0.4,0.7] , [0.2,0.4] , (0.7,0.8,0.6)) ([0.3,0.5] , [0.4,0.7] , [0.2,0.4] , (0.7,0.8,0.6))
([0.2,0.4] , [0.3,0.4] , [0.4,0.6] , (0.6,0.5,0.8)) ([0.2,0.4] , [0.3,0.4] , [0.4,0.6] , (0.6,0.5,0.8)) ([0.3,0.5] , [0.4,0.7] , [0.2,0.4] , (0.7,0.8,0.6)) ([0.3,0.5] , [0.4,0.7] , [0.2,0.4] , (0.7,0.8,0.6))
1 (2 1) =
([0.04,0.16] , [0.09,0.16] , [0.16,0.36] , (0.84,0.75,0.96)) ([0.09,0.25] , [0.16,0.49] , [0.04,0.16] , (0.91,0.96,0.84)) + ([0.04,0.16] , [0.09,0.16] , [0.16,0.36] , (0.84,0.75,0.96)) ([0.09,0.25] , [0.16,0.49] , [0.04,0.16] , (0.91,0.96,0.84)) (2 1) =
(
n) beanarrangementofLNCNs,then
(17)
Proof. AccordingtoEquations(1)–(4),wehave:
twoLNCNs(suppose ˚ k = 2)andtoproduceaninclusivevalue LNCHM( ˚ k) ( ˚ g1, ˚ g2)= ˚ p(α, ˚ α), ˚ p(β, ˚ β), ˚ p(γ, ˚ γ) describedasfollows;where: ( ˚ g1, ˚ g2)= ([0.2,0.3], [0.2,0.5], [0.2,0.5], (0.9,0.7,0.9)) , ([0.4,0.5], [0.3,0.5], [0.3,0.5], (0.8,0.8,0.6)) (i):
1 n ˚ k = ([0.3,0.5] ,0.74)
Therefore,weget: LNCM2(g1, ˚ g2)= ˚ p(α,α), ˚ p(β, ˚ β), ˚ p(γ,γ) =([0.28,0.39], [0.3,0.5] , [0.3,0.5] , (0.17,0.75,0.74)). Now,wewillstudysomeoftheidealpropertiesofLNCNs.
Property1. (Idempotency)If ( ˜ gˆ ı, ˚ gˆ ı )=( ˜ g, ˚ g)= ˚ p(α,α), ˚ p(β, ˚ β), ˚ p(γ,γ) ∀ (ˆ ı = 1,2,..., n),then: LNCHM ˚ k (g, ˚ g)= ˚ p(α, ˚ α), ˚ p(β, ˚ β), ˚ p(γ, ˚ γ) (18)
˚ p(β, ˚ β), ˚ p(γ,γ) =( ˜ g, ˚ g)
Property2. (Commutativity)Let ( ˜ gˆ ı, ˚ gˆ ı ) forall (ˆ ı = 1,2,..., n) beanassortmentofLNCNsand ( ˜ gı , ˚ gı ) be anypermutationof (gˆ ı, ˚ gˆ ı ),then: LNCHM ˚ k (gˆ ı , ˚ gˆ ı )= LNCHM ˚ k (gˆ ı, ˚ gˆ ı ) (19)
1≤ˆ ı1< ˆ ı˚ k ≤n
˚ k ∏ j=1ıj gıj ,
˚ k ∏ j=1
Proof. Theconclusionisobvious,becauseProperty 2 dependsonDefinition 13. LNCHM ˚ k (gı , ˚ gı )= ∑
˚ gˆ ıj
1 ˚ k (n ˚ k) = ∑ 1≤ı1< ık ≤n
˚ k ∏ j=1 gˆ ıj ,
= LNCHM ˚ k (gı, ˚ gı )
˚ k ∏ j=1
˚ gˆ ıj
1 ˚ k (n ˚ k)
Property3. (Monotonicity)Let (gı, ˚ gı )= ˚ p( ˚ αˆ ı , ˚ αˆ ı ), ˚ p( ˚ βı , ˚ βı ), ˚ p( ˚ γˆ ı , ˚ γˆ ı ) , fı, fı = ˚ p(qˆ ı ,qˆ ı ), ˚ p(rˆ ı ,rˆ ı ), ˚ p(sˆ ı ,sˆ ı ) (ˆ ı = 1,2,..., n) betwo collectionsofLNCNs;if ( ˜ αı, ˚ αı ) ≤ ( ˜ qı, qı ), (βı, ˚ βı ) ≤ (˜ rı, rı ), ( ˜ γı, ˚ γı ) ≤ ( ˜ sı, sı ) forall ˆ ı, then:
LNCHM ˚ k ( ˚ gˆ ı, ˚ gˆ ı ) ≤ LNCHM ˚ k fˆ ı, fˆ ı (20)
Proof. Since 0 ≤ (αˆ ı, ˚ αˆ ı ) ≤ (qˆ ı, qˆ ı ), (βˆ ı, ˚ βˆ ı ) ≥ (rˆ ı, rˆ ı ) ≥ 0, (γˆ ı, ˚ γˆ ı ) ≥ (sˆ ı, sˆ ı ) ≥ 0, t ≥ 0 andaccordingto Theorem 1,weget:
Let ( ˜ g, ˚ g)= LNCHM ˚ k ( ˜ gˆ ı, ˚ gˆ ı ), f, f = LNCHM ˚ k fˆ ı, fˆ ı and ψ( ˚ g) and Ψ( f ) bethescorefunctions of ˚ g and f .AccordingtothescorevalueinDefinition 11 andtheaboveinequality,wecansimplyhave ψ( ˚ g) ≤ Ψ( f ).Then,inthefollowing,wearguesomecases: 1. If ψ( ˚ g) ≤ Ψ( f ),wecanobtain LNCHM ˚ k (gˆ ı, ˚ gˆ ı ) ≤ LNCHM ˚ k fˆ ı, fˆ ı ; 2. if ψ( ˚ g)= Ψ( f ),then: 2t
(n k ) , andbasedontheaccuracyvalueinDefinition 11,then Φ( ˚ g)= Φ( f ).Finally,weget: LNCHM ˚ k (gı, ˚ gı ) ≤ LNCHM ˚ k fı, fı Property4. (Boundedness)Let ( ˜ gı, ˚ gı )=( ˚ pα ı , ˚ pβı , ˚ pγı , ˚ p ˚ αı , ˚ p ˚ βı , ˚ p ˚ γı )(ˆ ı = 1,2, , n) bethecollectionof LNCNsand: ˚ g+ = max( ˚ pmax(αˆ ı ), ˚ pmin(βı ), ˚ pmin(γˆ ı ), ˚ pmax( ˚ αˆ ı ), ˚ pmin( ˚ βı ), ˚ pmin( ˚ γˆ ı ) ), ˚ g = min(gˆ ı, ˚ gˆ ı )=( ˚ pmin(αı ), ˚ pmax(βı ), ˚ pmax(γı ), ˚ pmin( ˚ αˆ ı ), ˚ pmax( ˚ βı ), ˚ pmax( ˚ γˆ ı ) ), then ˚ g ≤ LNCHM ˚ k ( ˜ gı, ˚ gı ) ≤ ˚ g+ (21)
Proof. BasedonProperties 1 and 3,wehave:
LNCHM ˚ k (gı, ˚ gı ) ≥ LNCHM ˚ k (gˆ ı , ˚ gˆ ı )= ˚ g
LNCHM ˚ k (gı, ˚ gı ) ≤ LNCHM ˚ k (g+ ˆ ı , ˚ g+ ˆ ı )= ˚ g+ .
Theproofiscompleted. Inaddition,wewilldeliberateaboutsomedesirablecasesoftheLNCHMoperatorforthe parameter ˚ k
Definition14. Suppose ( ˚ gˆ ı, ˚ gˆ ı ) where ˆ ı = 1,2, , n isanassortmentoflinguisticneutrosophiccubic numbersandparameter ˚ k = 1,2, , n and ˚ w =( ˚ w1, ˚ w2 , ˚ wn )T theweightvectorof ˆ ıˆ ı with ˚ wˆ ı ∈ [0,1] and n ∑ ı=1
˚ wı = 1, thentheWLNCHMoperatorisdefinedas: WLNCHM ˚ k (gˆ ı, ˚ gˆ ı )= ∑ 1≤ı1< ık ≤n
k ∏ j=1
˚ wˆ ıj ˜ gˆ ıj, k ∏ j=1
˚ wˆ ıj ˚ gˆ ıj
1 ˚ k (n ˚ k) (24) where ˆ ı1, ˆ ı2,..., ˆ ı˚ k navigateallthek-tuplearrangementsof (1,2,..., ˚ n), (n ˚ k) isthebinomialcoefficientand (n ˚ k) = n! ˚ k!(n ˚ k)!
Example5. Let (gˆ ı, ˚ gˆ ı )= ((g1, ˚ g1), (g2, ˚ g2)) i = 1,2 and k = 1, where g1 = ([0.2,0.4] , [0.3,0.4] , [0.4,0.6] , (0.6,0.5,0.8)) , g2 = ([0.3,0.5] , [0.4,0.7] , [0.2,0.4] , (0.7,0.8,0.6)) and ˚ w = (0.5,0.5):
WLNCHM1 ((g1, ˚ g2), (g2, ˚ g2)) = ∑ ((( ˚ w11 g11, ˚ w11 ˚ g11)( ˚ w22 g22, ˚ w22 ˚ g22)))1 (2 1)
((( ˚ w11 g11, ˚ w11 ˚ g11), ( ˚ w22 g22, ˚ w22 ˚ g22)))1 + ((( ˚ w11 g11, ˚ w11 ˚ g11), ( ˚ w22 g22, ˚ w22 ˚ g22)))1 (2 1)
∑
(0.5)(0.5) ([0.2,0.4] , [0.3,0.4] , [0.4,0.6] , (0.6,0.5,0.8)) ([0.2,0.4] , [0.3,0.4] , [0.4,0.6] , (0.6,0.5,0.8)) (0.5)(0.5) ([0.3,0.5] , [0.4,0.7] , [0.2,0.4] , (0.7,0.8,0.6)) ([0.3,0.5] , [0.4,0.7] , [0.2,0.4] , (0.7,0.8,0.6))
1
(0.5)(0.5)
([0.2,0.4] , [0.3,0.4] , [0.4,0.6] , (0.6,0.5,0.8)) ([0.2,0.4] , [0.3,0.4] , [0.4,0.6] , (0.6,0.5,0.8)) (0.5)(0.5) ([0.3,0.5] , [0.4,0.7] , [0.2,0.4] , (0.7,0.8,0.6)) ([0.3,0.5] , [0.4,0.7] , [0.2,0.4] , (0.7,0.8,0.6))
(0.5)(0.5) ([0.2,0.4] , [0.3,0.4] , [0.4,0.6] , (0.6,0.5,0.8)) ([0.2,0.4] , [0.3,0.4] , [0.4,0.6] , (0.6,0.5,0.8)) (0.5)(0.5) ([0.3,0.5] , [0.4,0.7] , [0.2,0.4] , (0.7,0.8,0.6)) ([0.3,0.5] , [0.4,0.7] , [0.2,0.4] , (0.7,0.8,0.6))
([0.003,0.01] , [0.006,0.01] , [0.01,0.023] , (0.3,0.23,0.2)) ([0.006,0.02] , [0.01,0.034] , [0.03,0.01] , (0.32,0.2,0.3)) + ([0.003,0.01] , [0.006,0.01] , [0.01,0.023] , (0.3,0.23,0.2)) ([0.006,0.02] , [0.01,0.034] , [0.03,0.01] , (0.32,0.2,0.3)) (2 1) =
1 (2 1) =
([0.00002,0.0002] , [0.00006,0.00034] , [0.0003,0.0023] , (0.52,0.4,0.44)) + ([0.00002,0.0002] , [0.00006,0.00034] , [0.0003,0.0023] , (0.52,0.4,0.44)) (2 1) = ([0.00004,0.0004] , [0.00012,0.0007] , [0.0006,0.005] , (0.3,0.2,0.23)) (2 1) = ([0.00002,0.0002] , [0.00006,0.0004] , [0.0003,0.003] , (0.2,0.1,0.12))
DependingontheoperationsofLNCNsthatweregivenintheaboveEquations(1)–(4),withthehelpof Equation(24),wecanformulatethefollowingtheorem. Theorem2. Let (gˆ
, ˚ gˆ
)=( ˚ p(α, ˚
), ˚ p(β,
β), ˚ p(γ, ˚ γ) )(ˆ ı = 1,2, ... , n) bethecollectionofLNCNs, ˚ w = ( ˚ w1, ˚ w2 , ˚ wn )T betheweightvectorof ˆ
with ˚ wˆ ı ∈ [0,1], ˆ ı = 1,2, , n and n ∑ ı=1
˚ wˆ ı = 1. Then,the accumulatedvalueacquiredfromtheWLNCMoperatorinEquation(24)isobviouslyanLNCN,and:
˚ p t k ∏ j 1
k 1 ˚ k =
j
1 1 ˚ βıj t , βıj t wıj ˚ p t t ∏ 1≤ˆ ı1 < ˆ ık ≤n
t ˚ gıj
˚ k , ˚ p t t 1 k ∏ j 1 1 1 αıj t ,1 αıj t wıj 1 k , ˚ p t ∏ 1≤ˆ ı1 < ˆ ık ≤n
k 1 k ∏ j 1 1 βıj t , βıj t wıj 1 k , ˚ p t ∏ 1≤ı1 < ık ≤n
j 1 ˚ k ∏ j=1 1 γıj t , ˚ γıj t wıj 1 k
1 1
whichprovesTheorem.
Accordingtotheoperatingrulesofthe LNCNs,theWLNCHMoperatorsalsohavethesame propertiesinthefollowing:
Property5. (Commutativity)Let ( ˚ gı, ˚ gı ) forall (ˆ ı = 1,2,..., n) , beanassortmentof LNCNsand (gˆ ı , ˚ gˆ ı ) be anypermutationof (gı, ˚ gı ),then: WLNCHM ˚ k ( ˜ gı , ˚ gı )= LNCHM ˚ k ( ˜ gı, ˚ gı ) (26)
BasedonDefinition(13),theconclusionisobvious, WLNCHM ˚ k ( ˚ wıj gı , ˚ wıj ˚ gı ) = ∑ 1≤ˆ ı1< ˆ ı˚ k ≤n
˚ k ∏ j=1
˚ k ∏ j=1
˚ wıj gıj,
˚ k ∏ j=1
˚ wıj ˚ gıj
˚ wıj gıj,
1 k (n ˚ k) = ∑ 1≤ˆ ı1< ˆ ık ≤n
˚ k ∏ j=1
˚ wıj ˚ gıj
1 k (n ˚ k)
= WLNCHM ˚ k ( ˜ gı, ˚ gı )
Property6. (Monotonicity)Let (gˆ ı, ˚ gˆ ı )=( ˚ p(α, ˚ α), ˚ p(β, ˚ β), ˚ p(γ, ˚ γ) ), fˆ ı, fˆ ı = ˚ p(pı ,pı ), ˚ p(qı ,qı ), ˚ p(rı ,rı ) (ˆ ı = 1,2,..., n) betwocollectionsof LNCNs;if αı ≤ pı, ˜ βı ≤ qı, γı ≤ rı, and ˚ αı ≤ ˚ pı, ˚ βı ≤ qı, ˚ γı ≤ rı forall ˆ ı, then:
WLNCHM ˚ k ( ˜ gı, ˚ gı ) ≤ WLNCHM ˚ k ˜ fı, fı (27)
Property7. (Idempotency)If (gˆ ı, ˚ gˆ ı )=(g, ˚ g)=( ˚ p(α,α), ˚ p(β, ˚ β), ˚ p(γ,γ) ) forall (ˆ ı = 1,2,..., n),then:
WLNCHM ˚ k ( ˚ g, ˚ g)=( ˚ p(α, ˚ α), ˚ p(β, ˚ β), ˚ p(γ, ˚ γ) ) (28)
Property8. (Boundedness)Let (gı, ˚ gı )(ˆ ı = 1,2, , n) beanassortmentof LNCNsand ˚ g+ = max(gı, ˚ gı ), ˚ g = min(gı, ˚ gı ),then: ˚ g ≤ WLNCHMk ( ˜ gˆ ı, ˚ gˆ ı ) ≤ ˚ g+ (29)
BasedonProperties 5 and 6,wehave, WLNCHM ˚ k (gı, ˚ gı ) ≥ WLNCHM ˚ k (gı , ˚ gı )= ˚ g WLNCHM ˚ k (gı, ˚ gı ) ≤ WLNCHM ˚ k (g+ ı , ˚ g+ ı )= ˚ g+ .
4.EntropyofLNCSs
Entropyisusedtocontroltheunpredictabilityindifferentsetslikethefuzzyset (FS),intuitionistic fuzzyset (IFS),etc.In1965,Zadeh[37]firstdefinedtheentropyofFStodeterminetheambiguityina quantitativemanner.Thisnotionoffuzzinessplaysasignificantroleinsystemoptimization,pattern classification,controlandsomeotherareas.Healsogavesomepointsofitseffectsinsystemtheory. Recently,thenon-probabilisticentropywasaxiomatizedbyLucaetal.[38].Theintuitionisticfuzzy setsareintuitiveandhavebeenwidelyusedinthefuzzyliterature.Theentropy G ofafuzzyset H satisfiesthefollowingconditions,
1. G(H)= 0ifandonlyif H ∈ 2x;
2. G(H)= 1ifandonlyif µA (x)= 0.5, ∀x ∈ X;
3. G(H) ≤ G(ˆ ı) ifandonlyif H islessfuzzythan ˆ ı,i.e.,if µH (x) ≤ µı (x) ≤ 0.5, ∀x ∈ X orif µH (x) ≥ µˆ ı (x) ≥ 0.5, ∀x ∈ X; 4. G(HC )= G(H) Axioms1–4wereexpressedforfuzzysets(knownonlybytheirmembershipfunctions),and theyarestatedfortheintuitionisticfuzzysetsasfollows:
1. G(H)= 0ifandonlyif H ∈ 2x;(H non-fuzzy) 2. G(H)= 1ifandonlyif µH (x)= νH (x), ∀x ∈ X; 3. G(H) ≤ G(ˆ ı) ifandonlyif H islessthan ˆ ı,i.e.,if µH (x) ≤ µˆ ı (x) and νH (x) ≥ νi (x) for µˆ ı (x
DifferencesoccurinAxiom2and3.
Kaufmann[39]suggestedadistancemeasureofsoftentropy.Anewnon-probabilisticentropy measurewasintroducedbyKosko[40].In[41]MajumdarandSamantaintroducedthenotionoftwo single-valuedneutrosophicsets,theirpropertiesandalsodefinedthedistancebetweenthesetwosets. Theyalsoinvestigatedthemeasureofentropyofasingle-valuedneutrosophicset.TheentropyofIFSs wasintroducedbySzmidtandKacprzyk[42].Thefuzzinessmeasureintermsofdistancebetweenthe fuzzysetanditscomplimentwasputforwardbyYager[43].
The LNCS wasexaminedbymanagingundetermineddatawiththetruth,indeterminacyand falsitymembershipfunction.Fortheneutrosophicentropy,wewilltracetheKoskoideaforfuzziness calculation[40].Koskoproposedtomeasurethisinformationfeaturebyasimilarityfunctionbetween thedistancetothenearestcrispelementandthedistancetothefarthestcrispelement.Forneutrosophic information,werefertheworkbyPatrascu[45]wherehehasgiventhefollowingdefinitionincluding fromEquation(30)to(33).Itstatesthat:thetwocrispelementsare (1,0,0) and (0,0,1).Weconsider thefollowingvector: B = (µ ν, µ + ν 1, w) For (1,0,0) and (0,0,1),itresultsin BTru = (1,0,0) and BFal = ( 1,0,0) .Wewillnowcomputethedistancesasfollows: D (B, BTru ) = |µ ν 1| + |µ + ν 1| + w (30) D (B, BFal ) = |µ ν + 1| + |µ + ν 1| + w (31)
Theneutrosophicentropywillbedefinedbythesimilaritybetweenthesetwodistances. Thesimilarity Ec andneutrosophicentropy Vc aredefinedasfollows: Ec = 1 |D (B, BTru ) D (B, BFal ) | D (B, BTru ) + D (B, BFal ) (32) Vc = 1 |µ ν| 1| + |µ + ν 1| + w (33) Definition15. Supposethat H = xı, ˚ p(αH, ˚ αH)(xˆ ı ), ˚ p(βH, ˚ βH)(xı ), ˚ p(γH, ˚ γH)(xˆ ı ) | xı ∈ X isanLNCS; wedefinetheentropyofLNCSasafunction G˚ k : ˚ k(X) → [0, t], where t isanoddcardinalitywith t + 1 Thefollowingaresomeconditions.
1. G˚ k (H)= 0 îfHisacrispset;
2. G˚ k (H)=[1,1] ifandonlyif ˚ αH(x) t = ˚ βH(x) t = ˚ γH(x) t =[0.5,0.5] and G˚ k (H)= 1 ifandonlyif ˚ αH(x) t = βH(x) t = ˚ γH(x) t = 0.5, ∀x ∈ X;
˚ βHC (x) t ≥ ˚ βı (x) t )= G˚ k (H) Weneedtoconsiderthreefactorsfortheuncertainmeasureof LNCS; oneisthetruthmembership andfalsemembership,andtheotheristheindeterminacyterm.Wedefinetheentropymeasureof G˚ k ofan LNCSH,whichdependsonthefollowingterms: G˚ k (H)= 1 1 n ∑ x∈X
˚ βˆ ıC (x) t ˚ αH(x) t + ˚ γH(x) t
; ˚ βH (x) t
HC ˚ βHC (x) t =[0.5,0.5] [0.5,0.5]= 0, ˚ βH (x) t βHC (x) t = 0.5 0.5 = 0, ∀x ∈ X ⇒ Gk (H)= 1.
˚ ˚ βHC (x) t (34) Then,weprovethatEquation(34)canmeettheconditionofDefinition 15 Proof. 1. Foracrispset H,thereisnoindeterminacyfunctionforany LNCN of H.Hence, G˚ k (H)= 0issatisfied. 2. If H issuchthat ˚ αH(x) t = ˚ βH(x) t = ˚ γH(x) t =[0.5,0.5], ˚ αH(x) t , ˚ βH(x) t , ˚ γH(x) t = 0.5, ∀x ∈ X,then ˚ αH(x) t + ˚ γH(x) t =[1,1], ˚ αH(x) t + ˚ γH(x) t = 1 and ˚ βH (x) t
3. H islessuncertainthanI;weassume ˚ αH(x) t + ˚ γH(x) t ≥ ˚ α ˆ ı(x) t + ˚ γ ˆ ı(x) t , ˚ αH(x) t + ˚ γH(x) t ≥ ˚ α ˆ ı(x) t + ˚ γ ˆ ı(x) t and ˚ βH (x) t
˚ βHC (x) t ≥ ˚ βı (x) t
˚ βıC (x) t , ˚ βH (x) t
˚ βHC (x) t ≥ ˚ βı (x) t
˚ βıC (x) t Dependingonthe entropyvalueinEquation(34),wecanobtain Gk (H) ≤ Gk (ˆ ı) 4. HC = xˆ ı, ˚ pγH (xı ), ˚ pt βH (xı ), ˚ pαH (xı ), ˚ p ˚ γH (xı ), ˚ pt ˚ βH (xı ), ˚ p ˚ αH (xı ) | xˆ ı ∈ X , G˚ k (HC )= 1 1 n ∑x∈X ˚ γH (x) t + ˚ αH (x) t . ˚ βHC (x) t βH (x) t = G˚ k (H). Example6. Let ˚ p = { ˚ p0, ˚ p1, ˚ p2, ˚ p3, ˚ p4} bealinguistictermsetwithcardinality t + 1, ˚ g1 =( ˚ p3, ˚ p2, ˚ p1), ˚ g2 = ( ˚ p4, ˚ p3, ˚ p1, ), betwoLNCNsbasedon ˚ pandUbetheuniversalsetwhere: H = ([0.1,0.3], [0.4,0.5], [0.4,0.6], (0.4,0.6,0.7)) , ([0.1,0.2], [0.2,0.5], [0.1,0.4], (0.4,0.6,0.5))
isanLNCSinU.Then,theentropyofUwillbe: Gk (H)= 1 1 2 [0.1,0.3] 5 + [0.4,0.6] 5 [0.4,0.5] 5 5 [0.4,0.5] 5 + [0.1,0.2] 5 + [0.1,0.4] 5 . [0.1,0.4] 5 5 [0.1,0.4] 5
=[0.89,0.93]
5.TheMethodforMAGDMBasedontheWLNCHMOperator
Inthissection,wediscussMAGDM,basedontheWLNCHMoperatorwith LNCN Let U = {U1, U2,..., Um } bethesetofalternatives, V = {V1, V2,..., Vn } bethesetofattributes and ˚ w = ( ˚ w1, ˚ w2,..., ˚ wn )T betheweightvector.Then,by LNCNsandfromthepredefinedlinguistic termset ϕ = { ϕj | j ∈ [0, t]} (where t + 1 isanoddcardinality),thedecisionmakersareinvited toevaluatethealternatives Uı (ˆ ı = 1,2, , m) overtheattributes Vj (j = 1,2, , n) The DMs can assigntheuncertain LTS tothetruth,indeterminacyandfalsitylinguistictermsandthecertain LTS tothetruth,indeterminacyandfalsitylinguistictermsineach LNCNs,whichisbasedonthe LTS intheevaluationprocessofthelinguisticevaluationofeachattribute Vj (j = 1,2, , n) on eachalternative Uı (ˆ ı = 1,2, ... , m). Thus,weobtainthedecisionmatrix S =(sıj )m × n, ˚ gıj , ˚ gıj = ( ˚ pαıj , ˚ pβıj , ˚ pγıj , ˚ pαıj , ˚ pβıj , ˚ pγıj )(ˆ ı = 1,2,..., m; j = 1,2,..., n) asan LNCN Basedontheaboveinformation,theMAGDMontheWLNCMoperatorisdescribedasfollows: Step1:Regulatethedecisionmakingproblem. Step2:Calculate ˚ gˆ ıj = WLNCM(sˆ ı1, sˆ ı2, , sˆ ın ) toobtainthecollectiveapproximationvaluefor alternatives Uˆ ı withrespecttoattribute Vj.
Step3:Inthisstep,weoperatetheentropyofLNCSstofindouttheweightoftheelements. ˚ gj =( ˚ p(αj , ˚ αj ), ˚ p(βj , ˚ βj ), ˚ p(γj , ˚ γj ))
Step4:Inthisstep,wecalculatethevaluesofthescorefunction ϕ(S),accuracyfunction Φ(S) andcertainfunction Ψ(S) basedonEquations(12)–(14).
Step5:Inthisstep,wefindoutthesequenceofthealternatives Uı (ˆ ı = 1,2, ... , m) .Accordingto therankingorderofDefinition 8,withagreaterscorefunction ϕ(S),therankingorderofalternatives Uı isthebest.Ifthescorefunctionsarethesame,thentheaccuracyfunctionofalternativesUı islarger, andthen,therankingorderofalternativesUi isbetter.Furthermore,ifthescoreandaccuracyfunction botharethesame,thenthecertainfunctionofalternatives Uı islarger,andthen,therankingorderof alternativesUı isbest.
Step6:End.
6.NumericalApplications
Acorporationintendstochooseonepersontobetheareasupervisorfromfivecandidates (U1 U4),tobefurtherevaluatedaccordingtothethreeattributes,whichareshownasfollows: ideologicalandmoralquality (V1),professionalability (V2) andcreativeability (V3).Theweightsof theindicatorsare ˚ w =(0.5,0.3,0.2).
6.1.Procedure
([0.4,0.5], [0.1,0.2], [0.3,0.6], (0.6,0.3,0.7))
([0.3,0.5], [0.6,0.7], [0.4,0.6], (0.6,0.8,0.7))
U4
U
([0.4,0.6], [0.1,0.3], [0.3,0.5], (0.7,0.4,0.6))
0.7,0.8], [0.6,0.8], (0.8,0.9,1.0))
([0.2,0.4], [
([0.4,0.7], [
([0.3,0.4], [0.6,0.7], [0.5,0.6], (0.5,0.8,0.7))
([0.4,0.5], [0.7,0.9], [0.1,0.4], (0.6,1.0,0.8))
([0.2,0.3], [0.4,0.5], [0.7,0.8], (0.4,0.6,0.9))
([0.4,0.5], [0.1,0.2], [
0.6,0.3,0.7))
([0.1,0.3], [0.4,0.6], [0.2,0.5], (0.4,0.7,0.6))
U4
0.055,0.084]
[0.095,0.131], (0.139,0.101, 0.142
0.146,0.159
0.119,0.159
(0.149,0.169, 0.175
([0.101,0.119], [0.115,0.127], [0.110,0.135], (0.127,0.156, 0.142))
([0.110,0.135], [0.146,0.162], [0.055,0.115], (0.146,0.172, 0.142
([0.078,0.110], [0.110,0.135], [0.146,0.159], (0.123,0.110, 0.169))
([0.071,0.110], [0.055,0.149], [0.142,0.162], (0.123,0.159, 0.172
0.055,0.135
(0.142,0.156, 0.156
0.101,0.139
0.115,0.156
0.172,0.149, 0.169
0.123,0.131], [0.105,0.135], [0.123,0.153], (0.142,0.153, 0.165))
0.078,0.135], [0.139,0.146], (0.110,0.146, 0.159))
0.110,0.153]
[0.101,0.110], (0.123,0.162, 0.159
([0.078,0.135], [0.078,0.139], [0.055,0.149], (0.149,0.159, 0.165))
)) bethe LNCN and G˚ k (sj ) betheweightofattributes,i.e.,
G˚ k (sj )= 1 1 m ∑ x∈X
αSj (x) t + ˚ γSj (x) t
Step4:BytheWLNCHMoperator,wecalculatethecomprehensiveevaluationvalueofeach alternativeas:
U1 = ([0.132,0.182], [0.140,0.174], [0.127,0.192], (0.199,0.189,0.212))
U2 = ([0.128,0.186], [0.147,0.184], [0.141,0.187], (0.174,0.207,0.199))
U3 = ([0.093,0.153], [0.117,0.190], [0.147,0.191], (0.200,0.195,0.205))
U4 = ([0.103,0.121], [0.133,0.162], [0.152,0.171], (0.160,0.181,0.175))
Step5:Wefindthevaluesofscorefunction ϕ(S) as:
ϕ(S)= 1 9t [ 4t + ˚ α ˚ β ˚ γ + 2t + ˚ α ˚ β ˚ γ ],for ϕ(S) ∈ [0,1]
ϕ(S1)= 1 45 [20 + 0.13 + 0.2 (0.14 + 0.2 + 0.13 + 0.2) + 10 + 0.2 (0.2 + 0.21)] = 654
ϕ(S2)= 1 45 [20 + 0.2 + 0.2 (0.15 + 0.2 + 0.14 + 0.2) + 10 + 0.2 (0.2 + 0.2)] = 0.656
ϕ(S3)= 1 45 [20 + 0.1 + 0.2 (0.12 + 0.2 + 0.15 + 0.2) + 10 + 0.2 (0.2 + 0.21)] = 0.653
ϕ(S4)= 1 45 [20 + 0.1 + 0.1 (0.1 + 0.2 + 0.2 + 0.2) + 10 + 0.2 (0.2 + 0.2)] = 0.657
Step6:Accordingtothevalueofthescorefunction,therankingofthecandidatescanbeconfirmed, i.e., S4 S2 S1 S3.,so S4 isthebestalternatives.
Case2:IftheDMgivestheinformationabouttheattributesandweightandtheweightvector is ˚ w =(0.1,0.5,0.4),thenthescorefunction ϕ(Sˆ ı )(ˆ ı = 1,2,3,4) ofCase2canbeobtainedasfollows; ϕ(S1)= 0.451, ϕ(S2)= 0.435, ϕ(S3)= 0.504, ϕ(S4)= 0.492. Therankingofthesescorefunctionsis S3 S4 S1 S2 Thu,sduetothediverseweightsofattributes,therankingofCase2isdifferent fromthatofCase1.
IntheMADMmethod,theattributeweightscanreturnrelativevaluesinthedecisionmethod. However,duetotheissuessuchasdataloss,timepressureandincompletefieldknowledgeoftheDMs, theinformationaboutattributeweightsisnotfullyknownorcompletelyunknown.Throughsome methods,weshouldderivetheweightvectorofattributestogetpossiblealternatives.InCase2,the attributeweightsareusuallydeterminedbasedonDMs’opinionsorpreferences,whileCase1uses theentropyconceptstodetermineweightvaluesofattributestosuccessfullybalancethemanipulation ofsubjectivefactors.Therefore,theentropyofLNCSisappliedinthedecisionprocesstogiveeach attributeamoreobjectiveandreasonableweight.
6.2.ComparisonAnalysis
Fromthecomparisonanalysis,onecanseethattheadvancedmethodismoreappropriate forarticulatingandhandlingtheindeterminateandinconsistentinformationinlinguisticdecision makingproblemstoovercometheinsufficiencyofseverallinguisticdecisionmakingmethodsinthe existingwork.Infact,mostofthedecisionmakingproblemsbasedondifferentlinguisticvariables intheliteraturenotonlyexpressinconsistentandindeterminatelinguisticresults,butthelinguistic methodsuggestedinthestudyisageneralizationofexistinglinguisticmethodsandcanhandle andrepresentlinguisticdecisionmakingproblemswithLNNinformation.Wealsoseethatthe advancedmethodhasmuchmoreinformationthantheexistingmethodin[26,32,44].Inaddition, theliterature[26,32,44]isthesameasthebestandworstanddifferentfromourmethods.Thereason forthedifferencebetweenthegivenliteratureandourmethodmaybethedecisionthoughtprocess.
Someinitialinformationmaybemissingduringtheaggregationprocess.Moreover,theconclusions aredifferent.Differentaggregationoperatorsmayappear[32],andourmethodsareconsistentwith theaggregationoperatorandreceiveadifferentorder.However,[32]mayhavesomelimitations becauseoftheattributes.Theweightvectorisgivendirectly,andthepositiveandnegativeideal solutionsareabsolute. Otherthanthis, theranking intheliterature[26,32,44]isdifferentfromthe proposedmethod.ThereasonforthedifferencemaybeuncertaintyinLNNmembershipsincethe informationisinevitablydistortedinLIFN.Ourmethoddevelopstheneutrosophiccubictheoryand decisionmakingmethodunderalinguisticenvironmentandprovidesanewwayforsolvinglinguistic MAGDMproblemswithindeterminateandinconsistentinformation.
7.Conclusions
Inthispaper,weworkouttheideaof LNCNs,theiroperationallawsandalsosomeproperties anddefinethescore,accuracyandcertainfunctionsof LNCNsforranking LNCNs.Then,wedefine theLNCHMandWLNCHMoperators.Afterthat,wedemonstratetheentropyof LNCNsandrelate ittodeterminetheweights.Next,wedevelopMAGDMbasedonWLNCHMoperatorstosolve multi-attributegroupdecisionmakingproblemswith LNCN information.Finally,weprovidean exampleofthedevelopedmethod.
AuthorContributions: Alltheauthorsofthispapercontributedequally.Theyhavereadandapprovedthefinal versionofthepaper.Inparticular:conceptualization,M.G.;supervision,M.G.;communication,M.G.;formatting, H.A.W.;revisions,H.A.W.;projectadministration,F.S.;writingtheoriginaldraft,S.K.;finalproofreading,S.I.A.S.
Acknowledgments: Wewouldliketoexpressoursincerethankstotheanonymousrefereesofthispaperfortheir interestinourworkandalsoforspendingtheirvaluabletimeinreadingthismanuscriptcarefullyandgiving theirusefulcommentsforimprovingtheearlierversionofthepaper.
ConflictsofInterest: Theauthorsdeclarenoconflictofinterest.
References
1. Zadeh,L.A.Fuzzysets. Inform.Control 1965, 8,338–353.[CrossRef]
2. Atanassov,K.T.Intuitionisticfuzzysets. FuzzySetsSyst. 1986, 20,87–96.[CrossRef]
3. Jun,Y.B.;Kim,C.S.;Yang,K.O.Cubicsets. Ann.FuzzyMath.Inform. 2012, 4,83–98.
4. Akram,M.;Yaqoob,N.;Gulistan,M.CubicKU-subalgebras. Int.J.PureAppl.Math. 2013, 89,659–665. [CrossRef]
5. Yaqoob,N.;Mostafa,S.M.;Ansari,M.A.OncubicKU-idealsofKU-algebras. ISRNAlgebra 2013, doi10.1155/2013/935905.[CrossRef]
6. Rashid,S.;Yaqoob,N.;Akram,M.;Gulistan,M.Cubicgraphswithapplication. Int.J.Anal.Appl. 2018, 16, 733–750.
7. Aslam,M.;Aroob,T.;Yaqoob,N.Oncubic Γ-hyperidealsinleftalmost Γ-semihypergroups. Ann.FuzzyMath. Inform. 2013, 5,169–182.
8. Gulistan,M.;Yaqoob,N.;Vougiouklis,T.;Wahab,H.A.Extensionsofcubicidealsinweakleftalmost semihypergroups. J.Intell.FuzzySyst. 2018, 34,4161–4172.[CrossRef]
9. Gulistan,M.;Khan,M.;Yaqoob,N.;Shahzad,M.Structuralpropertiesofcubicsetsinregular LA-semihypergroups. FuzzyInf.Eng. 2017, 9,93–116.[CrossRef]
10. Khan,M.;Gulistan,M.;Yaqoob,N.;Hussain,F.GeneralcubichyperidealsofLA-semihypergroups. Afr.Mat. 2016, 27,731–751.[CrossRef]
11. Yaqoob,N.;Gulistan,M.;Leoreanu-Fotea,V.;Hila,K.CubichyperidealsinLA-semihypergroups. J.Intell. FuzzySyst. 2018, 34,2707–2721.[CrossRef]
12. Khan,M.;Jun,Y.B.;Gulistan,M.;Yaqoob,N.ThegeneralizedversionofJun’scubicsetsinsemigroups. J. Intell.FuzzySyst. 2015, 28,947–960.
13. Khan,M.;Gulistan,M.;Yaqoob,N.;Shabir,M.Neutrosophiccubic (α, β)-idealsinsemigroupswith application. J.Intell.FuzzySyst. 2018, 35,2469–2483.[CrossRef]
14. Ma,X.L.;Zhan,J.;Khan,M.;Gulistan,M.;Yaqoob,N.GeneralizedcubicrelationsinHv-LA-semigroups. J. Discret.Math.Sci.Cryptgr. 2018, 21,607–630.[CrossRef]
Symmetry 2018, 10,428 29of30
15. Gulistan,M.;Khan,M.;Yaqoob,N.;Shahzad,M.;Ashraf,U.Directproductofgeneralizedcubicsetsin Hv-LA-semigroups. Sci.Int. 2016, 28,767–779.
16. Smarandache,F. AUnifyingFieldinLogics:NeutrosophicLogic.Neutrosophy,NeutrosophicSet,Neutrosophic Probability;AmericanResearchPress:Rehoboth,NM,USA,1999.
17. Smarandache,F.Neutrosophicset,ageneralizationoftheintuitionisticfuzzyset. Int.J.PureAppl.Math. 2005, 24,287–297.
18. Wang,H.;Smarandache,F.;Zhang,Q.Y.;Sunderraman,R. SingleValuedNeutrosophicSets;InfiniteStudy: NewDelhi,India,2010.
19. De,S.K.;Beg,I.Triangulardensefuzzyneutrosophicsets. NeutrosophicSetsSyst. 2016, 13,25–38.
20. Gulistan,M.;Khan,A.;Abdullah,A.;Yaqoob,N.Complexneutrosophicsubsemigroupsandideals. Int.J. Anal.Appl. 2018, 16,97–116.
21. Jun,Y.B.;Smarandache,F.;Kim,C.S.Neutrosophiccubicsets. NewMath.Nat.Comput. 2017, 13,41–54. [CrossRef]
22. Jun,Y.B.;Smarandache,F.;Kim,C.S.P-unionandP-intersectionofneutrosophiccubicsets. Anal.Univ. OvidiusConstanta 2017, 25,99–115.[CrossRef]
23. Gulistan,M.;Yaqoob,N.;Rashid,Z.;Smarandache,F.;Wahab,H.Astudyonneutrosophiccubicgraphswith reallifeapplicationsinindustries. Symmetry 2018, 10,203.[CrossRef]
24. Zhan,J.;Khan,M.;Gulistan,M.;Ali,A.Applicationsofneutrosophiccubicsetsinmulti-criteria decision-making. Int.J.Uncertain.Quantif. 2017, 7,377–394.[CrossRef]
25. Hashim,R.M.;Gulistan,M.;Smrandache,F.ApplicationsofneutrosophicbipolarfuzzysetsinHOPE foundationforplanningtobuildachildrenhospitalwithdifferenttypesofsimilaritymeasures. Symmetry 2018, 10,331.[CrossRef]
26. Zadeh,L.A.TheconceptofalinguisticvariableanditsapplicationtoapproximatereasoningPartI. Inf.Sci. 1975, 8,199–249.[CrossRef]
27. Herrera,F.;Herrera-Viedma,E.;Verdegay,L.Amodelofconsensusingroupdecisionmakingunderlinguistic assessments. FuzzySetsSyst. 1996, 79,73–87.[CrossRef]
28. Herrera,F.;Herrera-Viedma,E.linguisticdecisionanalysis:Stepsforsolvingdecisionproblemsunder linguisticinformation. FuzzySetsSyst. 2000, 115,67–82.[CrossRef]
29. Xu,Z.S.Amethodbasedonlinguisticaggregationoperatorsforgroupdecisionmakingwithlinguistic preferencerelations. Inf.Sci. 2004, 166,19–30.[CrossRef]
30. Chen,Z.C.;Liu,P.H.;Pei,Z.Anapproachtomultipleattributegroupdecisionmakingbasedonlinguistic intuitionisticfuzzynumbers. Int.J.Comput.Intell.Syst. 2015, 8,747–760.[CrossRef] 31. Zhang,H.LinguisticintuitionisticfuzzysetsandapplicationinMAGDM. J.Appl.Math. 2014.[CrossRef] 32. Fang,Z.B.;Ye,J.Multipleattributegroupdecision-makingmethodbasedonlinguisticneutrosophicnumbers. Symmetry 2017, 9,111.[CrossRef] 33. Peng,H.G.;Wang,J.Q.;Cheng,P.F.Alinguisticintuitionisticmulti-criteriadecision-makingmethodbased ontheFrankHeronianmeanoperatoranditsapplicationinevaluatingcoalminesafety. Int.J.Mach.Learn. Cybern. 2017, 9,1053–1068.[CrossRef] 34. Ye,J.Aggregationoperatorsofneutrosophiclinguisticnumbersformultipleattributegroupdecisionmaking. SpringerPlus 2016, 5,1691.[CrossRef][PubMed] 35. Li,Y.Y.;Zhang,H.;Wang,J.Q.Linguisticneutrosophicsetsandtheirapplicationinmulticriteria decision-makingproblems. Int.J.Uncertain.Quantif. 2017, 7.[CrossRef] 36. Hara,T.;Uchiyama,M.;Takahasi,S.E.Arefinementofvariousmeaninequalities. J.Inequal.Appl. 1998, 4, 932025.[CrossRef] 37. Zadeh,L.A.Fuzzysetsandsystems. Int.J.Gen.Syst. 1990, 17,129–138.[CrossRef] 38. DeLuca,A.;Termini,S.Adefinitionofanonprobabilisticentropyinthesettingoffuzzysetstheory. Inf.Control 1972, 20,301–312.[CrossRef] 39. Kaufmann,A.;Bonaert,A.P.Introductiontothetheoryoffuzzysubsets-vol.1:Fundamentaltheoretical elements. IEEETrans.Syst.ManCybern. 1977, 7,495–496.[CrossRef] 40. Kosoko,B.Fuzzyentropyandconditioning. Inf.Sci. 1986, 40,165–174.[CrossRef] 41. Majumdar,P.;Samanta,S.K.Softnessofasoftset:Softsetentropy. Ann.FuzzyMath.Inf. 2013, 6,59–68. 42. Szmidt,E.;Kacprzyk,J.Entropyforintuitionisticfuzzysets. FuzzySetsSyst. 2001, 118,467–477.[CrossRef]
43. Yager,R.R.Onthemeasureoffuzzinessandnegation,PartI:Membershipintheunitinterval. Int.J.Gen.Syst. 1979, 5,189–200.[CrossRef]
44. Ye,J.AnextendedTOPSISmethodformultipleattributegroupdecisionmakingbasedonsinglevalued neutrosophiclinguisticnumbers. J.Intell.FuzzySyst. 2015, 28,247–255. 45. Patrascu,V.Theneutrosophicentropyanditsfivecomponents. NeutrosophicSets.Syst. 2015, 7,40–46. 46. Liu,P.;You,X.SomelinguisticneutrosophicHamymeanoperatorsandtheirapplicationtomulti-attribute groupdecisionmaking. PLoSONE 2018, 13,e0193027.[CrossRef][PubMed]
47. Ye,J.Linguisticneutrosophiccubicnumbersandtheirmultipleattributedecision-makingmethod. Information 2017, 8,110.[CrossRef]
c 2018bytheauthors.LicenseeMDPI,Basel,Switzerland.Thisarticleisanopenaccess articledistributedunderthetermsandconditionsoftheCreativeCommonsAttribution (CCBY)license(http://creativecommons.org/licenses/by/4.0/).
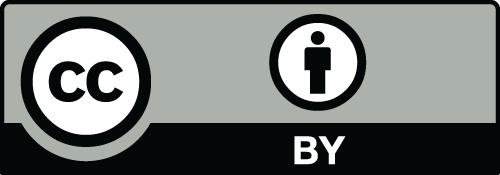