Article
CertainCompetitionGraphsBasedonIntuitionistic NeutrosophicEnvironment
MuhammadAkram* ID andMaryamNasir
DepartmentofMathematics,UniversityofthePunjab,NewCampus,Lahore54590,Pakistan; maryamnasir912@gmail.com
* Correspondence:m.akram@pucit.edu.pk;Tel.:+92-42-99231241
Received:7September2017;Accepted:19October2017;Published:24October2017
Abstract: Theconceptofintuitionisticneutrosophicsetsprovidesanadditionalpossibilitytorepresent imprecise,uncertain,inconsistentandincompleteinformation,whichexistsinrealsituations.Thisresearch articlefirstpresentsthenotionofintuitionisticneutrosophiccompetitiongraphs.Then, p-competition intuitionisticneutrosophicgraphsand m-stepintuitionisticneutrosophiccompetitiongraphsarediscussed. Further,applicationsofintuitionisticneutrosophiccompetitiongraphsinecosystemandcareercompetition aredescribed.
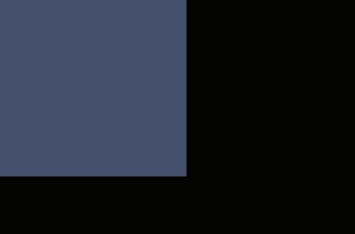
Keywords: intuitionisticneutrosophiccompetitiongraphs;intuitionisticneutrosophicopen-neighborhood graphs; p-competitionintuitionisticneutrosophicgraphs; m-stepintuitionisticneutrosophiccompetition graphs
MSC: 03E72;68R10;68R05
1.Introduction
Euler[1]introducedtheconceptofgraphtheoryin 1736,whichhasapplicationsinvariousfields, includingimagecapturing,datamining,clusteringandcomputerscience[2–5].Agraphisalsousedto developaninterconnectionbetweenobjectsinaknownsetofobjects.Everyobjectcanbeillustratedby avertex,andinterconnectionbetweenthemcanbeillustratedbyanedge.Thenotionofcompetition graphswasdevelopedbyCohen[6]in 1968,dependingonaprobleminecology.Thecompetition graphshavemanyutilizationsinsolvingdailylifeproblems,includingchannelassignment,modelingof complexeconomic,phytogenetictreereconstruction,codingandenergysystems.
Fuzzysettheoryandintuitionisticfuzzysetstheoryareusefulmodelsfordealingwithuncertainty andincompleteinformation.However,theymaynotbesufficientinmodelingofindeterminateand inconsistentinformationencounteredintherealworld.Inordertocopewiththisissue,neutrosophic settheorywasproposedbySmarandache[7]asageneralizationoffuzzysetsandintuitionisticfuzzy sets.However,sinceneutrosophicsetsareidentifiedbythreefunctionscalledtruth-membership (t), indeterminacy-membership (i) andfalsity-membership ( f ),whosevaluesaretherealstandardor non-standardsubsetofunitinterval ]0 ,1+ [.Therearesomedifficultiesinmodelingofsomeproblems inengineeringandsciences.Toovercomethesedifficulties,Smarandachein1998[8]andWangetal.[9] in2010definedtheconceptofsingle-valuedneutrosophicsetsandtheiroperationsasageneralizationof intuitionisticfuzzysets.Yangetal.[10]introducedtheconceptofthesingle-valuedneutrosophicrelation basedonthesingle-valuedneutrosophicset.Theyalsodevelopedkernelsandclosuresofasingle-valued neutrosophicset.Theconceptofthesingle-valuedintuitionisticneutrosophicsetwasproposedby BhowmikandPal[11,12].
Information 2017, 8,132;doi:10.3390/info8040132
www.mdpi.com/journal/information
Thevaluablecontributionoffuzzygraphandgeneralizedstructureshasbeenstudiedbyseveral researchers[13–22].Smarandache[23]proposedthenotionoftheneutrosophicgraphandseparatedthem intofourmaincategories.Wu[24]discussedfuzzydigraphs.Fuzzy m-competitionand p-competition graphswereintroducedbySamantaandPal[25].Samantaetal.[26]introduced m-stepfuzzycompetition graphs.Dhavaseelanetal.[27]definedstrongneutrosophicgraphs.AkramandShahzadi[28]introduced thenotionofasingle-valuedneutrosophicgraphandstudiedsomeofitsoperations.Theyalsodiscussed thepropertiesofsingle-valuedneutrosophicgraphsbylevelgraphs.AkramandShahzadi[29]introduced theconceptofneutrosophicsoftgraphswithapplications.Broumietal.[30]proposedsingle-valued neutrosophicgraphsanddiscussedsomeproperties.Ye[31–33]haspresentedseveralnovelconcepts ofneutrosophicsetswithapplications.Inthispaper,wefirstintroducetheconceptofintuitionistic neutrosophiccompetitiongraphs.Wethendiscuss m-stepintuitionisticneutrosophiccompetitiongraphs. Further,wedescribeapplicationsofintuitionisticneutrosophiccompetitiongraphsinecosystemand careercompetition.Finally,wepresentourdevelopedmethodsbyalgorithms.
Ourpaperisdividedintothefollowingsections:InSection 2,weintroducecertaincompetition graphsusingtheintuitionisticneutrosophicenvironment.InSection 3,wepresentapplicationsof intuitionisticneutrosophiccompetitiongraphsinecosystemandcareercompetition.Finally,Section 4 providesconclusionsandfutureresearchdirections.
2.IntuitionisticNeutrosophicCompetitionGraphs
Wehaveusedstandarddefinitionsandterminologiesinthispaper.Forothernotations,terminologies andapplicationsnotmentionedinthepaper,thereadersarereferredto[34–44].
Definition1. [38]Let X beafixedset.Ageneralizedintuitionisticfuzzyset I of X isanobjecthavingthe form I={(u, µ I (u), νI (u))|u ∈ U},wherethefunctions µ I (u) :→ [0,1] and νI (u) :→ [0,1] definethedegreeof membershipanddegreeofnon-membershipofanelementu ∈ X,respectively,suchthat:
min{µ I (u), νI (u)}≤ 0.5,forallu ∈ X.
Thisconditioniscalledthegeneralizedintuitionisticcondition.
Definition2. [11]Anintuitionisticneutrosophicset(IN-set)isdefinedas ˘ A =(w,tA(w),iA(w),fA(w)),where: t ˘ A (w) ∧ f ˘ A (w) ≤ 0.5, tA (w) ∧ iA (w) ≤ 0.5, i ˘ A (w) ∧ f ˘ A (w) ≤ 0.5, forall,w ∈ X,suchthat: 0 ≤ t ˘ A (w)+ i ˘ A (w)+ f ˘ A (w) ≤ 2.
Definition3. [12]Anintuitionisticneutrosophicrelation(IN-relation)isdefinedasanintuitionisticneutrosophic subsetofX × Y,whichhasoftheform: R = {((w,z),tR (w,z),iR (w,z),fR (w,z)) : w ∈ X,z ∈ Y}, wheretR,iR andfR areintuitionisticneutrosophicsubsetsofX × Ysatisfyingtheconditions: 1. oneofthesetR (w,z),iR (w,z) andfR (w,z) isgreaterthanorequalto 0.5, 2. 0 ≤ tR (w, z)+ iR (w, z)+ fR (w, z) ≤ 2
Definition4. Anintuitionisticneutrosophicgraph(IN-graph) G =(X, h, k) (inshort G)on X (vertexset)is atripletsuchthat:
1. tk (w, z) ≤ th (w) ∧ th (z),ik (w, z) ≤ ih (w) ∧ ih (z),fk (w, z) ≤ fh (w) ∨ fh (z), 2. tk (w, z) ∧ ik (w, z) ≤ 0.5,tk (w, z) ∧ fk (w, z) ≤ 0.5,ik (w, z) ∧ fk (w, z) ≤ 0.5, 3. 0 ≤ tk (w, z)+ ik (w, z)+ fk (w, z) ≤ 2,forallw, z ∈ X, where, th,ih andfh → [0,1]
denotethetruth-membership,indeterminacy-membershipandfalsity-membershipofanelementw ∈ Xand: tk,ik andfk → [0,1]
denotethetruth-membership,indeterminacy-membershipandfalsity-membershipofanelement (w, z) ∈ E (edgeset).
Wenowillustratethiswithanexample.
Example1. ConsiderIN-graph G onnon-emptysetX,asshowninFigure 1. a(0 1, 0 4, 0 5)
(0. 1, 0. 2, 0. 4) (0 .5 , 0 .2 , 0 .1) (0.1, 0.2, 0.3) (0 . 5, 0 . 2, 0 . 2) (0 5, 0 2, 0 1)
b(0 6, 0 3, 0 2) c(0 8, 0 3, 0 4) d(0 7, 0 4, 0 2)
Figure1. Intuitionisticneutrosophicgraph(IN-graph).
Definition5. Let −→ G beanintuitionisticneutrosophicdigraph(IN-digraph),thenintuitionisticneutrosophic out-neighborhoods(IN-out-neighborhoods)ofavertexwareanIN-set: N+ (w)=(X+ w ,t+ w ,i+ w ,f + w ), where, X+ w = {z|k1 −−−→ (w, z) > 0,k2 −−−→ (w, z) > 0,k3 −−−→ (w, z) > 0}, suchthat t+ w : X+ w → [0,1] definedby t+ w (z)= k1 −−−→ (w, z), i+ w : X+ w → [0,1] definedby i+ w (z)= k2 −−−→ (w, z) and f + z : X+ z → [0,1] definedbyf + w (z)= k3 −−−→ (w, z)
Definition6. Let −→ G beanIN-digraph,thentheintuitionisticneutrosophicin-neighborhoods(IN-in-neighborhoods) ofavertexwareanIN-set:
N (w)=(Xw ,tw ,iw ,fw ), where, Xw = {z|k1 −−−→ (z, w) > 0,k2 −−−→ (z, w) > 0,k3 −−−→ (z, w) > 0}, suchthat tw : Xw → [0,1] definedby tw (z)= k1 −−−→ (z, w), iw : Xw → [0,1] definedby iw (z)= k2 −−−→ (z, w) and fw : Xw → [0,1] definedbyfw (z)= k3 −−−→ (z, w)
2017, 8,132
Example2. Consider −→ G =(X, h, k) tobeanIN-digraph,suchthat, X = {a, b, c, d, e}, h = {(a, 0.5, 0.3, 0.1), (b, 0.6, 0.4, 0.2), (c, 0.8, 0.3, 0.1), (d, 0.1, 0.9, 0.4), (e, 0.4, 0.3, 0.6)} andk = {(−→ ab, 0.3, 0.3, 0.1), (−→ ae, 0.3, 0.2, 0.4), (−→ bc, 0.5, 0.2, 0.1), (−→ ed, 0.1, 0.2, 0.5), (−→ dc, 0.1, 0.2, 0.3), (−→ bd, 0.1, 0.3, 0.3)},asshowninFigure 2
(0 . 3 , 0 . 2 , 0 4) (0 3, 0 3, 0 1) (0 .5 , 0.2 , 0.1) (0 . 1 , 0 . 3 , 0 . 3) (0 1, 0 2, 0 5) (0. 1,0. 2,0. 3)
a(0 5, 0.3, 0 1) b(0 6, 0 4, 0 2) c(0 8, 0 3, 0 1) d(0 1, 0 9, 0 4) e(0 4, 0 3, 0 6)
Figure2. IN-digraph. Then, N+ (a)= {(b, 0.3, 0.3, 0.1), (e, 0.3, 0.2, 0.4)}, N+ (c)= ∅, N+ (d)= {(c, 0.1, 0.2, 0.3)}, and N (b)= {(a, 0.3, 0.3, 0.1)}, N (c)= {(b, 0.5, 0.2, 0.1), (d, 0.1, 0.2, 0.3)}.Similarly,wecancalculate IN-outandin-neighborhoodsoftheremainingvertices.
Definition7. TheheightofanIN-set ˘ A =(w,tA,iA,f A ) isdefinedas: H( ˘ A)=(sup w∈X t ˘ A (w), sup w∈X i ˘ A (w), inf w∈X f ˘ A (w))=(H1( ˘ A),H2( ˘ A),H3( ˘ A))
Forexample,theheightofanIN-set ˘ A = {(a, 0.5, 0.7, 0.2), (b, 0.1, 0.2, 1), (c, 0.3, 0.5, 0.3)} in X = {a,b,c} isH( ˘ A)=(0.5, 0.7, 0.2).
Definition8. Anintuitionisticneutrosophiccompetitiongraph(INC-graph) C(−→ G ) ofanIN-digraph −→ G =(X,h,k) isanundirectedIN-graph G =(X, h, k),whichhasthesameintuitionisticneutrosophicsetofverticesasin −→ G and hasanintuitionisticneutrosophicedgebetweentwovertices w, z ∈ X in C(−→ G ) ifandonlyif N+(w) ∩ N+(z) is anon-emptyIN-setin −→ G .Thetruth-membership,indeterminacy-membershipandfalsity-membershipvaluesofedge (w,z) in C(−→ G ) are: tk (w, z)=(th (w) ∧ th (z))H(N+ (w) ∩ N+ (z)), ik (w, z)=(ih (w) ∧ ih (z))H(N+ (w) ∩ N+ (z)), fk (w, z)=( fh (w) ∨ fh (z))H(N+ (w) ∩ N+ (z)), respectively.
Example3. Consider −→ G =(X, h, k) tobeanIN-digraph,suchthat, X = {a, b, c, d}, h = {(a, 0.1, 0.4, 0.5), (b, 0.6, 0.3, 0.2), (c, 0.8, 0.3, 0.4), (d, 0.7, 0.4, 0.2)} and k = {(−→ ab, 0.1, 0.2, 0.4), (−→ ac , 0.1, 0.2, 0.3), (−→ bc , 0.5, 0.2, 0.2), (−→ bd, 0.5, 0.2, 0.1), (−→ cd, 0.5, 0.2, 0.1)},asshowninFigure 3.
a(0 1, 0 4, 0 5)
(0. 1, 0. 2, 0. 4) (0 .5 , 0 .2 , 0 .1) (0 1, 0 2, 0 3) (0 5, 0 . 2, 0 . 2) (0 5, 0 2, 0 1)
b(0 6, 0 3, 0 2) c(0 8, 0 3, 0 4) d(0 7, 0 4, 0 2)
Figure3. IN-digraph.
Bydirectcalculations,wehaveTables 1 and 2 representingIN-outandin-neighborhoods,respectively.
Table1. IN-out-neighborhoods. w N+ (w) a{(b,0.1,0.2,0.4),(c,0.1,0.2,0.3)} b{(d,0.5,0.2,0.1)} c{(b,0.5,0.2,0.2),(d,0.5,0.2,0.1)} d ∅
Table2. IN-in-neighborhoods. w N (w) a ∅ b{(a,0.1,0.2,0.4),(c,0.1,0.2,0.3)} c{(a,0.1,0.2,0.3)} d{(b,0.5,0.2,0.1),(c,0.5,0.2,0.1)}
TheINC-graphofFigure 3 isshowninFigure 4
a(0 1, 0 4, 0 5)
(0 3, 0 06, 0 16) (0 01, 0 06, 0 2)
b(0 6, 0 3, 0 2) c(0 8, 0 3, 0 4) d(0.7, 0.4, 0.2)
Figure4. Intuitionisticneutrosophiccompetitiongraph(INC-graph). Therefore,thereisanedgebetweentwoverticesinINC-graph C(−→ G ),whosetruth-membership, indeterminacy-membershipandfalsity-membershipvaluesaregivenbytheaboveformula.
Definition9. ForanIN-graph G =(X, h, k),where h =(h1, h2, h3) and k =(k1, k2, k3),thenanedge (w, z), w,z ∈ Xiscalledindependentstrongif: 1 2 [h1(w) ∧ h1(z)] < k1(w, z), 1 2 [h2(w) ∧ h2(z)] > k2(w, z), 1 2 [h3(w) ∨ h3(z)] > k3(w, z)
Otherwise,itiscalledweak.
Theorem1. Suppose −→ G isanIN-digraph.If N+ (w) ∩ N+ (z) containsonlyoneelementof −→ G ,thentheedge (w,z) of C(−→ G ) isindependentstrongifandonlyif:
|[N+ (w) ∩ N+ (z)]|t > 0.5, |[N+ (w) ∩ N+ (z)]|i < 0.5, |[N+ (w) ∩ N+ (z)]| f < 0.5.
Proof. Suppose, −→ G isanIN-digraph.Suppose N+ (w) ∩ N+ (z)=(a, ˘ p, q, r),where ˘ p, q and r arethe truth-membership,indeterminacy-membershipandfalsity-membershipvaluesofeithertheedge (w, a) ortheedge (z, a),respectively.Here,
|[N+ (w) ∩ N+ (z)]|t = ˘ p = H1(N+ (w) ∩ N+ (z)), |[N+ (w) ∩ N+ (z)]|i = q = H2(N+ (w) ∩ N+ (z)), |[N+ (w) ∩ N+ (z)]| f = r = H3(N+ (w) ∩ N+ (z))
Then, k1(w, z)= ˘ p × [h1(w) ∧ h1(z)], k2(w, z)= q × [h2(w) ∧ h2(z)], k3(w, z)= r × [h3(w) ∨ h3(z)]
Therefore,theedge (w, z) in C(−→ G ) isindependentstrongifandonlyif ˘ p > 0.5, q < 0.5 and r < 0.5 Hence,theedge (w, z) of C(−→ G ) isindependentstrongifandonlyif:
|[N+ (w) ∩ N+ (z)]|t > 0.5, |[N+ (w) ∩ N+ (z)]|i < 0.5, |[N+ (w) ∩ N+ (z)]| f < 0.5.
WeillustratethetheoremwithanexampleasshowninFigure 5.
a(0 7, 0 5, 0 4)
b(0 8, 0 4, 0 5)
a(0 7, 0 5, 0 4)
b(0 8, 0 4, 0 5)
c(0 8, 0 5, 0 4) d(0 3, 0 4, 0 5)
(0 6, 0 4, 0 4) (0 . 7 , 0 3 , 0 3) (0 2, 0 3, 0 4) (0 . 2 , 0 3 , 0 . 3) (b) (a)
(0 .42 , 0 .15 , 0 .12)
d(0 3, 0 4, 0 5) c(0 8, 0 5, 0 4)
Figure5. INC-graph.(a)IN-digraph;(b)correspondingINC-graph. Theorem2. IfalltheedgesofanIN-digraph −→ G areindependentstrong,then:
k1(w, z) (h1(w) ∧ h1(z))2 > 0.5, k2(w, z) (h2(w) ∧ h2(z))2 < 0.5, k3(w, z) (h3(w) ∨ f3(z))2 < 0.5 foralledges (w,z) in C(−→ G )
Proof. SupposealltheedgesofIN-digraph −→ G areindependentstrong.Then:
1 2 [h1(w) ∧ h1(z)] < k1 −−−→ (w, z), 1 2 [h2(w) ∧ h2(z)] > k2 −−−→ (w, z), 1 2 [h3(w) ∨ h3(z)] > k3 −−−→ (w, z),
foralltheedges (w, z) in −→ G .LetthecorrespondingINC-graphbe C(−→ G ).
Case(1):When N+ (w) ∩ N+ (z)= ∅ forall w, z ∈ X,thentheredoesnotexistanyedgein C(−→ G ) between w and z.Thus,wehavenothingtoproveinthiscase.
Case(2):When N+(w) ∩ N+(z) = ∅,let N+(w) ∩ N+(z)= {(a1, m1, n1, ˘ p1), (a2, m2, n2, ˘ p2),..., (al, ml, nl, ˘ pl )},where mi, ni and ˘ pi arethetruth-membership,indeterminacy-membershipand falsity-membershipvaluesofeither −−−→ (w, ai) or −−−→ (z, ai) for i = 1,2,..., l,respectively.Therefore, mi =[k1 −−−→ (w, ai ) ∧ k1 −−−→ (z, ai )], ni =[k2 −−−→ (w, ai ) ∧ k2 −−−→ (z, ai )], ˘ pi =[k3 −−−→ (w, ai ) ∨ k3 −−−→ (z, ai )], fori = 1,2,..., l
Suppose, H1(N+ (w) ∩ N+ (z))= max{mi, i = 1,2,..., l} = mmax, H2(N+ (w) ∩ N+ (z))= max{ni, i = 1,2,..., l} = nmax, H3(N+ (w) ∩ N+ (z))= min{ ˘ pi, i = 1,2,..., l} = ˘ pmin
Obviously, mmax > k1 −−−→ (w, z) and nmax < k2 −−−→ (w, z) and ˘ pmin < k3 −−−→ (w, z) foralledges −−−→ (w, z) showthat:
mmax h1(w) ∧ h1(z) > k1 −−−→ (w, z) h1(w) ∧ h1(z) > 0.5, nmax h2(w) ∧ h2(z) < k2 −−−→ (w, z) h2(w) ∧ h2(z) < 0.5, pmin h3(w) ∨ h3(z) < k3 −−−→ (w, z) h3(w) ∧ h3(z) < 0.5, therefore,
k1(w, z)=(h1(w) ∧ h1(z))H1(N+ (w) ∩ N+ (z)), k1(w, z)=[h1(w) ∧ h1(z)] × mmax, k1(w, z) (h1(w) ∧ h1(z)) = mmax, k1(w, z) (h1(w) ∧ h1(z))2 = mmax (h1(w) ∧ h1(z)) > 0.5,
k2(w, z)=(h2(w) ∧ h2(z))H2(N+ (w) ∩ N+ (z)), k2(w, z)=[h2(w) ∧ h2(z)] × nmax, k2(w, z) (h2(w) ∧ h2(z)) = nmax, k2(w, z) (h2(w) ∧ h2(z))2 = nmax (h2(w) ∧ h2(z)) < 0.5, and: k3(w, z)=(h3(w) ∨ h3(z))H3(N+ (w) ∩ N+ (z)), k3(w, z)=[h3(w) ∨ h3(z)] × ˘ pmin, k3(w, z) (h3(w) ∨ h3(z)) = ˘ pmin, k3(w, z) (h3(w) ∨ h3(z))2 = ˘ pmin (h3(w) ∨ h3(z)) < 0.5. Hence, k1(w,z) (h1(w)∧h1(z))2 > 0.5, k2(w,z) (h2(w)∧h2(z))2 < 0.5,and k3(w,z) (h3(w)∨h3(z))2 < 0.5 foralledges (w, z) in C(−→ G ) Theorem3. Let C(−→
2. th1 h2 = th1 (w1) ∧ th2 (w2),ih1 h2 = ih1 (w1) ∧ ih2 (w2),fh1 h2 = fh1 (w1) ∨ fh2 (w2). 3. tk ((w1, w2)(w1, z2))=[th1 (w1) ∧ th2 (w2) ∧ th2 (z2)] ×∨x2 {th1 (w1) ∧ t−→ l2 (w2 x2) ∧ t−→ l2 (z2 x2)}, (w1, w2)(w1, z2) ∈ EC(−→ G1)∗ EC(−→ G2)∗ , x2 ∈ (N+ (w2) ∩ N+ (z2))∗
4. ik ((w1, w2)(w1, z2))=[ih1 (w1) ∧ ih2 (w2) ∧ ih2 (z2)] ×∨x2 {ih1 (w1) ∧ i−→ l2 (w2 x2) ∧ i−→ l2 (z2 x2)}, (w1, w2)(w1, z2) ∈ EC(−→ G1)∗ EC(−→ G2)∗ , x2 ∈ (N+ (w2) ∩ N+ (z2))∗
5. fk ((w1, w2)(w1, z2))=[ fh1 (w1) ∨ fh2 (w2) ∨ fh2 (z2)] ×∨x2 { fh1 (w1) ∨ f−→ l2 (w2 x2) ∨ f−→ l2 (z2 x2)}, (w1, w2)(w1, z2) ∈ EC(−→ G1)∗ EC(−→ G2)∗ , x2 ∈ (N+ (w2) ∩ N+ (z2))∗ .
6. tk ((w1, w2)(z1, w2))=[th1 (w1) ∧ th1 (z1) ∧ th2 (w2)] ×∨x1 {th2 (w2) ∧ t−→ l1 (w1 x1) ∧ t−→ l1 (z1 x1)}, (w1, w2)(z1, w2) ∈ EC(−→ G1)∗ EC(−→ G2)∗ , x1 ∈ (N+ (w1) ∩ N+ (z1))∗ .
7. ik ((w1, w2)(z1, w2))=[ih1 (w1) ∧ ih1 (z1) ∧ ih2 (w2)] ×∨x1 {ih2 (w2) ∧ i−→ l1 (w1 x1) ∧ i−→ l1 (z1 x1)}, (w1, w2)(z1, w2) ∈ EC(−→ G1)∗ EC(−→ G2)∗ , x1 ∈ (N+ (w1) ∩ N+ (z1))∗ .
8. fk ((w1, w2)(z1, w2))=[ fh1 (w1) ∨ fh1 (z1) ∨ fh2 (w2)] ×∨x1 { fh2 (w2) ∨ f−→ l1 (w1 x1) ∨ t−→ l1 (z1 x1)}, (w1, w2)(z1, w2) ∈ EC(−→ G1)∗ EC(−→ G2)∗ , x1 ∈ (N+ (w1) ∩ N+ (z1))∗ .
9. tk ((w1, w2)(z1, z2))=[th1 (w1) ∧ th1 (z1) ∧ th2 (w2) ∧ th2 (z2)] × [th1 (w1) ∧ t−→ l1 (z1w1) ∧ th2 (z2) ∧ t−→ l2 (w2z2)], (w1, z1)(w2, z2) ∈ E
10. ik ((w1, w2)(z1, z2))=[ih1 (w1) ∧ ih1 (z1) ∧ ih2 (w2) ∧ ih2 (z2)] × [ih1 (w1) ∧ i−→ l1 (z1w1) ∧ ih2 (z2) ∧ i−→ l2 (w2z2)], (w1, z1)(w2, z2) ∈ E 11. fk ((w1, w2)(z1, z2))=[ fh1 (w1) ∨ fh1 (z1) ∨ fh2 (w2) ∨ fh2 (z2)] × [ fh1 (w1) ∨ f−→ l1 (z1w1) ∨ fh2 (z2) ∨ f−→ l2 (w2z2)], (w1, z1)(w2, z2) ∈ E .
Proof. UsingsimilarargumentsasinTheorem2.1.[39],itcanbeproven. Example4. Consider −→ G1 =(X1, h1, l1) and −→ G2 =(X2, h2, l2) tobetwoIN-digraphs,respectively,asshown inFigure 6.Theintuitionisticneutrosophicoutandin-neighborhoodsof −→ G1 and −→ G2 aregiveninTables 3 and 4 TheINC-graphs C(−→ G1) and C(−→ G2) aregiveninFigure 7
Table3. IN-outandin-neighborhoodsof −→ G1 w ∈ X1 N+ (w) N (w) w1 {w2(0.2,0.2,0.3)} ∅ w2 ∅ {w1(0.2,0.2,0.3), w3(0.3,0.1,0.1)} w3 {w2(0.3,0.2,0.1)}{w4(0.3,0.1,0.1)} w4 {w3(0.3,0.1,0.1)} ∅
Table4. IN-outandin-neighborhoodsof −→ G2 w ∈ X2 N+ (w) N (w) z1 {z3(0.3,0.2,0.2)} ∅ z2 {z3(0.3,0.1,0.1)} ∅ z3 ∅ {z1(0.3,0.2,0.2), z2(0.3,0.1,0.1)}
z1 (0 4, 0 3, 0 2)
w1 (0 3, 0 4, 0 5)
w2 (0 4, 0 3, 0 1) w3 (0 5, 0 2, 0 1) w4 (0 4, 0 3, 0 1)
z2 (0 4, 0 3, 0 5)
(0 .3 ,0 .2 ,0 . 2) (0 . 3 , 0 . 1 , 0 1)
z3 (0 7, 0 2, 0 3)
→G1 →G2 (0 .2 ,0 .2 ,0 . 3) (0 . 3, 0. 2, 0. 1) (0 .2 ,0 .2 ,0 . 3)
Figure6. IN-digraphs.
w1 (0.3, 0.4, 0.5) w2 (0 4, 0 3, 0 1) w3 (0 5, 0 2, 0 1) w4 (0 4, 0 3, 0 1)
z1 (0 4, 0 3, 0 2)
C ( →G2 )
C ( →G1 ) (0 . 12 , 0 03 , 0 . 1) (0 . 06, 0. 04, 0. 15)
z2 (0 4, 0 3, 0 5)
z3 (0 7, 0 2, 0 3)
Figure7. INC-graphsof −→ G1 and −→ G2
WenowconstructtheINC-graph GC(−→ G1)∗ C(−→ G2)∗ ∪ G =(w, k),where w =(tw, iw, fw) and k =(tk,ik,fk), from C(−→ G1)∗ and C(−→ G2)∗ usingTheorem 2.14.WeobtainedtwosetsofedgesbyusingCondition (1)
EC(−→ G1)∗ EC(−→ G2)∗ ={(w1, z1)(w1, z2), (w2, z1)(w2, z2), (w3, z1)(w3, z2), (w4, z1)(w4, z2), (w1, z1)(w3, z1), (w1, z2)(w3, z2), (w1, z3)(w3, z3)}, E ={(w2, z1)(w1, z3), (w2, z1)(w3, z3), (w2, z2)(w1, z3) (w2, z2)(w3, z3), (w3, z1)(w4, z3), (w3, z2)(w4, z3)}.
Thetruth-membership,indeterminacy-membershipandfalsity-membershipofedgescanbecalculatedbyusing Conditions (3) to (11) as, k((w1, z1)(w1, z2))=(th1 (w1) ∧ th2 (z1) ∧ th2 (z2), ih1 (w1) ∧ ih2 (z1) ∧ ih2 (z2), fh1 (w1) ∨ fh2 (z1) ∨ fh2 (z2)) ×(th1 (w1) ∧ tl2 (z1z3) ∧ tl2 (z2z3), ih1 (w1) ∧ il2 (z1z3) ∧ il2 (z2z3), fh1 (w1) ∨ fl2 (z1z3) ∨ fl2 (z2z3) =(0.3,0.3,0.5) × (0.3,0.1,0.5) =(0.09,0.03,0.25), k((w2, z1)(w1, z3))=(th1 (w2) ∧ th2 (z1) ∧ th1 (w1) ∧ th2 (z3), ih1 (w2) ∧ ih2 (z1) ∧ ih1 (w1) ∧ ih2 (z3), fh1 (w2) ∨ fh2 (z1) ∨ fh1 (w1) ∨ fh2 (z3)) ×(th1 (w2) ∧ tl1 (w1w2) ∧ tl2 (z3) ∧ tl2 (z1z3), ih1 (w2) ∧ il1 (w1w2) ∧ il2 (z3) ∧ il2 (z1z3), fh1 (w2) ∨ fl1 (w1w2) ∨ fl2 (z3) ∨ fl2 (z1z3)) =(0.3,0.2,0.5) × (0.2,0.2,0.3) =(0.06,0.04,0.15)
Allthetruth-membership,indeterminacy-membershipandfalsity-membershipdegreesofadjacentedgesof GC(−→ G1)∗ C(−→ G2)∗ and G aregiveninTable 5.
Table5. Adjacentedgesof GC(−→ G1 )∗ C(−→ G2 )∗ ∪ G .
(w, ´ w)(z, ´ z) k (w, ´ w)(z, ´ z)
(w1, z1)(w1, z2)(0.09,0.03,0.25) (w2, z1)(w2, z2)(0.12,0.03,0.1) (w3, z1)(w3, z2)(0.12,0.02,0.1) (w4, z1)(w4, z2)(0.12,0.03,0.1) (w1, z1)(w3, z1)(0.06,0.04,0.15)
(w1, z3)(w3, z3)(0.06,0.04,0.15)
(w2, z1)(w1, z3)(0.06,0.04,0.15)
(w2, z1)(w3, z3)(0.12,0.04,0.09)
(w2, z2)(w1, z3)(0.06,0.02,0.15)
(w2, z2)(w3, z3)(0.12,0.02,0.15)
(w3, z1)(w4, z3)(0.12,0.02,0.09)
(w3, z2)(w4, z3)(0.12,0.02,0.15) (w1, z2)(w3, z2)(0.06,0.04,0.25)
TheINC-graphobtainedbyusingthismethodisgiveninFigure 8 wheresolidlinesindicatepartofINC-graph obtainedfrom GC(−→ G1)∗ C(−→ G2)∗ ,andthedottedlinesindicatethepartof G . TheCartesianproduct −→ G1 −→ G2 ofIN-digraphs −→ G1 and −→ G2 isshowninFigure 9.TheIN-out-neighborhoods of −→ G1 −→ G2 arecalculatedinTable 6.TheINC-graphsof −→ G1 −→ G2 areshowninFigure 10
w1 w2 w3 w4
w1 w2 w3 w4
(0 09, 0 03, 0 25) (0 06, 0 04, 0 15) (0 06, 0 04, 0 25)
(0 12, 0 02, 0 1) (0 12, 0 03, 0 1)
(0. 06,0. 02,0. 15) (0. 06, 0. 02, 0. 15) (0 12, 0 03, 0 1) (0 .12, 0.04 , 0.09) (0 .12 , 0 .02 , 0 .15) (0 .12, 0.02, 0.09)
z1 z2 z3 (0 3, 0 3, 0 5) (0 4, 0 3, 0 2) (0.4, 0.2, 0.2) (0 4, 0 3, 0 2)
(0 3, 0 3, 0 5) (0 4, 0 3, 0 5) (0 4, 0 2, 0 5) (0 4, 0 3, 0 5)
(0 .12 , 0 .02 , 0 .15)
(0.3, 0.2, 0.5) (0 4, 0 2, 0 3) (0 5, 0 2, 0 3) (0 4, 0 2, 0 3)
(0 . 06 , 0 . 04 , 0 . 15)
z1 z2 z3
(0 2, 0 2, 0 3) (0 3, 0 2, 0 2) (0.3, 0.1, 0.2)
(0.3,0.2,0.5) (0.3,0.2,0.2) (0 3,0.2,0.2) (0.3,0.2,0.2)
Figure8. GC(−→ G1 )∗ C(−→ G2 )∗ ∪ G (0 3, 0 3, 0 5) (0 4, 0 3, 0 2) (0 4, 0 2, 0 2) (0 4, 0 3, 0 2)
(0 2, 0 2, 0 5)
(0 4, 0 3, 0 5)
(0.3, 0.2, 0.5)
(0.4, 0.2, 0.5)
(0 3, 0 1, 0 5)
(0.3, 0.3, 0.5) (0 3, 0 1, 0 5) (0.3, 0.1, 0.1) (0 3, 0 1, 0 1) (0 3, 0 1, 0 1)
Figure9. −→ G1 −→ G2
(0 2, 0 2, 0 3) (0 3, 0 2, 0 3) (0 3, 0 1, 0 3)
(0 3, 0 2, 0 5) (0 4, 0 2, 0 3) (0 5, 0 2, 0 3) (0 4, 0 2, 0 3) (0.4, 0.3, 0.5)
Table6. IN-out-neighborhoodsof −→ G1 −→ G2
(w, z) N+ (w, z)
(w1, z1) {((w2, z1),0.2,0.2,0.3), ((w1, z3),0.3,0.2,0.5)} (w1, z2) {((w1, z3),0.3,0.1,0.5), ((w2, z2),0.2,0.2,0.5)}
(w1, z3) {((w2, z3),0.2,0.2,0.3)}
(w2, z1) {((w2, z3),0.3,0.2,0.2)}
(w2, z2) {((w2, z3),0.3,0.1,0.1)}
(w2, z3) ∅
(w3, z1) {((w3, z3),0.3,0.2,0.2), ((w2, z1),0.3,0.2,0.2)} (w3, z2) {((w2, z2),0.3,0.2,0.5), ((w3, z3),0.3,0.1,0.1)}
(w3, z3) {((w2, z3),0.3,0.2,0.3)}
(w4, z1) {((w4, z3),0.3,0.2,0.2), ((w3, z1),0.3,0.1,0.2)} (w4, z2) {((w4, z3),0.3,0.1,0.1), ((w3, z2),0.3,0.1,0.5)} (w4, z2) {((w3, z3),0.3,0.1,0.3)} w1 w2 w3 w4
(0 09, 0 03, 0 25) (0 06, 0 04, 0 15) (0 06, 0 04, 0 25)
(0. 06,0. 02,0. 15) (0. 06, 0. 02, 0. 15) (0 12, 0 03, 0 1) (0 .12, 0.04 , 0.09) (0 .12 , 0 .02 , 0 .15) (0 .12, 0.02, 0.09)
z1 z2 z3 (0 3, 0 3, 0 5) (0 4, 0 3, 0 2) (0 4, 0 2, 0 2) (0 4, 0 3, 0 2)
(0 3, 0 3, 0 5) (0 4, 0 3, 0 5) (0 4, 0 2, 0 5) (0 4, 0 3, 0 5)
(0 12, 0 02, 0 1) (0 12, 0 03, 0 1)
(0 .12 , 0 .02 , 0 .15)
(0 3, 0 2, 0 5) (0 4, 0 2, 0 3) (0 5, 0 2, 0 3) (0 4, 0 2, 0 3)
(0 06 , 0 04 , 0 15) Figure10. C(−→ G1 −→ G2). Itcanbeseenthat C(−→ G1 −→ G2) ∼ = GC(−→ G1)∗ C(
G fromFigures 8 and 10 Definition10. Theintuitionisticneutrosophicopen-neighborhoodofavertex w ofanIN-graph G =(X, h, k) is IN-set N(w)=(Xw,tw,iw,fw ),where, Xw
{z|k1(w, z) > 0,k2(w, z) > 0,k3(w, z) > 0}, and tw : Xw → [0,1] definedby tw (z)= k1(w, z), iw : Xw → [0,1] definedby iw (z)= k2(w, z) and fz : Xw → [0,1] definedby fw (z)= k3(w, z).Foreveryvertex w ∈ X,theintuitionisticneutrosophic singletonset, Aw =(w, h1, h2, h3),suchthat: h1 : {w}→ [0,1], h2 : {w}→ [0,1], h3 : {w}→ [0,1] definedby h1(w)= h1(w), h2(w)= h2(w) and h3(w)= h3(w),respectively.Theintuitionisticneutrosophic closed-neighborhoodofavertexwis N[w]= N(w) ∪ Aw
Definition11. Suppose G =(X, h, k) isanIN-graph.Thesingle-valuedintuitionisticneutrosophic open-neighborhoodgraphof G isanIN-graph N(G)=(X,h,k ),whichhasthesameintuitionisticneutrosophic setofverticesin G andhasanintuitionisticneutrosophicedgebetweentwovertices w, z ∈ X in N(G) if andonlyif N(w) ∩ N(z) isanon-emptyIN-setin G.Thetruth-membership,indeterminacy-membershipand falsity-membershipvaluesoftheedge (w,z) aregivenby:
k1(w, z)=[h1(w) ∧ h1(z)]H1(N(w) ∩ N(z)), k2(w, z)=[h2(w) ∧ h2(z)]H2(N(w) ∩ N(z)), k3(w, z)=[h3(w) ∨ h3(z)]H3(N(w) ∩ N(z)), respectively
Definition12. Suppose G =(X, h, k) isanIN-graph.Thesingle-valuedintuitionisticneutrosophic closed-neighborhoodgraphof G isanIN-graph N(G)=(X, h, k ),whichhasthesameintuitionisticneutrosophic setofverticesin G andhasanintuitionisticneutrosophicedgebetweentwovertices w, z ∈ X in N[G] if andonlyif N[w] ∩ N[z] isanon-emptyIN-setin G.Thetruth-membership,indeterminacy-membershipand falsity-membershipvaluesoftheedge (w,z) aregivenby: k1(w, z)=[h1(w) ∧ h1(z)]H1(N[w] ∩ N[z]), k2(w, z)=[h2(w) ∧ h2(z)]H2(N[w] ∩ N[z]), k3(w, z)=[h3(w) ∨ h3(z)]H3(N[w] ∩ N[z]), respectively
Example5. Consider G =(X, h, k) tobeanIN-graph,suchthat X = {a, b, c, d}, h = {(a, 0.5, 0.4, 0.3), (b, 0.6, 0.3, 0.1), (c, 0.7, 0.3, 0.1), (d, 0.5, 0.6, 0.3)},and k = {(ab, 0.3, 0.2, 0.2), (ad, 0.4, 0.3, 0.2), (bc, 0.5, 0.2, 0.1), (cd, 0.4, 0.2, 0.2)},asshowninFigure 11.Then,correspondingintuitionisticneutrosophicopenand closed-neighborhoodgraphsareshowninFigure 12. a(0 5, 0 4, 0 3) b(0 6, 0 3, 0 1) c(0 7, 0 3, 0 1) d(0 5, 0 6, 0 3)
(0 . 4 , 0 . 3 , 0 . 2)
(0 3, 0 2, 0 2) (0 . 5 , 0 . 2 , 0 . 1) (0 4, 0 2, 0 2)
Figure11. IN-digraph.
2017, 8,132
a(0 5, 0 4, 0 3)
b(0 6, 0 3, 0 1)
d(0 5, 0 6, 0 3)
(0 2, 0 06, 0 06) (0 2, 0 06, 0 06) (0 15, 0 06, 0 06)
c(0 7, 0 3, 0 1)
a(0 5, 0 4, 0 3)
(a) (b)
b(0 6, 0 3, 0 1)
(0 .2 , 0 .06 , 0 .06)
c(0 7, 0 3, 0 1) d(0 5, 0 6, 0 3)
(0 2 , 0 12 , 0 09) (0 2, 0 06, 0 06)
(0 . 3 , 0 06 , 0 01) (0 2, 0 06, 0 06)
Figure12. (a) N(G); (b) N[G]
Theorem4. ForeachedgeofanIN-graph G,thereexistsanedgein N[G] Proof. Suppose (w, z) isanedgeofanIN-graph G =(V, h, k).Suppose N[G]=(V, h, k ) isthe correspondingclosedneighborhoodofanIN-graph.Suppose w, z ∈ N[w] and w, z ∈ N[z].Then, w, z ∈ N[w] ∩ N[z].Hence, H1(N[w] ∩ N[z]) = 0, H2(N[w] ∩ N[z]) = 0, H3(N[w] ∩ N[z]) = 0. Then, k1(w, z)=[h1(w) ∧ h1(z)]H1(N[w] ∩ N[z]) = 0, k2(w, z)=[h2(w) ∧ h2(z)]H2(N[w] ∩ N[z]) = 0, k3(w, z)=[h3(w) ∨ h3(z)]H3(N[w] ∩ N[z]) = 0.
Thus,foreachedge (w, z) inIN-graph G,thereexistsanedge (w, z) in N[G]. Definition13. ThesupportofanIN-set ˘ A =(w,tA,iA,f A ) inXisthesubset ˆ AofXdefinedby: ˆ A = {w ∈ X:t ˘ A (w) = 0,i ˘ A (w) = 0,f ˘ A (w) = 1} and |supp( ˆ A)| isthenumberofelementsintheset.
Wenowdiscuss p-competitionintuitionisticneutrosophicgraphs. Suppose p isapositiveinteger.Then, p-competitionIN-graph Cp (−→ G ) oftheIN-digraph −→ G =(X, h, k) isanundirectedIN-graph G =(X, h, k),whichhasthesameintuitionisticneutrosophic setofverticesasin −→ G andhasanintuitionisticneutrosophicedgebetweentwovertices w, z ∈ X in
Cp (−→ G ) ifandonlyif |supp(N+ (w) ∩ N+ (z))|≥ p.Thetruth-membershipvalueofedge (w, z) in Cp (−→ G ) is t(w, z)= (i p)+1 i [h1(w) ∧ h1(z)]H1(N+ (w) ∩ N+ (z));theindeterminacy-membershipvalueofedge (w, z) in Cp (−→ G ) is i(w, z)= (i p)+1 i [h2(w) ∧ h2(z)]H2(N+ (w) ∩ N+ (z));andthefalsity-membership valueofedge (w, z) in Cp (−→ G ) is f (w, z)= (i p)+1 i [h3(w) ∨ h3(z)]H3(N+ (w) ∩ N+ (z)) where i = |supp(N+ (w) ∩ N+ (z))|
Thethree-competitionIN-graphisillustratedbythefollowingexample. Example6. Consider −→ G =(X, h, k) tobeanIN-digraph,suchthat X = {w1, w2, w3, z1, z2, z3}, h = {(w1, 0.5, 0.1, 0.2), (w2, 0.1, 0.6, 0.3), (w3, 0.1, 0.2, 0.5), (z1, 0.7, 0.2, 0.1), (z2, 0.5, 0.2, 0.3), (z3, 0.3, 0.7, 0.2)} and k = {( −−−−→ (w1, z1),0.4, 0.1, 0.1), ( −−−−→ (w1, z2),0.5, 0.1, 0.3), ( −−−−→ (w1, z3),0.2, 0.1, 0.1), ( −−−−→ (w2, z1),0.1, 0.1, 0.2), ( −−−−→ (w2, z2),0.1, 0.1, 0.2), ( −−−−→ (w2, z3),0.1, 0.5, 0.2), ( −−−−→ (w3, z1),0.1, 0.1, 0.1)( −−−−→ (w3, z2),0.1, 0.1, 0.2)},asshownin Figure 13.Then, N+ (w1)= {(z1, 0.4, 0.1, 0.1), (z2, 0.5, 0.1, 0.3), (z3, 0.2, 0.1, 0.1)}, N+ (w2)= {(z1, 0.1, 0.1, 0.2), (z2, 0.1, 0.1, 0.2), (z3, 0.1, 0.5, 0.2)} and N+ (w3)= {(z1, 0.1, 0.1, 0.1), (z2, 0.1, 0.1, 0.2)} Therefore, N+ (w1) ∩ N+ (w2)= {(z1, 0.1, 0.1, 0.2), (z2, 0.1, 0.1, 0.3), (z3, 0.1, 0.1, 0.2)}, N+ (w1) ∩ N+ (w3)= {(z1, 0.1, 0.1, 0.1), (z2, 0.1, 0.1, 0.3)} and N+ (w2) ∩ N+ (w3)= {(z1, 0.1, 0.1, 0.2), (z2, 0.1, 0.1, 0.2)}
Now, i = |supp(N+ (w1) ∩ N+ (w2))| = 3.For p = 3, t(w1, w2)= 0.003, i(w1, w2)= 0.003 and f (w1,w2)= 0.02.AsshowninFigure 14
w1 (0 5, 0 1, 0 2)
z1 (0 7, 0 2, 0 1)
w2 (0 1, 0 6, 0 3) w3 (0 1, 0 2, 0 5)
Figure13. IN-digraph.
z2 (0 5, 0 2, 0 3)
z3 (0 3, 0 7, 0 2)
w1 (0 5, 0 1, 0 2)
z1 (0 7, 0 2, 0 1)
(0 003 , 0 . 003 , 0 02)
w2 (0 1, 0 6, 0 3)
z2 (0 5, 0 2, 0 3)
w3 (0 1, 0 2, 0 5)
z3 (0 3, 0 7, 0 2)
Figure14. Three-competitionIN-graph.
2017, 8,132
WenowdefineanotherextensionofINC-graphknownasthe m-stepINC-graph. −→ P m z,w:adirectedintuitionisticneutrosophicpathoflength m from z to w. N+ m (z):single-valuedintuitionisticneutrosophic m-stepout-neighborhoodofvertex z. Nm (z):single-valuedintuitionisticneutrosophic m-stepin-neighborhoodofvertex z. Cm −→ (G): m-stepINC-graphoftheIN-digraph −→ G
Definition14. Suppose −→ G =(X, h, k) isanIN-digraph.The m-stepIN-digraphof −→ G isdenotedby −→ G m =(X,h,k) wheretheintuitionisticneutrosophicsetofverticesof −→ G isthesameastheintuitionisticneutrosophicsetofverticesof −→ G m andhasanedgebetween z and w in −→ G m ifandonlyifthereexistsanintuitionisticneutrosophicdirectedpath −→ P m z,w in −→ G
Definition15. Theintuitionisticneutrosophic m-stepout-neighborhoodofvertex z ofanIN-digraph −→ G =(X,h,k) isIN-set: N+ m (z)=(X+ z ,t+ z ,i+ z ,f + z ),where X+ z = {w| thereexistsadirectedintuitionisticneutrosophicpathoflength m from z to w, −→ P m z,w }, t+ z : X+ z → [0, 1], i+ z : X+ z → [0, 1] and f + z : X+ z → [0, 1] aredefinedby t+ z = min{t −−−−−→ (w1,w2), (w1, w2) isanedgeof −→ P m z,w }, i+ z = min{i −−−−−→ (w1,w2), (w1, w2) isanedgeof −→ P m z,w } and f + z = max{ f −−−−−→ (w1,w2), (w1, w2) isanedgeof −→ P m z,w },respectively.
Definition16. Theintuitionisticneutrosophic m-stepin-neighborhoodofvertex z ofanIN-digraph −→ G =(X,h,k) isIN-set: Nm (z)=(Xz ,tz ,iz ,fz ),where Xz = {w| thereexistsadirectedintuitionisticneutrosophicpathoflength m from w to z, −→ P m w,z }, tz : Xz → [0, 1], iz : Xz → [0, 1] and fz : Xz → [0, 1] aredefinedby tz = min{t −−−−−→ (w1,w2), (w1, w2) isanedgeof −→ P m w,z }, iz = min{i −−−−−→ (w1,w2), (w1, w2) isanedgeof −→ P m w,z } and fz = max{ f −−−−−→ (w1,w2), (w1, w2) isanedgeof −→ P m w,z },respectively.
Definition17. Suppose −→ G =(X, h, k) isanIN-digraph.The m-stepINC-graphofIN-digraph −→ G isdenoted by Cm (−→ G )=(X, h, k),whichhasthesameintuitionisticneutrosophicsetofverticesasin −→ G andhasan edgebetweentwovertices w, z ∈ X in Cm (−→ G ) ifandonlyif (N+ m (w) ∩ N+ m (z)) isanon-emptyIN-setin −→ G .Thetruth-membershipvalueofedge (w, z) in Cm (−→ G ) is t(w, z)=[h1(w) ∧ h1(z)]H1(N+ m (w) ∩ N+ m (z)); theindeterminacy-membershipvalueofedge (w, z) in Cm (−→ G ) is i(w, z)=[h2(w) ∧ h2(z)]H2(N+ m (w) ∩ N+ m (z)); andthefalsity-membershipvalueofedge (w,z) in Cm (−→ G ) isf (w,z)=[h3(w) ∨ h3(z)]H3(N+ m (w) ∩ N+ m (z)).
Thetwo-stepINC-graphisillustratedbythefollowingexample. Example7. Consider −→ G =(X, h, k) isanIN-digraph,suchthat, X = {w1, w2, z1, z2, z3}, h = {(w1, 0.3, 0.4, 0.6), (w2, 0.2, 0.5, 0.3), (z1, 0.4, 0.2, 0.3), (z2, 0.7, 0.2, 0.1), (z3, 0.5, 0.1, 0.2), (z4, 0.6, 0.3, 0.2)}, and k = {( −−−−→ (w1,z1), 0.2, 0.1, 0.2), ( −−−−→ (w2,z4), 0.1, 0.2, 0.3), ( −−−−→ (z1,z3), 0.3, 0.1, 0.2), ( −−−−→ (z1,z2), 0.3, 0.1, 0.2), ( −−−−→ (z4,z2), 0.2, 0.1, 0.1),and ( −−−−→ (z4,z3), 0.4, 0.1, 0.4)},asshowninFigure 15 Then, N+ 2 (w1)= {(z2, 0.2, 0.1, 0.2), (z3, 0.2, 0.1, 0.2)} and N+ 2 (w2)= {(z2, 0.1, 0.1, 0.3), (z3, 0.1, 0.1, 0.4)}.Therefore, N+ 2 (w1) ∩ N+ 2 (w2)= {(z2, 0.1, 0.1, 0.3), (z3, 0.1, 0.1, 0.4)}.Thus, t(w1, w2)= 0.02, i(w1, w2)= 0.04 andf (w1, w2)= 0.18.ThisisshowninFigure 16.
2017, 8,132
w1 (0 3, 0 4, 0 6)
w2 (0 2, 0 5, 0 3)
z1 (0 4, 0 2, 0 3)
w1 (0 3, 0 4, 0 6)
z1 (0 4, 0 2, 0 3)
z4 (0 6, 0 3, 0 2) (0 . 2 , 0 1 , 0 2) (0 . 1 , 0 . 2 , 0 . 3) (0. 2, 0. 1, 0. 1) (0 .3 , 0 .1 , 0 .2) (0 . 4, 0. 1, 0. 4) (0 .3 ,0 .1 ,0 . 2)
z2 (0 7, 0 2, 0 1)
z3 (0 5, 0 1, 0 2)
Figure15. IN-digraph.
(0 02, 0 04, 0 18)
w2 (0 2, 0 5, 0 3)
z2 (0 7, 0 2, 0 1)
z4 (0 6, 0 3, 0 2)
z3 (0 5, 0 1, 0 2)
Figure16. Two-stepINC-graph.
Definition18. Theintuitionisticneutrosophic m-stepout-neighborhoodofvertex z ofanIN-digraph −→ G =(X,h,k) isIN-set:
Nm (z)=(Xz,tz,iz,fz ),where
Xz = {w| thereexistsadirectedintuitionisticneutrosophicpathoflength m from z to w, Pm z,w }, tz : Xz → [0, 1], iz : Xz → [0, 1] and fz : Xz → [0, 1] aredefinedby tz = min{t(w1, w2), (w1, w2) isanedgeof Pm z,w }, iz = min{i(w1,w2), (w1, w2) isanedgeof Pm z,w } and fz = max{ f (w1, w2), (w1, w2) isanedgeof Pm z,w },respectively.
Definition19. Suppose G =(X, h, k) isanIN-graph.Then,the m-stepintuitionisticneutrosophic neighborhoodgraph(IN-neighborhood-graph) Nm (G) isdefinedby Nm (G)=(X, h, κ),where h =(h1, h2, h3), κ =(κ1, κ2, κ3), κ1 : X × X → [0, 1], κ2 : X × X → [0, 1] and κ3 : X × X → [0,1] aresuchthat:
κ1(w, z)= h1(w) ∧ h1(z)H1(Nm (w) ∩ Nm (z)),
κ2(w, z)= h2(w) ∧ h2(z)H2(Nm (w) ∩ Nm (z)), κ3(w, z)= h3(w) ∨ h3(z)H3(Nm (w) ∩ Nm (z)), respectively
Theorem5. IfalltheedgesofIN-digraph −→ G =(X, h, k) areindependentstrong,thenalltheedgesof Cm (−→ G ) areindependentstrong.
Proof. Suppose −→ G =(X, h, k) isanIN-digraphand Cm (−→ G )=(X, h, k) isthecorresponding m-stepINC-graph.Sincealltheedgesof −→ G areindependentstrong,then H1(N+ m (w) ∩ N+ m (z)) > 0.5, H2(N+ m (w) ∩ N+ m (z)) < 0.5 and H3(N+ m (w) ∩ N+ m (z)) < 0.5.Then, t(w, z)=(h1(w) ∧ h1(z))H1(N+ m (w) ∩ N+ m (z)),or t(w, z) > 0.5(h1(w) ∧ h1(z)),or t(w,z) (h1(w)∧h1(z)) > 0.5, i(w, z)=(h2(w) ∧ h2(z))H2(N+ m (w) ∩ N+ m (z)),or i(w, z) < 0.5(h2(w) ∧ h2(z)),or i(w,z) (h2(w)∧h2(z)) < 0.5 and f (w, z)=(h3(w) ∨ h3(z))H3(N+ m (w) ∩ N+ m (z)),or f (w, z) < 0.5(h3(w) ∨ h3(z)),or f (w,z) (h3(w)∨h3(z)) < 0.5.
Hence,theedge (w, z) isindependentstrongin Cm (−→ G ).Since, (w, z) istakentobethearbitrary edgeof Cm (−→ G ),thusalltheedgesof Cm (−→ G ) areindependentstrong.
3.Applications
Competitiongraphsareveryimportanttorepresentthecompetitionbetweenobjects.However, still,theserepresentationsareunsuccessfultodealwithallthecompetitionsofworld;forthatpurpose, INC-graphsareintroduced.Now,wediscusstheapplicationsofINC-graphstostudythecompetition alongwithalgorithms.TheINC-graphshavemanyutilizationsindifferentareas.
3.1.Ecosystem
Considerasmallecosystem:humaneatstrout;baldeagleeatstroutandsalamander;trouteats phytoplankton,mayflyanddragonfly;salamandereatsdragonflyandmayfly;snakeeatssalamanderandfrog; frogeatsdragonflyandmayfly;mayflyeatsphytoplankton;dragonflyeatsphytoplankton.Theseninespecies human,baldeagle,salamander,snake,frog,dragonfly,trout,mayflyandphytoplanktonaretakenasvertices. Letthedegreeofexistenceintheecosystemofhumanbe 60%,thedegreeofindeterminacyofexistencebe 30% andthedegreeoffalse-existencebe 10%,i.e.,thetruth-membership,indeterminacy-membershipand falsity-membershipvaluesofthevertexhumanare (0.6, 0.3, 0.1).Similarly,weassumethetruth-membership, indeterminacy-membershipandfalsity-membershipvaluesofotherverticesas (0.7, 0.3, 0.2), (0.4, 0.3, 0.5), (0.3, 0.5, 0.1), (0.3, 0.4, 0.5), (0.3, 0.5, 0.2), (0.7, 0.3, 0.2), (0.6, 0.4, 0.2) and (0.3, 0.5, 0.2).Supposethathuman likestoeattrout 20%,indeterminatetoeat 10% anddisliketoeat,say 10%.Thelikeness,indeterminacyand dislikenessofpreysforpredatorsareshowninTable 7
Itisclearthatiftroutisremovedfromthefoodcycle,thenhumanmustbelifeless,andinsuchasituation baldeagle,phytoplankton,dragonflyandmayflygrowinanundisciplinedmanner.Thus,wecanevaluate thefoodcyclewiththehelpofINC-graphs.
Table7. Likeness,indeterminacyanddislikenessofpreysandpredators.
NameofPredatorNameofPreyLiketoEatIndeterminatetoEatDisliketoEat
HumanTrout 201010
BaldeagleTrout 202020
BaldeagleSalamander 302030 SnakeSalamander 202010 SnakeFrog 302040 SalamanderDragonfly 202020 SalamanderMayfly 202040
FrogDragonfly 303030
TroutDragonfly 204010
TroutMayfly 301010
TroutPhytoplankton 201010 DragonflyPhytoplankton 101010 MayflyPhytoplankton 303020 FrogMayfly 101010
ForthisfoodwebFigure 17,wehavethefollowingTable 8 ofIN-out-neighborhoods.
Human
(0 6, 0 3, 0 1) (0 7, 0 3, 0 2) (0 4, 0 3, 0 5)
(0 2 , 0 1 , 0 1) (0 2, 0 2, 0 2)
Trout
Baldeagle Salamander
(0 3, 0 5, 0 2) (0 6, 0 4, 0 2) (0 3, 0 5, 0 2)
Phytoplankton
Dragonfly
Snake Frog Mayfly
(0 . 1, 0. 1, 0. 1) (0 2 , 0 .1 , 0 . 1) (0 . 1, 0. 1, 0. 1)
(0 3, 0 2, 0 3) (0 2, 0 2, 0 1) (0 . 3, 0. 2, 0. 4) (0 2, 0 4, 0 1) (0 2, 0 2, 0 . 2) (0 .2 ,0 .2 ,0 . 4) (0 3, 0 3, 0 2) (03 , 0 .1 , 01)
Figure17. IN-foodweb. Table8. IN-out-neighborhoods.
w ∈ X N+ (w)
(0 3, 0 3, 0 3)
(0 3, 0 5, 0 1) (0 3, 0 4, 0 5) (0 7, 0 3, 0 2)
Human {(Trout,0.2,0.1,0.1)}
Baldeagle {(Trout,0.2,0.2,0.2), (Salamander,0.3,0.2,0.3)}
Salamander {(Dragonfly,0.2,0.2,0.2), (Mayfly,0.2,0.2,0.4)} Snake {(Salamander,0.2,0.2,0.1), (Frog,0.3,0.2,0.4)} Frog {(Dragonfly,0.3,0.3,0.3), (Mayfly,0.1,0.1,0.1)} Mayfly {(Phytoplankton,0.3,0.3,0.2)}
Phytoplankton ∅
Dragonfly {(Phytoplankton,0.1,0.1,0.1)}
Trout {(Phytoplankton,0.2,0.1,0.1), (Mayfly,0.3,0.1,0.1), (Dragonfly,0.2,0.4,0.1)}
Therefore, N+(Human ∩ Baldeagle)= {(Trout, 0.2, 0.1, 0.2)}, N+(Baldeagle ∩ Snake)= {(Salamander, 0.2, 0.2, 0.3)}, N+(Salamander ∩ Frog)= {(Dragonfly, 0.2, 0.2, 0.3), (Mayfly, 0.1, 0.1, 0.4)}, N+(Salamander ∩ Trout)= {(Dragonfly, 0.2, 0.2, 0.2), (Mayfly, 0.2, 0.1, 0.4)}, N+(Trout ∩ Frog)= {(Dragonfly, 0.2, 0.3, 0.3), (Mayfly, 0.1, 0.1, 0.1)}, N+(Mayfly ∩ Trout)= {(Phytoplankton, 0.2, 0.1, 0.2)}, N+(Mayfly ∩ Dragonfly)= {(Phytoplankton, 0.1, 0.1, 0.2)} and N+(Dragonfly ∩ Trout)= {(Phytoplankton,0.1,0.1,0.1)}
Now,thereisanedgebetweenhumanandbaldeagle;snakeandbaldeagle;salamanderandtrout; salamanderandfrog;troutandfrog;troutanddragonfly;troutandmayfly;dragonflyandmayflyinthe INC-graph,whichhighlightsthecompetitionbetweenthem;andfortheotherpairofspecies,thereisno edge,whichindicatesthatthereisnocompetitionintheINC-graphFigure 18.Forexample,thereisan edgebetweenhumanandbaldeagleindicatinga 12% degreeoflikenesstopreyonthesamespecies, a3%degreeofindeterminacyanda4%degreeofnon-likenessbetweenthem.
Human
(0 6, 0 3, 0 1) (0 7, 0 3, 0 2) (0 4, 0 3, 0 5)
(0 12, 0 03, 0 04)
Trout
(0 06, 0 06, 0 06) (0 06, 0 06, 0 15) (0 03, 0 04, 0 02)
Phytoplankton
Algorithm1: Ecosystem.
(0 3, 0 5, 0 1) (0 3, 0 4, 0 5) (0 7, 0 3, 0 2)
(0 06, 0 06, 0 1) (0 06, 0 12, 0 05) (0 06, 0 05, 0 04)
(0 03, 0 04, 0 04)
(0 3, 0 5, 0 2) (0 6, 0 4, 0 2) (0 3, 0 5, 0 2)
Figure18. CorrespondingINC-graph
Wepresentourmethod,whichisusedinourecosystemapplicationinAlgorithm 1
Step1. Inputthetruth-membership,indeterminacy-membershipandfalsity-membershipvalues forsetof n species.
Step2. Ifforanytwodistinctvertices wi and wj, t(wi wj ) > 0, i(wi wj ) > 0, f (wi wj ) > 0,then (wj, t(wi wj ), i(wi wj ), f (wi wj )) ∈ N+ (wi ).
Step3. RepeatStep2forallvertices wi and wj tocalculateIN-out-neighborhoods N+ (wi )
Step4. Calculate N+ (wi ) ∩ N+ (wj ) foreachpairofdistinctvertices wi and wj.
Step5. Calculate H[N+ (wi ) ∩ N+ (wj )].
Step6. If N+ (wi ) ∩ N+ (wj ) = ∅,thendrawanedge wi wj
Step7. RepeatStep6forallpairsofdistinctvertices.
Step8. Assignmembershipvaluestoeachedge wi wj usingtheconditions:
t(wi wj )=(wi ∧ wj )H1[N+ (wi ) ∩ N+ (wj )] i(wi wj )=(wi ∧ wj )H2[N+ (wi ) ∩ N+ (wj )] f (wi wj )=(wi ∨ wj )H3[N+ (wi ) ∩ N+ (wj )]
3.2.CareerCompetition
ConsidertheIN-digraphFigure 19 representingthecompetitionbetweenapplicantsforacareer. Let {Rosaleen, Nazneen, Abner, Amara, Casper} bethesetofapplicantsfortheparticularcareers {Medicine, Pharmacy, Anatomy, Surgery}.Thetruth-membershipvalueofeachapplicantrepresentsthe degreeofloyaltyquality;theindeterminacy-valuerepresentstheindeterminatestateofloyalty;andthe false-membershipvaluerepresentsthedisloyaltyofeachapplicanttowardstheircareers.Letthedegreeof truth-membershipofNazneenofherloyaltytowardshercareerbe 30%:degreeofindeterminacyis 50%, anddegreeofdisloyaltyis 10%,i.e.,thetruth-membership,indeterminacyandfalsity-membershipvalues ofthevertexNazneenare (0.3, 0.5, 0.1).Thetruth-membershipvalueofeachdirectededgebetween anapplicantandacareerrepresentstheeligibilityforthatcareer;theindeterminacy-valuerepresents theindeterminatestateofthatcareer;andthefalse-membershipvaluerepresentsnon-eligibilityforthat particularcareer.
Abner (0 3, 0 5, 0 6)
Surgery (0 3, 0 4, 0 5)
Nazneen (0 3, 0 5, 0 1)
(0. 2, 0. 2, 0. 2) (0 .1 , 0 .4 , 0 . 2) (0 2, 0 3, 0 4) (0 2, 0 4, 0 5) (0 .3 , 0 .4 , 0 .5)
Pharmacy Medicine
(0 7, 0 3, 0 2)
(0.2, 0 4, 0.5) (0 .1 ,0 .2 ,0 . 3) (0. 1,0. 5,0. 2)
(0 .5 , 0 .3 , 0 .1)
(0 2, 0 5, 0 3) (0 3, 0 6, 0 2)
Amara (0 6, 0 5, 0 2)
Figure19. IN-digraph.
Anatomy
(0 .2 , 0 .5 , 0 . 3)
Rosaleen (0 3, 0 5, 0 2) Casper (0 1, 0 6, 0 3)
Thus,inTable 9, N+ (Nazneen) ∩ N+ (Rosaleen)= {(Surgery, 0.2, 0.2, 0.4)}, N+ (Nazneen) ∩ N+ (Amara)= {(Pharmacy, 0.1, 0.4, 0.3)}, N+ (Nazneen) ∩ N+ (Abner)= {(Pharmacy, 0.1, 0.4, 0.5)}, N+ (Nazneen) ∩ N+ (Casper)= ∅, N+ (Rosaleen) ∩ N+ (Amara)= ∅, N+ (Rosaleen) ∩ N+ (Casper)= ∅, N+ (Rosaleen) ∩ N+ (Abner)= ∅, N+ (Amara) ∩ N+ (Casper)= {(Medicine, 0.1, 0.2, 0.3)}, N+ (Amara) ∩ N+ (Abner)= {(Medicine, 0.3, 0.3, 0.5), (Pharmacy, 0.2, 0.4, 0.5)} and N+ (Casper) ∩ N+ (Abner)= {(Medicine,0.1,0.2,0.5), (Anatomy,0.1,0.4,0.5)}.
Table9. IN-out-neighborhoods.
w ∈ X N+ (w)
Nazneen {(Surgery,0.2,0.2,0.2), (Pharmacy,0.1,0.4,0.2)} Rosaleen {(Surgery,0.2,0.3,0.4)}
Amara {(Medicine,0.5,0.3,0.1), (Pharmacy,0.2,0.5,0.3)} Casper {(Medicine,0.1,0.2,0.3), (Anatomy,0.1,0.5,0.2)} Abner {(Medicine,0.3,0.4,0.5), (Anatomy,0.2,0.4,0.5), (Pharmacy,0.2,0.4,0.5)}
TheINC-graphisshowninFigure 20.Thesolidslinesindicatethestrengthofcompetitionbetween twoapplicants,anddashedlinesindicatetheapplicantcompetingfortheparticularcareer.Forexample, NazneenandRosaleenbotharecompetingforthecareer,surgery,andthestrengthofcompetition betweenthemis (0.06, 0.1, 0.08).InTable 10, W(z, c) representsthecompetitionofapplicant z forcareer c withrespecttoloyaltyquality,indeterminacyanddisloyaltytocompetewiththeothers.Thestrength tocompetewiththeotherapplicantswithrespecttoaparticularcareeriscalculatedinTable 10
FromTable 10,NazneenandRosaleenhaveequalstrengthtocompetewiththeotherforthecareer, surgery.AbnerandCasperhaveequalstrengthofcompetitionforthecareer,anatomy.Amaracompetes withtheothersforthecareer,pharmacyandmedicine.
Nazneen (0 3, 0 5, 0 1)
Abner (0 3, 0 5, 0 6)
(0 .09 , 0 .20 , 0 . 30)
(0 .01 , 0 .20 , 0 . 30)
Surgery (0 3, 0 4, 0 5)
Medicine
(0 7, 0 3, 0 2)
Casper (0 1, 0 6, 0 3)
(0. 03, 0. 20, 0. 3) (0 01, 0 10, 0 09)
Amara (0 6, 0 5, 0 2)
(0 .06, 0.1, 0.08) (0 .03 ,0 .20 ,0 . 06)
Pharmacy
(0 2, 0 5, 0 3) (0 3, 0 6, 0 2)
Rosaleen (0 3, 0 5, 0 2)
Anatomy
Figure20. CorrespondingINC-graph.
Table10. Strengthofcompetitionoftheapplicantforaparticularcareer.
(Applicant,Career)InCompetitionW(Applicant,Career)S(Applicant,Career)
(Nazneen, Surgery) Rosaleen (0.06,0.1,0.08) 0.88 (Rosaleen, Surgery) Nazneen (0.06,0.1,0.08) 0.88
(Abner, Anatomy) Casper (0.01,0.20,0.30) 0.51 (Casper, Anatomy) Abner (0.01,0.20,0.30) 0.51
(Nazneen, Pharmacy) Abner, Amara (0.03,0.20,0.18) 0.65
(Abner, Pharmacy) Amara, Nazneen (0.06,0.20,0.30) 0.56
(Amara, Pharmacy) Nazneen, Abner (0.06,0.20,0.18) 0.68
(Amara, Medicine) Abner, Casper (0.05,0.15,0.195) 0.705
(Casper, Medicine) Abner, Amara (0.01,0.15,0.195) 0.665
(Abner, Medicine) Casper, Amara (0.05,0.20,0.30) 0.55
Wepresentourmethod,whichisusedinourcareercompetitionapplicationinAlgorithm 2
Algorithm2: CareerCompetition
Step1. Inputthetruth-membership,indeterminacy-membershipandfalsity-membership valuesforsetof n applicants.
Step2. Ifforanytwodistinctvertices zi and zj, t(zi zj ) > 0, i(zi zj ) > 0, f (zi zj ) > 0,then (zj, t(zi zj ), i(zi zj ), f (zi zj )) ∈ N+ (zi )
Step3. RepeatStep2forallvertices zi and zj tocalculateIN-out-neighborhoods N+ (zi ) Step4. Calculate N+ (zi ) ∩ N+ (zj ) foreachpairofdistinctvertices zi and zj. Step5. Calculate H[N+ (zi ) ∩ N+ (zj )] Step6. If N+ (zi ) ∩ N+ (zj ) = ∅,thendrawanedge zi zj Step7. RepeatStep6forallpairsofdistinctvertices. Step8. Assignmembershipvaluestoeachedge zi zj usingtheconditions:
t(zi zj )=(zi ∧ zj )H1[N+ (zi ) ∩ N+ (zj )] i(zi zj )=(zi ∧ zj )H2[N+ (zi ) ∩ N+ (zj )] f (zi zj )=(zi ∨ zj )H3[N+ (zi ) ∩ N+ (zj )]
Step9. If z, r1, r2, r3,..., rn aretheapplicantscompetingforcareer c,thenthestrengthof competition W(z, c)=(t(z, c), i(z, c), f (z, c)) ofeachapplicant z forthecareer c is: W(z, c)= (t(zr1)+t(zr2)+ +t(zrn ),i(zr1)+i(zr2)+ +i(zrn ), f (zr1)+ f (zr2)+ + f (zrn )) n Step10. Calculate S(z, c),thestrengthofcompetitionofeachapplicant z forcareer c. S(z, c)= t(z, c) (i(z, c)+ f (z, c))+ 1.
4.Conclusions
Graphsserveasmathematicalmodelstoanalyzemanyconcretereal-worldproblemssuccessfully. Certainproblemsinphysics,chemistry,communicationscience,computertechnology,sociology andlinguisticscanbeformulatedasproblemsingraphtheory.Intuitionisticneutrosophicsettheory isamathematicaltooltodealwithincompleteandvagueinformation.Intuitionisticneutrosophicset theorydealswiththeproblemofhowtounderstandandmanipulateimperfectknowledge.Inthis researchpaper,wehavedescribedtheconceptofintuitionisticneutrosophiccompetitiongraphs.Wehave alsopresentedapplicationsofintuitionisticneutrosophiccompetitiongraphsinecosystemandcareer competition.Weaimtoextendourresearchworkoffuzzificationto(1)fuzzysoftcompetitiongraphs, (2)fuzzyroughsoftcompetitiongraphs,(3)bipolarfuzzysoftcompetitiongraphsand(4)theapplication offuzzysoftcompetitiongraphsindecisionsupportsystems.
AuthorContributions: MuhammadAkramandMaryamNasirconceivedanddesignedtheexperiments; MaryamNasirperformedtheexperiments;MuhammadAkramandMaryamNasiranalyzedthedata;MaryamNasir contributedreagents/materials/analysistools;MuhammadAkramwrotethepaper.
ConflictsofInterest: Theauthorsdeclarethattheyhavenoconflictofinterestregardingthepublicationofthis researcharticle.
References
1. Euler,L.Solutioproblemsadgeometriamsituspertinentis. Comment.Acad.Sci.Imp.Petropolitanae 1736, 8, 128–140.(InLatin)
2. Marcialis,G.L.;Roli,F.;Serrau,A. GraphBasedandStructuralMethodsforFingerprintClassification;Springer: Berlin/Heidelberg,Germany,2007.
Information 2017, 8,132 25of26
3. Mordeson,J.N.;Nair,P.S. FuzzyGraphsandFuzzyHypergraphs1998,2nded.;PhysicaVerlag:Berlin/Heidelberg, Germany,2001.
4. Schenker,A.;Last,M.;Banke,H.;Andel,A. ClusteringofWebDocumentsUsingaGraphModel;Springer: Berlin/Heidelberg,Germany,2007.
5. Shirinivas,S.G.;Vetrivel,S.;Elango,N.M.Applicationsofgraphtheoryincomputerscienceanoverview. Int.J. Eng.Sci.Technol. 2010, 2,4610–4621.
6. Cohen,J.E. IntervalGraphsandFoodWebs:AFindingandaProblems;Document17696-PR;RANDCorporation: SantaMonica,CA,USA,1968.
7. Smarandache,F.Neutrosophicset-ageneralizationoftheintuitionisticfuzzyset.InProceedingsoftheIEEE InternationalConferenceGranularComputing,Atlanta,GA,USA,10–12May2006;pp.38–42.
8. Smarandache,F. Neutrosophy.NeutrosophicProbability,Set,andLogic,ProQuestInformation&Learning;InfoLearnQuest: AnnArbor,MI,USA,1998.
9. Wang,H.;Smarandache,F.;Zhang,Y.;Sunderraman,R.Singlevaluedneutrosophicsets. MultisapaceMultistruct. 2010, 4,410–413.
10. Yang,H.-L.;Guo,Z.-L.;She,Y.;Liao,X.Onsinglevaluedneutrosophicrelations. J.Intell.FuzzySyst. 2016, 30,1045–1056.
11. Bhowmik,M.;Pal,M.Intuitionisticneutrosophicset. J.Inf.Comput.Sci. 2009, 4,142–152.
12. Bhowmik,M.;Pal,M.Intuitionisticneutrosophicsetrelationsandsomeofitsproperties. J.Inf.Comput.Sci. 2010, 5,183–192.
13. Kauffman,A. IntroductionaLaTheorieDesSousemsemblesFlous;Masson:Paris,France,1973.(InFrench)
14. Rosenfeld,A.Fuzzygraphs.In FuzzySetsandtheirApplication;Zadeh,L.A.,Fu,K.S.,Shimura,M.,Eds.; AcademicPress:NewYork,NY,USA,1975;pp.77–95.
15. Bhattacharya,P.Someremarkonfuzzygraphs. PatternRecognit.Lett. 1987, 6,297–302.
16. Akram,M.;Davvaz,B.Strongintuitionisticfuzzygraphs. Filomat 2012, 26,177–196.
17. Akram,M.;Dudek,W.A.Intuitionisticfuzzyhypergraphswithapplications. Inf.Sci. 2013, 218,182–193.
18. Akram,M.;Al-Shehrie,N.O.Intuitionisticfuzzycyclesandintuitionisticfuzzytrees. Sci.WorldJ. 2014, 7,654–661.
19. Akram,M.;Siddique,S.Neutrosophiccompetitiongraphswithapplications. J.Intell.FuzzySyst. 2017, 33,921–935.
20. Akram,M.;Luqman,A.Bipolarneutrosophichypergraphswithapplications. J.Intell.FuzzySyst. 2017, 33,1699–1713.
21. Al-Shehrie,N.O.;Akram,M.Bipolarfuzzycompetitiongraphs. ArsComb. 2015, 121,385–402.
22. Sahoo,S.;Pal,M.Intuitionisticfuzzycompetitiongraphs. J.Appl.Math.Comput.Sci. 2016, 52,37–57. 23. Smarandache,F. TypesofNeutrosophicGraphsandNeutrosophicAlgebraicStructurestogetherwithTheirApplications inTechnologySeminar;UniversitateaTransilvaniadinBrasov,FacultateadeDesigndeProdussiMediu:Brasov, Romania,2015. 24. Wu,S.Y.Thecompositionsoffuzzydigraphs. J.Res.Educ.Sci. 1986, 31,603–628. 25. Samanta,S.;Pal,M.Fuzzy k-competitionandp-competitiongraphs. FuzzyInf.Eng. 2013, 2,191–204. 26. Samanta,S.;Akram,M.;Pal,M. m-stepfuzzycompetitiongraphs. J.Appl.Math.Comput. 2015, 47,461–472. 27.
Dhavaseelan,R.;Vikramaprasad,R.;Krishnaraj,V.Certaintypesofneutrosophicgraphs. Int.J.Math.Sci.Appl. 2015, 5,333–339.
28. Akram,M.;Shahzadi,G.Operationsonsingle-valuedneutrosophicgraphs. J.UncertainSyst. 2017, 11,176–196. 29. Akram,M.;Shahzadi,S.Neutrosophicsoftgraphswithapplication. J.Intell.FuzzySyst. 2017, 32,841–858.
30. Broumi,S.;Talea,M.;Bakali,A.;Smarandache,F.Singlevaluedneutrosophicgraphs. J.NewTheory 2016, 10,86–101.
31. Ye,J.Single-valuedneutrosophicminimumspanningtreeanditsclusteringmethod. J.Intell.Syst. 2014, 23,311–324.
32. Ye,J.Improvedcorrelationcoefficientsofsingle-valuedneutrosophicsetsandintervalneutrosophicsetsfor multipleattributedecisionmaking. J.Intell.FuzzySyst. 2014, 27,2453–2462.
Information 2017, 8,132 26of26
33. Ye,J.Amulticriteriadecision-makingmethodusingaggregationoperatorsforsimplifiedneutrosophicsets. J.Intell.FuzzySyst. 2014, 26,2459–2466.
34. Atanassov,K.T.Intuitionisticfuzzysets.VIIITKR’sSession,DepositedinCentralforSciencesTechnicalLibrary ofBulgarianAcademyofScience,1697/84,Sofia,Bulgaria1983. Int.J.Bioautom. 2016, 20,S1–S6. 35. Liang,R.;Wang,J.;Li,L.Multi-criteriagroupdecisionmakingmethodbasedoninterdependentinputsof singlevaluedtrapezoidalneutrosophicinformation. NeuralComput.Appl. 2016,doi:10.1007/s00521-016-2672-2. 36. Liang,R.;Wang,J.;Zhang,H.Amulti-criteriadecision-makingmethodbasedonsingle-valuedtrapezoidalneutrosophic preferencerelationswithcompleteweightinformation. NeuralComput.Appl. 2017,doi:10.1007/s00521-017-2925-8. 37. Lundgren,J.R.;Maybee,J.S.FoodWebsWithIntervalCompetitionGraph.In GraphsandApplication,Proceedings oftheFirstColoradoSymposiumonGraphTheory;Wiley:NewYork,NY,USA,1984.
38.
Mondal,T.K.;Samanta,S.K.Generalizedintuitionisticfuzzysets. J.FuzzyMath. 2002, 10,839–862.
39. Nasir,M.;Siddique,S.;Akram,M.Novelpropertiesofintuitionisticfuzzycompetitiongraphs. J.UncertainSyst. 2017, 2,49–67.
40. Peng,H.;Zhang,H.;Wang,J.Probabilitymulti-valuedneutrosophicsetsanditsapplicationinmulti-criteria groupdecision-makingproblems. NeuralComput.Appl. 2016,doi:10.1007/s00521-016-2702-0.
41. Sarwar,M.;Akram,M.Novelconceptsofbipolarfuzzycompetitiongraphs. J.Appl.Math.Comput. 2017, 54,511–547.
42. Tian,Z.;Wang,J.;Wang,J;Zhang,H.Simplifiedneutrosophiclinguisticmulti-criteriagroupdecision-making approachtogreenproductdevelopment. GroupDecis.Negot. 2017, 26,597–627.
43. Wang,H.;Madiraju,P.;Zang,Y.;Sunderramn,R.Intervalneutrosophicsets. Int.J.Appl.Math.Stat. 2005, 3,1–18.
44. Zadeh,L.A.Fuzzysets. Inf.Control 1965, 8,338–353.
c 2017bytheauthors.LicenseeMDPI,Basel,Switzerland.Thisarticleisanopenaccess articledistributedunderthetermsandconditionsoftheCreativeCommonsAttribution(CC BY)license(http://creativecommons.org/licenses/by/4.0/).
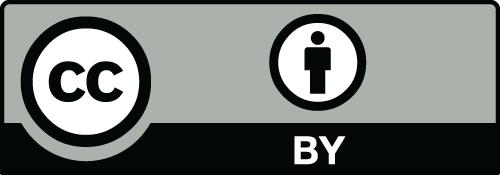