Paper:
CorrelationCoefficientsofRefined-SingleValuedNeutrosophic
SetsandTheirApplicationsinMultipleAttribute Decision-Making
ChangxingFan
DepartmentofComputerScience,ShaoxingUniversity 508HuanchengWestRoad,Shaoxing,Zhejiang312000,China E-mail:fcxjszj@usx.edu.cn [ReceivedJuly21,2018;acceptedOctober19,2018]
Thepaperpresentsthecorrelationcoefficientof refined-singlevaluedneutrosophicsets(RefinedSVNSs)basedontheextensionofthecorrelationof singlevaluedneutrosophicsets(SVNSs),andthena decisionmakingmethodisproposedbytheuseof theweightedcorrelationcoefficientofRefined-SVNSs. Throughtheweightedcorrelationcoefficientbetween theidealalternativeandeachalternative,wecanrank allalternativesandthebestoneofallalternativescan beeasilyidentifiedaswell.Finally,toprovethisdecisionmakingmethodproposedinthispaperisuseful todealwiththeactualapplication,weuseanexample toillustrateit.
efficientsofRefined-SVNSsanduseadecisionmaking exampletoprovethismethodinthispaper.
Therestorganizationsofthispaperareasfollows.Section2:brieflyintroducesNS,SVNSandRefined-SVNS. Section3:introducestheCorrelationCoefficientsand theCorrelationCoefficientsofRefined-SVNS.Section4: givesadecisionmakingmethodbasedontheweighted correlationcoefficientmeasuresofRefined-SVNSs.Section5:presentsanexamplewithRefined-SVNStoillustratetheproposedmethodsandgivesaconclusion.Finally,Section6:concludes.
2.SomeConceptsofNS,SVNS,andRefinedSVNS
Keywords: refined-SVNSs,correlationcoefficients,decisionmaking,SVNSs
1.Introduction
Neutrosophicset(NS)proposedbySmarandache[1]is animportanttooltosolvemulti-criteriadecisionmaking problems.Sincethen,manynewextensionsaboutincomplete,uncertainandimpreciseinformationhavebeenpresented.Forexamples,in2005,Wangetal.[2]introduced theconceptofanintervalneutrosophicset(INS).Thesinglevaluedneutrosophicset(SVNS)wasintroducedfor thefirsttimebySmarandachein1998inhisbook[3];reviewedin[4],whichisalsomentionedbyWangetal.[5] in2010.In2013,Smarandacherefinedtheneutrosophic set:truthvalue T isrefinedintotypesofsub-truthssuch as T1 , T2 ,etc.,similarlyindeterminacy I issplit/refined intotypesofsub-indeterminacies I1 , I2 ,etc.,andthesubfalsehood F issplitinto F1 , F2 ,etc.Therefore,Smarandache[6]introducedtheconceptofaRefined-SVNS.Ina decisionmakingproblem,ifthegivencriteriahavemany sub-criteria,wewillsubdividethesecriteria.In2014, Ye[7]presentedaconceptofasimplifiedneutrosophicset (SNS).NowINS,SNS,andSVNShavebeendeveloped bymanyresearchersinvariousfields[8–22].Butthere arefewstudiesandresearchesontheRefined-SVNS.So weproposeadecisionmakingmethodoncorrelationco-
Definition1 [1].Set X beauniverseofdiscourse,witha genericelementdenoted x in X .ThenaNSisdefinedas:
A = x, TA (x), IA (x), FA (x) x ∈ X ,
inwhich TA (x) : X → ] 0, 1+ [ meanstruthmembership function, IA (x) : X → ] 0, 1+ [ meansindeterminacymembershipand FA (x) : X → ] 0, 1+ [ meansfalsitymembershipfunction.Thefunctions TA (x), IA (x),and FA (x) are realstandardornonstandardsubsetsof ] 0, 1+ [ andthere isnorelationonthesumof TA (x), IA (x),and FA (x),so 0 ≤ sup TA (x)+ sup IA (x)+ sup FA (x) ≤ 3+ .
Obviously,justusingthisDefinition1,wecannotapply theneutrosophicsettodealwiththepracticalproblems. Therefore,Smarandache[3]introducedtheconceptofa SVNS,whichisanextensionofNS.
Definition2 [3].Set X beauniverseofdiscourse,witha genericelementdenoted x in X .ThenaSVNSisdefined as:
A = x, TA (x), IA (x), FA (x) x ∈ X ,
inwhich TA (x), IA (x), FA (x) ∈ [0, 1],0 ≤ TA (x)+ IA (x)+ FA (x) ≤ 3.
Whenwedealthepracticalproblem,thegivencriteria maybehavemanysub-criteria,weshouldsubdividethese criteria.Therefore,Smarandache[6]introducedthecon-
ceptofaRefined-SVNS.
Definition3 [6].Set X beauniverseofdiscourse,witha genericelementdenoted x in X .ThenaRefined-SVNSis definedas:
A = x, T1A (x), T2A (x),..., TkA (x) , I1A (x), I2A (x),..., IkA (x) , F1A (x), F2A (x),..., FkA (x) x ∈ X
here k isapositiveinteger, T1A (x), T2A (x),..., TkA (x) ∈ [0, 1], I1A (x), I2A (x),..., IkA (x) ∈ [0, 1], F1A (x), F2A (x),..., FkA (x) ∈ [0, 1],and0 ≤ TiA (x)+ IiA (x)+ FiA (x) ≤ 3for i = 1, 2,..., k
Definition4 [14].Set X beauniverseofdiscourse,and L and M betwoSVNSs, L = { x, TL (xi ), IL (xi ), FL (xi ) | xi ∈ X } and M = { x, TM (xi ), IM (xi ), FM (xi ) | xi ∈ X }.The correlationcoefficientsmeasureoftwoSVNSs L and M is: N (L, M )= C (L, M ) max{C (L, L), C (M , M )} = n ∑ k =1 TL (xi ) TM (xi )+ IL (xi ) IM (xi )+ FL (xi ) FM (xi ) max n ∑ k =1 T 2 L (xi )+ I 2 L (xi )+ F 2 L (xi ) , n ∑ k =1 T 2 M (xi )+ I 2 M (xi )+ F 2 M (xi ) . ....(1)
Theorem1. Thecorrelationcoefficientsmeasure N (L, M ) satisfiesthefollowingproperties(1)–(3)[14]:
(1)0 ≤ N (L, M ) ≤ 1;
(2) N (L, M )= 1ifandonlyif L = M ; (3) N (L, M )= N (M , L); Theirproofscanbeconsultedin[14].
3.CorrelationCoefficientsMeasureMethods ofRefined-SVNSs
Definition5. Let X = {x1 , x2 ,..., xn } beauniverseof discourse,and L and M betwoRefined-SVNSs, L = { xi , (T1L (xi ), T2L (xi ),..., Tki L (xi )), (I1L (xi ), I2L (xi ),..., Iki L (xi )), (F1L (xi ), F2L (xi ),..., Fki L (xi )) |xi ∈ X }, M = { xi , (T1M (xi ), T2M (xi ),..., Tki M (xi )), (I1M (xi ), I2M (xi ),..., Iki M (xi )), (F1M (xi ), F2M (xi ),..., Fki M (xi )) |xi ∈ X }
Here ki isapositiveinteger,andall T jL (xi ), I jL (xi ), FjL (xi ) and T jM (xi ), I jM (xi ), FjM (xi ) ∈ [0, 1](i = 1, 2,..., n; j = 1, 2,..., ki )
AsanextensionofDefinition4,wepresentacorrelationcoefficientsmeasurebetweentwoRefined-SVNSs L and M asfollows:
N (L, M )= C (L, M ) max{C (L, L), C (M , M )} = n ∑ i=1
ki ∑ j =1 T jL (xi ) · T jM (xi )+ I jL (xi ) · I jM (xi ) +FjL (xi ) T jM (xi ) ki max n ∑ i=1
ki ∑ j =1 T jL 2 (xi )+ I jL 2 (xi )+ FjL 2 (xi ) ki , n ∑ i=1
ki ∑ j =1 T jM 2 (xi )+ I jM 2 (xi )+ FjM 2 (xi ) ki ...................(2)
Theorem1. Thecorrelationcoefficientsmeasure N (L, M ) betweentwoRefined-SVNSs L and M satisfies thefollowingproperties:
(1) N (L, M )= N (M , L); (2)0 ≤ N (L, M ) ≤ 1; (3) N (L, M )= 1ifandonlyif L = M ;
Proof.
(1)For T jL (xi ) T jM (xi )+I jL (xi ) I jM (xi )+ FjL (xi ) FjM (xi )= T jM (xi ) T jL (xi )+ I jM (xi ) I jL (xi )+ FjM (xi ) FjL (xi ),sowecanget N (L, M )= N (M , L)
ki ∑ j =1 T jL (xi ) T jM (xi )+ I jL (xi ) I jM (xi ) +FjL (xi ) · FjM (xi ) ki
1
Table1. TheRefined-SVNSdecisionmatrix D ) ) get C (L, M )2 ≤ 1 k1 2 T1L 2 (x1 )+ I1L 2 (x1 )+ F1L 2 (x1 ) T1M 2 (x1 )+ I1M 2 (x1 )+ F1M 2 (x1 ) + ··· + Tk1 L 2 (x1 )+ Ik1 L 2 (x1 )+ Fk1 L 2 (x1 ) Tk1 M 2 (x1 )+ Ik1 M 2 (x1 )+ Fk1 M 2 (x1 ) + ··· + 1 kn 2 T1L 2 (x1 )+ I1L 2 (x1 )+ F1L 2 (x1 ) ·
T1M 2 (x1 )+ I1M 2 (x1 )+ F1M 2 (x1 ) + +(Tkn L 2 (x1 )+ Ikn L 2 (x1 )+ Fkn L 2 (x1 ) · Tkn M 2 (x1 )+ Ikn M 2 (x1 )+ Fkn M 2 (x1 ) = C (L, L)C (M , M )
For C (L, M )2 ≤ C (L, L)C (M , M ),just C (L, M ) ≤ C (L, L)1/2C (M , M )1/2 . Then, C (L, M ) ≤ max{C (L, L), C (M , M )}. Sowecanget N (L, M )= C (L, M ) /max{C (L, L), C (M , M )}≤ 1.
(3)If L = M then T jL (xi )= T jM (xi )I jL (xi )= I jM (xi ),and FjL (xi )= FjM (xi ) forany xi ∈ X and i = 1, 2,..., n,sowe canget N (L, M )= 1,ifandonlyif L = M
Usually,allattributeshaveweights,maybethese weightsareatthesamevaluesordifferent,buttheyare allbelong0to1andthetotalvalueofthemis1.Now,we assumethattheweightofeachattribute Ci (i = 1, 2,..., n) is wi .Then,wecanintroducetheweightedcorrelationcoefficientsmeasurebetweentwoRefined-SVNSs L and M
4.BuildingaDecision-MakingModelUsing theCorrelationCoefficients
Inadecision-makingproblem,thereareasetofalternatives A = {A1 , A2 ,..., Am } andasetofattributes C = {C1 , C2 ,..., Cn }.Sometimes Ci (i = 1, 2,..., n) maybe subdividedintosomesub-attribute Cij (i = 1, 2,..., n, j = 1, 2,..., ki ),thenwecanuseaRefined-SVNStoexpress it: Ar = Ci , (T1Ar (Ci ), T2Ar (Ci ),..., Tki Ar (Ci )), (I1Ar (Ci ), I2Ar (Ci ),..., Iki Ar (Ci )), (F1Ar (Ci ), F2Ar (Ci ),..., Fki Ar (Ci )) Ci ∈ C , r = 1, 2,..., m and i = 1, 2,..., n.
(4)
WecoulduseaRefined-SVNStodenotethevaluesofthe threefunctions Tki Sr (Gi ), Iki Sr (Gi ), Fki Sr (Gi ) forconvenience,soweestablishtheRefined-SVNSdecisionmatrix D,whichisshownin Table1
Step1: BasedontheRefined-SVNSdecisionmatrix
, wecangettheidealsolution(idealRefined-SVNS) A
i Whentheattributesarebenefit, A
i isshownasfollows:
Table2. TheRefined-SVNSdecisionmatrix D forfouralternativesonthreeattributes/sevensub-attributes. ) ) )
Whentheattributesarecost, A∗ i isshownasfollows:
A∗ i = T1Am min , T2Am min ,..., Tki Am min , I1Am min , I2Am min ,..., Iki Am min , F1Am min , F2Am min ,..., Fki Am min , for i = 1, 2,..., n
....(6)
Sowecangettheidealalternative A∗ = {A∗ 1 , A∗ 2 ,..., A∗ n }
Step2: Whentheweightsofattributesaregivenby w = (w1 , w2 ,..., wn ) with wi ≥ 0and ∑n i=1 wi = 1.Thecorrelationcoefficientsmeasurebetweentheidealalternative A∗ andeachalternative Ar (r = 1, 2,..., m) canbecalculatedaccordingtoEqs.(4)and(5).Thenwecanobtain thevaluesof W (Ar , A∗ ) for r = 1, 2,..., m.
Step3: Accordingthevaluesof W (Ar , A∗ ) for r = 1, 2,..., m,allalternativescanberankedinadescending orderandthealternativeofbiggest W (Ar , A∗ ) valuejust isthebestchoice.
Step4: End.
5.IllustrativeExamples
Inthissection,wegivetwoexampleswithmultiple attributetodemonstratetheapplicationoftheproposed methodinthispaper.
5.1.Example1
Now,wediscussthedecision-makingproblemadapted from[22].Aconstructioncompanywantstodetermine theselectingproblemofconstructionprojects.Now fourconstructionprojectsareprovidedbydecisionmakers,thenwecangetasetoffouralternatives A = A1 , A2 , A3 , A4 .Then,intheseconstructionprojects,which onecanbeselecteddependentonthreemainattributes. Theseattributesarefinancialstate(C1 ),environmental protection(C2 )andtechnology(C3 ),andatthesametime theseattributescanbedividedintosevensub-attributes: budgetcontrol(C11 )andrisk/returnratio(C12 );publicrelation(C21 ),geographicallocation(C22 ),andhealthand safety(C23 );technicalknowhow(C31 )andtechnologicalcapability(C32 ).Then,decisionmakersevaluatethe valueofthefourpossiblealternativesundertheabove attributesbysuitabilityjudgments.Withthesevalues
wecanconstructtheRefined-SVNSdecisionmatrix D, whichisshownin Table2.
Fortheseattributesarebenefit,sowecanobtainthe idealalternative A∗ byusingEq.(4)fromtheRefinedSVNSdecisionmatrix D
A∗ = { (0.8, 0.8), (0.1, 0.1), (0.2, 0.2) , (0 9, 0 8, 0 8), (0 1, 0 1, 0 1), (0 1, 0 1, 0 1) , (0 8, 0 8), (0 1, 0 2), (0 1, 0 1) }
Withtheweightvectorofthethreeattributesby w = (0 4, 0 3, 0 3) ontheopinionoftheexpertsandEq.(3), wecanobtaintheweightedcorrelationcoefficientsmeasurevaluesbetweentheidealalternation A∗ andeachalternative Ar (r = 1, 2, 3, 4),themeasurevaluesarelisted asfollows: W (A1 , A∗ )= 0 9156, W (A2 , A∗ )= 0 9603, W (A3 , A∗ )= 0.9308, and W (A4 , A∗ )= 0.8861. Becauseofthemeasurevaluesare W (A2 , A∗ ) > W (A3 , A∗ ) > W (A1 , A∗ ) > W (A4 , A∗ ),therankingorder justis A2 A3 A1 A4 .Therefore,wecangetthealternative A2 asthebestchoiceamongallalternatives.
Comparingwiththemethodof[22],thecorrelationcoefficientsmeasurebetweentwoRefined-SVNSsproposed inthispaperisrelativelysimplerandeasier,andwecan obtainthesamechoiceasin[22]throughtheweighted correlationcoefficientsmeasurevaluesbetweentheideal alternation A∗ andeachalternative Ar (r = 1, 2, 3, 4)
5.2.Example2
Auniversitywantstoranktheacademywithsome mainattributes.Nowtherearefiveacademieswillbe ranked,thenwecangetasetoffiveacademics A = {A1 , A2 , A3 , A4 , A5 }.Therankoftheseacademicsdependsonthreemainattributesandsevensub-attributes: (1)Teaching(C1 ):teachingconditions(C11 ),teachers troop(C12 )andteachinglevel(C13 );(2)Thescientificresearch(C2 ):teachers’scientificresearch(C21 ),students’ scientificresearch(C22 );(3)Server(C3 ):socialreputation (C31 ),theemploymentsituation(C32 ).
Expertsevaluatethevalueofthefiveacademicsunder theaboveattributesbysomedata.Withthesevalueswe canconstructtheRefined-SVNSdecisionmatrix,which isshownin Table3.
Fortheseattributesarebenefit,sowecanobtain theidealalternative A*byusingformula(4)fromthe
Table3. TheRefined-SVNSdecisionmatrix D forfiveacademicsonthreeattributes/sevensub-attributes. ) ) )
Refined-SVNSdecisionmatrix D
A∗ = { (0.8, 0.9, 0.8), (0.2, 0.2, 0.1), (0.1, 0.1, 0.2) , (0 9, 0 7), (0 1, 0 1), (0 2, 0 3) , (0 8, 0 9), (0 1, 0 2), (0 1, 0 1) } W (A1 , A∗ )= 0 9950, W (A2 , A∗ )= 0 9207, W (A3 , A∗ )= 0.8779, W (A4 , A∗ )= 0.8987, W (A5 , A∗ )= 0 8947
So,therankingoffiveacademicsis A1 A2 A4 A5 A3 .Therefore,theacademicnamed A1 isthebest oneofallevaluatedacademics.
Fromabovetwoexamples,wecanseethatthecorrelationcoefficientsofrefined-neutrosophicsetcanbeused inactualengineeringandscientificapplicationstohelp peopletodosomedecisionproblems.
6.Conclusions
Wepresentedthecorrelationcoefficientsmeasureof Refined-SVNSsinthispaperandweusethismethod todealwithtwoactualdecision-makingapplications. Throughthecorrelationcoefficientsmeasurebetweenthe idealalternativeandeachalternative,therankingorder ofallalternativescanbegotandthebestalternativecan beselectedaswell.Finally,therankingorderinthefirst examplewithcorrelationcoefficientsmeasureisprobablyagreewiththerankingresultsof[22],thesecondexamplewithcorrelationcoefficientsmeasurecangetthe rankoffiveevaluatedacademics,sothemethodproposedinthispaperissuitableforactualapplicationsin decision-makingproblemswithRefined-SVNS.Inthefuture,weshallgoonstudyingthecorrelationcoefficients measurebetweenRefined-SVNSsandextendingtheproposeddecision-makingmethodtomanyotherfields.
Acknowledgements
ThisresearchwasfundedbytheNationalNaturalScienceFoundationofChinagrantnumber[61603258],[61703280];GeneralResearchProjectofZhejiangProvincialDepartmentof Educationgrantnumber[Y201839944];PublicWelfareTechnologyResearchProjectofZhejiangProvincegrantnumber [LGG19F020007];PublicWelfareTechnologyApplicationResearchProjectofShaoxing Citygrantnumber[2018C10013].
References:
[1]F.Smarandache,“Neutrosophy:Neutrosophicprobability,set,and logic,”AmericanResearchPress,1998.
[2]H.Wang,F.Smarandache,Y.Q.Zhang,andR.Sunderraman,“Intervalneutrosophicsetsandlogic:Theoryandapplicationsincomputing,”Hexis,2005.
[3]F.Smarandache,“Aunifyingfieldinlogics.InNeutrosophy:NeutrosophicProbability,SetandLogic,”AmericanResearchPress, 1999.
[4]F.Smarandache,“Neutrosophy.Neutrosophicprobability,set,and logic.Analyticsynthesisandsyntheticanalysis,”AmericanResearchPress,p.105,1998.
[5]H.Wang,F.Smarandache,Y.Q.Zhang,andR.Sunderraman,“Singlevaluedneutrosophicsets,”MultispaceandMultistructure,Vol.4, pp.410-413,2010.
[6]F.Smarandache,“n-ValuedRefinedNeutrosophicLogicandIts ApplicationsinPhysics,”ProgressinPhysics,Vol.4,pp.143-146, 2013.
[7]J.Ye,“Amulticriteriadecision-makingmethodusingaggregation operatorsforsimplifiedneutrosophicsets,”J.ofIntelligentand FuzzySystems,Vol.26,pp.2459-2466,2014.
[8]J.YeandF.Smarandache,“SimilarityMeasureofRefinedSingleValuedNeutrosophicSetsandItsMulticriteriaDecisionMaking Method,”NeutrosophicSetsandSystems,Vol.12,pp.41-44,2016.
[9]J.Ye,“Similaritymeasuresbetweenintervalneutrosophicsetsand theirapplicationsinmulticriteriadecision-making,”J.ofIntelligent andFuzzySystems,Vol.26,pp.165-172,2014.
[10]J.Ye,“Multicriteriadecision-makingmethodusingthecorrelation coefficientundersingle-valuedneutrosophicenvironment,”Int.J. ofGeneralSystems,Vol.42,pp.386-394,2013.
[11]S.YeandJ.Ye,“Dicesimilaritymeasurebetweensinglevalued neutrosophicmultisetsanditsapplicationinmedicaldiagnosis,” NeutrosophicSetsandSystem,Vol.6,pp.49-54,2014.
[12]H.-Y.Zhang,J.-Q.Wang,andX.-H.Chen,“Intervalneutrosophic setsandtheirapplicationinmulticriteriadecisionmakingproblems,”TheScientificWorldJ.,2014.
[13]J.Ye,“Improvedcosinesimilaritymeasuresofsimplifiedneutrosophicsetsformedicaldiagnoses,”ArtificialIntelligencein Medicine,Vol.63,No.3,pp.171-179,2015.
[14]J.Ye,“AnotherFormofCorrelationCoefficientbetweenSingleValuedNeutrosophicSetsandItsMultipleAttributeDecisionMaking Method,”NeutrosophicSetsandSystems,Vol.1,pp.8-13,2013.
[15]C.X.FanandJ.Ye,“TheCosineMeasureofRefined-SingleValuedNeutrosophicSetsandRefined-IntervalNeutrosophicSetsfor MultipleAttributeDecision-Making,”J.Intell.FuzzySyst.,Vol.33, pp.2281-2289,2017.
[16]S.Broumi,M.Talea,F.Smarandache,andA.Bakali,“SingleValued NeutrosophicGraphs:Degree,OrderandSize,”IEEEInt.Conf.on FuzzySystems(FUZZ),pp.2444-2451,2016.
[17]S.Broumi,A.Bakali,M.Talea,andF.Smarandache,“IsolatedSingleValuedNeutrosophicGraphs,” NeutrosophicSetsandSystems, Vol.11,pp.74-78,2016.
[18]S.Broumi,F.Smarandache,M.Talea,andA.Bakali,“DecisionMakingMethodBasedontheIntervalValuedNeutrosophicGraph,” 2016FutureTechnologiesConf.(FTC),pp.44-50,2016.
[19]S.Broumi,A.Bakali,M.Talea,F.Smarandache,andL.Vladareanu,“ComputationofShortestPathProbleminaNetworkwithSVTrapezoidalNeutrosophicNumbers,”Proc.ofthe2016Int.Conf. onAdvancedMechatronicSystems,pp.417-422,2016.
[20]S.Broumi,A.Bakali,M.Talea,F.Smarandache,andL.Vladareanu,“ApplyingDijkstraAlgorithmforSolvingNeutrosophicShortestPathProblem,”Proc.ofthe2016Int.Conf.onAdvancedMechatronicSystems,No.30,pp.412-416,2016.
Fan,C.
[21]C.X.Fan,E.Fan,andJ.Ye,“TheCosineMeasureofSingle-Valued NeutrosophicMultisetsforMultipleAttributeDecision-Making,” Symmetry,Vol.10,Issue5,doi:10.3390/sym10050154,2018.
[22]J.YeandF.Smarandache,“SimilarityMeasureofRefinedSingleValuedNeutrosophicSetsandItsMulticriteriaDecisionMaking Method,”NeutrosophicSetsandSystems,Vol.12,pp.41-44,2016.
Name: ChangxingFan
Affiliation: DepartmentofComputerScience,Shaoxing University
Address: 508HuanchengWestRoad,Shaoxing,Zhejiang312000,China
BriefBiographicalHistory: 1996-JiangxiUniversityofScienceandTechnology 2000-ShaoxingUniversity 2006-TongjiUnivarsity MainWorks: • Intelligentinformationprocessing,Neutrosophicsettheory
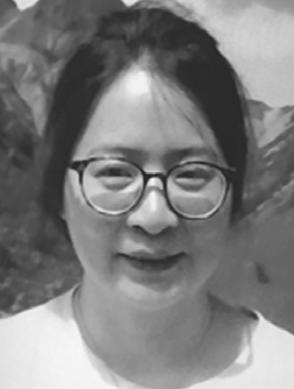
426JournalofAdvancedComputationalIntelligence
andIntelligentInformatics