BOHRInternationalJournalofFinancialMarketandCorporateFinance 2021,Vol.1,No.1,pp.50–60 https://doi.org/10.54646/bijfmcf.010 www.bohrpub.com
MeasurementModelsforMarketRiskManagementinNigeria
AdeolaKudiratBanjo1 andOmotayoJohncallyAbere2,∗
1DepartmentofActuarialScience,LagosStateUniversityofScienceandTechnology(LASUSTECH), Lagos,Nigeria
2DepartmentofActuarialScience&Insurance,UniversityofLagos,Nigeria
∗Correspondingauthor:johncally68@yahoo.com
Abstract. Marketriskisconnectedwiththepricefluctuationsandothermarketfactormovementsonfourofthe world’smostprominentfinancialmarkets:Debtsecuritiesmarket,stockmarket,currencymarketandcommodity market.Thehighlevelofunpredictabilityinthemarketinwhichorganizationsoperateisasignificantelement drivingthefastgrowthofriskmeasurementandmanagement.Firmsareexposedtomoremarketriskinavolatile environment,whichoffersamotivatorforthemtodevelopnewandimprovedriskmodels.Thispapergenerally analysestheperformanceofdifferentmodelswhichtrytosolve,estimate,measureandmanagevariousmarketrisks inordertoderiveparameterswhichaidgooddecisionmaking.
Keywords: Insurancemarketrisk,volatility,riskmanagement,valueatrisk(VaR).
INTRODUCTION
BackgroundtotheStudy
AccordingtoAma(2009),There’sachancethatagoalorientedsystem’sfavourableexpectationswon’tbemet. Anghelache,Voineagu,CuletuandBaltac(2013)viewed Marketriskisdefinedasthepossibilityoflosingmoney onapositionduetochangesinmarketpricingandother riskvariables.Becauseeachcategorizationmaypertain todistinctaspectsofmarkethazards,thereisnoone classificationformarketrisks.Equityrisk,interestrate risk,currencyrisk,commodityrisk,marginrisk,shape risk,holdingperiodrisk,basisrisk,image/reputational risk,andsoonarethemostprevalentformsofmarkethazards.Theequityriskisthepossibilitythatstock indices/pricesorimpliedvolatilitywillchange,whereas theinterestrateriskisthepossibilitythatinterestratesor impliedvolatilitywillchange.Thecurrencyriskistherisk ofchangesininternationalexchangerates.Marginrisks arisefromtheuncertaintyoffuturecashwithdrawalsasa consequenceofmargincallstocoverunfavorableposition valuechanges.Imageriskconcernsitselfwiththereputationofanorganization.Iftheimage/reputationisdented inonewayortheother,businessesinthatmarketwill
beaffectedadversely.AnexampleofthisinNigeriain 2017wasthecasebetweentheGTBankversusthechairmanofInnosonVehicleManufacturing(IVM),DrInnocent IfediasoChukwuma.Anotherillustrationistheharmnonsettlementofclaimshasdonetothereputationofinsurance industryinNigeria.
Allthesemarketrisksposethreatstotheattainment ofmajorobjectiveandtomaximizationofadvantages (suchastheutilityfortheconsumersandprofitforthe enterprise).Risksaretakenbyallenterprisesdependingontwofactors:thebusinessanalysts’viewoflikelihood/probabilityoflossoccurrencesandviewofmoney basedonwealth(Banjo,2019).Marketriskislinkedtoprice changesandothermarketelementmovementsonfour ofthemostmajoreconomicmarkets:themarketfordebt securities,themarketforequitysecurities,andthemarket forcommodities.stockmarket,currencymarketandcommoditymarket.
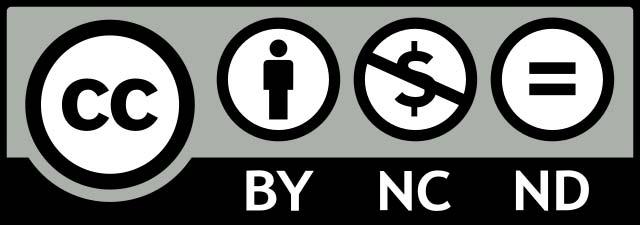
ProblemAnalysis
Themarket’sbiggesttreasureiscloselyalignedtoadequateawarenessofmovementsofpricesandothermarketrelatedfactorswhichhelptocombattheadverse effectsofvariousmarketrisks.Salientpotentialinthe
marketisduetoalackofskillsorunderstandinginrecognizingfutureestimations,thetreasurehasbeenkept untapped.Therefore,aframeworkisneededtomeasure marketriskadequatelysothatthelevelofanyuncertainty canbereducedtothebarestminimum,ifnottotallyeradicated.InNigeria,alackofknowledgeofthegenuine marketsituationhasresultedinunnecessarilyhighinflationandshortagesofgoodsandservices.Itmustbenoted thatmanyriskassessment/managementstandardshave beencriticizedforprovidingnoquantifiableimprovement inmarkethazards,whiletrustinestimatesandjudgmentsappearstobeincreasing.Furthermore,thevariance, covariance,andhistorySimulationtechniquestocalculatingVaRallpresumethatpreviouscorrelationsarestable andwillnotalterorbreakdowninthefutureorunder marketstress(LangrinandRoach,2009).Theduration marketturmoilandperiodsofseverevolatilitymakethis assumptioninappropriateashistoricalcorrelationstends tobreakdown,especiallyduringfinancialcrisesIncontrasttoanupwardgoingmarket,allindustrialsectionsface alargeincreaseincorrelations.Thisphenomenaisreferred asasymmetrycorrelation/dependencehasgreatimpacts onthemarketvalue.
Theamountofmoneythatmightbelostasaresultof marketriskcanbecalculatedinavarietyofways.Theconventionsofvalueatrisk(VaR)modelsarewidelyknown andrecognizedinshort-termriskmanagementpractice.It mustbenotedthatVaR’saccuracyisconstrainedbyavarietyoflimitingassumptions.Itisassumedthattheportfolio compositionoftheportfoliosbeingmeasureddoesnot varyovertime.Infact,becauseweliveinadynamicworld, thislimitingassumptionmaybeconsideredinappropriate overlongertimehorizons,asmanyofthepositionsinthe portfolioormarketvaluesmayhavechanged.Theonly thingconstantischange.
Oneofthemajorproblemsininsurancebusinessisclaim settlement.ItisofnonewsthatmanyinsurersinNigeriaareorhavebeeninvolvedinlitigationsasaresultof non-settlementofclaims.Thissituationhasreallyaffected theimage/growthoftheindustrynegatively.Thisproblemariseswhenaclaimoraninsurancemarketriskwhich hasa100%probabilityofoccurringisignoreddueto lackofidentificationabilityorwrongusageofunderwritingriskmodel(suchasusingindividualunderwriting riskmodelforcollectiveunderwritingriskmodeland viceversa).Theriskandreturnofinsurancebusinessis directlyrelated.Thatis,thehighertheriskthehigheristhe likelihood/probabilityofthereturns.Itmaybetoorisky toinvestinsurancefundsonsecuritiesofhigherinterest returnsbecausehigherreturnsalsogohand-in-handwith highsecurityvolatilitywhichcanleadtolossofinvested funds.Lossofinvestedfundswillinturnaffectinsurance claimsettlement.Therefore,thereisaneedtobalancethe discrepancybetweentheactuarialandthefinancialmethodsofassessingandmeasuringinsurancerisks,especially whendealingwithsingleandgrouppolicies.
ObjectiveoftheStudy
Tomanagemarketsriskseffectively,oneneedstocriticallyidentify,actuariallyassessandcarefullyprioritize theidentifiedandassessedrisksthroughtheusageof anappropriatemarketriskmodel.Thegeneralobjective ofthisstudyistoanalysetheperformanceofdifferent modelswhichattempttosolve,measureandmanagevariousmarketrisksinordertoderiveestimatesorparameterswhichappropriatelyaiddecisionmakingprocessand reduce/predictwhatthepotentialriskscenariocouldbe. Therefore,thespecificobjectives,whichmustbeattained inthecourseofthisresearchwork,arelistedbelow.
• Todevelopaframeworkthatwillmakefuture estimates/forecasts/predictionsmorereliableand authentic.
• Toexhibithowacertainchangeininterestlevelscan impactonthemarketvalue.
• TomodifyconceptsofVaRModelinordertoaid informeddecisionmakingoverlongerperiodhorizons.
• Toderiveanappropriatemarketriskmodelfor asinglepolicyandtheoverallmarketriskfor agroupofpolicies,whosevolatility/deviation(of expectedclaimvalueandactualclaimincurred)will beinsignificant.
ResearchQuestions
Thefollowingresearchquestionsmustbeansweredina specificmannerinthisstudy.
• Howcanwemakeourstatisticalestimatestomeasuremarketrisksadequatelyinordertoreduce uncertaintylevelstothebarestminimum?
• Howcandurationperiodsofhighvolatilityandmarketturbulenceduringfinancialcrisesaffectmarket value?
• Howdoothermoments(thirdandfourth)helpto modifyVaRMethodofriskmeasurement/modeling?
• Howcanwestrikeabalancebetweentheactuarialandthefinancialmethodsofassessinginsurance marketrisksinordertocombatclaimsettlement problems?
ScopeandLimitationoftheAreaofStudy
Thisstudygenerallyfocusesontheanalysisofvarious modelsthatattempttoproffersolutionstomarketrisk problems.ItsscopeshallbelimitedtosituationsinNigerianmarkets(financialandinsurance),althoughreferences willbemadetoglobalsituations.Themajorlimitation envisagedinthisstudyislikelytobedifficultyingetting appropriate/relevantdataand/orunwillingnessofmarketoperatorstoreleasesensitivedataforanalysisforthe fearoffallingintothehandsofcompetitorsorleakingtheir
financialoperations/informationtowisecustomerswho canusesuchinformationagainsttheminmakingmore profitforthemselveswhichleadstonoorlowprofitfor theservicingoperators.
SignificanceoftheStudy
Thereisneedtocontrolvariousmarketrisksandbalance thepossibilityofgains.Thedynamicinterdependencies ofmarketrisksaredeterminedbytheoptimalmanagementofeachtypeofmarketrisksearliermentioned, takingintoaccountthemodels/systemsofrisksandpotentialfortransformationoftherisks.Thegreatdegreeof unpredictabilityinthemarketinwhichfirmsoperateis asignificantelementdrivingthefastgrowthofriskmeasurementandmanagement.Thefindingsofthisstudywill beextremelyusefultomarketparticipantsandstakeholders.Itmayalsoserveasanassettostudents/lecturersand othersintherelatedfieldofstudy.Finally,policymakers, investors,andthegeneralpublicwillgreatlybenefitfrom theresearch.
THEORETICALOVERVIEW
AccordingtoNavarrete(2006),Thevariancecovariance andhistorysimulationtechniquestocomputingVaRare effectivealternativestoMonte-Carlosimulationsforall specifiedmultivariatemodels.Theyenhancethevariance covariancematrixestimation.TheGaussiancopulaand wellspecificmarginalmaybeusedtoprovideaforecast ofassetdistributionusingMonte-Carlosimulation.Allowingforempiricalfeaturesinstockreturns(suchasautoregression,asymmetricvolatility,skewness,andkurtosis) inthemodellingprocessisthoughtcrucial.Negatively basedcorrelationsandvariancecovariancessufferfrom substantialestimateerrorsifthesequalitiesarenottaken intoconsideration.Fluctuationsinmarketpricesandother marketfactorsplaymajorroleonthemarketvalueofan investment.Oneofthemostsignificantandunderappreciatedaspectsofmarketriskmonitoringandmodellingis modelrisk.Itcastsalongshadowoverallaspectsofrisk management,andprudencedictatesthatwetreatitseriously.Werelyondataandindexestoestimatevolatility.We shouldcontinuetoquestionourselveswhatwouldhappen ifourassumptionsweretocollapse.
FinancialRisk
AccordingtoAndreJ.Blaauw,GroupChiefRiskOfficer ofUnitedBankforAfricaPLCin2009,marketriskcan occurwhenbanksacceptfinancialassetssubjecttomarketpricevolatilityascollateralforloans.Poormarket riskmanagementprocedurescanswiftlyresultinconsiderablelossesinturbulentmarketconditions,aswell ascatastrophicinstitutionalcollapseinextremecases. AccordingtoKallestrap(2012),theglobalmarketcrisishas
revealedthatmarketsarebecomingmoreinterconnected, complicated,andunpredictablethanpreviouslythought. Themanagementofmarketriskishighlycomplexbutsize ofthemarketriskexposurecanbereducedinsuchaway toallowbanksavoidpotentiallossesthatcanbeincurred underextremeandadversemarketvolatility(Hubbard, 2009).
Financiallossesasaresultofmarketvolatilityhave resultedinagradualdeteriorationinoureconomicbasis andforeignreserves.Thishasalsodiscouragedsmalland medium-sizedbusinesses,whichareoneoftheprimary driversofanyeconomy.Inbanking,theknowledgeof marketrisksandhowtomodelthemareverycrucialto successfulbusinessoperations.EnyiandAdebawo(2014) suspectedthatthe2008financialsectorcollapseinNigeria wascausedbyanincorrectriskassessmentandexposure model.Portfoliotheoryandthecapitalassetpricemodel shouldbereviewedperiodicallyinordertoguideinvestors onriskoflossoffinancialvalueduetofluctuationsin marketpricesandotherfactorsasmanyformsofmarket risksarise.Bankruptcyisthemostdramaticexampleof marketriskmanagementfailure.Derivingvarianceparametersfrompastmarketratedatamightaidinestimating theprobableriskscenarioforaparticularstatisticalconfidencelimit.InterestrateriskisdefinedbyEltonand Gruber(1995)asthelikelihoodthatchangesininterest rateswillhaveanegativeimpactonacertainfinancial instrumentorportfolio.AccordingtoEmilia(2010),the mostoftenutilisedmethodsforanalysingbankinterest rateriskincludediscrepancyanalysis,simulationmethods, anddurationmethods.TheDiscrepancyAnalysisMethod measuresthedifferencebetweeninterest-sensitiveassets andliabilitiesoveraspecifictimeperiod.Whentheamount oftheassetsbeingexaminedduringaspecifictimeexceeds thesumoftheliabilities,thebankhasapositivedisparity. Ifabankhasanegativedisparityandinterestratesrise, netinterestincomewillfallsincehigherinterestrateshave moreobligationsthanassets.
Inthesamevein,revenueswillalsodecreaseifthebank hasapositivedifferenceandinterestratesfall.Therefore, thediscrepancyhelpstoshowtherisktowhichinterestincomeisexposed.Unfortunately,thismethoddoes notrecordchangesinpaymentdatesthatmayoccurasa resultofchangesintheinterestenvironment.Thismethod issuitablewhenthereisidenticalmovementofinterest rates.Anewmodelisrequiredduetothenon-similar behaviorofinterestratesacrossdifferenttimeperiodsin dynamicmarkets.Thesimulationapproachisbasedonthe ideathatinterestratefluctuationsaredynamicratherthan static.Monte-Carlosimulationisusedasastandardfor theevaluationofportfolios,andincorporatesmanymarketvariables.Butinorderforthismodeltobereliable,itis necessaryateachcalculationtoperformasufficientlylarge numberofrepetition/recalculation.Thisisaverydemandingtaskintermsofcomputingpower.Duetothedemands andcomplexityofthissystem,itissometimesdifficultfor
abank’smanagementtokeeptractofwhattheirriskmanagersareactuallydoing
Profit/lossisuncertainduetomovementofthemarketfactors.Therefore,aframeworkisneededtomeasure financialriskeffectively.Berkowitz(2001)believedthat thetraditionalsolutionassumesamean-varianceframework.Inotherwords,weestimatemarketrisksinterms ofthemeanandvariationorstandarddeviationofprofit/ loss.Nonetheless,theassumptionofnormalityhasitslimitationsasthedistributionrequiresonlytwoparameters (i.e.firstmoment(mean)andsecondmoment(variance) (DowdK,2002).AccordingtoEltonandGruber(1995), Portfoliotheory,forexample,assumesthatthebehavior ofthereturnsonanygroupofassetscanbecharacterized intermsofanexpectedreturnvectorandavariancecovariancematrixthatdescribestherelationshipbetween individualreturns.Aspreviouslyindicated,oneofthe mostseriousVaRhasthedrawbackofnotbeingsubadditive.Tosolvethisdifficulty,wemustfirstexplainthe conceptofsub-additivity.Ariskmeasurep(˚u)issaidtobe non-sub-additiveifthetotalofthemeasuredrisksofpositionsXandYislessthanorequaltothetotalofthevarious positions’estimatedriskswhenexaminedindependently, i.e.
Sub-additiveindicatesthatthesumofindividualhazardsdoesnotraisetotalrisks.Ifrisksaresub-additive, combiningthemwilloverestimatecombinedrisk,meaningthatthesumofriskscanbeusedasaconservative estimateofcombinedrisk.Thisenablesdecentralizeddecisionmakinginsideafirmsinceasupervisormayalways usethetotaloftherisksoftheunitsreportingtohimasa cautiousriskassessment.Iftherisksarenotsub-additive, however,addingthemtogetherleadsinanunderestimate oftheoverallrisk,makingthesumofhazardsuselessas ariskmeasure.Wewantourriskassessmentstobeconservativelyorimpartiallybiasedinriskmanagement.Asa result,foranyriskmeasure,sub-additiveisahighlydesirableattribute.Unfortunately,VaRisseldomsub-additive, anditcanonlybeifwemaketheimplausibleassumptionthatProfit/Lossorreturnsareslightlymorewidely orconsistentlydistributed.Inaddition,bymeetingthe sub-additivecriterion,coherentriskmetricsaccuratelyrepresentdiversificationeffectsandsimplifydecentralized decision-making.
Forinstance,ifXandYaretworiskpositions’futurevalues,ariskmeasurep(·)issaidtobecoherentifitsatisfies thefollowingproperties:
Where anynumber
Thesub-additivepropertyhasalreadybeenexplained. Thehomogenousandmonotonouspropertiesareplausibleaprioricriteria,andcombinedtheyindicatethatthe functionp( )isconvex.(Scaillet,2000).Lastbutnotleast theconditionspecifiesthataddingafixedamountnto thepositionwillreduceriskbythesamevolumesince theend-of-periodportfoliovaluewillgrow.Thehighestpredictedlossonacollectionoflossvaluesandtheir associatedprobabilitiescanbeviewedasaconsistentrisk indicator.
Riskmodellingusesanumberofstrategiestoanalyzea portfolioandanticipatethepotentiallossessustainedfor avarietyofhazards.Econometricapproachesareusedin financialriskmodellingtoassesstheaggregateriskina financialportfolio(AlmgrenandChriss,2002).Manybig financialintermediaryorganizationsuseriskmodellingto assistportfoliomanagersindeterminingtheamountof capitalreservestokeepandtosteertheirpurchasesand salesofvariousclassesoffinancialassets.Previously,risk analysiswasdonesubjectively,butnowthatsophisticated computingtoolsisavailable,quantitativeriskanalysiscan bedonerapidlyandeasily.
AccordingtoMandelbrotandRichard(2006),modelingchangeswithlimitedvariancedistributionsisknown tobeineffective.Theydiscoveredthatpricechangesin financialmarketsdonotfollowaGaussiandistribution. Largechangesupordownaremorecommonthanwhata Gaussiandistributionwithanassumedstandarddeviation wouldpredict.Furthermore,thefairvalueorfuturecash flowsofaspecificfinancialinstrumentmayfluctuatedue tochangesincurrencyexchangerates.Understandingand managementofriskincurrencyexchangeratevolatility canbeverycomplicatedbecausethereexistsanimperfect correlationbetweencurrencyexchangeratesandinterest marketrates(Alexander,2001).
InsuranceRisk
Inacompetitiveinsurancemarket,themarketriskload indicatesequilibriumpricing(Zhang,2006).Themarket riskloadandtheinsurerspecificriskloadcanbecalculatedindependentlyfromthetotalriskloadforapolicy. Throughpremiumpayments,uncertaintyoffuturelossis eliminatedorreducedbytheinsured.Thepremiumscollectedbytheinsurerserveastherewardfortakingthe insuredrisk.Therefore,thepremiumsserveasthecostof theinsurancemarketrisk.Theactuarialestimateofinsurancemarketriskhasevolvedsignificantly.Accordingto classicpremiumprinciples,ariskloadisdeterminedbythe volatilityoftheinsuredlossitself,andvolatilityisquantifiedbythevarianceorstandarddeviation.Accordingto Zhang,thesemethodologiesareunsatisfactorysincethey
quantifytheriskoftheinsuredbutnottheriskofthe insurer.
Becausetheinsurancemarketiscompetitiveandmarketparticipantsarerationaldecisionmakers,aneffective insuranceriskmodelshouldaccountnotjustthevolatility ofthepolicylossbutalsotheinsurancecompany’sportfolioandmarketcompetitiverisk.Thefrictionalcostof capitalisoneoftheriskfactorsassociatedwithaninsurer’s capitalstructure.Asaresult,it’sappropriatetodividethe insurer’stotalriskintotwocategories:marketriskand specificrisk,whichmaybequantifiedseparately.Kato andYoshiba(2000)discoveredthattheriskmodelreflects twokeypricingperspectives:actuarialandfinancial.The risk/returnoftheinsurancecompaniesisaddressedunder actuarialviewwhilethefinancialviewexaminesportfolioofshareholders.Theactuarialtechniquehandlesmutual selectionbutpayslittleconsiderationtothewell-beingof theshareholders.Thetwomodelsworkwelltogether.
BacktestandStresstest
Backtesting
Backtestingmightprovideuswithadditionalinformation regardingtheappropriatenessorinsufficiencyoftherisk modelbeingutilized.Thisprocedurehelpstoevaluatethe marketriskmodel.Inordertodoso,acertainVaRconfidencelevel,specificmarketinstrumentpositions,anda specificcollectionofmarketprice/returndatamustbesupplied.Whenevaluatingmarketriskmodels,backtesting iscrucial.Itnecessitatestheuseofquantitativestatistical approachestodeterminewhetherornotamarketevaluationmodelisacceptable.Itmaybeusedforthreedifferent things.Thefirstistoallowtheconclusionthattheevaluationsarestatisticallyconsistentwiththerelevantoutputs tobereached.Italsoassistsriskmanagersindetecting flawswithintheirriskmodels.Finally,itaidsinranking theperformanceofseveralalternativemarketmodels.The validityofthevalueatriskmodels,likethatofothermodels,shouldbeverifiedonaregularbasis.
Stresstests
Inthesimilarvein,stresstestsarerequiredtoverify whetherafinancialorganizationisstrongenoughtowithstandmajormarketshocks.Becausepreviousvolatility maynotholdinthefuture,wemuststress-testhistoricalparameterstoassuretherobustnessofmaximumloss estimations.Thisisbestaccomplishedthroughsimulation, inwhichaportfolioisrunthroughalargenumberof probablemarketsituations(JamshidianandZhu,1997). Thetestsassesstheimpactofunusual,butnotimpossible,marketsituationsonrevenueorfinancialpositions. Indoingso,adecisionshouldbemadeastowhetherto stressonlyonevariableovertimeoragroupofnumerousvariables.Thestresstestfindingscanbeutilizedfor
avarietyofpurposes,includingriskreportingandidentification,risklimitestablishment,capitalallocation,and premiumadjustment.Withstresstests,theeffectsofexceptionaleventsinthemarketareanalyzed.
METHODOLOGYANDMODEL
SPECIFICATION
Introduction
Thissectiondescribeshowdataandresourcesforthestudy wereacquired.Itstatessomeproblemsencounteredinthe processofdatagathering.Italsofocusesonthemany modelsandmetricsthatwillbeusedtoprovideempirical supportforthestudy.
ResearchDesign
Designreferstotheplanandstrategyofinvestigation conceivedsoastocontrolvariances(Asika,2004).Data collectingisnotconfinedtoasinglemethod.Personal interviewsand/orbusinessobservationswereconducted withspecialists,acquaintances,andfamilymembersinthe relevantorganizations.Themajorityofthesecondarydata camefromtheinternet,periodicreports,forums,articles, newspapers,textbooks,paperpresentations,journals,and otherpublicationsfrombusinessorbusiness-relatedorganizations.
PopulationoftheStudy
ThisstudyfocusesontheanalysisofNigerianmarketrisks throughtheuseofdifferentmodels.Thestudy’sgeographicalscopeisnotrestrictedtoNigerianbusinessesbutmay alsobeappliedtobroaderscenarios.
SampleSizeoftheStudy
Basedonthemodels,analyseswillbecarriedoutoninsuranceandfinancialrisks.Furthermore,inorderforthis studytobearesoundingsuccess,themajoranalysiswillbe doneonthelendinginterestratesinNigeria(from1970to 2018)obtainedfromtheCentralBankofNigeria.Thefocus istoderiveestimatesforfutureinterestratessothatthe marketplayerscanposition/preparethemselvesagainst adversemovementsoftheserates.Thesamplesizeisdeterminedbythesizeofthepopulationstudied.
ProblemsEncountered
Themainissueinthisstudywasfinancialfirms’unwillingnesstoprovidetheirdataforresearchpurposes.Some claimedthatthemainreasonwastopreventcompetitors fromgainingaccesstotheirsecretsthroughdetailedanalysisoftheirrawdata,whileothersclaimedthataletteror directivefromtheofficeofthe“GovernorofCentralBank
orPresidentoftheFederalRepublicofNigeria(GCFR)” wasrequiredbeforesensitivedatacouldbereleasedfor researchpurposes.Thiscauseddelayinthedurationof thestudy.Also,figuresofthemajordataobtainedfrom differentsourceswereslightlydifferentformeachother. Forinstance,alendingrateof16.23%inaparticularyear fromonesourcecouldbe16.22%inanothersource.This mightbeduetoapproximationorfluctuationproblems. Throughproperlyconstructedandpreciselyformulated models,stepsweremadetolimittheconsequencesofthis toabareminimum.Nonetheless,thequalityofdatacollected/usedwashighlypraisedandsuggestedforfuture researchersorpersonsdirectlyinvolvedinthestudyby concernedfansofthisfieldofstudy.
ModelSpecification
Thissectiondescribesthemodelsthatwillbeutilisedon thevariousmetricstoprovideempiricalevidenceforthe study.
Financialrisk VALUEatRISK(VaR)MODEL
ArandomvariableXisgivenatypicaldistribution,which mean µ andvariance σ2 (orstandarddeviation σ)forthe probabilitythatXtakesthevalue x,f (x)obeysthefollowingprobabilitydensityfunction(pdf) f (x)= 1 σ√2π exp 1 2 [(x µ)/σ]2 (6)
Where x isdefinedover ∞ < x < ∞ Ifthemean(µ)=oandthestandarddeviation(σ)=1, thisisknownasastandardnormal
DURATIONMODEL
Tocalculatetheduration,oneneedstocalculatetheNet PresentValue(NPV)first.
Thesummationisthendividedbytheinstrumentvalue inordertoarriveattheduration.
NPV = n ∑ i=1 Pi (1 + r)i (7)
Where i = yearofpayment; P = principaltobepaid; r = interestrate.
LEASTSQUAREMODEL
Table 1 willbeanalysedbyLeastSquareMethodwhich willgiveapracticalestimateofthetimetrend.Itis
assumedthatthetrendislinear.Hencetheequationofthe lineartrendisdefinedas:
ˆ Y = a + bX (8)
Where: X = transformedtime(t); ˆ Y = theestimatedtrendvalueforagivenperiod; a = valueofthetrendlineattimezero(i.e.@t = 0); b = theslopeofthetrendline(i.e.thechangeinYperunit changeintime).
Theconstants a and b canbecalculatedusing:
b = n ∑ XY ∑ X ∑ Y n ∑ X2 (∑ X)2 (9)
a = ∑ X2 ∑ Y ∑ X ∑ XY n ∑ X2 (∑ X)2 (10)
At ∑ X = 0;
b = ∑ XY ∑ X2 (11) a = ∑ Y n (12)
StandardErroroftheEstimate(Se)
Se isameasurethatassessesthereliabilityoftheresult obtained.Itmeasuresthevariabilityorscatteringofthe observedvaluesaroundtheregressionline.Itisevaluated usingtheformulabelow.
Se = ∑ (Y Y)2 n 2 (13)
Insurancerisk
Assuminganinsurancemarketcontains N policieswith randomlosses x1,x2,... xN,wherethemarketpremiumfor policy i is Pi.
Thepremiumwillbeinvestedinariskfreeassetwith rateofreturn rf.If Ri istherateofreturnonpremium,the meanandthecovarianceoftherandomreturnsare:
µi = E(Ri )=(1 + rf) E(Xi) Pi (14)
σij = Cov(Ri, Ri ) = cov xi pi , xj pj = 1 Pi Pj cov(Xi Xj ) (15)
Table1. HistoricallendinginterestratesinNigeria(1970–2018). YearInterestRate(%)YearInterestRate(%)YearInterestRate(%)YearInterestRate(%) 19707198210.25199421200616.90 19717198310199520.18200716.94 19727198412.5199619.74200815.48 1973719859.25199713.54200918.36 19747198610.25199818.29201017.59 19756198717.5199921.32201116.02 19766198816.5200017.98201216.79 19776198926.8200118.29201316.72 19787199025.5200224.4201416.55 19797.5199120.01200320.48201516.85 19807.5199229.8200419.15201616.87 19817.75199336.09200517.95201717.88 201818.00
Source:CBNStatisticalBulletin(www.cbn.gov.ng).
DATAANALYSIS,PRESENTATIONAND
INTERPRETATION
InsuranceRisks
FromtheEquations(14)and(15)statedinsection“Insurancerisk”,itiseasytomodeltheriskforasinglepolicy i andtheoverallmarketriskforall n policies. Theriskof ith policycanbederivedbymultiplyingthe premiumdiscountfactor(i.e. Pi (1+r f ) )byitsexpectedvalue inEquation(14). (16)
Also,for n policies,theoverallmarketriskloadis; (17)
FinancialRisks
VALUEATRISK(VaR)MODEL
InlinewithEquation(6),Figure 1 isderivedandexplained below.
INTERPRETATION
Accordingtothegraph,it’smorelikelythattheconsequenceswilloccurtowardsthemean µ.Thestandard deviationdeterminesthedistributionofprobabilitymass aroundthemean .Toputitanotherway,thehigher
thestandarddeviation,themorescatteredtheprobability mass.
Asaresult,thepdfissymmetricaboutthemean.The randomvariable X isaslikelytotakeaparticularvalue x µ astotakethecorrespondingnegativevalue (x µ) Thepdftailsawayonbothsides,withtheleft-handtail correspondingtoextremelylowrandomrealizations.
Variables,whiletheright-handtailcorrespondstohigh realizationandmorelikelyoccurrenceofrandomevent. Assumingaparticular x-value(say 1.645)ischosenfrom anormaldistributiontable,wecanregardthisvalueasa profitof 1.645 (left-handside)orlossof 1.645 (right-hand side).Invariably,itmeansthatthereisa5%(0.05)probabilityofmakingalossor95%(0.95)probabilityofmaking profit.
Thevalue 1.645 canthenbedescribedasthevalueatrisk (VaR)at 95% confidencelevel.
SUB-ADDITIVITYOFVARMODEL
Aportfoliooftwoshortbetsonvery-out-of-the-money binaryoptionsisagoodcounter-examplethatshowsVaR’s sub-additivity.Assumethateachbinarychoicehasa 6% probabilityofapayoutof ₦1.5manda 94% probabilityof apayoutof ₦0.
Therewardsarebasedonseparatelydistributedunderlyingvariables,thereforethepayoffononebinaryoption isindependentofthepayoutontheother.IftheVaRconfidencelevel(cl)issetto95%andtheholdingperiodisset totheamountoftimebeforetheoptionsexpire,eachpositionhasaVaRof0atthe95%level.Ifwecombinethetwo positions,however,theprobabilityofaN0payoutfallsto lessthan 95% andtheVaRispositiveandequalto ₦1.5m.
Asaresult,theVaRofcombinedpositionsislargerthan thesumoftheVaRsofindividualpositions,asshown inEquation(2).Therefore,VaRisnotsub-additive.The
Figure1. TheNormalProbabilityDensity.
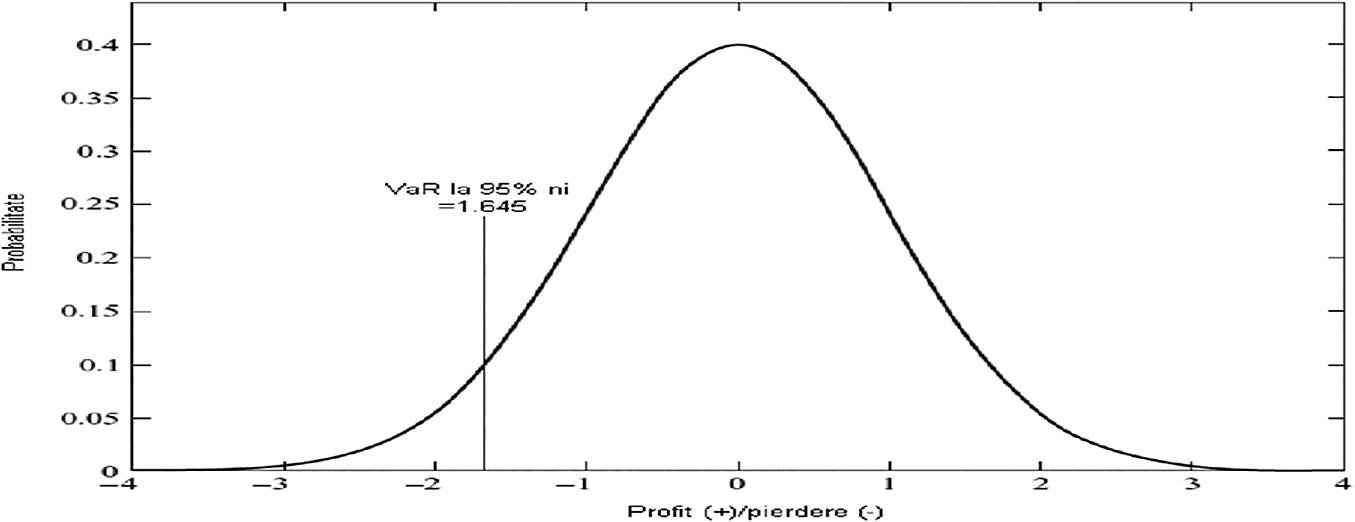
Table2. TheVaRofindividualpositions. PositionXPositionY
(₦’m)PayoutProbability(₦’m)PayoutProbability 1.50.06 1.50.06 00.9400.94 VaRat95cl1.00VaRat95cl1.00
Table3. TheVaRofcombinedpositions. Payout
(₦’000,000)CalculationProbability 30.062 =0.06X0.060.0036 1.5(0.06)(0.94) + (0.94)(0.06)0.1128 = 2(0.94)(0.06) 00.942 =0.94x0.940.8836 VaRat1.00 confidencelevel(cl)
illustrationofthegivenexampleisexplainedintheTable 2. Furthermore,VaRisnotsub-additiveandsoisnotaconsistentriskmeasure.Asaresult,anotherideaforgauging marketriskistheconditionalvalue-at-risk(CVaR).Thisis sub-addictiveandcoherentforgenericlossdistributions, includingdiscretedistributions.
However,thepresumptionofnormalcyhaslimitations asthedistributionrequiresonlytwoparameters(i.e.first moment(mean)andsecondmoment(variance)(DowdK, 2002)).Otherinstances(thirdandfourth)canbecrucial. Thethirdinstantindicatesthedistribution’sasymmetry. Thiscausesskewness.
Skewness = E(x µ)3 σ3 (18)
Asymmetricornormaldistributionhasaskewness coefficientof0,whileanasymmetricdistributionhasa skewnesscoefficientofnon-zero.
Ifthedistributionisskewed,wemustaccountfor itsskewnessifwearetoappropriatelyestimateitsrisk
probabilities.Thefourthmoment(kurtosis)parameter indicatesthefatnessofthedistribution’stails.
Kurtosis = E(x µ)4 σ4 (19)
Thetailsofthedistributionwillbethesameasthenormaldistributionifthekurtosisparameterissetto3.A kurtosisvaluegreaterthan3showsbroadertails,implying thatanextremeevent(i.e.lossseverity)ismorefrequent butlesslikelytobehuge.Atailvalueoflessthan3suggeststhatextremeoccurrencesarelesslikelytobegreater thanexpected.Theextremeeventsherecaneitherresultto gainorloss.Exceptwhendealingwithasymmetric(i.e. zero-skew)distributionwithakurtosisof3,thenormalityassumptionisunsuitableandcanleadtosubstantial mistakesinmarketriskanalysis.Becauseofitsstrongrelationshipwithsomeofthemostprominentwaystofinancial riskmeasurement,itiscriticaltoverifyfornormalcy.For example,VaRcoupledwithdailymarketriskmonitoring andcontrol,captherisk/lossatacertainlevelofconfidence.
Implementingvolatility-basedlimits,whichadjustthe sizeofpositionlimitsautomaticallyinresponsetorising marketvolatility,isagoodwaytoguaranteemarketrisks arecappedatanypre-determinedlevel.
DURATIONMODEL
InaccordancewithEquation(7),imagineabankpurchases a5-yearbondwithanominalvalueof$2000andanannual interestrateof10%.
NPV = 5 ∑ i=1 $2000 (1 + 0.1)i = $7,581.57
Duration(D) = $7,581.5 =
INTERPRETATION: Theperiodof3.79yearsisameasure oftheaveragelife-cycleoftheinterestsensitivityofthe 5-yearbond.
Table4. Primelendingrateanalysis(2010–2018).
YearInterestRate(Y)TransformedTimeTrend(X)X,YX2 Y (Y Y)2 201017.59 4 70.361616.529331.125014 201116.02 3 48.06916.65450.40259 201216.79 2 33.58416.779670.000107 201316.72 1 16.72116.904830.034163
201416.5500017.030.2304
201516.85116.85117.155170.093127 201616.87233.74417.280330.168373 201717.88353.64917.40550.22515 201818.00472.001617.530670.220274
∑ Y = 153.27
∑ X = 0 ∑ = 7.51 ∑ 2 = 60 ∑ = 2.499198
Table5. Primelendingrateanalysis(2011–2017).
YearInterestRate(Y)TransformedTimeTrend(X)X,YX2 Y (Y Y)2 201116.02 3 48.06916.18250.026406 201216.79 2 33.58416.392140.15829 201316.72 1 16.72116.601790.013975 201416.5500016.811430.068345
201516.85116.85117.021070.029265 201616.87233.74417.230710.130115 201717.88353.64917.440360.193286
∑ Y = 117.68
∑ X = 0 ∑ X,Y = 5.87 ∑ X2 = 28 ∑ = 0.619682
Table6. Primelendingrateanalysis(2012–2018). YearInterestRate(Y)TransformedTimeTrend(X)X,YX2 ˆ Y (Y Y)2 201216.79 3 50.37916.42250.135056 201316.72 2 33.44416.646430.005413 201416.55 1 16.55116.870360.102629 201516.8500017.094290.059676 201616.87116.87117.318210.200896 201717.88235.76417.542140.114147 201818354917.766070.054723
∑ Y = 119.66 ∑ X = 0 ∑ X,Y = 6.27 ∑ X2 = 28 ∑ = 0.672539
Unfortunately,thismethoddoesnotrecordchangesin paymentdatesthatmayoccurasaresultofchangesinthe interestenvironment.Thedurationmethodforresolution displaysthetimeandquantityofcashflowsreceived beforetheinstrument’scontractuallystatedmaturityterm. Thelongerthematurityperiodandthewaitforthenext changeintheinstrumentprice,thelowerthepayments receivedaheadtomaturity.Becausetheperiodislonger,a changeininterestrateshasahigherinfluenceoneconomic value.
LEASTSQUAREMODEL
Inthissection,Table 1 wasbeanalysedbyusingLeast SquareModelinrelationtoEquations(8)to(13)already statedintheprevioussection.
FromEquation(12), a = 153.27 9 = 17.03
UsingEquation(11), b = 7.51 60 = 0.12517
FromEquation(8), Y = 17.03 + 0.12517(20)
FromEquation(13), Se = 0.5975 (21) Y = 16.8114 + 0.2096X (22)
Table7. Comparisonofresults. DecisionStandardProbability
TableEquationError(Se)ofError
4 Y = 17.03+0.12517 X 0.597535.70%
5 Y = 16.8114+0.2096 X 0.352012.93%
6 Y = 17.0943+0.2239 X 0.366813.45%
Se = 0.352(23) Y = 17.0943 + 0.2239X (24)
Se = 0.3668(25)
FromTable 7,thebestequationtobeusedfortheestimatesoffuturelendinginterestrateis Y = 16.8114 + 0.2096 X asderivedfromTable 5 becausethestandarderrorand thechanceoferroraretheleast.
FINALRESULT
Table8. Estimatedlendingrates(2023–2025). YearXInterestRate(%) 2023918.70 20241018.91 20251119.12
CONCLUSIONANDRECOMMENDATION
ConclusionandSummary
Anyfinancialornon-financialentitywithaportfolioof financialassetsisexposedtomanytypesofrisksand,as aresult,shouldapplyriskmeasurementandmanagement strategiestooptimizethewayariskistaken.Asaresult, theriskoflargeeconomiclossesorevenbankruptcyis reduced,andtheinstitutionsbecomemorecompetitive. Oncetheestimatesofthedistributionoffuturechangesare available(e.g.asshowninTable 8),toestimatetherisk, you’llneedtouseariskmeasurement.Itisalsonecessary tovalidatethemodelthroughback-testing(asshownin Table 7).Theriskattachedtoaparticularinvestmentis relatedtothereturnonsuchinvestment.Managementof theriskcanbeeffectivelyattainedwhenhistoricalinformationreflectsthecurrentmarketprocessofsecuritiesand insiderprivilegeinformationispubliclyavailable.Risks developnotjustasaresultofchangesinmarketfactors, butalsoasaresultofactionstakenbymarketplayerswho arewillingtotakerisks(i.e,riskseekers),transferrisks(i.e, riskaverters)orareriskindifferent(i.e,riskneutrals).To havepreciselyrecordedamountsandparameters,market riskrequiressufficientmanagementandanalyticalsystemstoanalyzemajorriskfactorsandtoutilizecommonly
acceptedfinancialconceptsandproceduresforriskmeasurement.
Recommendations
Facedwithcontinuouslychangingmarketrudimentsand increasingregulations,financialinstitutions(i.e,bankand non-bank)needtoregularlyreviewandoptimizetheir models,processesandsystemsformeasuringandmanagingrisks.Thiswillbefollowedbyaplannedandcosteffectiveuseofresourcestoreduce,monitor,andmanage thelikelihoodorimpactofunanticipatedoccurrences,or tooptimizetherealizationofpossibilities,sothatrisk uncertaintydoesnotdetractfromtheendeavor’scommercialobjectives.Variousfinancialorganizationsshouldhave suitableprocessesinplacethatreflecttheirmarketrisk exposure.
InagreementwithBanjo(2019),avoidingmarketrisks andotheruncertaintieswithnegativeeffectsisacommon strategyformanagingmarketrisksandotheruncertainties withnegativerepercussions(i.e,riskavoidance);reducingthenegativeeffect/probabilityoftherisks(i.e,pre-loss andpost-lossriskminimisation);transmittingallorpart ofthedangertoathirdparty(insurance)(i.e.risktransfermechanism);andevenkeepingsomeoralloftherisks’ potential/actualrepercussions(i.e,riskretention).
Therefore,choiceofamodeldependsonthepositions forwhichthemodelistobeused;andtheuser’sriskmanagementcapacitiesandtechnicalpossibilities.
REFERENCES
Alexander,C.(2001). MarketModels: AGuidetoFinancialDataAnalysis. JohnWiley&Sons,Chichester.ISBN9780471899754.
Almgren,R.,andChriss,N.(2000).Optimalexecutionofportfoliotransactions. JournalofRisk3(Winter),5–39.
Ama,G.N.(2009).CorporateRiskReportingPracticesandtheirDeterminants:AstudyofSelectedQuotedFirmsinNigeria.Nigeria ResearchJournalofAccountancy(NRJA),1,81–96.
Anghelache,C.,Voineagu,V.,Culetu,D.,andBaltac,A.G.(2013).Methods,TheoriesandModelstoMeasureMarketRiskofthePortfolioof Shares. RomanianStatisticalReviewnr.8.
Asika,N.(2004). ResearchMethodology:AProcessApproach.Mukugamu &BrothersEnterprises,Lagos.
Banjo,A.K.(2019). InsuranceFundamentals (4thEd.).Lagos,Nigeria:DekinbanVenturesLtd.ISBN:987-32959-5-0.
Berkowitz,J.(2001).Testingdensityforecasts,withapplicationstorisk management. JournalofBusinessandEconomicStatistics 19,465–474.
Blaauw,A.J.(2009). MarketRiskManagementinNigeria.Unitedbankfor Africa(UBA)Plc.Accessedfrom www.ubagroup.com on01/02/ 2022.10:00GMT.
Danu,M.(2014).DimensionofMarketRisk.StudiesandScientific Researches,EconomicsEdition,issue19.Accessedfrom https:// EconPapers.repec.org/article on20/02/202201:06GMT.
Dowd,K.(2002). AnIntroductiontoMarketRiskManagement.JohnWiley &SonsLtd,Chichester.
Elton,E.J.,andGruber,M.J.(1995). ModernPortfolioTheoryandInvestment Analysis.FifthEdition.NewYork:JohnWileyandSons,Chichester. Emilia,M.(2010).ModelsforAnalysisandAssessmentofMarketRisks inBanking.UniversityofNationalEconomy,Bulgaria. FactaUniversitatisEconomicJournal,Vol.7.No.4.
Enyi,P.,andAdebawo,O.(2014).ImpactofRiskExposuresontheMarket ValueofNigerianBanks(2006-2012). AmericaInternationalJournalof ContemporaryResearch,Vol.4.No.10. Hubbard,D.(2009). TheFailureofRiskManagement. JohnWilley&and sonsp.46. Jamshidian,F.,andZhu,Y.(1997). ScenarioSimulation:Theoryand Methodology.FinanceandStochastics1:43–67. Kallestrap,R.(2012). TheDynamicsofBankandSovereignCreditRisk.PhD ThesisattheCopenhagenBusinessSchoolandtheCentralBankof Denmark.RetrievedfromNationalBank en.dk/c1256BE9...strup_p hd_Thesis_2012.pdf 9/12/21,10:10GMT. Kato,T.,andYoshiba,T.(2000).ModelRiskanditsControl.BankofJapan InstituteforMonetaryandEconomicStudies: DiscussionPaperNo. 2000-E-15.
Langrin,R.B.,andRoach,K.(2009).MeasuringtheEffectsofConcentrationandRiskonBankReturns:Evidencefromapanelindividual loanportfolioinJamaica. Business,FinanceandEconomicsinEmerging Economies.4(1):74–119.
Mandelbrot,B.,andRichard,H.(2006). TheMisbehaviorofMarket:AFractalViewofFinancialTurbulence. Navarrete,E.(2006). PracticalCalculationofExpectedandUnexpectedLosses inOperationalRiskbySimulationMethods.ScalarConsulting1–10. Scaillet,O.(2000). Non-ParametricEstimationandSensitivityAnalysisof ExpectedShortfall. Mimeo.IRES,CatholicUniversityofLouvain. Zhang,Y.(2006). APortfolioTheoryofMarketRiskLoad.CausalityActuarial SocietyForum.