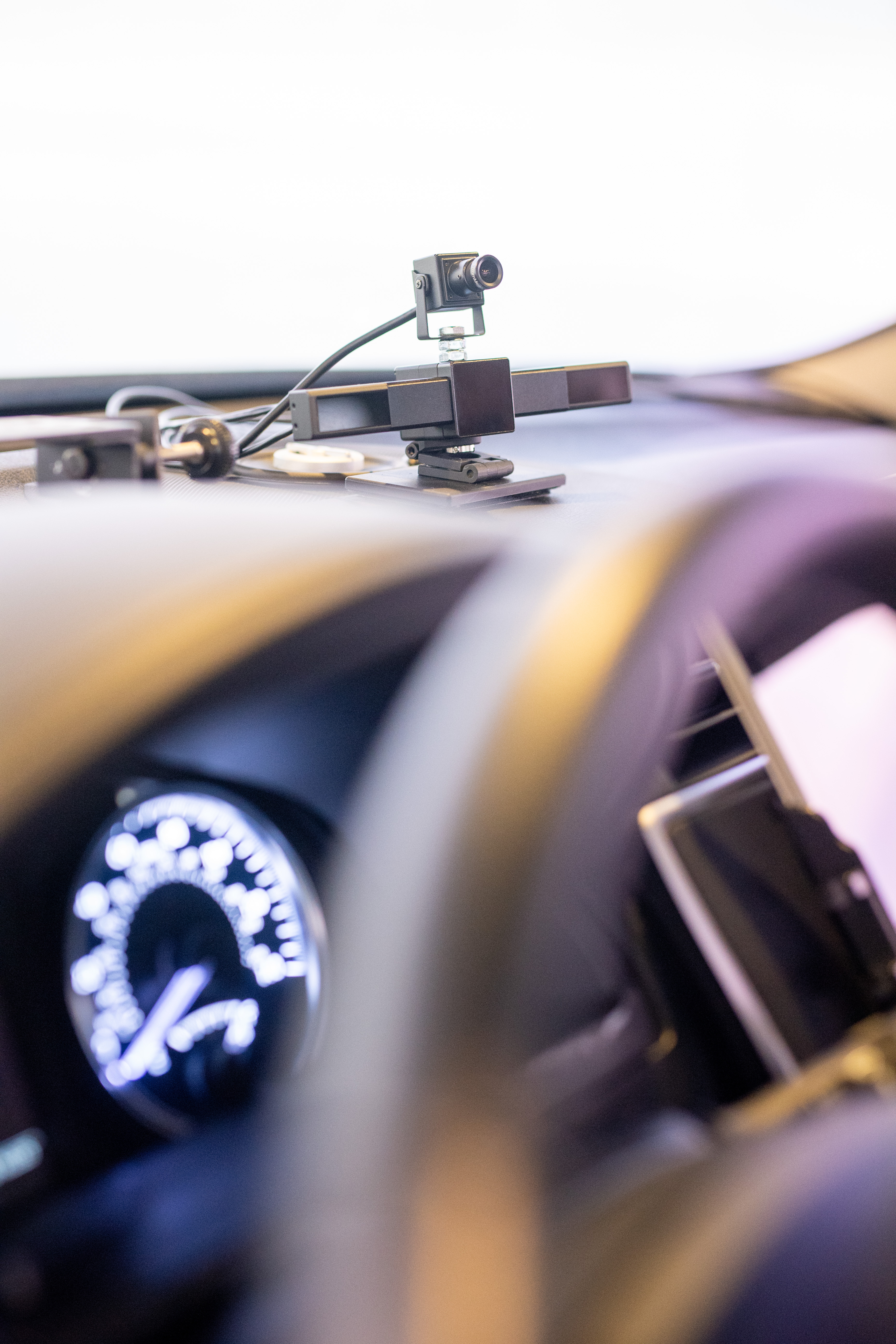
6 minute read
Assessing Driver Monitoring Systems
ASSESSING DRIVER MONITORING SYSTEMS
A new generation of driver monitoring systems (DMS) are being developed to identify when drivers are drowsy, distracted, and/or under the influence of alcohol or cannabis. DSRI researchers have been busy assessing these systems across multiple projects.
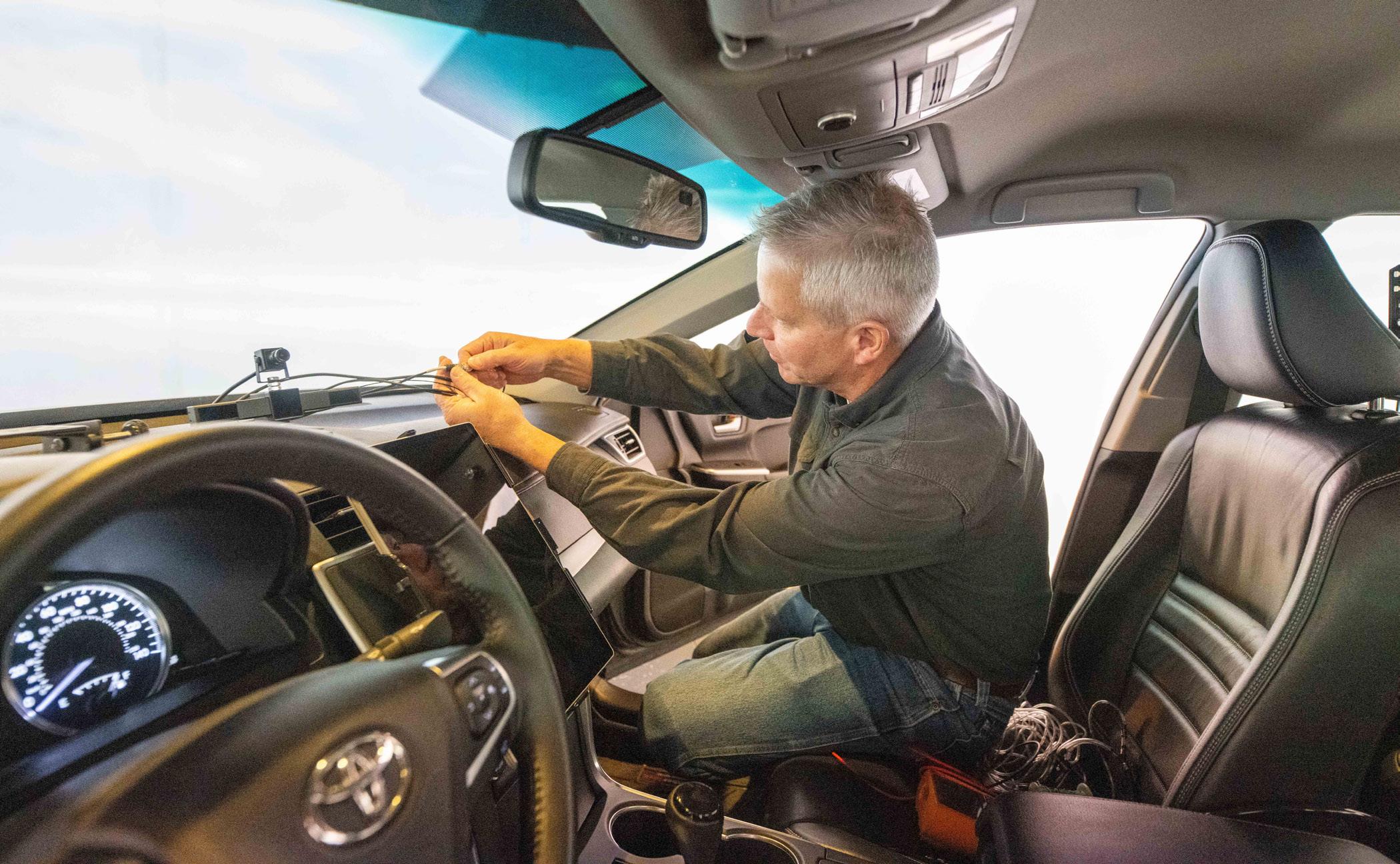
Alcohol DMS
Sponsored by the National Highway Traffic Safety Administration (NHTSA)
Researchers are analyzing the effectiveness of driver monitoring systems on their ability to detect if someone is driving while drunk—specifically, if they are over the legal limit or not.
“It’s important to differentiate between drivers who are drunk and drivers who are drowsy or otherwise impaired,” explained Tim Brown, director of drugged driving research at DSRI, “and figuring out which measures are most sensitive in differentiating the type of impairment.”
The study will have participants drive in a driving simulator at four distinct times: 1) when they are alert and sober, 2) when they are drowsy and sober, 3) when their BAC is at 0.08, and 4) again when their BAC is at 0.12. Investigators will collect data on driver performance, eye tracking, head and body movements, heart rate, and respiration, among others. The pilot data collection started in November of 2024.
Distraction and Drowsiness DMS
Sponsored by NHTSA, with partner Westat
The study aims to use DMS to detect if a driver is distracted and identify when this occurs alongside drowsiness. This research will help differentiate between distraction and drowsiness, enabling researchers to understand the effects of distraction with and without the presence of drowsiness. Pilot data collection was in late 2024 in the NADS-1, with primary data collection to occur in 2025.
Drowsiness DMS with Exponent
Sponsored by NHTSA, with partner Exponent
The team is assisting NHTSA by conducting research to provide recommendations for test procedures to evaluate DMS systems that are designed to detect drowsiness. In collaboration with Exponent, they developed a protocol to gather data on drowsy drivers and are evaluating it through DSRI’s driving simulator and Exponent’s test track.
Seeing Machines DMS for cannabis
Sponsored by the Institute for Cannabis Research, with partners Seeing Machines, University of Colorado Anschutz Medical Campus, and Swinburne University
Researchers used a Seeing Machines DMS to study changes in eye behaviors after cannabis use. They looked at what measures allow us to identify who is acutely under the influence of cannabis and whether signals of acute use are consistent between occasional and frequent users.
Findings showed that there were changes in scanning patterns of cannabis users before and after the consumption of cannabis. Additionally, changes in average eye opening were found to decrease following use. Final manuscripts are being written as of December 2024.
Alcohol Detection with IIHS
Sponsored by the Insurance Institute for Highway Safety (IIHS), with partner Seeing Machines
What measures from a DMS are predictive of alcohol impairment? Researchers examined different eye-related measures (e.g., blink rate, pupil size) to assess: 1) if the driver recently used alcohol, and 2) if these measures are consistent across users relative to BAC levels.
Findings showed that median eye opening and percent of time spent focused on the forward roadway could be used as predictors of alcohol-impaired driving. The final report will be available in early 2025.
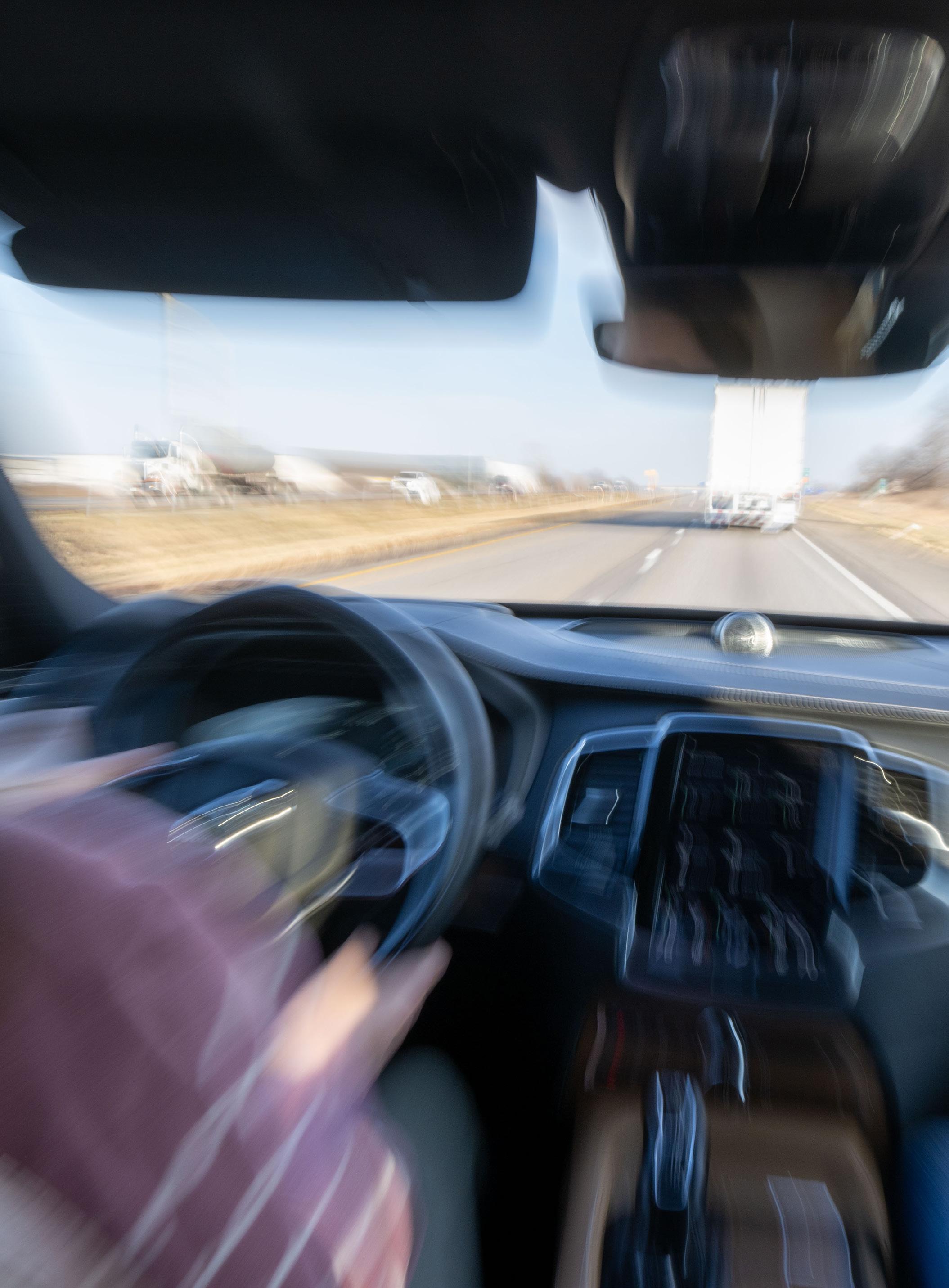
Aisin DMS
The DSRI team detected and analyzed impairment from alcohol and cannabis using a DMS provided by Aisin, a global automotive supplier. The Aisin DMS was installed on one of DSRI’s quarter cab miniSims, and the team used face and eye data from the DMS as well as vehicle-based measures for identifying impairment. The analysis was completed in September, with a final report and publication in progress, led by Chris Schwarz, director of engineering and modeling research.
The findings show that an alcohol model that used vehicle and DMS face features was effective at classifying alcohol impairment. A cannabis model that used mostly eye features also had good performance, but they suspect it to be a brittle result that will not generalize well to new data.
Overall, camera-based DMS devices hold promise for detecting different types of impairment that lead to lapses in attention and possible crashes. “The most promising path forward,” Schwarz said, “is to find ways to integrate DMS data with other types of signals from the vehicle and other sensors to provide the most holistic picture possible of driver state.”
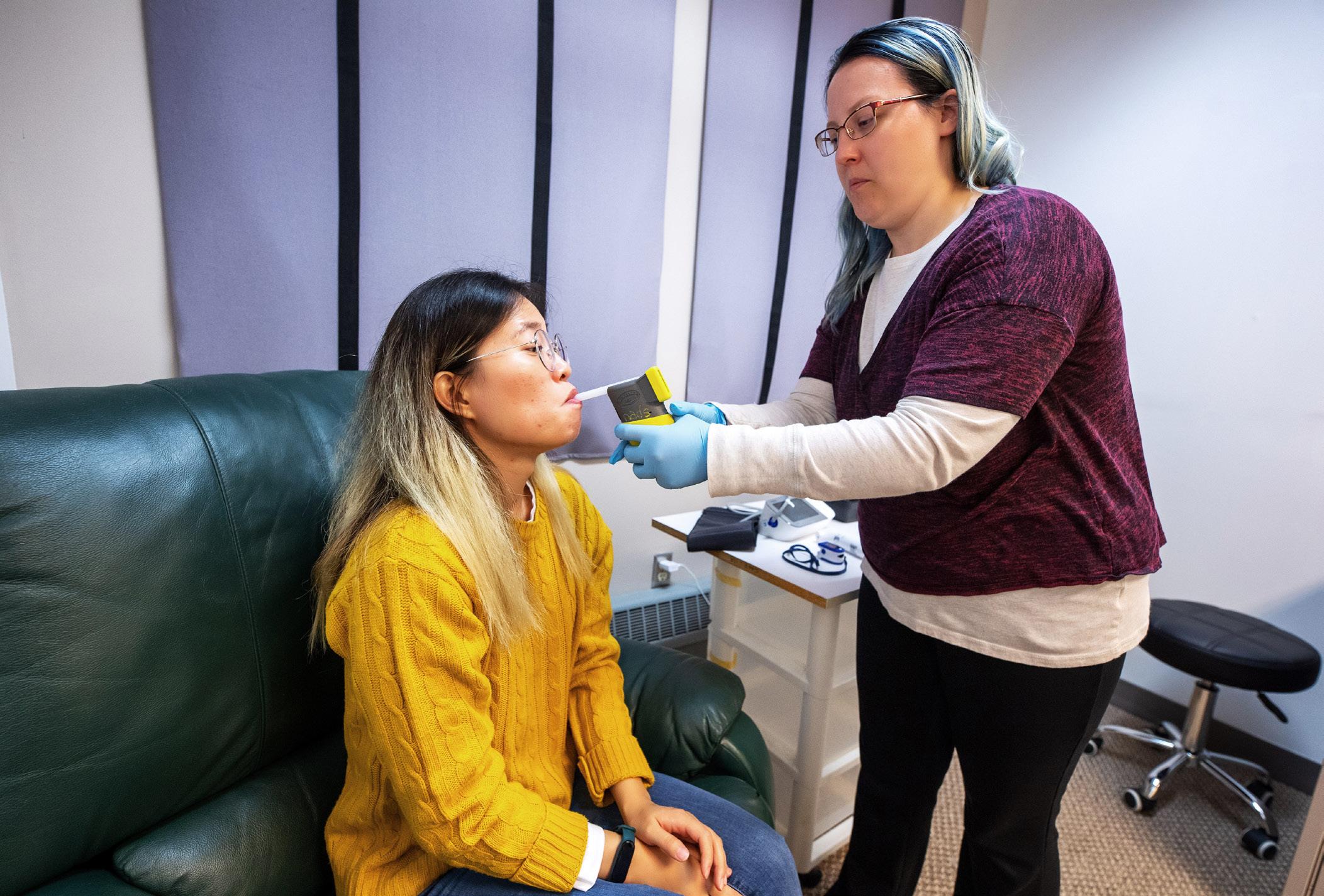
So which sensors are the most effective?
DSRI has also created a uniform data set from six past DMS studies that can be built upon and applied to future studies. In the analysis of these six studies, the team looked at which sensors were the most useful in detecting and differentiating types of impairment.
“Vehicle-based sensors become much less useful as soon as you turn on automation because at that point you stop getting any information about the driver,” explained Schwarz. “So these newer measures like gaze location become more valuable as a measurement tool.” Camera-based DMS are becoming more common in vehicles without the presence of automation; even a car being driven manually may have a camera-based DMS looking for signs of impairment.
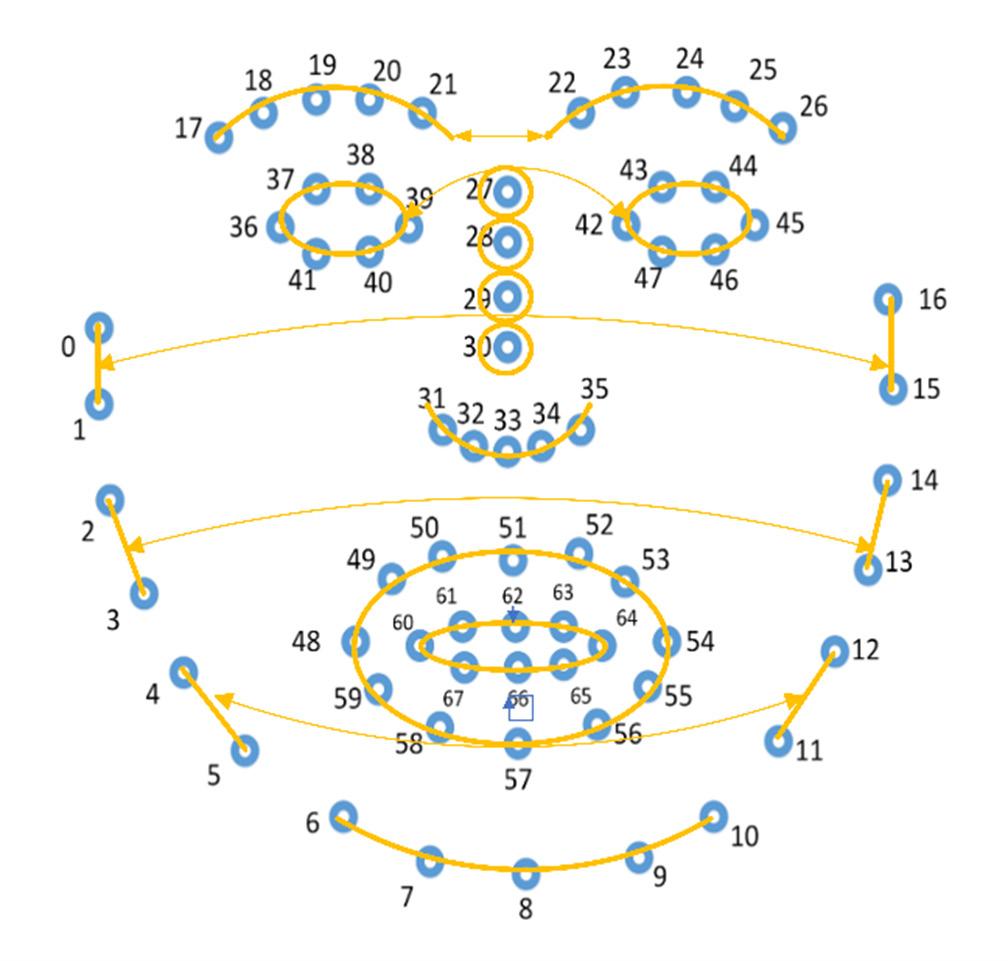
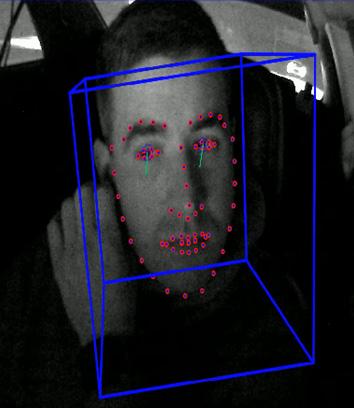
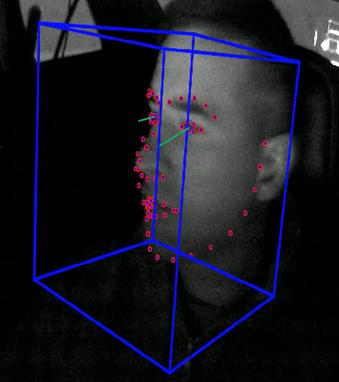
OTHER DRUGGED DRIVING STUDIES
Drunk Driving Telltale
Sponsored by NHTSA, with partner Westat
Researchers are doing a survey followed by two phases of testing with an in-person simulator drive where some participants will be dosed with alcohol and others will not. A telltale light will display, meant to indicate to the driver that they are too impaired to be driving. The study is meant to test understanding and interpretations of the initial icon set, then narrow to a final icon used for the telltale.