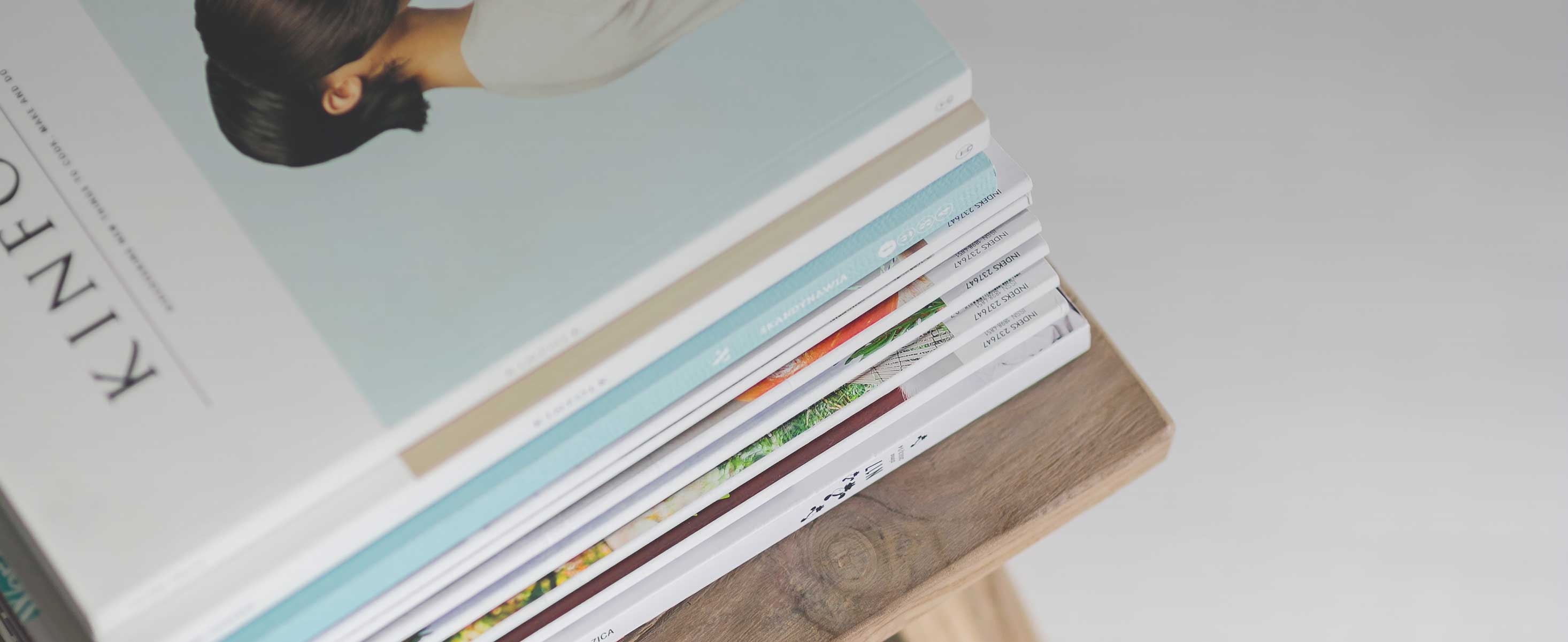
6 minute read
A New Computer-Based Tool for Managing Glaucoma Care
BY BRYAN C. STAGG, MD
Clinicians caring for patients with glaucoma must incorporate large amounts of longitudinal data from many sources accurately and efficiently to make critical decisions regarding patient care.1 Further complicating this decision-making process, clinicians must gather and synthesize this complex data amid busy clinics filled with competing distractions and pressures. The data are complex, with high test-retest variability and patient-specific variation.2
Advances in the field of predictive modeling and machine learning for glaucoma have the potential to help with physician information overload and improve patient care.3-4 However, for the results of these models to meaningfully improve clinical practice, the predictions need to be integrated into clinical workflow in a way that influences clinician and patient behavior.4-5 Optimized, workflow-appropriate clinical decision support (CDS) systems can help address this challenge.6
CDS Systems and Visual Field Testing
CDS systems are computer-based tools designed to improve clinician decision-making for individual patients at the point the decisions are made.7 However, not all CDS tools are effective.8 CDS systems are most effective when implemented within clinical workflow, developed using rigorous, user-centered iterative design principles, and integrated with the electronic health record using standards-based CDS technology that is scalable to other institutions.4-9
Optimal visual field testing frequency for an individual patient with glaucoma is a difficult clinical decision that could be dramatically improved with predictive modeling and effective CDS.3 Five to 30% of patients actively treated for glaucoma continue to have significant visual field loss and need additional eye pressure lowering.10-12 We periodically monitor visual function using visual field tests to identify patients who are being treated for glaucoma and continue to have progressive visual field loss to prevent blindness.
Visual field testing that isn’t frequent enough can lead to considerable delays in detecting glaucomatous progression and subsequent vision loss.13 For example, in many patients with a baseline mean deviation (MD) of -10 dB, annual visual field testing would take 12.7 years to detect glaucoma progression of -0.5 dB/year in 80% of eyes, at which point a patient could have lost 6.35 dB, resulting in significant disability.14
Determining the optimal testing frequency for an individual patient is complicated because visual field tests have high test-retest variability. The ability to detect progressive visual field loss depends on the test-retest variability (which varies between patients), the rate of visual field loss, and the number of tests performed over a given time.4
Figure 1 illustrates this with a hypothetical patient with rapid true visual field MD progression (-2 dB/year). In this case, because of test-retest variability, visual field progression is not detected until several years have passed. Annual testing would be insufficient for this patient. Testing that isn’t frequent enough risks unidentified vision loss, while testing that is too frequent is burdensome for patients and overuses resources.2
The frequency of visual field testing should vary based on patient characteristics.13 For example, to detect a MD change -1.0 dB/year in patients with baseline MD of -5 dB, standard automated perimetry testing approximately every 6 months was the average frequency needed to detect progression within 5 years in patients with high test variability (>2 dB). Patients with low variability (<1 dB) in this same group needed testing approximately every 18 months to detect progression within 5 years on average.
I surveyed 105 clinicians who care for patients with glaucoma about clinical decision-making and interest in a CDS system for glaucoma.3 Clinicians identified visual field timing as a difficult clinical decision, and 90/105 (85.7%) felt that determining visual field timing would benefit from CDS. Overall, the clinicians expressed strong interest in using a CDS system for glaucoma, with 94/105 (89.5%) interested. This high level of interest increases the likelihood of successful CDS implementation.15
Using a statistical model that calculates a personalized frequency for visual field testing, I developed a prototype Glaucoma CDS System. Data from my survey guided this prototype.3 Most clinicians felt that automatic data entry, an easy-to-use interface, and minimal time to use the tool would be “very important.”
CDS systems such as this can potentially reduce clinician cognitive burden and improve patient care. As these systems are developed, it is important they are designed to be integrated within patient care to support rather than replace patient and clinician decision-making.
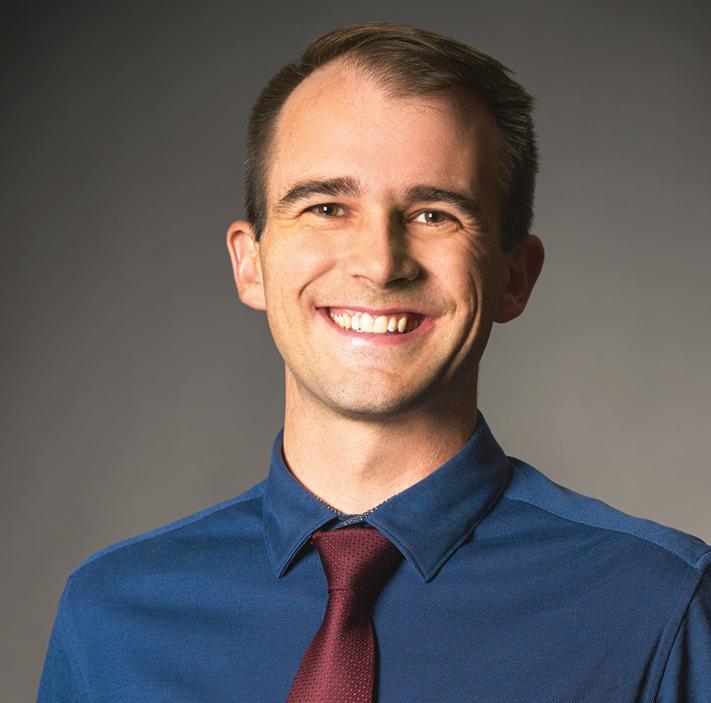
Dr. Stagg specializes in the diagnosis and treatment of glaucoma. His research focuses on using modern technology to personalize glaucoma care to ensure that each patient gets appropriate treatment.
REFERENCES
1. Prum BE, Rosenberg LF, Gedde SJ, et al. Primary Open-Angle Glaucoma Preferred Practice Pattern(®) Guidelines. Ophthalmology. 2016;123(1):P41-P111.
2. Crabb DP, Russell RA, Malik R, et al. Frequency of Visual Field Testing When Monitoring Patients Newly Diagnosed with Glaucoma: Mixed Methods and Modelling. NIHR Journals Library; 2014. Accessed June 10, 2019. http://www.ncbi.nlm.nih.gov/books/NBK259972/.
3. Stagg B, Stein JD, Medeiros FA, Cummins M, Kawamoto K, Hess R. Interests and needs of eye care providers in clinical decision support for glaucoma. BMJ Open Ophthalmology. 2021;6(1):e000639.
4. Stagg BC, Stein JD, Medeiros FA, et al. Special Commentary: Using Clinical Decision Support Systems to Bring Predictive Models to the Glaucoma Clinic. Ophthalmology Glaucoma. Published online August 15, 2020.
5. Matheny ME, Whicher D, Israni ST. Artificial Intelligence in Health Care: A Report From the National Academy of Medicine. JAMA. Published online December 17, 2019.
6. Tcheng JE, National Academy of Medicine (U.S.), eds. Optimizing Strategies for Clinical Decision Support: Summary of a Meeting Series. National Academy of Medicine; 2017.
7. Berner E, ed. Clinical Decision Support Systems: Theory and Practice. 3rd Edition. Springer International Publishing; 2016.
8. Bright TJ, Wong A, Dhurjati R, et al. Effect of Clinical Decision-Support Systems: A Systematic Review. Ann Intern Med. 2012;157(1):29.
9. Kawamoto K, Kukhareva P, Shakib JH, et al. Association of an Electronic Health Record Add-on App for Neonatal Bilirubin Management With Physician Efficiency and Care Quality. JAMA Netw Open. 2019;2(11):e1915343.
10. Chauhan BC, Mikelberg FS, Artes PH, et al. Canadian Glaucoma Study: 3. Impact of risk factors and intraocular pressure reduction on the rates of visual field change. Arch Ophthalmol. 2010;128(10):1249-1255.
11. Chauhan BC, Malik R, Shuba LM, Rafuse PE, Nicolela MT, Artes PH. Rates of Glaucomatous Visual Field Change in a Large Clinical Population. Invest Ophthalmology Vis Sci. 2014;55(7):4135-4143.
12. Heijl A, Buchholz P, Norrgren G, Bengtsson B. Rates of visual field progression in clinical glaucoma care. Acta Ophthalmology. 2013;91(5):406-412.
13. Stagg B, Mariottoni EB, Berchuck S, et al. Longitudinal visual field variability and the ability to detect glaucoma progression in black and white individuals. Br J Ophthalmology. Published online May 13, 2021.
14. Abe RY, Diniz-Filho A, Costa VP, Wu Z, Medeiros FA. Predicting Vision-Related Disability in Glaucoma. Ophthalmology. 2018;125(1):22-30.
15. Kawamanto K, Flynn MC, Kukhareva P, et al. A Pragmatic Guide to Establishing Clinical Decision Support Governance and Addressing Decision Support Fatigue: a Case Study. AMIA Annu Symp Proc. 2018;2018:624-633.
FINANCIAL DISCLOSURE
Dr. Stagg has no financial interest in any of the products mentioned in this article.