Outsourced Data Labeling Service:
What Makes it a Feasible Option?
Artificial Intelligence and Machine Learning are no longer the technologies of the coming age. They have become an indispensable part of our daily lives. Right from unlocking our phones via biometric identification, sending automated replies via emails/messages, getting traffic updates from GPS, or getting recommendations based on your choice of music, series, brands, etc. all of these are just marvelous applications of AI and ML.
Moreover, autonomous vehicles have traveled from a science fiction plot into an unbelievable-yet-true thing of reality. Businesses across dozens of verticals ranging from manufacturing, healthcare, military, sports, smart city planners, renewable energy, automation, and so on leverage AI/ML-based models to interpret trends, identify patterns, solve problems and harness the true potential of their data in the best possible way. But what is the driving force behind all these AI/ML applications? It’s the data annotation process.
Understanding Data Annotation in Machine Learning
Machines certainly do not have ‘brains’ of their own and cannot interpret things as humans do. At the same time, feeding these computers with raw data wouldn’t make any sense. They need properly tagged and labeled datasets to acquire intelligence and perform the desired actions. So, this is what data annotation does helps machines to make sense of the input data.
Simply put, data annotation is the process of adding tags, labels, descriptions, and meta tags to datasets, which can be in the form of text, images, audio, or videos. These tags and labels provide a context to the Machine Learning algorithms that help
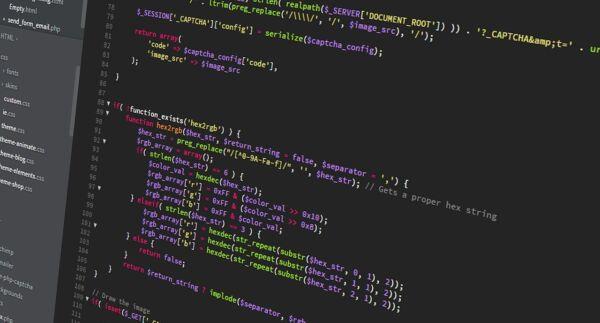
them easily comprehend their environment. The AI/ML-based models can identify different objects, calculate attributes, and perform other desired tasks.
Annotation in Machine Learning is, therefore, a critical step. Any errors in data labeling not only impact the algorithm’s performance but also cause AI-based models to make false decisions. In the worst-case scenario, AI/ML-based model investments can turn into business losses. So, how to go ahead with this?
In-House Data or Outsourcing Data Annotation Process: Which is the Best Option?
Constant access to relevant and accurately labeled data is an important prerequisite for Machine Learning algorithms before they go live. The more input data they are fed, the more accurate and reliable are their predictions. Hence, constant streams of well-tagged data for a long time are a challenge for many companies; adding to this, are the budget constraints as operational expenditures immediately shoot up when you hire skilled annotators and implement relevant tagging technology. As a result, businesses need to make a choice whether to create an in-house team or opt for established AI data labeling companies. Here you go:
• Process Management
In-House: Handling an in-house data labeling team, whether it is big or small, is a daunting task as it involves a tiring recruitment and training process. A huge amount of time and other resources are spent on monitoring and managing these employees. In addition, troubleshooting the tool and ensuring quality (in terms of accuracy and relevance) in training datasets might be burdensome for data professionals and annotators. As a result, they get distracted from the core activity, which is adding unbiased information to the input datasets.
Outsourcing: You can either consult a professional annotator or avail of data annotation services to get enhanced quality training datasets. They take care of the entire tagging process and focus on creating precise data labels, making sure that your AI/ML model is trained properly. These experts can also look after troubleshooting data labeling tools, detect problems, and get them fixed in real-time to avoid any blunders.
• Versatility
In-House: As annotation in the Machine Learning process is gradually gaining importance and becoming a top priority for many organizations, there’s a shortage of skilled annotators in the market. And even if you have an in-house data annotation team, it is intended to fulfill a specific requirement. But what if the demand for labeled data fluctuates over time?
Suppose, you’ve got a requirement where more text needs to be annotated in the next month. To fulfill this, you either end up overloading your in-house team with work at times or underworking at others. Such inefficiencies negatively impact the bottom line as the demand for datasets changes and organizations expand their business.
Outsourcing: Professional data annotation companies can easily handle spikes in the data volumes as they work day in and out on such tasks. They can easily sync with the changing needs of your AI/ML models; hence, you get the best possible data training sets within the stipulated time and budget. Isn’t this a smart way to eliminate inefficiencies and make room for resource optimization?
• Staff Training and Skill Development
In-house: Getting an in-house data annotation department includes lots of staff training along with adequate technology implementation. Experienced annotators and data professionals need to teach the untrained ones about the project goals, business objectives, and particular obligations of the specific projects, eliminating individual biases, other than training them on how to operate annotation tools. This not only affects the allocation of resources but also hampers the overall business productivity which may have profitably been used for tasks with higher importance.
Outsourcing: Established data annotation companies already have a pool of data professionals, skilled annotators, and Subject Matter Experts (SMEs) who can swiftly adapt to fluctuating demands for training datasets. Having the right blend of skills and experience ensures excellence in every data labeling endeavor.
• Operational Costs
In-house: Especially for startups as well as small-and-medium-sized businesses or enterprises with strict budget constraints, getting a data annotation team in-house might be a costly option managing and building the infrastructure required to train Machine Learning algorithms involves significant costs in terms of acquiring an office space, expenses of hiring employees, and buying the annotation tools.
Outsourcing: When you collaborate with a data labeling service provider, you only have to pay for the genuine services availed. From manually tagging data samples to leveraging AI data annotation tools to training machines, they offer reasonable pricing for all your needs. This way, you not only cut down on operational costs but do not compromise on accuracy or quality either.
Click here on Published Blog:
https://www.datasciencesociety.net/outsourced-data-labelingservice-what-makes-it-a-feasible-option/