International Journal of Fuzzy Logic Systems (IJFLS) Vol.12, No.1/2/3/4, October 2022
ACONSENSUSMODELFORGROUPDECISIONMAKING WITHHESITANTFUZZYINFORMATION
Abstract. Thisarticlepresentsamoreimprovedconsensus-basedmethodfor dealingwithmulti-persondecisionmaking(MPDM)thatuseshesitantfuzzy preferencerelations(HFPRís)thatarenítintheusualformat.Weproposed aLukasiewicztransitivity(TL-transitivity)-basedtechniqueforestablishing normalisedhesitantfuzzypreferencerelations(NHFPRís)atthemostessentiallevel,afterthat,amodelbasedonconsensusisconstructed.Afterthat,a transitiveclosureformulaiscreatedinordertobuildTL -consistenthesitant fuzzypreferencerelations(HFPRís)andsymmetricalmatrices.Afterwards, aconsistencyanalysisisperformedtodeterminethedegreeofconsistencyof thedatagivenbythedecisionmakers(DMs),asaresult,theconsistency weightsmustbeassignedtothem.Aftercombiningconsistencyweightsand preset(predeÖned)priorityweights,theÖnalpriorityweightsvectorofDMs isobtained(ifthereareany).Theconsensusprocessdetermineseitherdata analysisandselectionofasuitablealternativeshouldbedonedirectlyorexternally.TheenhancementprocessaimstoimprovetheDMísconsensusmeasure, despitetheimplementationofanindicatorforlocatingsluggishpoints,inthe circumstancethatanunfavorableagreementisachieved.Finally,acomparisoncasedemonstratestherelevanceande§ectivenessoftheproposedsystem. Theconclusionsindicatethatthesuggestedstrategycanprovideinsightinto theMPDMsystem.
1. Introduction
Decision-makingisanessentialcomponentofhumanexistence.Severalofthem urgedecision-makerstomake"rational"or"great"judgments[1]basedonasetof criteriaforassessingparticularpossibilities.WhenselectingapreferencespossibilityinpracticaldomainswithasetofcriteriathatvariesandmaybeclassiÖed,there isfrequentlyacriteraconáict[2].Multi-criteriadecisionsupportapproachesare oftenusedfordecisionmakerstoevaluatechoiceoptions.Onewaydecisionsupport isimplementedisasameanstoevaluatetheuseofenvironmental,economic,and ecologicalfactors.
Multi-persondecisionmaking,alsoreferredtoasMPDM,isanimportantmethod forachievingoptimalchoiceresultsinmodernsociety.However,givenvaryinglevels ofexpertiseandinterpretation,DMíscontributionstotheevaluationwillvarydependingontheirabilities.Thisthereforepresentsachallengewhentryingtocome togetherandagreeonaconclusion.Thisisakeychallengeinthedecision-making processasitbeginswithassessing,giventhatdecisionsaretypicallybestsuited tomanydi§erentlevelsofconsideration.Oneofthedi¢cultiesinvolvedwithconsensusdecision-makingisachievingunanimousandacceptableoutcomes.Sincea
Keywordsandphrases. Fuzzypreferencerelation(FPR),Hesitantfuzzypreferencerelation (HFPR),AdditiveTransivity.
GDMisaconsensus-drivenprocess,variousstrategiesforreachingane§ectiveconsensushavebeenpositedandinvestigated,resultinginanexcessivepercentageof data.Zhangetal.[3]havedevelopedacost-e§ectivemodelformaximumsupport degreeconsensusprocessing,whichcanalsobeappliedforreluctantinformation andlinguisticjudgmentswherethedegreeofcertaintyislow.Lietal.[4]proposed ane§ectiveinteractivetechniqueforachievingconsensusatalowandunpredictable cost,Öxingcommunicationproblemsbetweenhumanparticipants.Aprobabilistic hesitantfuzzypreferencerelationisatypeoffamilyofdecisionproblemsinwhich eachmemberhasitsownassociatedrateofgreaterorlesspreference.LiandWang [5]presentedanautomaticiterativeapproachtoobtainaconsensuslevel.Tianand Wangetal.andZhangetal.[6,7]developedconsensus-reachingmodelsofhuman behaviour,asdidHerrera-Viedmaetal.[8],whostudiedmodelanalysisinfuzzy environments.
Theyusedaconsensusmodelwithheterogeneouslarge-scaleGDMwithsatisfactionandindividualconcernstoexaminehowindividualsinteractwithoneanother. Thehesitantfuzzypreferencerelation(HFPR)conceptcreatedbyXiaandXu [9]iscurrentlybeingemployedinMPDMasane¢cientandsimpleapproachfor exchangingalternativefactsacrossgroupsofDMís.Theconsensus-buildingmechanism,whichisbasedonpreferencerelations,isregularlyutilizedasaapparatus inMPDMstrategies.Thefuzzypreferencerelation(HFPR)conceptmadebyXia andXuisasofnowbeingusedasanproÖcientandbasicinstrumentforagather ofDMstotradeelectiveactualities.ManyacademicshaveexploredtheHFPR suggestedin[9]inthecontextofGDM[12,13,14],butsigniÖcantdisagreements persist,forexample,whileo§eringthedecisiondegreetowhichanalternative x1 is superiortoanotheralternative x2 foragroupofthreeDMs.IfnoneoftheDMsare abletoagreeontheirappraisal,thepreferreddegreeof x1 to x2 canbeasetmade upoftheircombinedjudgementsexpressedasthehesitantfuzzyelement(HFE). TheHFPRhastheimmediatebeneÖtofprovidingtheDMswithasetofvalues displayingtheoutcomesoftheevaluation.However,theHFEssupplyalimited amountofcomponents,whichmightmakereachinganagreementdi¢cult.Most consensusmodels,forexample,arefocusedoncalculatingthedistancebetweentwo HFPRs,anddeterminingane§ectivedistancebetweenthemisquitechallenging [15].
Manyscholarshavecreatedvariouswaysforobtainingthepriorityweightvector, aswellasconsensusreachingmodels.Zhuetal.[16]weretheÖrsttoproposeaand bnormalisationprocedures.Thenormalization-basedstrategydemandedthatany twoHFEshavethesameamountofelements.Thisstrategywashighlyregarded byanumberofdecision-makingacademics.ZhangandWu[17]developedgoal programmingmodelsfortheincompletehesitantmultiplicativepreferencerelation (HFPR)andestimatedthepriorityweightvectorsusingaandbnormalisation approaches.Mengetal.[18]developedauniqueconsistencytechniqueforhesitant multilicativepreferencerelations,whichyieldedthehesitantfuzzypriorityweight vector.Zhangetal.[19]establishedvariouspreferencerelationsbasedonq-rung orthopairfuzzysetsandstudiedamethodforextractingpriorityweightsfromsuch relations.Sincemanyresearchershavebeencompelledtousethesetwoprocedures indeÖnitely,aplethoraofothernormalisationmethodshavebeendeveloped.For example,Xuetal.[20]createdaconsensusmodelfortacklingwaterallocation managementproblemsusinganadditiveconsistency-basednormalisingtechnique.
International Journal of Fuzzy Logic Systems (IJFLS) Vol.12, No.1/2/3/4, October 2022
Becauseoftheirlimitedcompetenceandexperience,DMsmaystruggletoconstruct entirepreferenceconnectionsduringpairedjudgmentsonalternatives.Approaches thataidinthemanagementofHFPRswithinsu¢cientinformationarerequired.
Zhangetal.[21]suggestedatechniqueforguessingmissingHFPRcomponents. KhalidandBeg[22]suggestedanalgorithmfortheDMsthatmakesuseofahesitant upperboundcondition.InpairedevaluationsofDMíspreferences,theconsistency offuzzypreferencerelations(HFPR)iscrucialinthedecision-makingprocess. ThedistancemetricbetweennormalisedHFPRandconsistentHFPRiscrucialin determiningtheconsistencydegreeofHFPR.Zhuetal.[23]developedaregression approachandmethodologyfortransformingHFPRintoafuzzypreferencerelation withthemaximumlevelofconsistency.
Inthispaper,wedeÖneadditivereciprocitywhichisane§ectwaytocalculatetheDMísintheMPDMproblems.Additivereciprocityismoresu¢cientans easymethodtodeterminetheunknownandmissingvalues.Thisworkproposesa consensus-basedsolutionfordealingwiththeMPDMproblemutilisingconsistent HFPRs.Theauthorspresentanenhancedapproachforsuggestingagreementin groupdecisionmakingbasedonTL-consistencyinthecontextofHFPRs.They alsosuggestatechniqueforacceleratingtheexecutionofahigherconsensuslevel onaneasypath.WhenanFPRhasmissingpreferences,theproposedtechnique estimatesmoreappropriateandconsistentvalues.Theattributeofconsistencyis linkedtothetransitivityproperty,forwhichnumerousrelevantformsorconditions havebeenproposed.IntheÖrstphase,weusetheTL-transitivitycharacteristic toassessthemissingpreferencesofIFPRs.Then,weproposemodiÖedconsistency matricesofexpertsthatmustmeetTL-consistency.Theexpertsareassigneda levelofrelevancebasedonaccuracyweightscombinedwithconÖdenceweights.
AnewapproachfornormalisingtheHFPRsisdevelopedusingadditivereciprocityandthenextendedtotheMPDMissueutilisingconsistencyandconsensus metrics.Section4includesacomparisonexampletoevaluatethee¢cacyofthe suggestedstrategy.Thistextisarrangedasfollows:inSection2,somefundamental deÖnitionsarepresentedtoassistthereaderinunderstandingthework;inSection 3,anovelprocessisproposedanditse¢ciencyisevaluated.Section5compares theÖndingsachievedusingoursuggestedapproachtothosefoundintheliterature. TheÖnalparthassomeconclusions.
2. Preliminaries
AfuzzysetisanotionestablishedbyL.A.Zadeh[24]in1965todescribehow anitemismoreorlesstiedtoacertaincategorytowhichwedesiretoadapt.Some basicinformationisprovidedinthisparttoassisteveryoneunderstandthetopic better.
DeÖnition1. FuzzySet [24]:ThefuzzysetisdeÖnedasfollows:If X isadiscourse universeand x isaspeciÖcelementof X,thenafuzzyset A deÖnedon X canbe expressedasacollectionoforderedpairs A = f(x; A(x));x 2 Xg Aspartofthe membershipfunction A : X ! [0; 1],thedegreetowhich x relatesto A isdenoted as A(x)
DeÖnition2. HesitantFuzzySet [25]:Let X representauniversalset.AnHFS on X isdeÖnedasafunctionthatreturnsasubsetof [0; 1] whenappliedto X.The membershipdegreeof x 2 X isrepresentedbythisvalue,whichisreferredtoasthe hesitantfuzzyelement(HFE).
International Journal of Fuzzy Logic Systems (IJFLS) Vol.12, No.1/2/3/4, October 2022
DeÖnition3. FuzzyPreferenceRelation [26]:Ifthesetofalternatives A = fA1;A2;::::;Ang exists,thenthefuzzypreferencerelation U on A A withthe requirement uij 0;uij + uji =1 exists,iscalled additivereciprocity [27];i;j = 1; 2;::::;n; thedegreetowhichtheoption Ai ispriortothealternative Aj isdenoted by uij :Ai and Aj ,representedby Ai Aj areindi§erenceat uij =0 5 ; 0 uij < 0 5 denotesthat Aj ispreferableover Ai whichisindicatedby Aj Ai; thelower thevalueof uij ,thebetter,thestrongerthepreferencefor Aj over Ai,thebetter; 0 5 <uij 1 denotesthat Ai ispreferableto Aj ,whichissymbolisedby Ai Aj , thegreater uij ísvalue,thehigherthepreferencefor Ai over Aj ,thebetter.Itshould beemphasisedthatinafuzzypreferencerelation,thevalue uij isanumberbetween 0 and 1.
DeÖnition4. Hesitantfuzzypreferencerelation [28]:let A = fA1;A2;::::;Ang isa Öxedset,thenamatrix H =(hij )n n @ A A presentsahesitantfuzzypreference relation H on A,where hij = fhij j =1; 2;::::lhij g isanHFEreáectingallthe possibledegreestowhichAi ispreferredoverAj ,in hij hij isthe thelement, thenumberofvaluesin hij isrepresentedby lhij .Furthermore, hij mustmeetthe followingrequirements:
8 < : hij + hji =1;i;j =1; 2;:::;n hii = f0:5g;i =1; 2;::::;n lhij = lhji ;i;j =1; 2;:::;n
DeÖnition5. IncompleteFuzzyPreferenceRelation [11]:If U =(uij )n n isa preferencerelation,thenUisreferredtoasaincompletefuzzypreferencerelation, iftheDMisunabletoprovidesomeofitselements,itisrepresentedbytheunknown numberx,andtheDMwillbeabletoo§ertheremaining.
DeÖnition6. AdditiveTransitivity:if 8 i;k;j 2 X holds,then R isknownas additivetransitive:
R(x;z)= R(x;y)+ R(y;z) 0:5
DeÖnition7. ukasiewiczTransitivity:UissaidtobeTL-transitiveifitholds
8 i;k = j 2f1; 2;:::;ng : uik max(uij + ujk 1; 0):
DeÖnition8. ConsistentFuzzyPrefrenceRelation:AFuzzyPrefrenceRelationU issaidtobeTL-transivity(consistent),iffor 8 i;k = j 2f1; 2;:::;ng : uik max(uij + ujk 1; 0)(TL-transivity)issatisÖed.
3. Methodology
Throughoutthissection,weo§eredasuperiortechniquefordealingwithMPDM issuesusingHFPRs,whichincludedthefollowingphases:normalisation,consistencymeasures,consensusmeasures,consensusenhancingprocess,allocatingpriorityweightstoDMs,andselectionprocess.
3.1. Normalization. Withinthispart,itisproposedthatanewapproachfornormalisingHFPRsbedeveloped,becauseforanytwohesitantfuzzypreferencevalues (HFPVs), hij and hlm, jhij j6= jhlmj for i;j;l;m 2f1; 2;::::;ng inthemajority ofcases,Setsofpairwisecomparisonswiththeircardinalitiesatthe ijthand lmth placesarerepresentedby jhij j & jhlmj.Weuseadditivereciprocitytodiscoverthe unknownpreferencesofIFPRsintheÖrststage.Regardingthenormalisingofthe
International Journal of Fuzzy Logic Systems (IJFLS) Vol.12, No.1/2/3/4, October 2022
givenHFPRs,weproposeanewmethodforcalculatingthecomponentsthatwill beaddedtoHFPVs.Otherintermediatevaluescannotbeaddedtotheestimated elementsinceitisjustthemaximumorlowestentryofHFPV.Thesuggestedtechniqueisbasedonadditivereciprocity,inwhichwegeneratetheincompletefuzzy preferencerelation(s)IFPRsusingunknownpreferencevaluesforthecomponents tobeadded.
3.2. DetermineMissingValues. An IFPR basedonTL-consistencycanonly becompletedifeachoptionamongtheknownpreferencevaluesisinvestigatedat leastonce.Asaresult,thesystemmustrequestthattheexpertcreateasu¢cient numberofpreferences,witheachpossibilitybeingexaminedatleastonceinorder forthe IFPR tobecomeacomplete FPR.Thesequenceinwhichthemissing preferencevaluesaremeasuredhasane§ectontheÖnaloutcome.Forthepurpose ofconstructingtheunknownormissingpreferencevaluesinan IFPRR =(rij )n n, thebelowmentionedsetsareusedtodenotethealternativeíspairsforknownand unknownormissingpreferencevalues
(3.2) where rij 2 [0; 1] arethepreferencevaluesof ai over aj , rij + rji =1=) rii = 0 5 8 i 2f1; 2;::::;ng.Asaresult,basedonTL-transitivity rik max (rik + rkj 1; 0),toestimatetheunknownpreferencevalue rij ofalternative ai overalternative aj ,thefollowingsetcanbedeÖned.
(3.3) Eij = fk = i;jj(i;k) 2 Ke; (k;j) 2 Ke and (i;j) 2 Ueg for i;j;k 2f1; 2; 3;:::;ng.UsingtheEquation(3.3),theÖnalvalueof rij iscalculatedasfollows:
(3.4) rij = avek2Eij (max(rik + rkj 1; 0));if jEij j6=0 0 5;otherwise and
(3.5) rji =1 rij (additivereciprocity)
Finally,theentireFPR R =(rij )n n isproduced.Now,twoadditionalsetsof knownandunknownelements, Ke and Ue,aredeÖnedasfollows:
(3.6) Ke = Ke [f(i;j)g; and Ue = Ue f(i;j)g
Asaresult,anormalisedhesitantfuzzypreferencerelation(NHFPR) H =(hij )n n with hij + hji =1;hij = fhij j =1; 2;:::; jhij jg for jhij j = jhjij
isconstructed.Becauseofthegrowthincomplexity,thereareseveraldecisionmakingproceduresthatoccurinmulti-personsituationsintherealworldand becauseoftheunpredictabilityofthesocioeconomicenvironment,itismoredi¢cult forasingledecisionmakercanassessalloftheinterconnectedcomponentsofa decision-makingchallenge.
International Journal of Fuzzy Logic Systems (IJFLS) Vol.12, No.1/2/3/4, October 2022
3.3. ConsistencyAnalysis. Someconsistencymeasurements,suchastheamount ofconsistencybetweentwoalternatives,thedegreeofconsistencyamongalternatives,andHFPRíslevelofconsistency,arespeciÖedinthissubsection.Theterm consistencyindex(CI)referstothedegreeofconsistencywithavaluebetween 0 and 1.Assume Hq istheHFPRforthedecisionmaker Dq(1 q l),thenafter receivingtheNHFPR H q,Thefollowingtransitiveclosureformulamaybeused toobtainTL-consistentHFPR H q:
(3.7) ~ h q ij =max k=i;j(h q ij ; max(h q ik ;h q kj 1; 0)) ; ~ h q ij + ~ h q ji =1
where h q ij = fhij j =1; 2;:::; jhij jg.Afteranalysingthedistancebetweenthem inthefollowingmanner,wecancalculatetheHFPR H q consistencylevelbased onitssimilaritytothecorrespondingTL-consistency H q
1. TL consistencyindex(TLCI)forapairofalternativesrankedasfollows:
(3.8) TLCI(h q ij )=1 1
ij j jhij j X =1
d( h q ij ; h q ij )
where d( h q ij ; h q ij ) denotesthedistanceobtainedby d( h q ij ; h q ij )= j h q ij h q ij j Thegreaterthelevelof TLCI(h q ij ),themoreconsistent h q ij isincomparison totheotherHFPVswhenitcomestotheoptions ai and aj
2. TLCI foralternativesai,1 i n,asfollows:
with TLCI(ai) 2 [0; 1].If TLCI(ai)=1,thenthealternative ai preferencevaluesare totallyconsistent;ontheotherhand,thelower TLCI(ai),themoreinconsistency thereisinthesepreferencevalues.
3.Finally, TLCI againstNHFPRtheaverageoperatorisusedtoassess H q:
TLCI(H q) 2 [0; 1].If
H q istotallyconsistent,if
LCI(H q) isless, H q ismoreinconsistent.Equation(3.10)assignsDM Dq to theconsistencyindex,howevertheglobalconsistencyindex(CI) maybecalculated usingtheaverageoperatorandisprovidedas:
CI 2 [0; 1].The TLCI iscalculatedinthreesteps,eachcomprisingEquations(3.8)-(3.10),ExpertswhoproducedtheHFPRwithbetterconsistencyindices shouldbegivenmoreweights.Asaresult,thefollowingrelationshipmaybeused toassignconsistencyweightstoexperts: (3.12)
with Cw(Dq) 2 [0; 1]& l X q=1
Cw(Dq)=1
3.4. ConsensusAnalysis. Somelevelsforestimatingglobalconsensusamong decisionmakersaredeÖnedinthissubsection.Itiscriticaltodeterminetheamount ofconsensusamongdecisionmakersafterexaminingNHFPRs H q;q =1; 2;:::;l Afteraggregatingthesimilaritymatrices Sqr =(sqr ij )n n foreachpairofdecision makers (Dq;Dr)(q =1;
similaritymatrixthatmaybebuiltasfollows:
; 2;:::; jhij jg Thefollowinglevelsareusedtodeterminethedegreeofglobalconsensusamongdecision-makers:
1.Thedegreeofconsensusonapairofalternatives
2.Thedegreeofconsensusonalternatives
3.Thedegreeofconsensusontherelationrepresentedby CR isspeciÖedatthe thirdleveltodeterminetheglobalconsensusdegreeamongallDMs: (3.16)
Ifallspecialistsreachalevelofglobalconsensus,acomparisonwiththethresholdconsensusdegree isrequired,thenatureoftheproblemistypicallypredetermined.If CR isachieve,itmeansthattherehasbeenasu¢cientlevel ofconsensusobtained,andtheprocessofdecision-makingmaybegin.Asidefrom that,theconsensusdegreeisunstable,andexpertsarebeingpressedtoaltertheir preferences.
3.5. EnhancementMechanism. Theenhancementmechanismactsasamoderatorintheconsensus-buildingprocess,providingdecisionmakerswithdetailed informationtohelpthemimprovetheirresults.Incaseofinadequateconsensus level,wemustdeterminetheplaceswherepreferencevalueswillbeupdated,so thatdecision-makerscanattainanappropriatelevelofconsensusInthisregard, thefollowingisadeÖnitionofanidentiÖer:
(3.17) Iq = f(i;j)j cdij <CR & hq ij isaknownvalueg
OncethepositionshavebeenestablishedusinganidentiÖer,theenhancementmechanismrecommendsthattherelevantDM Dq augmenttheelement hq ij ofHFPV hq ij , ifitislessthanthemeanvalue h q ij;ave oftheparticipantísopinions,ortodeclineif thevalueismorethanthemean,andtoremainthesameifthevalueisequaltothe
International Journal of Fuzzy Logic Systems (IJFLS) Vol.12, No.1/2/3/4, October 2022
mean.Toautomaticallyimprovetheconsensus,theDMswouldnothavetogive theirupdatedcomponentsintheautomatedprocedure.Insuchacase,thenew element hq ij;new maybeevaluatedusingthefollowingequation,newfor cdij <CR:
(3.18) hq ij;new = hq ij +(1 )h q ij;ave where 2 [0; 1] isknownastheoptimizationparameter.Thenewevaluatedvalues willundoubtedlybeclosertothemeanvaluesthanthepreviousones,andsothe degreeofconsensuswillincrease.
3.6. RatingofDecisionMakers. ToanalysedecisionmakersíÖnalpriorityevaluations,emergingconsistencyweightsandpredeÖnedpriorityweightsareemployed, whichareasfollows:
(3.19) w(Dq)= wq Cw(Dq) l X q=1
wq Cw(Dq) where wq; 1 q l; denotesthedecisionmakeríspredeÖnedpriorityweights,while l X q=1
w(Dq)=1: IftheDMsdonotusepredeÖnedpriorityweights,then,astheÖnal priorityrating,theirconsistencyweightswillbeused.
3.7. AggregatedNHFPR. Onaregularbasis,thepreferencelevelassociated witheachDMmaybeweighteddi§erently.Afterreviewingdecisionmakeríspriority ratings,theirviewsaretobeconsolidatedintoaglobalone.Usingtheweighted averageoperator,wecreatethecollectiveconsistentNHFPR H c asfollows: (3.20) H c =(h c ij )n n =(
3.8. RankingofAlternatives. WhentheDMsattainanappropriatedegreeof consensus,theprocessofrankingthealternativesbeginsandthebestoneischosen. Therankingvalue v(ai) foralternative ai; (i =1; 2;:::n); isdeÖnedinthiscontext, asfollows:
4. ComparativeExample
Abankwantstoputaparticularamountofmoneyintothebestoption.To reducetherisksofmakingjudgmentsinthishighlycompetitiveandfast-paced industry,thecompanyísleaderenliststheassistanceofagroupofexpertsinthe decision-makingprocessinthehopesofreachingaconsensus.Thefollowingisa panelwiththreeoptions:
a1 istheautomobileindustry,
a2 isthefoodindustry,
a3 isthecomputerindustry
International Journal of Fuzzy Logic Systems (IJFLS) Vol.12, No.1/2/3/4, October 2022
;q =1; 2; 3 fromthreeconsultingdepartmentsistaskedwithassessingthethreeoptions. ai;i =1; 2; 3:Followingpairwisecomparisons,theDMs
Threespecialistshavebeenconsulted,D
; 2; 3, respectivelywiththresholdconsensuslevel
ConsistencyAnalysis:
TheHFPRísconsistencylevelsasstatedbydecisionmakersweremeasuredusing expressions(3.7)ñ(3.12).
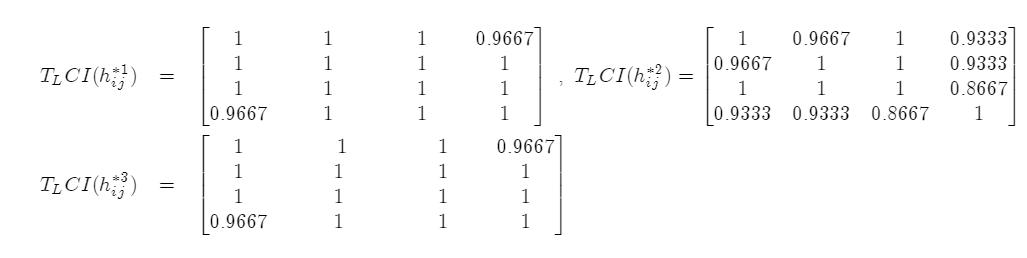
International Journal of Fuzzy Logic Systems (IJFLS) Vol.12, No.1/2/3/4, October 2022
CollectiveNHFPRconstruction: Afterapplying(3.20),wegetthecollectiveNHFPR
Theequation(3.21)isusedtogettheÖnalrankingorderofthealternativesafter analysingtherankingvalues.
)=1 Asaresult,thepreferredorderofalternativesis
Asaresult,DMsmustaltertheirpreferenceswiththehelpof(3.17).Thefollowing arethemeanvaluesoftheexpertíspreferences:
TheidentiÖer(3.17)nowo§ersthefollowingsetofoptionsforimprovingrelevant preferencevalues.
theirpreferencerelationsasaresult:
International Journal of Fuzzy Logic Systems (IJFLS) Vol.12, No.1/2/3/4, October 2022
Equation(3.1)ñ(3.6)wereutilisedtogenerateNHFPRsinordertonormalisethe provideddata
ConsistencyAnalysis:
TheHFPRísconsistencylevelsasstatedbydecisionmakersweremeasuredusing expressions(3.7)ñ(3.12).
International Journal of Fuzzy Logic Systems (IJFLS) Vol.12, No.1/2/3/4, October 2022
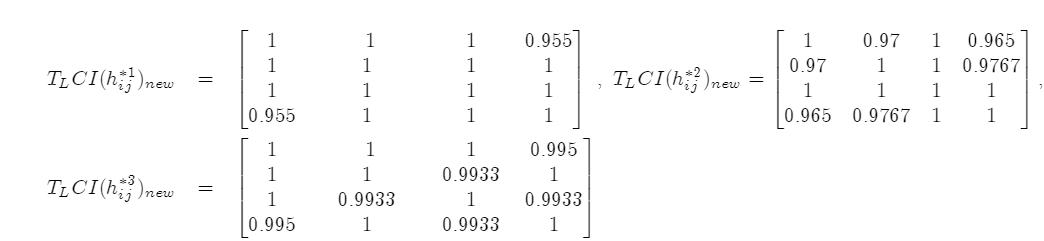
FinalRankingofalternatives:
Theequation(3.21)isusedtogettheÖnalrankingorderofthealternativesafter analysingtherankingvalues.
5. Comparison
BycomparingourresultstothoseofXuetal.[29]basedonconsistencymeasure, consensusmeasure,andÖnalranking,weclearlyverifythesuggestedtechnique.AccordingtoXuetal.[29],theinitialconsistencylevels,consensuslevel,andultimate rankingofalternativesareasfollows: CR =0 7554;a4 a3 a2 a1 respectively.
Additivereciprocityisincorporatedintooursuggestedstrategytoevaluatethe HFPVísunidentiÖedcomponentsthroughoutthenormalisationprocessandcreate theconsistentHFPRsinaccordance.
TABLE:TheresultswerecomparedoftheXuetal.resultsandthesuggested method

6. Conclusions
Aconsensus-basedtechniquefordealingwiththeMPDMproblemusingconsistentHFPRsisproposedinthispaper.Inthisregard,thenotionofHFPRswas derivedfromtheworkofXu[20],andweproposeane§ectiveadditivereciprocitybasedstrategyfornormalisingHFPRs.
Theaboveexampledemonstratesastep-by-stepprocedurefornormalisingthe HFPR.Followingaconsistencystudy,DMswereassignedconsistencyweights.To carrygreaterweightintheaggregationprocess,DMswithahighlevelofconsistencyshouldbegivenlargerweights.Furthermore,animprovementMechanism isprovidedtoaidintheimplementationofahigherdegreeofagreementona straightforwardpath.WhentheDMshavereachedanadequatelevelofconsensus,
International Journal of Fuzzy Logic Systems (IJFLS) Vol.12, No.1/2/3/4, October 2022
thetechniquemovesontotheselectionstep,whichincludesmethodsforaggregatingandrankingtodeterminethebestsolution.Acomparativecaseiscreatedto highlightthefeasibilityande¢cacyoftheproposedmethod.TheÖndingsprovide usabetterunderstandingoftheMPDMprocess.
7. FutureWork
Themainfutureworkofthisproblemisthatwecanextendthisproblemby utilizingdualhesitantfuzzysetandbyapplyingaggregationoperatorwecanmake thisproblemmorecompact.Thisproblemcanalsobeextendedbyusingtriangular fuzzynumber.
References
[1]WæatrÛbski,J.,Jankowski,J.,Ziemba,P.,Karczmarczyk,A.,&Zio˜o,M.(2019).Generalised frameworkformulti-criteriamethodselection. Omega,86,107-124.
[2]Stojcic,M.,Zavadskas,E.K.,Pamucar,D.,Stevic, Z.,&Mardani,A.(2019).Application ofMCDMmethodsinsustainabilityengineering:Aliteraturereview2008ñ2018. Symmetry, 11(3),350.
[3]Zhang,C.,Zhang,H.,&Wang,J.(2018).Personalizedrestaurantrecommendationmethod combininggroupcorrelationsandcustomerpreferences. InformationSciences,454,128-143.
[4]Li,Y.,Zhang,H.,&Dong,Y.(2017).Theinteractiveconsensusreachingprocesswiththe minimumanduncertaincostingroupdecisionmaking.
[5]Li,J.,Wang,J.Q.,&Hu,J.H.(2019).Consensusbuildingforhesitantfuzzypreference relationswithmultiplicativeconsistency. Computers&IndustrialEngineering,128,387-400.
[6]Tian,Z.P.,Wang,J.Q.,Zhang,H.Y.,&Wang,T.L.(2018).Signeddistance-basedconsensus inmulti-criteriagroupdecision-makingwithmulti-granularhesitantunbalancedlinguistic information. Computers&IndustrialEngineering,124,125-138.
[7]Zhang,H.,Dong,Y.,&Herrera-Viedma,E.(2017).Consensusbuildingfortheheterogeneous large-scaleGDMwiththeindividualconcernsandsatisfactions. IEEETransactionsonFuzzy Systems,26(2),884-898.
[8]Herrera-Viedma,E.,Cabrerizo,F.J.,Kacprzyk,J.,&Pedrycz,W.(2014).Areviewof softconsensusmodelsinafuzzyenvironment. InformationFusion,17,4-13. AppliedSoft Computing,60,202-212.
[9]Xia,M.,&Xu,Z.(2013).ManaginghesitantinformationinGDMproblemsunderfuzzy andmultiplicativepreferencerelations. InternationalJournalofUncertainty,Fuzzinessand Knowledge-BasedSystems,21(06),865-897.
[10]Zhang,C.,Liao,H.,&Luo,L.(2019).Additiveconsistency-basedpriority-generatingmethod ofq-rungorthopairfuzzypreferencerelation. InternationalJournalofIntelligentSystems, 34(9),2151-2176.
[11]Xu,Z.S.(2004).Goalprogrammingmodelsforobtainingthepriorityvectorofincomplete fuzzypreferencerelation. Internationaljournalofapproximatereasoning,36(3),261-270.
[12]He,Y.,&Xu,Z.(2017).Aconsensusreachingmodelforhesitantinformationwithdi§erent preferencestructures. Knowledge-BasedSystems,135,99-112.
[13]Wu,Z.,Jin,B.,&Xu,J.(2018).Localfeedbackstrategyforconsensusbuildingwith probability-hesitantfuzzypreferencerelations. AppliedSoftComputing,67,691-705.
[14]Xu,Y.,Chen,L.,RodrÌguez,R.M.,Herrera,F.,&Wang,H.(2016).Derivingthepriorityweightsfromincompletehesitantfuzzypreferencerelationsingroupdecisionmaking. Knowledge-BasedSystems,99,71-78.
[15]Meng,F.,&An,Q.(2017).Anewapproachforgroupdecisionmakingmethodwithhesitant fuzzypreferencerelations. Knowledge-BasedSystems,127,1-15.
[16]Zhu,B.,Xu,Z.,&Xu,J.(2013).Derivingarankingfromhesitantfuzzypreferencerelations undergroupdecisionmaking. IEEETransactionsonCybernetics,44(8),1328-1337.
[17]Zhang,Z.,&Wu,C.(2014).Adecisionsupportmodelforgroupdecisionmakingwithhesitant multiplicativepreferencerelations. InformationSciences,282,136-166.
[18]Meng,F.,Tang,J.,An,Q.,&Chen,X.(2019).Anewprocedureforhesitantmultiplicative preferencerelations. InternationalJournalofIntelligentSystems,34(5),819-857.
International Journal of Fuzzy Logic Systems (IJFLS) Vol.12, No.1/2/3/4, October 2022
[19]Li,H.,Yin,S.,&Yang,Y.(2019).Somepreferencerelationsbasedonq-rungorthopairfuzzy sets. InternationalJournalofIntelligentSystems,34(11),2920-2936.
[20]Xu,Y.,Cabrerizo,F.J.,&Herrera-Viedma,E.(2017).Aconsensusmodelforhesitant fuzzypreferencerelationsanditsapplicationinwaterallocationmanagement. AppliedSoft Computing,58,265-284.
[21]Zhang,Z.,Wang,C.,&Tian,X.(2015).Multi-criteriagroupdecisionmakingwithincomplete hesitantfuzzypreferencerelations. AppliedSoftComputing,36,1-23.
[22]Khalid,A.,&Beg,I.(2017).Incompletehesitantfuzzypreferencerelationsingroupdecision making. InternationalJournalofFuzzySystems,19(3),637-645.
[23]Zhu,B.(2013).Studiesonconsistencymeasureofhesitantfuzzypreferencerelations. Procedia ComputerScience,17,457-464.
[24]Zadeh,L.A.,Klir,G.J.,&Yuan,B.(1996). Fuzzysets,fuzzylogic,andfuzzysystems: selectedpapers (Vol.6).WorldScientiÖc.
[25]Torra,V.(2010).Hesitantfuzzysets. Internationaljournalofintelligentsystems,25(6), 529-539.
[26]Tanino,T.(1988).Fuzzypreferencerelationsingroupdecisionmaking. Non-conventional preferencerelationsindecisionmaking,54-71.
[27]Orlovsky,S.(1978).Decision-makingwithafuzzypreferencerelation. Fuzzysetsandsystems, 1(3),155-167.
[28]Xia,M.,Xu,Z.,&Chen,N.(2013).Somehesitantfuzzyaggregationoperatorswiththeir applicationingroupdecisionmaking. GroupDecisionandNegotiation,22(2),259-279.
[29]Xu,Y.,Cabrerizo,F.J.,&Herrera-Viedma,E.(2017).Aconsensusmodelforhesitant fuzzypreferencerelationsanditsapplicationinwaterallocationmanagement.AppliedSoft Computing,58,265-284.
Currentaddress :DepartmentofMathematics,COMSATSUniversityIslamabadatLahore, Lahore5400,Pakistan
E-mailaddress : smbukhari735@gmail.com
DepartmentofMathematics,COMSATSUniversityIslamabadatLahore,Lahore 5400,Pakistan
E-mailaddress : atiqurehman@cuilahore.edu.pk
DepartmentofBasicSciences,UniversityofEngineeringandTechnologyTaxila, Pakistan
E-mailaddress : mariabibi782@gmail.com