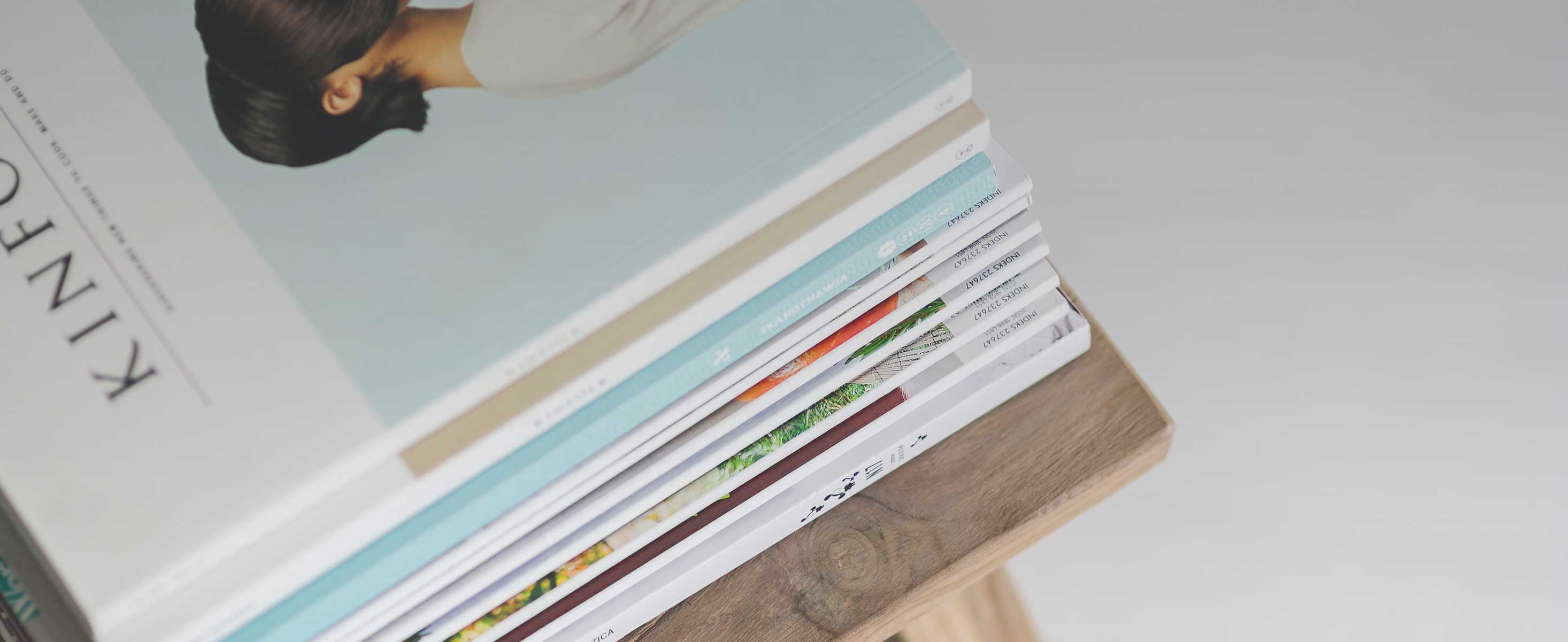
2 minute read
Data-Driven Decision-Making in the Human Development Sectors
Such an advance could help tailor education to each student and increase the efficiency of the educational system. The process is comparable to that of a “classroom” situation in which the teacher can respond to the noncognitive responses of the child (emotional, motivational, and so on) and adjust teaching accordingly.
However, there are multiple risks. The system reduces complex emotional states to a basic coding of facial movements and makes inferences from that about learning. Studies have found that efforts to read a person’s internal states from facial movements alone are at best incomplete or at worst may entirely lack validity. The scientific validity of the Facial Action Coding System being used by education technology companies in affective computing is also being questioned.
On the pedagogical side, there is the question of what the children are being trained for and what effect this has on overall child development. The constant monitoring may have a chilling effect on children when they realize they are being “scored” by machines, and it may affect peer relations and reinforce biases and prejudices. Furthermore, it is not clear that AI-enabled applications are better at developing creativity and resilience compared with peer-to-peer interaction and human mentorship.
In addition, there are issues about how the extensive data collected about students and their families, including payment history, are stored and used by the provider of the service. Questions such as who owns the data and whether it can be used for commercial purposes by the company or sold to others, including for surveillance purposes, are paramount. Companies expand their facial biometrics collection through the provision of such services, which reinforces their position in the market and contributes to their “legitimacy.”11 There is also the issue of potential explicit or implicit biases in the algorithms and whether they will unfairly typecast a student based on incomplete or biased assessments.
Health, education, and social protection pose great challenges for data collection through traditional means and even more so for data analysis and use in decision-making. These challenges stem from the large geographical spread of service locations, poorly paid and relatively unskilled frontline providers, and weak monitoring. Ubiquitous and faster digital connectivity, coupled with data collected “automatically” from devices and sensors on human beings and inanimate objects, has transformed this situation. These trends, combined with satellite data and geospatial information becoming increasingly available, are enhancing the scope for data and evidence to support real-time decision-making and monitoring and evaluation of development interventions in the social sectors.
Applications range from the individual organization level (such as hospitals or universities) to entire government departments. For example, analysis of student performance data can enable university faculty to identify students at risk of dropping