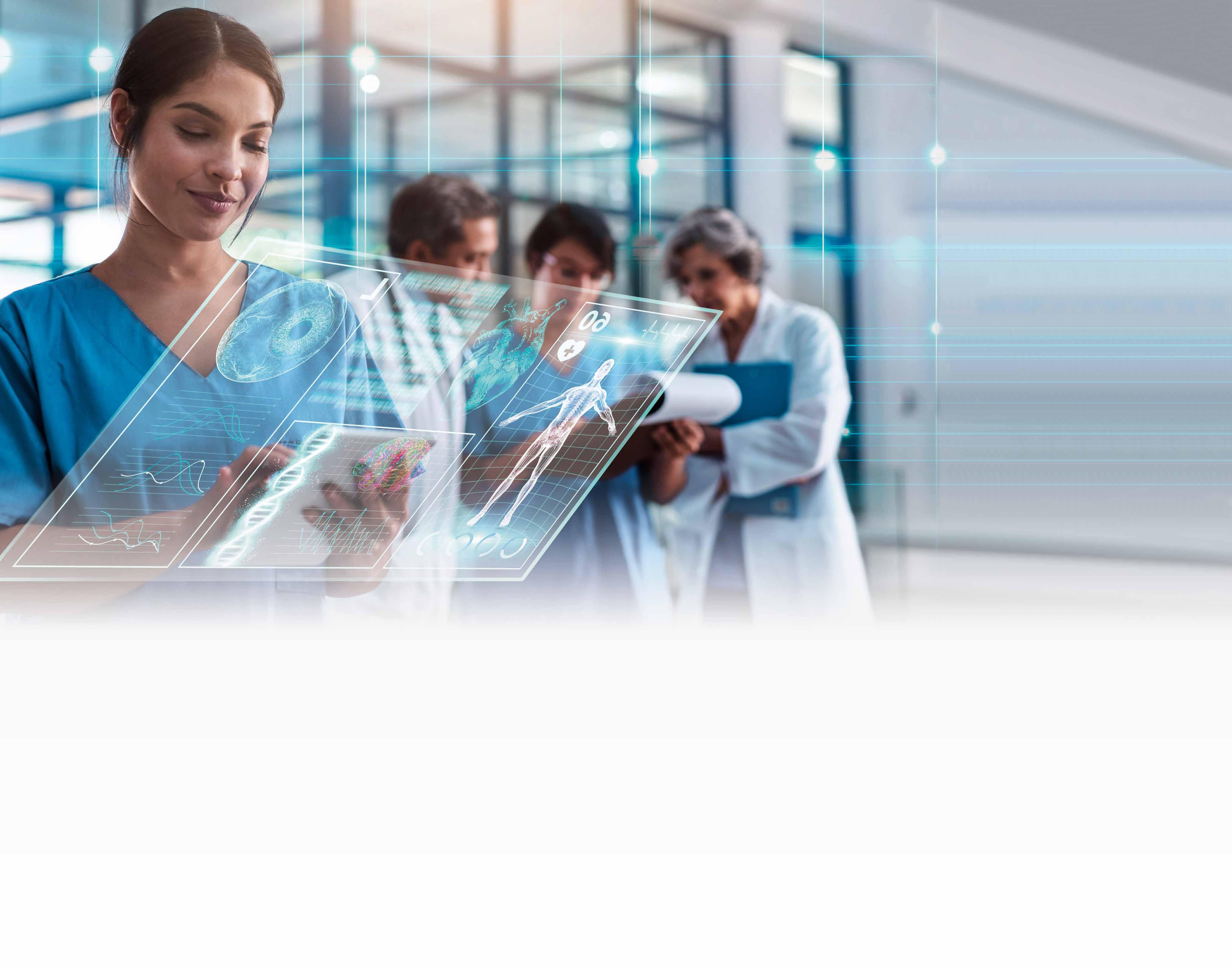
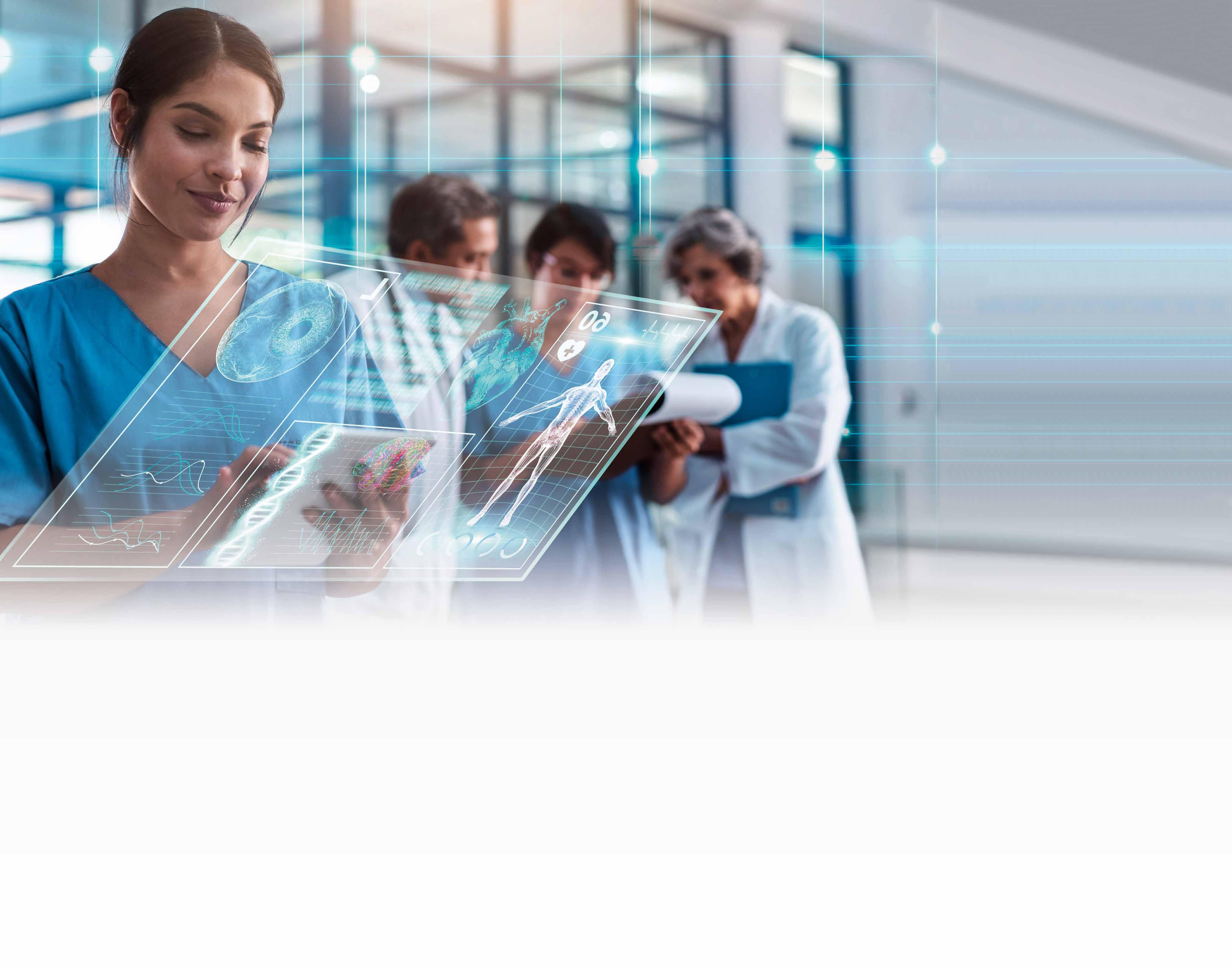
AI tools to detect disease
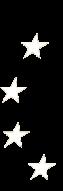
Artificial intelligence tools are increasingly being used to analyse medical images. The team behind the MedEx project are developing new machine learning methods to glean information from medical images and help detect disease at an earlier stage than currently possible, as Professor Michael Kampffmeyer explains.
An enormous number of medical images are generated in hospitals every day, providing a rich source of information for researchers looking to diagnose disease at an earlier stage than currently possible. Artificial intelligence (AI) tools play an increasingly important role in the analysis of these images, and provide valuable support for medical professionals. “An AI model may for instance predict the key points in an image that are typically used to compute distances, then a medical expert can check. AI tools mainly act as guidance tools,” says Michael Kampffmeyer, Professor in the Machine Learning Group at the University of Tromsø - The Arctic University of Norway. As part of his work in the MedEx project, Professor Kampffmeyer is developing new machine-learning methodology to detect disease from different types of medical images. “We have done some work on MRI, CT and ultrasound images. The idea is to develop fundamental methods, which can then be applied to different settings and imaging modalities,” he outlines.
Deep learning technologies
The focus at this stage in the project is on detecting lung cancer and diabetic retinopathy from medical images, with researchers using deep learning technologies to help diagnose these conditions at an earlier stage. The general idea with training a deep learning model is that some input
data is provided, with the objective ground truth, and the model learns how to map the transition from the input data to the output.
“Initially the prediction will be completely random. We then compare this prediction to the ground truth, and update the model to do a better job of learning this mapping from the input data to the output,” explains
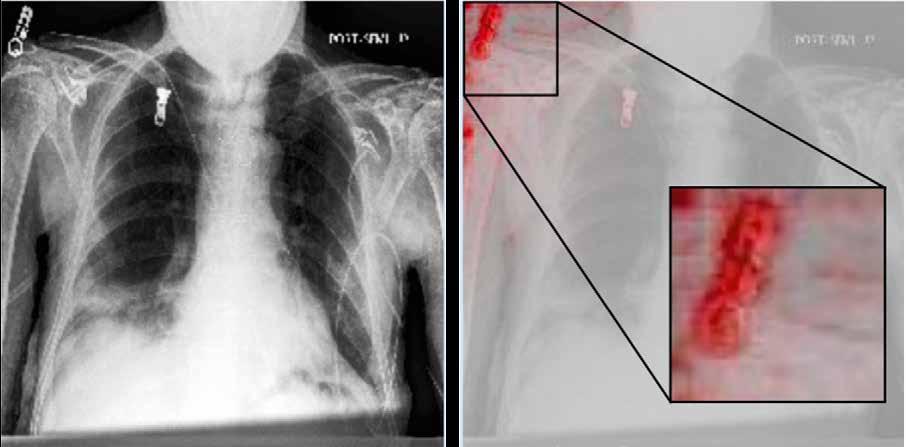
Professor Kampffmeyer. These models usually work better with annotated data, which makes it easier for the technology to classify the information; now however Professor Kampffmeyer is looking to reduce the amount of annotations required. “We are trying to develop more efficient models in the sense that we need less annotated data,” he says. “This will reduce the workload on clinicians, in terms of providing us with datasets to train these models on.”
Researchers are now working to ensure that these models are highly rigorous and provide reliable results when exposed to real data. There are many different ways in which a model can map the transition between input and output data, and Professor Kampffmeyer says it’s important to avoid taking short cuts. “For example, some hospitals have more patients with specific conditions like pneumonia. We’ve seen that models might focus on simply recognising where the data comes from in this situation, rather than solving the problem (see Fig.1 for example). These models will then not be effective on
A next step will be to take these methods and test them in practical settings, building on insights from clinical professionals. A unit has been established to essentially act as an interface between AI researchers and the hospital in Tromsø, which will facilitate the testing and ongoing improvement of innovative new methods. “We collaborate closely with medical experts and hold regular meetings with ophthalmologists for example,” says Professor Kampffmeyer. The ultimate goal is to apply these systems in clinical settings, and while there are still many hurdles to overcome on the machine learning and development side, Professor Kampffmeyer says progress is being made.
“We are not far off testing our method with ultrasound images. We hope to get closer to applying some of these systems,” he continues. “Alongside, we are also working on a lot of challenges on the methodology side. We are trying to make these models even less annotation-dependent and more data-efficient, while also making the explainability method more robust.”
“We have done some work on MRI, CT and ultrasound images. The idea is to develop fundamental methods, which can then be applied to different settings and imaging modalities.”
real data,” he explains. The aim is to develop a rigorous methodology, while at the same time providing explainable results which can be interpreted by medical professionals. “A kind of black box approach that simply tells you whether a patient has pneumonia or not is not really enough for a doctor,” continues Professor Kampffmeyer. “You need a bit more context than simply a bald answer, especially if you want to use these AI models in more difficult settings, where a diagnosis can’t be reached simply by looking at a single image.”
The project team are developing new, inherently explainable algorithms, that provide both a prediction and an explanation of the underlying reasoning behind it, which will help build trust in these methods amongst doctors. These methods are relatively general, so can be applied on various different types of data, and Professor Kampffmeyer and his colleagues have explored other medical applications in the course of the project. One is in screening for breast cancer, using mammogram images. “AI technology could make the screening process more efficient by identifying which people are at higher risk, then they could be invited for more frequent screening,” explains Professor Kampffmeyer.
NEXT GENERATION EXPLAINABLE MEDICAL COMPUTER VISION
Next Generation Explainable Medical Computer Vision
Project Objectives
The NFR FRIPRO project MedEx aims to develop generic explainable models that can learn in the presence of limited annotated datasets, addressing the current challenges of deep learning in the medical sector. The anticipated scientific solutions will have a reach beyond the health domain and contribute to moving the state-of-the-art within the field of machine learning.
Project Funding
This project is funded by the Research Council of Norway RCN FRIPRO grant no. 315029.
Project Partners
• UiT The Arctic University of Norway, Norway (Coordinator)
• Norwegian Computing Center, Norway
Contact Details
Project Coordinator, Professor Michael Kampffmeyer
UiT Machine Learning Group Department of Physics and Technology
UiT The Arctic University of Norway
Hansine Hansens veg 18, 9019 Tromsø Norway
T: +47 90602098
E: michael.c.kampffmeyer@uit.no
W: https://app.cristin.no/projects/show. jsf?id=2506954
W: https://sites.google.com/view/ michaelkampffmeyer/
Other data modalities
The techniques developed in the project could also potentially be applied on other data modalities beyond images, a topic that Professor Kampffmeyer hopes to investigate further in future, helping diagnose disease earlier and improving the prospects of effective treatment. Patient health records also provide valuable information for example, and Professor Kampffmeyer says this can be harnessed to help clinicians. “The aim is to use all the available information in order to help clinicians make better decisions. We want to use the data that has been recorded more effectively,” he outlines. The wider backdrop to this research is the challenge of maintaining highquality healthcare at a time of significant demographic change and rising demand, and Professor Kampffmeyer believes AI technology has an important role to play in this respect. “Hospitals are very keen to keep costs down, because they are not able to meet increased demand from patients under the current model as the European population grows older. Making effective use of AI is a necessary step to make healthcare systems more efficient,” he says.
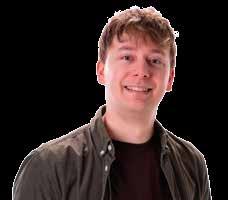
Michael Kampffmeyer is a Professor in Machine Learning at UiT The Arctic University of Norway and a Senior Research Scientist II at the Norwegian Computing Center in Oslo. His research interests include medical image analysis, explainable AI, and learning from limited labels. Kampffmeyer received his PhD degree from UiT in 2018. He is a general chair of the annual Northern Lights Deep Learning Conference, NLDL.


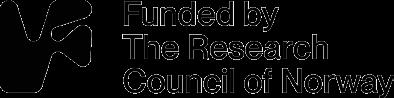
Professor Michael Kampffmeyer
Fig. 1: Left: Original image. Right: Visualisation of the model’s focus (highlighted in red). Rather than concentrating on the lung regions as expected for pneumonia detection, the model predominantly focuses on the text annotations indicating the hospital where the data was collected.