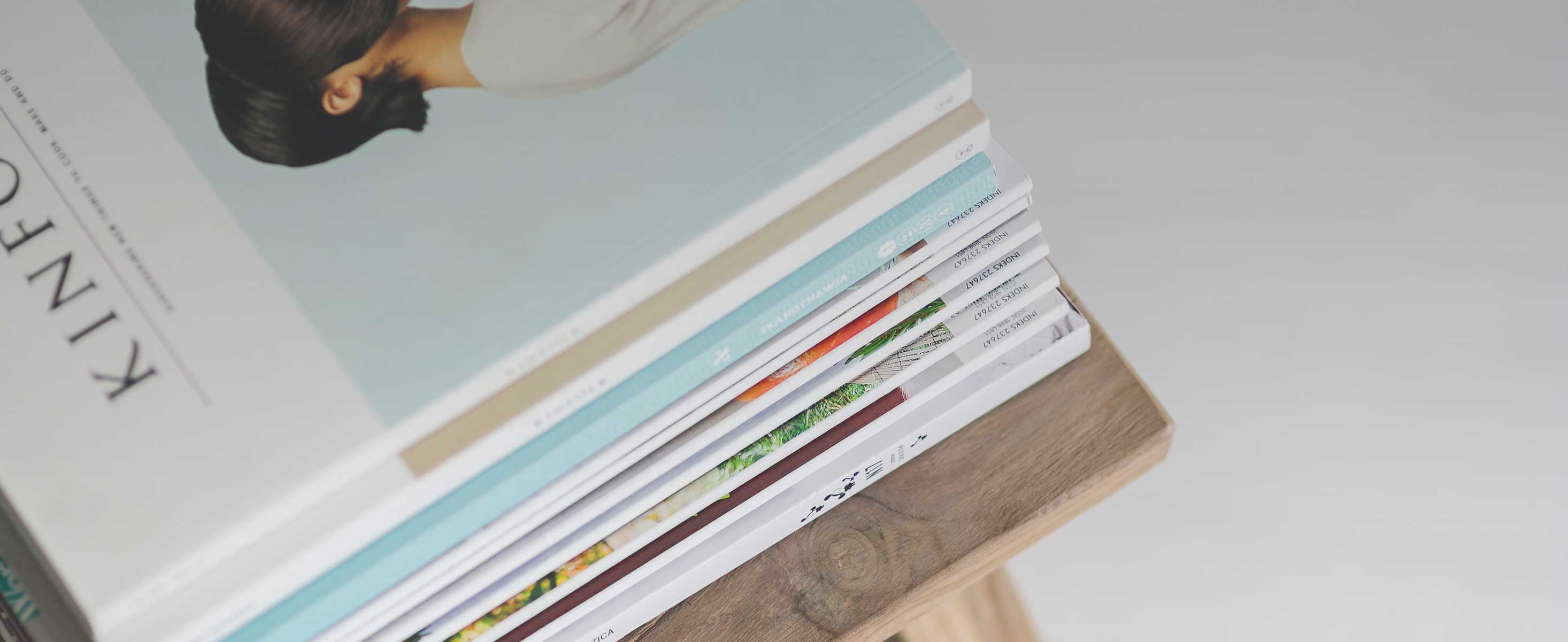
16 minute read
4.2 Determining data ownership: A case study from the Demand for Safe Spaces project
Data ownership is the assignment of rights and privileges over data sets, including control over who may access, possess, copy, use, distribute, or publish data or products created from the data. For more details, see the DIME Wiki at https://dimewiki.worldbank .org/Data_Ownership.
Derivatives of data are new data points, new data sets, or outputs such as indicators, aggregates, visualizations, and other research products created from the original data. Determining data ownership
Before acquiring any data, it is critical to establish data ownership. Data ownership can sometimes be challenging to establish, because different jurisdictions have different laws regarding data and information, and the research team may have its own regulations. In some jurisdictions, data are implicitly owned by the people to whom the information pertains. In others, data are owned by the people who collect the information. In still others, ownership is highly unclear, and there are varying norms. The best approach is always to consult with a local partner and to enter into specific legal agreements establishing ownership, access, and publication rights. These agreements are particularly critical when confidential data are involved—that is, when people are disclosing information that could not be obtained simply by observation or through public records.
If the research team is requesting access to existing data, it must enter into data license agreements to access the data and publish research outputs based on the information. These agreements should make clear from the outset whether and how the research team can make the original data public or whether it can publish any portion or derivatives of the data. If the data are publicly accessible, these agreements may be as simple as agreeing to terms of use on the website from which the data can be downloaded. If the data are original and not yet publicly accessible, the process is typically more complex and requires a documented legal agreement or memorandum of understanding.
If the research team is generating data directly, such as survey data, it is important to clarify up front who owns the data and who will have access to the information (see box 4.2 for an example of how data ownership considerations may vary within a project). These details need to be shared with respondents when they are offered the opportunity to consent to participate in the study. If the research team is not collecting the data directly—for example, if a government, private company, or research partner is collecting the data—an explicit agreement is needed establishing who owns the resulting data.
BOX 4.2 DETERMINING DATA OWNERSHIP: A CASE STUDY FROM THE DEMAND FOR SAFE SPACES PROJECT
The Demand for Safe Spaces study used three data sources, all of which had different data ownership considerations.
1. Crowdsourced ride data from the mobile app. The research team acquired crowdsourced data through a contract with the technology firm responsible for developing and deploying the application. The terms of the contract specified that all intellectual property in derivative works developed using the data set are the property of the World Bank.
(Box continues on next page)
BOX 4.2 DETERMINING DATA OWNERSHIP: A CASE STUDY FROM THE DEMAND FOR SAFE SPACES PROJECT (continued)
2. Platform survey and implicit association test data. A small team of consultants collected original data using a survey instrument developed by the research team. The contract specified that the data collected by the consultants and all derivative works are the sole intellectual property of the World Bank. 3. Crime data. The team also used one variable (indicating crime rate at the Supervia stations) from publicly accessible data produced by Rio’s Public Security Institute. The data are published under
Brazil’s Access to Information Law and are available for download from the institute’s website.
An institutional review board (IRB) is an institutional body formally responsible for ensuring that research under its oversight meets minimum ethical standards. For more details, see the DIME Wiki at https://dimewiki.worldbank .org/IRB_Approval.
Data licensing is the process of formally granting rights and privileges over data sets to people who are not the owner of the data. For more details, see the DIME Wiki at https://dimewiki.worldbank .org/Data_License_Agreement.
The contract for data collection should include specific terms as to the rights and responsibilities of each stakeholder. It must clearly stipulate which party owns the data produced and that the research team maintains full intellectual property rights. The contract should also explicitly indicate that the contracted firm is responsible for protecting the privacy of respondents, that the data collection will not be delegated to any third parties, and that the data will not be used by the firm or subcontractors for any purpose not expressly stated in the contract, before, during, or after the assignment. The contract should also stipulate that the vendor is required to comply with ethical standards for social science research and to adhere to the specific terms of agreement with the relevant institutional review board (IRB) or applicable local authority. Finally, it should include policies on the reuse, storage, and retention or destruction of data.
Research teams that acquire original data must also consider data ownership downstream, through the terms they use to release those data to other researchers or to the general public. The team should consider whether it can publish the data in full after removing personal identifiers. For example, the team must consider whether it would be acceptable for the data to be copied and stored on servers anywhere in the world, whether it would be preferable to manage permissions on a case-by-case basis, and whether data users would be expected to cite or credit them. Similarly, the team can require users to release the derivative data sets or publications under similar licenses or offer use without restriction. Simple license templates are available for offering many of these permissions, but, at the planning stage, all licensing agreements, data collection contracts, and informed consent processes used to acquire the data need to detail those future uses specifically.
Obtaining data licenses
Data licensing is the formal act of the owner giving some rights to a specific user, while retaining ownership of the data set. If the team does not own the data set to be analyzed, it must enter into a licensing agreement to access the data for research purposes. Similarly, if the team
does own a data set, it must consider whether the data set will be made accessible to other researchers and what terms of use will be required.
If the research team requires access to existing data for novel research, it is necessary to agree on the terms of use with the data owner, typically through a data license agreement. These terms should specify what data elements will be received, the purposes for which the data will be used, and who will have access to the data. The data owner is unlikely to be highly familiar with the research process and may be surprised at some of the uses to which the data could be put. It is essential to be forthcoming about the uses up front. Researchers typically want to hold intellectual property rights to all research outputs developed with the data and a license for all uses of derivative works, including public distribution (unless ethical considerations contraindicate this right). Holding these rights allows the research team to store, catalog, and publish, in whole or in part, either the original licensed data set or data sets derived from the original. It is important to ensure that the license obtained from the data owner allows these uses and that the owner is consulted if exceptions for specific portions of the data are foreseen.
The Development Impact Evaluation (DIME) department follows the World Bank’s template data license agreement. The template specifies the specific objectives of the data sharing and whether the data can be used for the established purpose only or for other objectives as well. It classifies data into one of four access categories, depending on who can access the data by default and whether case-by-case authorization for access is needed. The data provider may impose similar restrictions on sharing derivative data and any or all of the associated metadata. The template also specifies the required citation for the data. Although it is not necessary to use the World Bank’s template or its access categories if the team is not working on a World Bank project, the information in the template is useful in two ways. First, it is necessary to base the data license agreement on a template. Ad hoc agreements can leave many legal ambiguities or gaps where the permissions given to the research team are unclear or incomplete. Second, it is strongly recommended that the data be categorized using some variation of this system. Doing so will create different standard procedures for each category, so that the intended processes for handling the data are clear.
Documenting data received from partners
Research teams granted access to existing data may receive those data in several ways: access to an existing server, physical access to extract certain information, or a one-time data transfer. In all cases, action is required to ensure that data are transferred through secure channels so that confidentiality is not compromised. The section on handling data securely explains how to do that. Compliance with ethical research standards may in some cases require a stricter level of security than initially proposed by the partner agency. It is also critical to request any and all available documentation for the data; this documentation could take the form of a data
Statistical disclosure risk is the likelihood of revealing information that can be used to associate data points with individual research participants, especially through indirect identifiers. For more details, see the DIME Wiki at https:// dimewiki.worldbank.org /De-identification. dictionary or codebook, a manual for the administrative data collection system, detailed reports or operating procedures, or another format. If no written documentation is available, the person(s) responsible for managing the data should be interviewed to learn as much as possible about the data; the interview notes should be archived with data documentation.
At this stage, it is very important to assess the documentation and cataloging of data and associated metadata. It is not always clear what pieces of information will jointly constitute a research data set, and many data sets are not organized for research. The original data should be retained exactly as received, alongside a copy of the corresponding ownership agreement or license. A simple README document is needed, noting the date of receipt, the source and recipient of the data, and a brief description of each file received. All too often data are provided in vaguely named spreadsheets or digital files with nonspecific titles. Documentation is critical for future access and reproducibility.
Eventually, a set of documents will be created that can be submitted to a data catalog and given a reference and citation. Metadata— documentation about the data—are critical for future use of the data. Metadata should include documentation of how the data were created, what they measure, and how they are to be used. For survey data, this documentation should include the survey instrument and associated manuals; the sampling protocols and field adherence to those protocols and any sampling weights; what variable(s) uniquely identifies the data set(s) and how different data sets can be linked; and a description of field procedures and quality controls. DIME uses the Data Documentation Initiative (DDI), which is supported by the World Bank’s Microdata
Catalog (https://microdata.worldbank.org).
As soon as the desired pieces of information are stored together, it is time to think about which ones are the components of what will be called a data set. Often, when receiving data from a partner, even highly structured materials such as registers or records are not, as received, equivalent to a research data set; they require initial cleaning, restructuring, or recombination to be considered an original research data set. This process is as much an art as a science: it is important to keep information together that is best contextualized together, but information also needs to be as granular as possible, particularly when there are varying units of observation. There is often no single correct way to structure a data set, and the research team will need to decide how to organize the materials received. Soon, research data sets will be built from this set of information and become the original clean data, which will be the material published, released, and cited as the starting point of the data. (If funders or publishers request that “raw” data be published or cataloged, for example, they should receive this data set, unless they specifically require data in the original format received.) These first data sets created from the received materials need to be cataloged, licensed, and prepared for release. This is a good time to begin assessing the disclosure risk and to seek publication licenses in collaboration with data providers, while still in close contact with them.
This section details specific considerations for acquiring high-quality data through electronic surveys of study subjects. If the project will not use any survey data, skip this section. Many excellent resources address how to design questionnaires and field supervision, but few cover the particular challenges and opportunities presented by electronic surveys. Many survey software options are available to researchers, and the market is evolving rapidly. Therefore, this section focuses on specific workflow considerations for digitally collected data and on basic concepts rather than on software-specific tools.
Electronic data collection technologies have greatly accelerated the ability to collect high-quality data using purpose-built survey instruments and therefore have improved the precision of research. At the same time, electronic surveys create new pitfalls to avoid. Programming electronic surveys efficiently requires a very different mind-set than writing paperbased surveys; careful preparation can improve the efficiency and data quality of surveys. This section outlines the major steps and technical considerations to follow when fielding a custom survey instrument, no matter the scale.
Questionnaire design is the process of creating a survey instrument, typically for data collection with human subjects. For details and best practices, see the DIME Wiki at https:// dimewiki.worldbank.org /Questionnaire_Design.
Computer-assisted personal interviews (CAPIs) are interviews that use a survey instrument programmed on a tablet, computer, or mobile phone using specialized survey software. For more details, see the DIME Wiki at https://dimewiki.worldbank .org/Computer-Assisted _Personal_Interviews_(CAPI). For CAPI questionnaire programming resources, see the DIME Wiki at https:// dimewiki.worldbank.org /Questionnaire_Programming. Designing survey instruments
A well-designed questionnaire results from careful planning, consideration of analysis and indicators, close review of existing questionnaires, survey pilots, and research team and stakeholder review. Many excellent resources discuss questionnaire design, such as that of the World Bank’s Living Standards Measurement Survey (Glewwe and Grosh 2000). This section focuses on the design of electronic field surveys, often referred to as computer-assisted personal interviews (CAPIs). Although most surveys are now collected electronically, by tablet, mobile phone, or web browser, questionnaire design (content development) and questionnaire programming (functionality development) should be seen as two strictly separate tasks. Therefore, the research team should agree on the content of all questionnaires and design a version of the survey on paper before beginning to program the electronic version. Doing so facilitates a focus on content during the design process and ensures that teams have a readable, printable version of the questionnaire. Most important, it means that the research, not the technology, drives the questionnaire’s design.
This approach is recommended for three reasons. First, an easyto-read paper questionnaire is very useful for training data collection staff, which is discussed further in the section on training enumerators. Second, finalizing the paper version of the questionnaire before beginning any programming avoids version-control concerns that arise from concurrent work on paper and electronic survey instruments. Third, a readable paper questionnaire is a necessary component of data
A theory of change is a theoretical structure for conceptualizing how interventions or changes in environment might affect behavior or outcomes, including intermediate concepts, impacts, and processes. For more details, see the DIME Wiki at https:// dimewiki.worldbank.org /Theory_of_Change.
Research design is the process of planning a scientific study so that data can be generated, collected, and used to estimate specific parameters accurately in the population of interest. For more details, see the DIME Wiki at https:// dimewiki.worldbank.org /Experimental_Methods and https://dimewiki .worldbank.org/Quasi -Experimental_Methods.
A preanalysis plan is a document containing extensive details about a study’s analytical approach, which is archived or published using a third-party repository in advance of data acquisition. For more details, see the DIME Wiki at https:// dimewiki.worldbank.org /Preanalysis_Plan.
A survey pilot is intended to test the theoretical and practical performance of an intended data collection instrument. For more details on how to plan, prepare for, and implement a survey pilot, see the DIME Wiki at https:// dimewiki.worldbank.org /Survey_Pilot. documentation, because it is difficult to work backward from the survey program to the intended concepts.
The workflow for designing a questionnaire is much like writing an essay: it begins from broad concepts and slowly fleshes out the specifics. It is essential to start with a clear understanding of the theory of change and research design of the project. The first step of questionnaire design is to list key outcomes of interest, the main covariates to control for, and any variables needed for the specific research design. The ideal starting point for this process is a preanalysis plan.
The list of key outcomes is used to create an outline of questionnaire modules. The modules are not numbered; instead, a short prefix is used, because numbers quickly become outdated when modules are reordered. For each module, it is necessary to determine if the module is applicable to the full sample or only to specific respondents and whether or how often the module should be repeated. For example, a module on maternal health applies only to households with a woman who has children, a household income module should be answered by the person responsible for household finances, and a module on agricultural production might be repeated for each crop cultivated by the household. Each module should then be expanded into specific indicators to observe in the field. To the greatest extent possible, using questions from reputable survey instruments that have already been fielded is recommended rather than creating questions from scratch (for links to recommended questionnaire libraries,
see the DIME Wiki at https://dimewiki.worldbank.org/Literature_Review _for_Questionnaire). Questionnaires for impact evaluation must also include ways to document the reasons for attrition and treatment contamination. These data components are essential for completing CONSORT records, a standardized system for reporting enrollment, intervention allocation, follow-up, and data analysis through the phases of a randomized trial (Begg et al. 1996).
Piloting survey instruments
A survey pilot is critical to finalize survey design. The pilot must be done out-of-sample, but in a context as similar as possible to the study sample. The survey pilot includes three steps: a prepilot, a content-focused pilot, and a data-focused pilot (see box 4.3 for a description of the pilots for the Demand for Safe Spaces project).
The first step is a prepilot. The prepilot is a qualitative exercise, done early in the questionnaire design process. The objective is to answer broad questions about how to measure key outcome variables and gather qualitative information relevant to any of the planned survey modules. A prepilot is particularly important when designing new survey instruments.
The second step is a content-focused pilot. The objectives at this stage are to improve the structure and length of the questionnaire, refine the phrasing and translation of specific questions, check for potential sensitivities and enumerator-respondent interactions, and