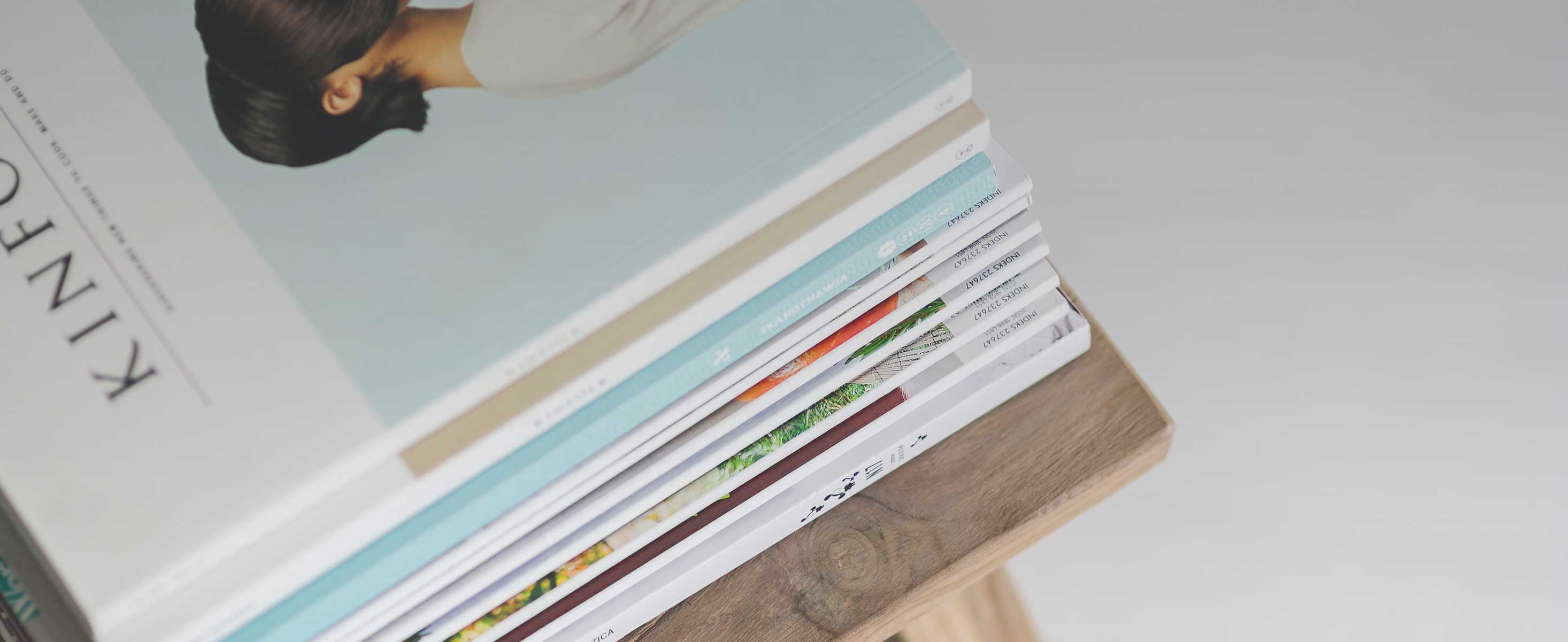
4 minute read
PMT, Machine Learning, and Big Data: What Do We Know?
How to Harness the Power of Data and Inference | 411
nuanced estimates of climatic impacts on PMT scores. The advantages of this approach are that data on aggregate shocks are often readily available and the estimation methods are the same as those used in the PMT. The disadvantage is that the use of aggregate information on covariate shocks is, essentially, a form of geographic targeting but the same impact is imputed to each household. Microclimates, geography, soil conditions, as well as farm practices may expose to drought or flooding only a portion of households in the same aggregate climatic conditions, yet all households in that location will be modeled as affected.
Leite (2015) simulates the impact of shocks in Kenya using the PMTplus approach, showing that small, shock-related adjustments to the PMT to reduce inclusion errors in times of shocks are possible. Simply applying the estimated impact of the shock on welfare to correct the cutoff point for the PMT and using an indicator of food insecurity, such as the World Food Programme’s Food Consumption Score, makes it possible to identify households that are vulnerable to poverty. Leite (2015) suggests that the best way to increase the precision of the selection would be to examine the most up-to-date geographic data to identify the shock-affected areas (geographic targeting) and then carry out a quick data collection exercise to gather food insecurity indicators to improve the precision of the model.
PMTplus has been applied to several African countries to shed light on vulnerability rates and sources within a traditional PMT model. Skoufias et al. (2019b) explore some of Hill and Porter’s (2017) ideas and use a hierarchical model based on Günther and Harttgen (2009); the analytic framework adopted is complementary to del Ninno and Mills‘ (2015) PMTplus approach as well as the Listahanan PMT.63 Skoufias et al. aim to predict households’ ex ante vulnerability to typhoons in the Philippines (Skoufias et al. 2019a) and variations in rainfall and the incidence of drought in Ethiopia, Niger, Nigeria, Tanzania, and Uganda (Skoufias et al. 2019b). They find that the exposure of a barangay (village) in the Philippines to a typhoon in the six months before the month of the interview is associated with a statistically significant decline in total per capita expenditures and specifically food and protein per capita expenditures. The estimated coefficients from the regression model were also used to estimate ex ante household vulnerability to poverty (the likelihood of household consumption falling below the poverty line) in the event of future natural disasters of different intensities.
PMT, Machine Learning, and Big Data: What Do We Know?
With more computational power, there is a new branch of research to investigate the promise of using more sophisticated algorithms to improve PMTs.
412 | Revisiting Targeting in Social Assistance
The traditional PMT models mentioned above historically used parametric regressions (OLS, logit, and quantile regressions). Machine learning algorithms use both parametric and nonparametric models that are more computationally intense (for example, nonlinear models and tree-based models) to score household welfare based on various observable household and location variables. The main traditional PMT models were briefly summarized in the section of this chapter on PMT; this section highlights the various new machine learning models along with what is known about how effective they can be; chapter 8 provides a fuller and more technical presentation of machine learning results by Areias et al. (forthcoming).
In addition, the new trends in big data (for example, remote sensing satellite data and CDRs) offer new proxies on which PMT models can be built. Machine learning can be applied to traditional survey data. It can also be applied to various forms of new big data. Earlier, this chapter described how big data, such as remote sensing satellite data, social media metadata, and CDRs, can be used to make geographic targeting much more accessible and frequent. In addition to reviewing the new machine learning models, this section considers the use of big data in PMT models, as well as the aforementioned ancillary data, to select households rather than small area locations.
Improving PMT with Machine Learning Models
The main difference between the traditional regression models usually used in PMT and the new machine learning models is their approach to model variance and model bias. Model variance is the degree to which a model would change if it had been trained on different data, and it is hoped that the model estimates do not vary too much across different training sets since high variance leads to lower precision. Model bias is the error that comes from errors in the model specification that uses simple linear relationships when most of the relationships are not linear. Traditional regression-based PMT focuses more on finding unbiased models, which does not mean that they have the lowest errors for prediction as they still can have high variance. Machine learning approaches accept a trade-off by introducing some error due to model bias in exchange for reducing error due to model variance in the estimation, thereby focusing on out-of-sample accuracy rather than correct model specification. The models are successful if the decrease in the error due to reduced variance is significantly more than the increase in error due to introducing bias. Moreover, the fact that machine learning methods are concerned primarily and explicitly with controlling model variance has allowed them to use very high-dimensional data, such as CDRs and satellite images, as useful inputs for estimation problems, without risking model generalizability.