FuzzyParameterizedComplexNeutrosophicSoft ExpertSetforDecisionunderUncertainty
AshrafAl-Quran 1 ,NasruddinHassan 2,* andShawkatAlkhazaleh 3
1 DepartmentofMathematics,FacultyofScience,JerashUniversity,Jerash26150,Jordan;a.quraan@jpu.edu.jo
2 SchoolofMathematicalSciences,FacultyofScienceandTechnology,UniversitiKebangsaanMalaysia, 43600UKMBangiSelangorDE,Malaysia
3 DepartmentofMathematics,FacultyofScience,ZarqaUniversity,Zarqa13132,Jordan;shmk79@gmail.com
* Correspondence:nas@ukm.edu.my;Tel.:+603-8921-3710
Received:5January2019;Accepted:26February2019;Published:15March2019
Abstract: Inthedefinitionofthecomplexneutrosophicsoftexpertset(CNSES),parametersset isaclassicalset,andtheparametershavethesamedegreeofimportance,whichisconsidered as1.Thisposesalimitationinmodelingofsomeproblems.Thispaperintroducestheconcept offuzzyparameterizedcomplexneutrosophicsoftexpertset(FP-CNSES)tohandlethisissueby assigningadegreeofimportancetoeachoftheproblemparameters.WefurtherdevelopFP-CNSES byestablishingtheconceptofweightedfuzzyparameterizedcomplexneutrosophicsoftexpertset (WFP-CNSES)basedontheideathateachexperthasarelativeweight.Thesenewmathematical frameworksreducethechanceofunfairnessinthedecisionmakingprocess.Someessentialoperations withtheirpropertiesandrelevantlawsrelatedtothenotionofFP-CNSESaredefinedandverified. Thenotationofmappingonfuzzyparameterizedcomplexneutrosophicsoftexpertclassesisdefined andsomepropertiesoffuzzyparameterizedcomplexneutrosophicsoftexpertimagesandinverse imageswasinvestigated.FP-CNSESisusedtoputforthanalgorithmondecision-makingby convertingitfromcomplexstatetorealstateandsubsequentlyprovidedthedetaileddecisionsteps. Then,weprovidethecomparisonofFP-CNSEStothecurrentmethodstoshowtheascendancyof ourproposedmethod.
Keywords: complexneutrosophicset;complexneutrosophicsoftexpertset;fuzzyparameterized singlevaluedneutrosophicsoftexpertset;singlevaluedneutrosophicset;softexpertset
1.Introduction
Inaworldwherenoteverythingiscertain,theneedtorepresentuncertaindatawassuccessfully fulfilledbyZadeh[1]byintroducingtheconceptoffuzzysets.Intuitionisticfuzzysetswereintroduced byAtanassov[2]asanextensionofZadeh’snotionoffuzzyset,whichprovedtobeabettermodel ofuncertainty.Thewords“neutrosophy”and“neutrosophic”wereintroducedforthefirsttimeby Smarandache[3].Then,neutrosophicset[4]wasdefinedasamoregeneralplatform,whichextendsthe conceptsofthefuzzysetandintuitionisticfuzzyset.Toapplyneutrosophicsettoreal-lifeproblems, itsoperatorsneedtobespecified.Thus,singlevaluedneutrosophicsetanditsbasicoperationswere defined[5]asaspecialcaseofneutrosophicset.Molodtsov[6]proposedtheconceptofsoftset, tobringatopologicalflavortothemodelsofuncertaintyandassociatefamilyofsubsetsofuniverseto parameters.Softsetwasthenextendedtothesoftexpertset[7],whichwasfurtherdevelopedtofuzzy softexpertset(FSES)[8],intuitionisticfuzzysoftexpertsets(IFSES)[9]andsinglevaluedneutrosophic softexpertsets(SVNSES)[10].Atthesametime,therehavebeensomepracticalapplicationsinsoft expertsettheoryanditsextensionsthatareusedindecisionmaking[11–13].
Symmetry 2019, 11,382;doi:10.3390/sym11030382 www.mdpi.com/journal/symmetry
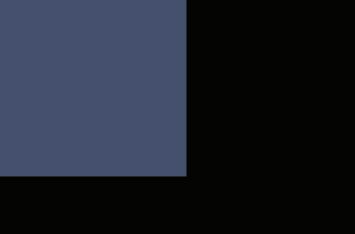
Amongthesignificantmilestonesinthedevelopmentofsoftsetsandsoftexpertsetsandtheir generalizationsistheintroductionofthefuzzyparameterizedaspect.Thisnewaspecthasfurther improvedthetheoriesofsoftandsoftexpertsetsandmadethembettersuitedtobeusedinsolving decisionmakingproblems,especiallywhenusedwiththemoreaccurategeneralizationsofsoft andsoftexpertsetssuchasFSESs,IFSESsandotherhybridmodelsmentionedabove.Thefuzzy parameterizedaspectwasfirstlyestablishedbyCagmanetal.[14]whointroducedtheconceptof fuzzyparameterizedsoftsetbygivingadegreeofimportancetoeachelementinthesetofparameters. Subsequently,thisaspectwasattachedand/oraddedtotheexistinggeneralizationsofthesoftsets, softexpertsetsandfuzzysets.BashirandSalleh[15]introducedthenotionoffuzzyparameterized softexpertsets(FP-SES),whileHazaymehetal.[16]thenotionoffuzzyparameterizedfuzzysoft expertsets(FP-FSES),followedbySelvachandranandSalleh[17]whointroducedthenotionof fuzzyparameterizedintuitionisticfuzzysoftexpertset(FP-IFSES)asageneralizationoftheworkby Hazaymehetal.[16].However,thesesetscanonlyhandleincompleteanduncertaintyinformationbut notindeterminateandinconsistentinformation,whichusuallyexistsinrealsituations.Totreatthis deficiency,Al-QuranandHassan[18]definedthefuzzyparameterizedsinglevaluedneutrosophicsoft expertset(FP-SVNSES),whichprovessuperiortothesemodelswiththreeindependentmembership functions.TheFP-SVNSESmodelisalsosignificantlymoreadvantageouscomparedtoSVNSES, asithasaddedadvantagestoSVNSESbyvirtueofthefuzzyparameterizedfeature,whichprovides moreinformationenhancingthequalityoftheinformationpresentedbytheSVNSES,whichinturn, increasestheaccuracyofthefinaldecision.
Thedevelopmentoftheuncertaintysetsthathavebeenmentionedabovearenotlimitedtothe realfieldbutextendedtothecomplexfield.Theintroductionoffuzzysetswasfollowedbytheir extensiontothecomplexfuzzyset[19,20].AlkouriandSalleh[21]introducedtheconceptofcomplex intuitionisticfuzzyset(CIFS)torepresentinformationthathappensrepeatedlyoveraperiodoftime. Tohandleimprecise,indeterminate,inconsistent,andincompleteinformationthathasperiodicnature, AliandSmarandache[22]introducedcomplexneutrosophicset,whereeachmembershipfunction associateswithaphaseterm.Thisfeaturegiveswave-likepropertiesthatcouldbeusedtodescribe constructiveanddestructiveinterferencedependingonthephasevalueofanelement,aswellas itsabilitytodealwithindeterminacy.Inspiredbythis,Al-QuranandHassan[23]generalizedthe CNSESfromthedefinitionsofthecomplexneutrosophicsetandsoftexpertsetonthebasisofthe SVNSES.Indepth,therationalesofintroducingthecomplexneutrosophicsetandthesoftexpertset areconsideredasapotentmotivationtotheintroductionoftheconceptofCNSES.CNSESisactually anextensionoftheSVNSEStothecomplexspace,whichmakesitsuperiortoalloftheaforementioned uncertaintysets.Subsequently,Al-QuranandHassan[24]studiedtheCNSESsfurtherbyestablishing anovelstructureofrelationbetweentwoCNSESs,calledcomplexneutrosophicsoftexpertrelation, toevaluatethedegreeofinteractionbetweentheCNSESs,whichisdefinedasasubsetoftheCartesian productoftheCNSESs.
Overtheyears,manytechniquesandmethodshavebeenproposedastoolstobeusedtofind thesolutionsofproblemsthatarenonlinearorvagueinnature,witheverymethodintroduced superiortoitspredecessors.Followinginthisdirection,weextendthestudiesonCNSESs[23]and FP-SVNSES[18]throughtheestablishmentofthenotionofFP-CNSEStokeeptheadvantagesofCNSES whileholdingtheFP-SVNSESfeatures.Ontheonehand,thenoveltyofCNSESappearsinitsability toprovideasuccinct,elegantandcomprehensiverepresentationoftwo-dimensionalneutrosophic informationaswellastheadequateparameterizationandtheopinionsoftheexperts,allinasingleset. Thistwo-dimensionalinformationispresentedbyamplitudeandphasetermssimultaneouslywhere thephasetermsgiveneutrosophicinformationthatmayinterfere,constructivelyordestructively, withtheneutrosophicinformationpresentedbytheassociatedamplitudeterms,thusmakingthis modelhighlysuitableforuseindecisionmakingproblemstoselectthebestalternative.Ontheother hand,FP-SVNSEShasthefuzzyparameterizedfeature,whichgivesadegreeofimportancetoeach
parameterinthedomainoftheSVNSES.Allofthesefeaturestogetherarecontainedintheproposed FP-CNSES.
Tofacilitateourdiscussion,wefirstreviewsomebackgroundoncomplexneutrosophicsetand FP-SVNSESinSection 2.InSection 3,weintroducetheconceptofFP-CNSESandgiveitstheoretic operations.InSection 4,westudyamappingonfuzzyparameterizedcomplexneutrosophicsoftexpert classesanditsproperties.InSection 5 wediscussanapplicationoftheFP-CNSESindecisionmaking. Section 6 providesacomprehensivecomparisonamongFP-CNSESandotherrecentapproaches tomanifestthedominanceofourproposedmethod.InSection 7,wedefinetheconceptofthe WFP-CNSESwhereexperts’relativeweightsareconsideredandappliedtosolveadecisionmaking problem.Section 8 outlinestheconclusionofthispaper.
2.Preliminaries
Inthissection,asummaryoftheliteratureoncomplexneutrosophicsetandFP-SVNSESrelevant tothispaperispresented.
Webeginbyrecallingthedefinitionofcomplexneutrosophicsetanditsbasicoperationsinthe followingtwodefinitions.
Definition1. [22]Let X beauniverseofdiscourse;acomplexneutrosophicset S in X ischaracterizedby atruthmembershipfunction TS (x),anindeterminacymembershipfunction IS (x),andafalsitymembership function FS (x) thatassignanelement x ∈ X acomplex-valuedgradeof TS (x), IS (x),and FS (x) in S Bydefinition,thevalues TS (x), IS (x),and FS (x) andtheirsummayallbewithintheunitcircleinthe complexplaneandareoftheform, TS (x)= pS (x) ejµS (x) , IS (x)= qS (x) ejνS (x) and FS (x)= rS (x) ejωS (x); pS (x), qS (x), rS (x) and µS (x), νS (x), ωS (x) are,respectively,realvaluedand pS (x), qS (x), rS (x) ∈ [0,1] suchthat 0 ≤ PS (x)+ qS (x)+ rS (x) ≤ 3+ .
Definition2. [22]Let A and B betwocomplexneutrosophicsets,where A ischaracterizedbyatruth membershipfunction TA (x)= pA (x).ejµA (x),anindeterminacymembershipfunction IA (x)= qA (x).ejνA (x) andafalsitymembershipfunction FA (x)= rA (x).ejωA (x) and B ischaracterizedbyatruthmembership function TB (x)= pB (x) ejµB (x),anindeterminacymembershipfunction IB (x)= qB (x) ejνB (x) andafalsity membershipfunctionFB (x)= rB (x) ejωB (x) Wedefinethethecomplement,subset,unionandintersectionoperationsasfollows.
1. ThecomplementofA,denotedas ˜ c(A),isspecifiedbyfunctions:
Tc(A) (u)= pc(A) (u) ejµc(A) (u) = rA (u) ej(2π µA (u)) , Ic(A) (u)= qc(A) (u).ejνc(A) (u) =(1 qA (u)).ej(2π νA (u)),and Fc(A) (u)= rc(A) (u) ejωc(A) (u) = pA (u) ej(2π ωA (u))
2. A issaidtobecomplexneutrosophicsubsetof B (A ⊆ B)ifandonlyifthefollowingconditionsaresatisfied:
(a)TA (u) ≤ TB (u) suchthatpA (u) ≤ pB (u) and µA (u) ≤ µB (u). (b)IA (u) ≥ IB (u) suchthatqA (u) ≥ qB (u) and νA (u) ≥ νB (u). (c)FA (u) ≥ FB (u) suchthatrA (u) ≥ rB (u) and ωA (u) ≥ ωB (u).
Al-QuranandHassan[18]definedtheFP-SVNSES,agreeFP-SVNSESanddisagreeFP-SVNSES asfollows.
Definition3. [18]Let U beauniverseset, E beasetofparameters, I E denoteallfuzzysubsetsof E, X be asetofexperts,and O = {1 = agree,0 = disagree} asetofopinions.Let Z = Ψ × X × O and A ⊆ Z, where Ψ ⊂ I E.Apair ( f , A)Ψ iscalledafuzzyparameterizedsinglevaluedneutrosophicsoftexpertset (FPSVNSES) overU,whereFisamappinggivenby fΨ : A → SVN(U), andSVN(U) denotesthesetofallsinglevaluedneutrosophicsubsetsofU.
Definition4. AnagreeFP-SVNSES ( f , A)Ψ1 over U isaFP-SVNSEsubsetof ( f , A)Ψ wheretheopinionsof allexpertsare“agree”andisdefinedasfollows:
( f , A)Ψ1 = FΨ ( ) : ∈ Ψ × X ×{1} .
AdisagreeFP-SVNSES ( f , A)Ψ0 over U isaFP-SVNSEsubsetof ( f , A)Ψ wheretheopinionsofall expertsare“disagree”andisdefinedasfollows:
( f , A)Ψ0 = FΨ ( ) : ∈ Ψ × X ×{0} .
3.FuzzyParameterizedComplexNeutrosophicSoftExpertSet
Inthissection,weintroducethedefinitionofFP-CNSES,whichisageneralizationoftheconcept ofFP-SVNSES.Wedefinesomeoperationsonthisconcept,namelysubset,equality,complement, unionandintersection.Wealsogivesomepropertiesontheseoperations.
WebeginbyproposingthedefinitionofFP-CNSES,andgiveanillustrativeexampleofit.
Definition5. Let U beauniverseset, E beasetofparameters, FZ(E) denoteallfuzzysubsetsof E, X bea setofexperts,and O = {1 = agree,0 = disagree} asetofopinions.Let Y = Γ × X × O and A ⊆ Y where Γ ⊂ FZ(E).Apair (H, A)Γ iscalledafuzzyparameterizedcomplexneutrosophicsoftexpertset(FP-CNSES) overU,whereHisamappinggivenby HΓ : A → CNU , andCNU denotesthepowercomplexneutrosophicsetofU. TheFP-CNSES (H, A)Γ canbewrittenasthefollowingsetoforderedpairs:
H,
Γ = a, u HΓ (a)(u) : u
U : a ∈ A , where A ⊆
, o :e
, x
(e) ,
and o ∈ O , suchthat µΓ (e) isthecorrespondingmembershipfunctionofthefuzzyset Γ and ∀u ∈ U, ∀a ∈ A, H
(a
, where
H
(a) (u)= pHΓ (a) (u).e
H
(a) (u) , IH(a) (u)= qH
Γ (a) (u) and FHΓ (a) (u)= rHΓ (a) (u) ejωHΓ (a) (u) with THΓ (a) (u), IHΓ (a) (u) and FHΓ (a) (u) representingthecomplex-valuedtruthmembershipfunction,complex-valuedindeterminacy membershipfunctionandcomplex-valuedfalsitymembershipfunction,respectively,forthe FP-CNSES (H, A)Γ.Thevalues THΓ (a) (u), IHΓ (a) (u), FHΓ (a) (u) arewithintheunitcircleinthe complexplaneandboththeamplitudeterms pHΓ (a) (u), qHΓ (a) (u), rHΓ (a) (u) andthephaseterms µHΓ (a) (u), νHΓ (a) (u), ωHΓ (a) (u) arerealvaluedsuchthat pHΓ (a) (u), qHΓ (a) (u), rHΓ (a) (u) ∈ [0,1] and0 ≤ pHΓ (a) (u)+ qHΓ (a) (u)+ rHΓ (a) (u) ≤ 3.
Example1. Supposethatacarcompanyproducestwodifferentmodelsofcarsandwishtotaketheopinionof itsteamofexpertsconcerningthesetwomodelsofcarsbeforeandaftertestingthesecars.Thesetwomodelsof carsformtheuniverseofelements, U = {u1, u2}.Theteamofexpertsisrepresentedbytheset X = {x1, x2}. Supposethattheteamofexpertsconsiderasetofparameters, E = {e1, e2, e3},where ei (i = 1,2,3) denotes thedecisions“reliability”,“comfortability”and“durability”,respectively,andsuppose Γ = e1 0.4 , e2 0.6 , e3 0.5 isa fuzzysubsetof FZ(E).Itistobenotedthattheparametersmaybeaffectedandalteredafterthecarsaretested. ByapplyingtheFP-CNSESandconsideringtheopinionsoftheexpertsinprocessone(beforetestingthecar) asamplitudetermsofmembership,non-membershipandindeterminatemembership,andsettingtheopinions oftheexpertsinthesecondprocess(aftertestingthecar)asphasetermsofmembership,non-membership, andindeterminacy,thefirstandsecondprocessesformaFP-CNSESasawhole,whichisshownbelow:
(H, A)Γ = e1 0.4 , x1,1 , u1 0.6e2π(0.5) ,0.1e2π(0) ,0.2e2π(0.4) , u2 0.5e2π(0.8) ,0.4e2π(0.7) ,0.3e2π(1) , e1 0.4 , x2,1 , u1 0.5e2π(0.4) ,0.7e2π(0.5) ,0.1e2π(0.2) , u2 0.8e2π(0.7) ,0.7e2π(0.5) ,0.4e2π(0.3) , e2 0.6 , x1,1 , u1 0.3e2π(0.2) ,0.5e2π(0.6) ,0.4e2π(0.5) , u2 0.6e2π(0.5) ,0.7e2π(0.6) ,0.1e2π(0.2) , e2 0.6 , x2,1 , u1 0.9e2π(0.8) ,0.5e2π(0.3) ,0.7e2π(0.4) , u2 0.5e2π(0.4) ,0.8e2π(0.9) ,0.1e2π(0.2) , e3 0.5 , x1,1 , u1 0.4e2π(0.2) ,0.5e2π(0.3) ,0.7e2π(0.6) , u2 0.5e2π(0.2) ,0.7e2π(0.2) ,0.8e2π(0.2) , e3 0.5 , x2,1 , u1 0.8e2π(0.1) ,0.3e2π(0.2) ,0.7e9π(0.2) , u2 0.1e2π(0.6) ,0.2e2π(0.4) ,0.6e2π(0.8) , e1 0.4 , x1,0 , u1 0.6e2π(0.5) ,0.7e2π(0.4) ,0.9e2π(0.3) , u2 0.9e2π(0.8) ,0.8e2π(0.8) ,0.7e2π(0.7) , e1 0.4 , x2,0 , u1 0.4e2π(0.2) ,0.6e2π(0.4) ,0.7e2π(0.8) , u2 0.2e2π(0.4) ,0.5e2π(0.4) ,0.6e2π(0.5) , e2 0.6 , x1,0 , u1 0.3e2π(0.2) ,0.6e2π(0.2) ,0.9e2π(0.2) , u2 0.6e2π(0.4) ,0.5e2π(0.6) ,0.7e2π(0.8) , e2 0.6 , x2,0 , u1 0.5e2π(0.8) ,0.3e2π(0.1) ,0.8e2π(0.9) , u2 0.3e2π(0.7) ,0.8e2π(0.7) ,0.7e2π(0.6) , e3 0.5 , x1,0 , u1 0.4e2π(0.7) ,0.9e2π(0.8) ,0.1e2π(0.3) , u2 0.8e2π(0.2) ,0.3e2π(0.2) ,0.5e2π(0.2) , e3 0.5 , x2,0 , u1 0.5e2π(0.4) ,0.6e2π(0.7) ,0.1e2π(0.9) , u2 0.1e2π(0) ,0.4e2π(0.3) ,0.1e2π(0.1)
IntheFP-CNSES (H, A)Γ above,theamplitudetermofthemembershipinthefirstprocessandthe phaseterminthesecondprocessformacomplex-valuedtruthmembershipfunction.Similarly,theamplitude termofnon-membershipinprocessoneandthephasetermofnon-membershipinthesecondprocessforma complex-valuedfalsitymembershipfunction.Inaddition,theamplitudetermofundecidednessinthefirst processandthephasetermofindeterminacyinthesecondprocessformthecomplex-valuedindeterminate membershipfunction.
Now,weputforwardthedefinitionofanagreeFP-CNSESandthedefinitionofadisagree FP-CNSES.
Definition6. AnagreeFP-CNSES (H, A)Γ1 over U isaFP-CNSEsubsetof (H, A)Γ wheretheopinionsof allexpertsare“agree”andisdefinedasfollows: (H, A)Γ1 = a, u HΓ (a)(u) : u ∈ U : a ∈ A ⊆ Γ × X ×{1}
Definition7. AdisagreeFP-CNSES (H, A)Γ0 over U isaFP-CNSEsubsetof (H, A)Γ wheretheopinionsof allexpertsare“disagree”andisdefinedasfollows: (H, A)Γ0 = a, u HΓ (a)(u) : u ∈ U : a ∈ A ⊆ Γ × X ×{0}
Inthefollowing,wegivesomebasicdefinitionsandsettheoreticoperationsofFP-CNSESs. WebeginbyproposingthedefinitionofthesubsetoftwoFP-CNSESsandtheequalityoftwo FP-CNSESs.
Definition8. Let (H, A)Γ and (G, B)∆ betwoFP-CNSESsoverauniverse U,wesaythat (H, A)Γ isafuzzy parameterizedcomplexneutrosophicsoftexpertsubsetof (G, B)∆ denotedby (H, A)Γ ⊆ (G, B)∆ ifandonlyif 1.A ⊆ B,and2. ∀a ∈ A, HΓ (a) iscomplexneutrosophicsubsetofG∆ (a)
Definition9. FortwoFP-CNSESs (H, A)Γ and (G, B)Γ overauniverse U,wesaythat (H, A)Γ isequalto (G, B)Γ andwewrite (H, A)Γ =(G, B)Γ if (H, A)Γ ⊆ (G, B)Γ and (G, B)Γ ⊆ (H, A)Γ
Inthefollowing,weproposethedefinitionofthecomplementofaFP-CNSESalongwithan illustrativeexampleandgiveapropositiononthecomplementofaFP-CNSES. Let U beauniverseofdiscourseand (H, A)Γ beaFP-CNSESon U,whichisasdefinedbelow:
(H, A)Γ = a, u HΓ (a)(u) : u ∈ U : a ∈ A Definition10. Thecomplementof (H, A)Γ isdenotedby (H, A)c Γ andisdefinedby
(H, A)c Γ = a, u Hc Γ (a)(u) : u ∈ U : a ∈¬A , where Hc Γ (a)(u)= THc Γ (a) (u), IHc Γ (a) (u), FHC Γ (a) (u) , suchthat: THc Γ (a) (u)= pHc Γ (a) (u) e jµHcΓ (a) (u) = rHΓ (a) (u) ej(2π µHΓ (a) (u)) , IHc Γ (a) (u)= qHc Γ (a) (u).e jνHcΓ (a) (u) =(1 qHΓ (a) (u)).ej(2π νHΓ (a) (u)) , FHc Γ (a) (u)= rHc Γ (a) (u).e jωHcΓ (a) (u) = pHΓ (a) (u).ej(2π ωHΓ (a) (u)) , and ¬A ⊆ Γc × X × O,where ˜ cisthefuzzycomplement.
Example2. ConsidertheapproximationgiveninExample 1,where
HΓ ( e1 0.4 , x1,1)= { u1 0.6ej2Π(0.5),0.1ej2Π(0),0.2ej2Π(0.4) , u2 0.5ej2Π(0.8),0.4ej2Π(0.7),0.3ej2Π(1) }
Byusingthecomplexneutrosophiccomplementandthefuzzycomplement,weobtainthecomplementof theapproximationgivenby
HΓ ( e1 0.6 , x1,1)= { u1 0.2ej2Π(0.5),0.9ej2Π(1),0.6ej2Π(0.6) , u2 0.3ej2Π(0.2),0.6ej2Π(0.3),0.5ej2Π(0 }
Proposition1. If (H, A)Γ isaFP-CNSESoverU,then (H, A)c Γ c = (H, A)Γ
FromDefinition 10,wehave (H, A)c Γ = a, u Hc Γ (a)(
) : u ∈ U : a ∈¬A ,where, Hc Γ (a)(u)
THc
(a) (
, IHc
, FHc
(
) (
) = pHc Γ (a) (u) e jµHcΓ (a) (u) , qHc Γ (a) (u) e jνHcΓ (a) (u) , rHc Γ (a) (u) e jωHcΓ (a) (u) , = rHΓ (a) (u) ej(2π µHΓ (a) (u)) , (1 qHΓ (a) (u)) ej(2π νHΓ (a) (u)) , pHΓ (a) (u) ej(2π ωHΓ (a) (u)) ,and ¬A ⊆ Γc × X × O Thus, ((H, A)c Γ )c = a, u (Hc Γ )c (a)(u ) : u ∈ U : a ∈¬(¬A) ,where (Hc Γ )c (a)(u)= rHc Γ (a) (u).e j(2π µHcΓ (a) (u)) , (1 qHc Γ (a) (u)).e j(2π νHcΓ (a) (u)) , pHc Γ (a) (u) .e j(2π ωHcΓ (a) (u)) ,
= pHΓ (a) (u) ej 2π (2π µHΓ (a) (u)) , 1 (1 qHΓ (a) (u)) ej 2π (2π νHΓ (a) (u)) , rHΓ (a) (u).ej 2π (2π ωHΓ (a) (u)) = pHΓ (a) (u).ejµHΓ (a) (u) , qHΓ (a) (u).ejνHΓ (a) (u) , rHΓ (a) (u).ejωHΓ (a) (u) , = THΓ (a) (u), IHΓ (a) (u), FHΓ (a) (u) , = HΓ (a)(u), and ¬(¬A) ⊆ (Γc )c × X × O andsince (Γc )c = Γ, thiscompletestheproof.
WeintroducethedefinitionofunionandintersectionoperationsoftwoFP-CNSESsalongwithan illustrativeexampleandsomepropositionsonthesetwooperations. Let (H, A)Γ and (G, B)∆ betwoFP-CNSESsoverauniverse U,where (H, A)Γ = a, u HΓ (a)(u) : u ∈ U : a ∈ A and (G, B)∆ = b, u G∆ (b)(u) : u ∈ U : b ∈ B
Definition11. Theunionof (H, A)Γ and (G, B)∆,denotedby (H, A)Γ ∪ (G, B)∆,istheFP-CNSES (K, C)Θ suchthatCΘ = AΓ ∪ B∆ and Θ = Γ∪∆, ∪ isthefuzzyunion,and ∀ ∈ CΘ, ∀ u ∈ U,
pHΓ ( ) (u) ejµHΓ ( ) (u) , if ∈ AΓ B∆ pG∆ ( ) (u).ejµG∆ ( ) (u) , if ∈ B∆ AΓ (pHΓ ( ) (u) ∨ pG∆ ( ) (u)) ej(µHΓ ( ) (u)∨µG∆ ( ) (u)) , if ∈ AΓ ∩ B∆, IKΘ ( ) (u)=
TKΘ ( ) (u)=
qHΓ ( ) (u) ejνHΓ ( ) (u) , if ∈ AΓ B∆ qG∆ ( ) (u).ejνG∆ ( ) (u) , if ∈ B∆ AΓ (qHΓ ( ) (u) ∧ qG∆ ( ) (u)) ej(νHΓ ( ) (u)∧νG∆ ( ) (u)) , if ∈ AΓ ∩ B∆,
rHΓ ( ) (u) ejωHΓ ( ) (u) , if ∈ AΓ B∆ rG∆ ( ) (u).ejωG∆ ( ) (u) , if ∈ B∆ AΓ (rHΓ ( ) (u) ∧ rG∆ ( ) (u)) ej(ωHΓ ( ) (u)∧ωG∆ ( ) (u)) , if ∈ AΓ ∩ B∆, where ∨ = max,and ∧ = min. Definition12. Theintersectionof (H, A)Γ and (G, B)∆,denotedby (H, A)Γ ∩ (G, B)∆,istheFP-CNSES (K, C)Θ suchthatCΘ = AΓ ∪ B∆ and Θ = Γ∩∆, ∩ isthefuzzyintersection,and ∀ ∈ CΘ, ∀ u ∈ U, TKΘ ( ) (u)=
FKΘ ( ) (u)=
pHΓ ( ) (u) ejµHΓ ( ) (u) , if ∈ AΓ B∆ pG∆ ( ) (u).ejµG∆ ( ) (u) , if ∈ B∆ AΓ (pHΓ ( ) (u) ∧ pG∆ ( ) (u)) ej(µHΓ ( ) (u)∧µG∆ ( ) (u)) , if ∈ AΓ ∩ B∆, IKΘ ( ) (u)=
Example3. ConsiderExample1.Let Γ = e1 0.3 , e2 0.1 , e3 0.9 beafuzzysubsetof E, and ∆ = e1 0.4 , e2 0.8 , e3 0.2 be anotherfuzzysubsetoverE.
AΓ = e1 0.3 , x1,1 , e2 0.1 , x2,1 , e3 0.9 , x2,0 , BΥ = e1 0.4 , x2,0 , e2 0.8 , x2,1 , e3 0.2 , x1,0 .
Suppose (H, A)Γ and (G, B)∆ aretwoFP-CNSESsoverthesameUgivenby
(H, A)Γ = e1 0.3 , x1,1 , u1 0.4e2π(0.5) ,0.5e2π(0.6) ,0.8e2π(0.3) , u2 0.3e2π(0.8) ,0.7e2π(0.1) ,0.6e2π(0.7) , e2 0.1 , x2,1 , u1 0.7e2π(0.4) ,0.9e2π(0.3) ,0.4e2π(0.1) , u2 0.6e2π(0.3) ,0.2e2π(0.6) ,0.4e2π(0.7) , e3 0.9 , x2,0 , u1 0.4e2π(0.1) ,0.3e2π(0.4) ,0.5e2π(0.9) , u2 0.6e2π(0.2) ,0.9e2π(0.8) ,0.1e2π(0.3) , and (G, B)∆ = e1 0.4 , x2,0 , u1 0.9e2π(0.5) ,0.5e2π(0.8) ,0.4e2π(0.4) , u2 0.1e2π(0.9) ,0.7e2π(0.2) ,0.6e2π(0.7) , e2 0.8 , x2,1 , u1 0.7e2π(0.6) ,0.4e2π(0.3) ,0.6e2π(0.4) , u2 0.7e2π(0.9) ,0.2e2π(0.4) ,0.1e2π(0.6) , e3 0.2 , x1,0 , u1 0.7e2π(0.4) ,0.2e2π(0.5) ,0.9e2π(0.3) , u2 0.1e2π(0.5) ,0.3e2π(0.7) ,0.9e2π(0.8)
Byusingthecomplexneutrosophicunionandthefuzzyunion(maximum),wehave
(H, A)Γ ∪ (G, B)∆ = e1 0.3 , x1,1 , u1 0.4e2π(0.5) ,0.5e2π(0.6) ,0.8e2π(0.3) , u2 0.3e2π(0.8) ,0.7e2π(0.1) ,0.6e2π(0.7) , e2 0.8 , x2,1 , u1 0.7e2π(0.6) ,0.4e2π(0.3) ,0.4e2π(0.1) , u2 0.7e2π(0.9) ,0.2e2π(0.4) ,0.1e2π(0.6) , e3 0.9 , x2,0 , u1 0.4e2π(0.1) ,0.3e2π(0.4) ,0.5e2π(0.9) , u2 0.6e2π(0.2) ,0.9e2π(0.8) ,0.1e2π(0.3) , e1 0.4 , x2,0 , u1 0.9e2π(0.5) ,0.5e2π(0.8) ,0.4e2π(0.4) , u2 0.1e2π(0.9) ,0.7e2π(0.2) ,0.6e2π(0.7) , e3 0.2 , x1,0 , u1 0.7e2π(0.4) ,0.2e2π(0.5) ,0.9e2π(0.3) , u2 0.1e2π(0.5) ,0.3e2π(0.7) ,0.9e2π(0.8)
Byusingthecomplexneutrosophicintersectionandthefuzzyintersection(minimum),wehave
(H, A)Γ ∩ (G, B)∆ = e1 0.3 , x1,1 , u1 0.4e2π(0.5) ,0.5e2π(0.6) ,0.8e2π(0.3) , u2 0.3e2π(0.8) ,0.7e2π(0.1) ,0.6e2π(0.7) , e2 0.8 , x2,1 , u1 0.7e2π(0.4) ,0.9e2π(0.3) ,0.6e2π(0.4) , u2 0.6e2π(0.3) ,0.2e2π(0.6) ,0.4e2π(0.7) , e3 0.9 , x2,0 , u1 0.4e2π(0.1) ,0.3e2π(0.4) ,0.5e2π(0.9) , u2 0.6e2π(0.2) ,0.9e2π(0.8) ,0.1e2π(0.3) , e1 0.4 , x2,0 , u1 0.9e2π(0.5) ,0.5e2π(0.8) ,0.4e2π(0.4) , u2 0.1e2π(0.9) ,0.7e2π(0.2) ,0.6e2π(0.7) , e3 0.2 , x1,0 , u1 0.7e2π(0.4) ,0.2e2π(0.5) ,0.9e2π(0.3) , u2 0.1e2π(0.5) ,0.3e2π(0.7) ,0.9e2π(0.8)
Let (L, D)Φ = , u LΦ ( )(u ) : u ∈ U : ∈ D ,where LΦ ( )(u)= TLΦ ( ) (u), ILΦ ( ) (u), FLΦ ( ) (u) . Weconsiderthecasewhen ∈ AΓ ∩ B∆ ∩ WΘ astheothercasesaretrivial. Then,wehave, TLΦ ( ) (u)= T(QΠ ( )∩SΘ ( )) (u)= T(HΓ ( )∪G∆ ( ))∩SΘ ( ) (u), = min(max(pHΓ ( ) (u), pG∆ ( ) (u)), pSΘ ( ) (u)) ejmin(max(µHΓ ( ) (u),µG∆ ( ) (u)),µSΘ ( ) (u)) , = max(min(pHΓ ( ) (u), pSΘ ( ) (u)), min(pG∆ ( ) (u), pSΘ ( ) (u))) ejmax(min(µHΓ ( ) (u),µSΘ ( ) (u)),min(µG∆ ( ) (u),µSΘ ( ) (u))) , = T(HΓ ( )∩SΘ ( ))∪(G∆ ( )∩SΘ ( )) (u), whichimpliesthat T(HΓ ( )∪G∆ ( ))∩SΘ ( ) (u)= T(HΓ ( )∩SΘ ( ))∪(G∆ ( )∩SΘ ( )) (u). Theproofsfortheidentityandfalsitytermsfollowsimilarly.Therefore, ((H, A)Γ ∪ (G, B)∆ )∩(S, W)Θ =((H, A)Γ ∩ (S, W)Θ )∪((G, B)∆ ∩(S, W)Θ ) (2)TheproofissimilartothatinPart(1)andthereforeisomitted.
4.MAPPINGONFP-CNSESs
Inthissection,weintroducethenotionofamappingonfuzzyparameterizedcomplex neutrosophicsoftexpertclasses.fuzzyparameterizedcomplexneutrosophicsoftexpertclasses arecollectionsofFP-CNSESs.Wedefinethefuzzyparameterizedcomplexneutrosophicsoftexpert imagesandfuzzyparameterizedcomplexneutrosophicsoftexpertinverseimagesofFP-CNSESs. Wegivesomeoperationsandpropertiesrelatedwiththisconcept.
Definition13. Let U beauniverseset, E beasetofparameters, FZ(E) denoteallfuzzysubsetsof E,where Γ ⊂ FZ(E), X beasetofexperts,and O = {1 = agree,0 = disagree} asetofopinions.Let Y = Γ × X × O. Then,thecollectionofallFP-CNSESsover U withparametersfrom Y iscalledafuzzyparameterizedcomplex neutrosophicsoftexpertclassandisdenotedas (U, Y).
Definition14. Let (U, Y) and (V, Y ) befuzzyparameterizedcomplexneutrosophicsoftexpertclasses.Let r : U → Vands : Y → Y bemappings.Then,amappingF : (U, Y) → (V, Y ) isdefinedasfollows: ForaFP-CNSES (H, A)Γ in (U, Y), (F (H, A)Γ , M),where M = s(Y) ⊆ Y isaFP-CNSESin (V, Y ) obtainedasfollows:
F (H, A)Γ (β)(v) = u∈r 1(v) α∈s 1(β)∩A HΓ (α) (u) ifr 1 (v) ands 1 (β) ∩ A = ∅, (0,1,1) otherwise.
For u ∈ r 1 (v), β ∈ M ⊆ Y , v ∈ V and ∀ α ∈ s 1 (β) ∩ A, (F (H, A)Γ , M) iscalledafuzzy parameterizedcomplexneutrosophicsoftexpertimageoftheFP-CNSES (H, A)Γ.If M = Y , thenweshall write (F (H, A)Γ , M) asF (H, A)Γ
Definition15. Let (U, Y) and (V, Y ) betwofuzzyparameterizedcomplexneutrosophicsoftexpertclasses. Letr : U → Vands : Y → Y bemappings.Then,amappingF 1 : (V, Y ) → (U, Y) isdefinedasfollows: ForaFP-CNSES (G, B)∆ in (V, Y ), (F 1 (G, B)∆ , N), where N = s 1 (B) , isaFP-CNSESin (U, Y) obtainedasfollows: F 1 (G, B)∆ (α)(u) = G∆ (s (α))(r (u)) ifs (α) ∈ B, (0,1,1) otherwise,
For α ∈ N ⊆ Y and u ∈ U (F 1 (G, B)∆, N) iscalledafuzzyparameterizedcomplexneutrosophicsoft expertinverseimageoftheFP-CNSES (G, B)∆.IfN = Y, wewrite (F 1 (G, B)∆, N) asF 1(G, B)∆
Definition16. Let F : (U, Y) → (V, Y ) beamappingand (H, A)Γ, (H , A )∆ beFP-CNSESsin (U, Y) Then,for β ∈ Y , ν ∈ V,theunionandintersectionofthefuzzyparameterizedcomplexneutrosophicsoftexpert imagesF(H, A)Γ andF(G, B)∆ aredefinedasfollows.
F (H, A)Γ ∪F H , A ∆ (β)(ν) = F (H, A)Γ (β)(ν) ∪F H , A ∆ (β)(ν) ,
F (H, A)Γ ˜ ∩F H , A ∆ (β)(ν) = F (H, A)Γ (β)(ν) ∩F H , A ∆ (β)(ν) , where ˜ ∪ and ˜ ∩ denotefuzzyparameterizedcomplexneutrosophicsoftexpertunionandintersectionoffuzzy parameterizedcomplexneutrosophicsoftexpertimagesin (V, Y )
Definition17. Let F : (U, Y) → (V, Y ) beamappingand (G, B)Σ, (G , B )Ω FP-CNSESsin (V, Y ).Then, for α ∈ Y, u ∈ U,theunionandintersectionofthefuzzyparameterizedcomplexneutrosophicsoftexpert inverseimagesF 1(G, B)Σ andF 1(G , B )Ω aredefinedasfollows.
F 1 (G, B)Σ ∪F 1 G , B Ω (α)(u) = F 1 (G, B)Σ (α)(u) ∪F 1 G , B Ω (α)(u) , (F 1(G, B)Σ ∩F 1(G , B )Ω )(α)(u)= F 1(G, B)Σ (α)(u)∩F 1(G , B )Ω (α)(u), where ∪ and ∩ denotefuzzyparameterizedcomplexneutrosophicsoftexpertunionandintersectionoffuzzy parameterizedcomplexneutrosophicsoftexpertinverseimagesin (U, Y).
Proposition3. Let F : (U, Y) → (V, Y ) beamapping.Then,forFP-CNSESs (H, A)Γ and (H , A )∆ inthe fuzzyparameterizedcomplexneutrosophicsoftexpertclass (U, Y),wehave:
1. F(φ)= φ.
2. F(ψ)= ψ
3. F ((H, A)Γ ∪ (H , A )∆ ) = F (H, A)Γ ∪F (H , A )∆
4. F ((H, A)Γ ∩ (H , A )∆ ) ⊆ F (H, A)Γ ∩F (H , A )∆.
5. If (H, A)Γ ⊆ (H , A )∆,thenF (H, A)Γ ⊆ F (H , A )∆
Proof. TheproofisstraightforwardbyDefinitions 14 and 16
Proposition4. Let F : (U, Y) → (V, Y ) beamapping.Then,forFP-CNSESs (G, B)Σ and (G , B )Ω inthe fuzzyparameterizedcomplexneutrosophicsoftexpertclass (V, Y ),wehave:
1. F 1(φ)= φ.
2. F 1( ˜ ψ)= ˜ ψ 3. F 1 ((G, B)Σ ∪ (G , B )Ω ) = F 1 (G, B)Σ ∪F 1 (G , B )Ω
withdegrees0.3,0.6and0.8,respectively.Thatis,thefuzzysubsetofparametersis Γ = e1 0.3 , e2 0.6 , e3 0.8 Let X = {x1, x2} beasetofexperts.Now,theteamofexpertsarerequestedtomakeadecisionaboutthemost desirablealternativebasedonthegoalsandtheconstraintsaccordingtoachosensubset Γ of FZ(E) toconstruct aFP-CNSES.
(H, A)Γ = e1 0.3 , x1,1 , u1 0.5e2π(0.4) ,0.2e2π(0.4) ,0.1e2π(0.2) , u2 0.6e2π(0.7) ,0.5e2π(0.4) ,0.1e2π(1) , e1 0.3 , x2,1 , u1 0.6e2π(0.5) ,0.6e2π(0.4) ,0.1e2π(0.3) , u2 0.9e2π(0.8) ,0.5e2π(0.6) ,0.5e2π(0.4) , e2 0.6 , x1,1 , u1 0.4e2π(0.3) ,0.7e2π(0.8) ,0.8e2π(0.7) , u2 0.4e2π(0.9) ,0.2e2π(0.5) ,0.1e2π(0.2) , e2 0.6 , x2,1 , u1 0.8e2π(0.9) ,0.4e2π(0.3) ,0.7e2π(0.4) , u2 0.5e2π(0.4) ,0.8e2π(0.6) ,0.6e2π(0.2) , e3 0.8 , x1,1 , u1 0.2e2π(0.2) ,0.3e2π(0.3) ,0.7e2π(0.6) , u2 0.5e2π(0.2) ,0.7e2π(0.2) ,0.8e2π(0.2) , e3 0.8 , x2,1 , u1 0.8e2π(0.1) ,0.3e2π(0.2) ,0.7e9π(0.2) , u2 0.1e2π(0.6) ,0.2e2π(0.4) ,0.6e2π(0.8) , e1 0.3 , x1,0 , u1 0.5e2π(0.5) ,0.5e2π(0.4) ,0.9e2π(0.3) , u2 0.9e2π(0.8) ,0.7e2π(0.8) ,0.5e2π(0.7) , e1 0.3 , x2,0 , u1 0.4e2π(0.6) ,0.7e2π(0.4) ,0.7e2π(0.8) , u2 0.5e2π(0.4) ,0.5e2π(0.4) ,0.3e2π(0.5) , e2 0.6 , x1,0 , u1 0.3e2π(0.2) ,0.6e2π(0.2) ,0.9e2π(0.2) , u2 0.7e2π(0.4) ,0.5e2π(0.8) ,0.6e2π(0.8) , e2 0.6 , x2,0 , u1 0.3e2π(0.5) ,0.3e2π(0.1) ,0.9e2π(0.3) , u2 0.3e2π(0.7) ,0.8e2π(0.7) ,0.7e2π(0.6) , e3 0.8 , x1,0 , u1 0.5e2π(0.4) ,0.4e2π(0.5) ,0.4e2π(0.3) , u2 0.8e2π(0.2) ,0.3e2π(0.2) ,0.5e2π(0.2) , e3 0.8 , x2,0 , u1 0.4e2π(0.6) ,0.2e2π(0.3) ,0.1e2π(0.6) , u2 0.3e2π(0) ,0.6e2π(0.4) ,0.3e2π(0.1)
IntheFP-CNSES (H, A)Γ above,theamplitudetermsofmembership,non-membershipand indeterminatemembershiprepresenttheopinionsoftheexpertsinphaseone(beforeusingthe products),whilethephasetermsofmembership,non-membership,andindeterminacyrepresentthe opinionsoftheexpertsinthesecondphase(aftertryingasampleofeachofthetwokindsofthe product).Thus,theamplitudetermofthemembershipinthefirstphaseandthephasetermofthe membershipinthesecondphaseformacomplex-valuedtruthmembershipfunctionoftheFP-CNSES (H, A)Γ.Similarly,theamplitudetermofnon-membershipinphaseoneandthephasetermof non-membershipinthesecondphaseformacomplex-valuedfalsitymembershipfunction.Inaddition, theamplitudetermofundecidednessinthefirstphaseandthephasetermofindeterminacyinthe secondphaseformthecomplex-valuedindeterminatemembershipfunction.Now,ourproblemisto selectthemostdesirablekindoftheproducttotheengineeringcompany.
Tosolvethisdecisionmakingproblem,weusetheFP-CNSES (H, A)Γ togetherwithageneralized algorithm.Thisalgorithmconvertsthefuzzyparameterizedcomplexneutrosophicsoftexpertvalues (FP-CNSEVs)tofuzzyparameterizedsingle-valuedneutrosophicsoftexpertvalues(FP-SVNSEVs) usingapracticalformulawhichgiveadecision-makingwithasimplecomputationalprocesswithout theneedtocarryoutdirectedoperationsoncomplexnumbers.Inthisformula,wegiveaweight totheamplitudeterms(aweighttotheexperts’opinionsbeforeusingtheproducts)bymultiplying theweightvectortoeachamplitudeterm.Similarlywegiveaweighttothephaseterms(aweight totheexperts’opinionsafterusingtheproducts)bymultiplyingtheweightvectortoeachphase term.Then,wecombinethevaluesoftheweightedamplitudetermsandphasetermstoobtain theFP-SVNSEVswhichrepresenttheexperts’opinionsonbothphasestogether.Thus,afterdoing thesesimplecalculationstoallmembershipfunctionsoftheFP-CNSES (H, A)Γ,weproceedto thefinaldecisionusingthefuzzyparameterizedsinglevaluedneutrosophicsoftexpertmethod (FPSVNSEM)[18].
Thegeneralizedalgorithmisasfollows.
Algorithm1: Fuzzyparameterizedcomplexneutrosophicsoftexpertmethod(FP-CNSEM).
1.InputtheFP-CNSES (H, A)Γ
2.ConverttheFP-CNSES (H, A)Γ totheFPSVNSES (H, A)Γ byobtainingtheweighted aggregationvaluesof T HΓ (ai ) (uj ), I HΓ (ai ) (uj ) and F HΓ (ai ) (uj ),∀ai ∈ A and ∀uj ∈ U asthe followingformulas:
THΓ (ai ) (uj )= w1 pHΓ (ai ) (uj )+ w2(1/2π)µHΓ (ai ) (uj ), IHΓ (ai ) (uj )= w1qHΓ (ai ) (uj )+ w2(1/2π)νHΓ (ai ) (uj ), FHΓ (ai ) (uj )= w1rHΓ (ai ) (uj )+ w2(1/2π)ωHΓ (ai ) (uj ), where pHΓ (ai ) (uj ), qHΓ (ai ) (uj ), rHΓ (ai ) (uj ) and µHΓ (ai ) (uj ), νHΓ (ai ) (uj ), ωHΓ (ai ) (uj ) arethe amplitudeandphasetermsintheFP-CNSES (H, A)Γ,respectively. T HΓ (ai ) (uj ), I HΓ (ai ) (uj ) and F HΓ (ai ) (uj ) arethetruth-membershipfunction,indeterminacy-membershipfunctionand falsity-membershipfunctionintheFP-SVNSES (H, A)Γ,respectively,and w1, w2 arethe weightsfortheamplitudeterms(thefirstdecisionprocess)andthephaseterms(thesecond decisionprocess),respectively,where w1 and w2 ∈ [0,1] and w1 + w2 = 1.
3.Findthevaluesof cij = T HΓ (ai ) (uj ) I HΓ (ai ) (uj ) F HΓ (ai ) (uj ), ∀uj ∈ U and ∀ai ∈ A
4.Computethescoreofeachelement uj ∈ U bythefollowingformulas: Kj = ∑ x∈X
n ∑ i=1 cij (µΓ (ei )), Sj = ∑ x∈X
n ∑ i=1 cij (µΓ (ei ))
fortheagreeFP-SVNSESanddisagreeFP-SVNSES,where µΓ (ei ) isthecorresponding membershipfunctionofthefuzzyset Γ, X isthesetoftheexpertsand n isthenumberofthe parameters(attributes).
5.Findthevaluesofthescore rj = Kj Sj foreachelement uj ∈ U
6.Determinethevalueofthehighestscore m = maxuj ∈U {rj }.Then,thedecisionistochoose element uj astheoptimalsolutiontotheproblem.Iftherearemorethanoneelementswith thehighest rj score,thenanyoneofthoseelementscanbechosenastheoptimalsolution.
Then,wecanconcludethattheoptimalchoicefortheteamoftheexpertsistoselectthekind uj asthemostdesirablekindoftheproducttothecompany.
Now,toconverttheFP-CNSES (H, A)Γ totheFP-SVNSES (H, A)Γ,supposethattheweight vectorfortheamplitudetermsis w1 = 0.3 andtheweightvectorforthephasetermsis w2 = 0.7 and obtaintheweightedaggregationvaluesof T HΓ (ai ) (uj ), I HΓ (ai ) (uj ) and F HΓ (ai ) (uj ),∀ai ∈ A and ∀uj ∈ U Toillustratethisstep,wecalculate T HΓ (a1) (u1), I HΓ (a1) (u1) and F HΓ (a1) (u1),suchthat a1 =( e1 0.3 , x1,1) asshownbelow: T HΓ ( e1 0.3 ,x1,1) (u1)= w1 pHΓ ( e1 0.3 ,x1,1) (u1)+ w2(1/2π)µHΓ ( e1 0.3 ,x1,1) (u1) = 0.3(0.5)+ 0.7(1/2π)(2π)(0.4) = 0.43 I HΓ ( e1 0.3 ,x1,1) (u1)= w1qHΓ ( e1 0.3 ,x1,1) (u1)+ w2(1/2π)νHΓ ( e1 0.3 ,x1,1) (u1) = 0.3(0.2)+ 0.7(1/2π)(2π)(0.4) = 0.34
F HΓ ( e1 0.3 ,x1,1) (u1)= w1r HΓ ( e1 0.3 ,x1,1) (u1)+ w2(1/2π)ωHΓ ( e1 0.3 ,x1,1) (u1) = 0.3(0.1)+ 0.7(1/2π)(2π)(0.2) = 0.17.
Then,theFP-SVNSEV (THΓ (a1) (u1), IHΓ (a1) (u1), FHΓ (a1) (u1))=(0.43,0.34,0.17)
Inthesamemanner,wecalculatetheothersFP-SVNSEVs, ∀a ∈ A and ∀u ∈ U asinTable 1
Table1. Valuesof (H, A)Γ u1 u2
e1 0.3 , x1,1 0.43,0.34,0.17 0.67,0.43,0.73 e1 0.3 , x2,1 0.53,0.46,0.24 0.83,0.57,0.53 e2 0.6 , x1,1 0.33,0.77,0.73 0.75,0.41,0.71 e2 0.6 , x2,1 0.87,0.33,0.49 0.43,0.66,0.32 e3 0.8 , x1,1 0.20,0.30,0.63 0.29,0.35,0.38 e3 0.8 , x2,1 0.31,0.23,0.35 0.45,0.34,0.74 e1 0.3 , x1,0 0.50,0.43,0.48 0.83,0.77,0.64 e1 0.3 , x2,0 0.54,0.49,0.77 0.43,0.43,0.44 e2 0.6 , x1,0 0.23,0.32,0.41 0.49,0.71,0.74 e2 0.6 , x2,0 0.44,0.16,0.48 0.58,0.73,0.63 e3 0.8 , x1,0 0.43,0.47,0.33 0.38,0.23,0.29 e3 0.8 , x2,0 0.54,0.27,0.45 0.09,0.46,0.16
Tables 2 and 3 givethevaluesof cij = T HΓ (ai ) (uj ) I HΓ (ai ) (uj ) F HΓ (ai ) (uj ) andthescoreofeach element uj ∈ U foragreeFP-SVNSESanddisagreeFP-SVNSES,respectively.
Table2. NumericaldegreeofagreeFP-SVNSES.
Uu1 u2 e1 0.3 , x1 0.08 0.49 e1 0.3 , x2 0.17 0.27 e2 0.6 , x1 1.17 0.37 e2 0.6 , x2 0.05 0.55 e3 0.8 , x1 0.73 0.44 e3 0.8 , x2 0.27 0.63
Kj = ∑ x∈X
3 ∑ i=1 cij (µΓ (ei )) K1 = 1.547 K2 = 1.636
Table3. NumericaldegreefordisagreeFP-SVNSES.
Uu1 u2
e1 0.3 , x1 0.41 0.58 e1 0.3 , x2 0.72 0.44 e2 0.6 , x1 0.50 0.96 e2 0.6 , x2 0.20 0.78 e3 0.8 , x1 0.37 0.14 e3 0.8 , x2 0.18 0.53
Sj = ∑ x∈X
3 ∑ i=1 cij(µΓ (ei )) S1 = 1.199 S2 = 1.886
Let Kj and Sj representthescoreofeachnumericaldegreefortheagreeFP-SVNSESanddisagree FP-SVNSES,respectively.ThesevaluesaregiveninTable 4.
Table4. Thescore rj = Kj Sj Kj = ∑ x∈X
3 ∑ i=1 cij(µΓ (ei )) Sj = ∑ x∈X
3 ∑ i=1 cij(µΓ (ei )) rj = Kj Sj
K1 = 1.547 S1 = 1.199 0.348 K2 = 1.636 S2 = 1.8860.25
Thus, m = maxuj ∈U {rj } = r2 .Therefore,theteamofexpertsadviseselectingthekind u2 ofthis productasadesirablealternative.
6.ComparisonbetweenFP-CNSESandOtherExistingMethods
Inthissection,wecompareourproposedFP-CNSESmodeltoFP-SVNSES[18]andFP-IFSES[17], whicharegeneralizationsofFP-SES[15]andFP-FSES[16].
TorevealthesignificanceofourproposedFP-CNSEScomparedtoFP-SVNSESandFP-IFSES, letusconsiderExample4above.Inthisexample,weapplytheFP-CNSEStohandleadecision-making problem,whichconsistsoftwodecisionprocesses.Ourproposedmodelisappliedtoboththedecision processesbyconsideringtheopinionsinprocessoneasamplitudetermsoftruthmembership,falsity membershipandindeterminacymembership,andsettingtheseconddecisionprocessasphaseterms oftruthmembership,falsitymembership,andindeterminacy.Thus,bothdecisionprocessesforma FP-CNSESaswhole.
Ontheotherhand,whenweapplytheFP-IFSEStobothprocesses,ittellsusonlyaboutthe truthmembershipandfalsitymembershipinthefirstdecisionprocess,butcannottellanythingabout theundecidedness.Thesituationissimilarintheseconddecisionprocess.Thus,FP-IFSESfailsto handlethissituation.Now,whenweapplytheFP-SVNSES,ittellsaboutthetruthmembership,falsity membershipandindeterminacymembershipinthefirstround,and,similarly,ittellsaboutthesecond roundbutitcannotdescribebothdecisionprocessessimultaneously.
Thus,bothFP-SVNSESandFP-IFSEScannotdirectlysolvesuchadecision-makingproblem withfuzzyparameterizedcomplexneutrosophicsoftexpertinformation.Incontrast,theFP-CNSES candirectlyaddressthefuzzyparameterizedsinglevaluedneutrosophicsoftexpertproblem, sincetheFP-SVNSESisaspecialcaseofFP-CNSESandcanbeeasilyrepresentedintheformof FP-CNSES.Inotherwords,theFP-SVNSESisaFP-CNSESwithphasetermsequalzeros.Forexample, theFP-SVNSEV(0.2,0.4,0.6)canberepresentedas (0.2ej2Π(0),0.4ej2Π(0),0.6ej2Π(0) ) bymeansof FP-CNSES.Furthermore,ourmethodisapplicableforfuzzyparameterizedintuitionisticfuzzysoft expertproblem,sinceFP-IFSESisaspecialcaseofFP-SVNSESandconsequentlyofFP-CNSES. Forexample,thefuzzyparameterizedintuitionisticfuzzysoftexpertvalue(0.1,0.6)canbe(0.1,0.3, 0.6)bymeansofFP-SVNSESandhencecanbe (0.1ej2Π(0),0.3ej2Π(0),0.6ej2Π(0) ) bymeansofFP-CNSES, sincethesumofthedegreesofmembership,nonmembershipandindeterminacyofanintuitionistic fuzzyvalueequalto1.Notethattheindeterminacydegreeinintuitionisticfuzzysetisprovidedby defaultandcannotbedefinedaloneunliketheSVNSwheretheindeterminacyisdefinedindependently andquantifiedexplicitly.
TheadvantageofusingFP-CNSESmanifestsinrepresentinginformationoftwodimensions foroneobjectinthesametime(i.e.,theexpert’stimecanbesavedbyconstructing/buildingone set(FP-CNSES)insteadoftwosets(FP-SVNSESs),nottomentionreducingtheamountofboth mathematicalcalculationsandinvestigationofseveraloperatorsinincorporatingtwoFP-SVNSESsto findtheoptimalsolution).ApracticalformulaisemployedtoconverttheFP-CNSEStotheFP-SVNSES, whichgivesadecision-makingwithasimplecomputationalprocesswithouttheneedtocarryout directedoperationsoncomplexnumbers.
7.WeightedFuzzyParameterizedComplexNeutrosophicSoftExpertSet
Expertsopinionsarevitalforanydecisionmakingprocessasmostreal-lifesituationsdeal withelementsandparametersthataresubjective,biasedandhavethepotentialtobedistortedand interpreteddifferentlybydifferentparties.Eachexpertmighthavehis/herownthought,whichdiffers fromothersinvariousaspectsbutalloftheexpertsshouldhaveacommongoaltoreachthefinal destination.Moreover,asthedomainsofexpertiseofdifferentexpertsaredifferent,oneexpertmay bemoreconfidentonhis/heropinionthantheotheronthesamesetofattributes.Forthistypeof environment,equalweightsassignmenttodifferentexpertsmayleadtoimproperandbiasedsolution. Feelingtheneedofprioritizingdifferentexpertsmotivatesustodevelopanewideaforassigning relativeweighttoeachexpertbyestablishinganovelnotioncalledweightedfuzzyparameterized complexneutrosophicsoftexpertset(WFP-CNSES).Therelativeweightisassignedtoeachofthe expertswherethechoiceoftheexpertsmaynotbeofequalimportance.
Thisnewmathematicalframeworkreducesthechanceofunfairnessinthedecisionmaking processandbringsmorecredibilitytothefinaldecision.
Webeginthissectionbyfirstproposingtheconceptoftheweightedfuzzyparameterized single-valuedneutrosophicsoftexpertset(WFP-SVNSES).
Definition18. Let U beauniverseset, E beasetofparameters, I E denoteallfuzzysubsetsof E, X bea setofexperts, I X denotesallfuzzysubsetsof X and O = {1 = agree,0 = disagree} asetofopinions. Let Z = Ψ × W × O and A ⊆ Z where Ψ ⊂ I E and W ⊂ I X .Apair ( f , A, )Ψ,W iscalledaweightedfuzzy parameterizedsingle-valuedneutrosophicsoftexpertsetoverU,wherefisamappinggivenby fΨ,W : A → SVN(U), andSVN(U) denotesthesetofallsingle-valuedneutrosophicsubsetsofU.
WethengeneralizetheWFP-SVNSESbyestablishingtheconceptoftheweightedfuzzy parameterizedcomplexneutrosophicsoftexpertset(WFP-CNSES),whichisactuallyanextended versionofWFP-SVNSES,onthecomplexspace.
Definition19. Let U beauniverseset, E beasetofparameters, FZ(E) denoteallfuzzysubsetsof E, X bea setofexperts, FZ(X) denotesallfuzzysubsetsof X and O = {1 = agree,0 = disagree} asetofopinions. Let Y = Γ × W × O and A ⊆ Y where Γ ⊂ FZ(E) and W ⊂ FZ(X) .Apair (H, A, )Γ,W iscalledaweighted fuzzyparameterizedcomplexneutrosophicsoftexpertset (WFP CNSES) over U,where H isamapping givenby HΓ,W : A → CNU , andCNU denotesthepowercomplexneutrosophicsetofU.
TheWFP-CNSES (H, A)Γ,W canbewrittenasthefollowingsetoforderedpairs: (H, A)Γ,W = a, u HΓ,W (a)(u ) : u ∈ U : a ∈ A ,where A ⊆ Γ × W × O = e µΓ (e) , x µW (x) , o : e ∈ E, x ∈ Xand o ∈ O , suchthat µΓ (e) and µW (x) arethecorresponding membershipfunctionsofthefuzzysets Γ and W,respectively.
FromDefinition19,itisclearthattheexpertsetintheWFP-CNSES (H, A)Γ,W isafuzzy set,wherethemembershipfunction µW (x) ofthefuzzyset W representsweightoftheexpert x, and µW (x) ∈ [0,1], ∀x ∈ X
Formoreillustration,weconsiderthefollowingexample.
Example5. ConsiderExample4.Supposethattheweightsfortheexperts x1 and x2 are 0.5 and 0.8,respectively. Then,thefuzzysubsetofexpertsis W = x1 0.5 , x2 0.8 andtheFP-CNSES (H, A)Γ inExample4isconvertedto theWFP-CNSES (H, A)Γ,W where,
(H, A)Γ,W = e1 0.3 , x1 0.5 ,1 , u1 0.5e2π(0.4) ,0.2e2π(0.4) ,0.1e2π(0.2) , u2 0.6e2π(0.7) ,0.5e2π(0.4) ,0.1e2π(1) , e1 0.3 , x2 0.8 ,1 , u1 0.6e2π(0.5) ,0.6e2π(0.4) ,0.1e2π(0.3) , u2 0.9e2π(0.8) ,0.5e2π(0.6) ,0.5e2π(0.4) , e2 0.6 , x1 0.5 ,1 , u1 0.4e2π(0.3) ,0.7e2π(0.8) ,0.8e2π(0.7) , u2 0.4e2π(0.9) ,0.2e2π(0.5) ,0.1e2π(0.2) , e2 0.6 , x2 0.8 ,1 , u1 0.8e2π(0.9) ,0.4e2π(0.3) ,0.7e2π(0.4) , u2 0.5e2π(0.4) ,0.8e2π(0.6) ,0.6e2π(0.2) , e3 0.8 , x1 0.5 ,1 , u1 0.2e2π(0.2) ,0.3e2π(0.3) ,0.7e2π(0.6) , u2 0.5e2π(0.2) ,0.7e2π(0.2) ,0.8e2π(0.2) , e3 0.8 , x2 0.8 ,1 , u1 0.8e2π(0.1) ,0.3e2π(0.2) ,0.7e9π(0.2) , u2 0.1e2π(0.6) ,0.2e2π(0.4) ,0.6e2π(0.8) , e1 0.3 , x1 0.5 ,0 , u1 0.5e2π(0.5) ,0.5e2π(0.4) ,0.9e2π(0.3) , u2 0.9e2π(0.8) ,0.7e2π(0.8) ,0.5e2π(0.7) , e1 0.3 , x2 0.8 ,0 , u1 0.4e2π(0.6) ,0.7e2π(0.4) ,0.7e2π(0.8) , u2 0.5e2π(0.4) ,0.5e2π(0.4) ,0.3e2π(0.5) , e2 0.6 , x1 0.5 ,0 , u1 0.3e2π(0.2) ,0.6e2π(0.2) ,0.9e2π(0.2) , u2 0.7e2π(0.4) ,0.5e2π(0.8) ,0.6e2π(0.8) , e2 0.6 , x2 0.8 ,0 , u1 0.3e2π(0.5) ,0.3e2π(0.1) ,0.9e2π(0.3) , u2 0.3e2π(0.7) ,0.8e2π(0.7) ,0.7e2π(0.6) , e3 0.8 , x1 0.5 ,0 , u1 0.5e2π(0.4) ,0.4e2π(0.5) ,0.4e2π(0.3) , u2 0.8e2π(0.2) ,0.3e2π(0.2) ,0.5e2π(0.2) , e3 0.8 , x2 0.8 ,0 , u1 0.4e2π(0.6) ,0.2e2π(0.3) ,0.1e2π(0.6) , u2 0.3e2π(0) ,0.6e2π(0.4) ,0.3e2π(0.1) .
SupposeweareinterestedinsolvingthedecisionmakingprobleminExample4,wherethedata arerepresentedbythemeansoftheWFP-CNSES (H, A)Γ,W above,whereeachexperthashis/herown weight.Then,wemayusethefollowingalgorithm,whichisageneralizationofAlgorithm 1 Now,weuseAlgorithm 2 toselectthemostdesirablekindoftheproducttotheengineeringcompany.
Algorithm2: Weightedfuzzyparameterizedcomplexneutrosophicsoftexpertmethod (WFP-CNSEM).
1.InputtheWFP-CNSES (H, A)Γ,W .
2.ConverttheWFP-CNSES (H, A)Γ,W totheWFP-SVNSES (H, A)Γ,W asitwasillustratedin step2ofAlgorithm1.NotethattheWFP-CNSES (H, A)Γ,W andtheFP-CNSES (H, A)Γ has thesameevaluationinformationandthedifferencebetweenthemliesinthestructureofthe expertsetwhichdoesnotaffecttheconversionprocess. 3.Findthevaluesof cij foragreeWFP-SVNSESanddisagreeWFP-SVNSESrespectively,where cij = T H
Tables 5 and 6 givethenumericaldegreeofagreeWFP-SVNSESanddisagreeWFP-SVNSES, respectively.
Table5. NumericaldegreeofagreeWFP-SVNSES.
Uu1 u2
e1 0.3 , x1 0.5 0.08 0.49
e1 0.3 , x2 0.8 0.17 0.27
e2 0.6 , x1 0.5 1.17 0.37 e2 0.6 , x2 0.8 0.05 0.55
e3 0.8 , x1 0.5 0.73 0.44 e3 0.8 , x2 0.8 0.27 0.63
Kj = ∑ x∈X ∑ i cij (µΓ (e))(µW (x)) K1 = 0.845 K2 = 1.093
Table6. NumericaldegreeofdisagreeWFP-SVNSES.
Uu1 u2
e1 0.3 , x1 0.5 0.41 0.58
e1 0.3 , x2 0.8 0.72 0.44
e2 0.6 , x1 0.5 0.50 0.96
e2 0.6 , x2 0.8 0.20 0.78 e3 0.8 , x1 0.5 0.37 0.14 e3 0.8 , x2 0.8 0.18 0.53 Sj = ∑ x∈X ∑ i cij (µΓ (e))(µW (x)) S1 = 0.744 S2 = 1.250
Let Kj and Sj representthescoreofeachnumericaldegreeofagreeWFP-SVNSESanddisagree WFP-SVNSES,respectively.ThesevaluesaregiveninTable 7.
Table7. Thescore rj = Kj Sj Kj = ∑ x∈X ∑ i cij(µΓ (e))(µW (x)) Sj = ∑ x∈X ∑ i cij (µΓ (e))(µW (x)) rj = Kj Sj K1 = 0.845 S1 = 0.744 0.101 K2 = 1.093 S2 = 1.2500.157
Thus, m = maxuj ∈U {rj } = r2 .Therefore,theteamofexpertsadviseselectingthekind u2 ofthis productasadesirablealternative.
UsingAlgorithms 1 and 2,weobtainedthesameresults.However,itisclearthatgivingmore considerationtotheexpert(weight)reducesthechanceofunfairnessinthedecisionmakingprocess andasaresultaddsmorecredibilitytothefinaldecision.
ToillustratethesignificanceoftheadjustableapproachusingWFP-CNSESascomparedtothatof FP-CNSES,letusconsiderExample5above.
ItcanbeseenthatWFP-CNSESisbasicallyaFP-CNSESwithweightedexpertsandevery FP-CNSEScanbeconsideredasaWFP-CNSESwitharelativeweightequalto1assignedtoall oftheexperts’opinions.
Forexample,theapproximation
e1 0.3 , x1 0.5 ,1 , u1 0.5e2π(0.4),0.2e2π(0.4),0.1e2π(0.2) , u2 0.6e2π(0.7),0.5e2π(0.4),0.1e2π(1)
canberepresentedas
e1 0.3 , x1 1 ,1 , u1 0.5e2π(0.4),0.2e2π(0.4),0.1e2π(0.2) , u2 0.6e2π(0.7),0.5e2π(0.4),0.1e2π(1) bymeansofFP-CNSES.
Inotherwords,theexpertsetintheFP-CNSESisaclassicalset,wheretheopinionsoftheexperts havethesamedegreeofimportance,whichisconsideredas1.Thislimitationmayleadtoimproper andbiasedsolution.Incontrast,theexpertsetintheWFP-CNSESisafuzzyset,i.e.,amembership degreethatrepresentsanimportancedegree(arelativeweight)betweenzeroandoneisassignedto eachoftheexperts.Thismakesthedecisionprocessmorerealisticandreducesthebiasedinformation givenbytheexperts.
8.Conclusions
WeintroducedtheconceptofFP-CNSES,whichisaFP-SVNSESdefinedinacomplexsetting. WedefinedFP-CNSESoperationsandtheirproperties.Wethenstudiedamappingonfuzzy parameterizedcomplexneutrosophicsoftexpertclassesanditsproperties.Anadjustableapproachto decisionmakingproblemsbasedonFP-CNSESwasalsointroduced.AcomparisonoftheFP-IFSES andFP-SVNSEStoFP-CNSESwasmadeandthepreferabilityofFP-CNSESwasrevealed.Finally, wedefinedthenotionofWFP-CNSESwhereexperts’relativeweightswereconsideredandapplied ittosolveadecisionmakingproblem.Thisadjustableapproachtodecisionmakingcanbehelpful todealwithmoreroomofuncertaintycomparedtousingFP-CNSESonthesameproblem.Boththe newlyproposedapproachesefficientlycapturetheincomplete,indeterminate,andinconsistent informationandextendexistingdecision-makingmethodstoprovideamorecomprehensiveoutlook fordecision-makers.WFP-CNSESseemstobeapromisingnewconcept,pavingthewaytoward numerouspossibilitiesforfutureresearch.Weintendtoinvestigatethisconceptfurtherbystudying itsoperationsandtheirpropertiestodevelopsomerealapplications.
AuthorContributions: A.A.-Q.proposedtheconceptofthefuzzyparameterizedcomplexneutrosophicsoft expertset;N.H.providedaconcreteexampleonthedecisionmakingproblem;A.A.-Q.andS.A.proposedthe FP-CNSESdecisionmakingmethod;andN.H.editedthemanuscript.Allauthorswrotethemanuscript.
Funding: UniversitiKebangsaanMalaysiaGUP-2017-105.
Acknowledgments: WeareindebtedtoUniversitiKebangsaanMalaysiaforprovidingfinancialsupportand facilitiesforthisresearchunderthegrantGUP-2017-105.
ConflictsofInterest: Authorsdeclarethattheyhavenoconflictofinterest.
References
1. Zadeh,L.A.Fuzzysets. Inf.Control. 1965, 8,338–353.[CrossRef]
2. Atanassov,K.Intuitionisticfuzzysets. FuzzySetsSyst. 1986, 20,87–96.[CrossRef]
3. Smarandache,F. Neutrosophy:NeutrosophicProbability,Set,andLogic; AmericanResearchPress:Rehoboth,IL, USA,1998.
4. Smarandache,F.Neutrosophicset—Ageneralisationoftheintuitionisticfuzzysets. Int.J.Pure.Appl.Math. 2005, 24,287–297.
5. Wang,H.;Smarandache,F.;Zhang,Y.;Sunderraman,R.Singlevaluedneutrosophicsets. Multisp.Multistruct. 2010, 4,410–413.
6. Molodtsov,D.Softsettheory:Firstresults. Comput.Math.Appl. 1999, 37,19–31.[CrossRef]
7. Alkhazaleh,S.;Salleh,A.R.Softexpertsets. Adv.Decis.Sci. 2011, 2011,757868.[CrossRef]
Symmetry 2019, 11,382
8. Alkhazaleh,S.;Salleh,A.R.Fuzzysoftexpertsetanditsapplication. Appl.Math. 2014, 5,1349–1368. [CrossRef]
9. Broumi,S.;Smarandache,F.Intuitionisticfuzzysoftexpertsetsanditsapplicationindecisionmaking. J.NewTheory 2015, 1,89–105.
10. Broumi,S.;Smarandache,F.Singlevaluedneutrosophicsoftexpertsetsandtheirapplicationindecision making. J.NewTheory 2015, 3,67–88.
11. Al-Quran,A.;Hassan,N.Neutrosophicvaguesoftexpertsettheory. J.Intell.FuzzySyst. 2016, 30,3691–3702. [CrossRef]
12. AbuQamar,M.;Hassan,N.GeneralizedQ-neutrosophicsoftexpertsetfordecisionunderuncertainty. Symmetry 2018, 10,621.[CrossRef]
13. Ulucay,V.;Sahin,M.;Hassan,N.Generalizedneutrosophicsoftexpertsetformultiple-criteria decision-making. Symmetry 2018, 10,437.[CrossRef]
14. Cagman,N.;Citak,F.;Enginoglu,S.FP-softsettheoryanditsapplications. Ann.FuzzyMath.Inform. 2011, 2,219–226.
15. Bashir,M.;Salleh,A.R.Fuzzyparameterizedsoftexpertset. Abstr.Appl.Anal 2012, 2012,258361.[CrossRef]
16. Hazaymeh,A.;Abdullah,I.B.;Balkhi,Z.;Ibrahim,R.Fuzzyparameterizedfuzzysoftexpertset. Appl.Math.Sci 2012, 6,5547–5564.
17. Selvachandran,G.;Salleh,A.R.Fuzzyparameterizedintuitionisticfuzzysoftexpertsettheoryandits applicationindecisionmaking. Int.J.SoftComput. 2016, 11,52–63.
18. Al-Quran,A.;Hassan,N.Fuzzyparameterisedsinglevaluedneutrosophicsoftexpertsettheoryandits applicationindecisionmaking. Int.J.Appl.Decis.Sci. 2016, 9,212–227.
19. Ramot,D.;Milo,R.;Friedman,M.;Kandel,A.Complexfuzzysets. IEEETrans.FuzzySyst. 2002, 10,171–186. [CrossRef]
20. Hu,B.;Bi,L.;Dai,S.Theorthogonalitybetweencomplexfuzzysetsanditsapplicationtosignaldetection. Symmetry 2017, 9,175.[CrossRef]
21. Alkouri,A.;Salleh,A.R.Complexintuitionisticfuzzysets. AIPConf.Proc 2012, 1482,464–470.
22. Ali,M.;Smarandache,F.Complexneutrosophicset. NeuralComput.Appl. 2017, 28,1817–1834.[CrossRef]
23.
24.
Al-Quran,A.;Hassan,N.Thecomplexneutrosophicsoftexpertsetanditsapplicationindecisionmaking. J.Intell.Fuzzy.Syst 2018, 34,569–582.[CrossRef]
Al-Quran,A.;Hassan,N.Thecomplexneutrosophicsoftexpertrelationanditsmultipleattribute decision-makingmethod. Entropy 2018, 20,101.[CrossRef]
c 2019bytheauthors.LicenseeMDPI,Basel,Switzerland.Thisarticleisanopenaccess articledistributedunderthetermsandconditionsoftheCreativeCommonsAttribution (CCBY)license(http://creativecommons.org/licenses/by/4.0/).
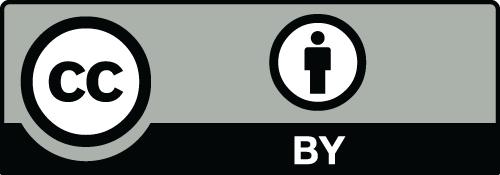