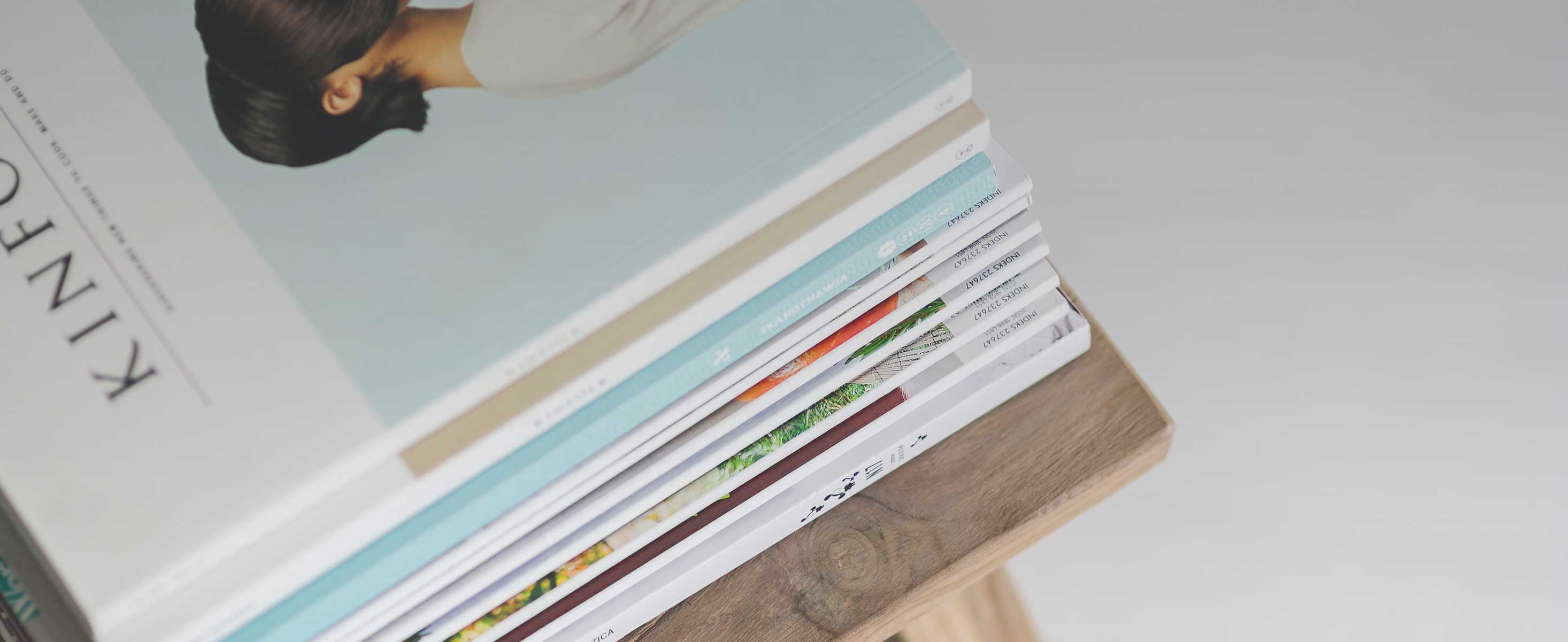
4 minute read
INFERENTIAL STATISTICS NON-PARAMETRIC TESTS
from Statistics Manual
by IFMSA-Egypt
Non-parametric Tests
When one of the assumptions of a Parametric Test is violated (especially Normality), it is not appropriate to use them anymore; you should use the Nonparametric Statistics instead. Non-parametric Tests are distribution-free and are used when the variables of interest are Categorical.
Advertisement
Also note, as established earlier, the less you know about the population, the less certain the results of your analysis become (since the non-parametric tests require less information than the parametric, classical, tests; they are always less precise).
Wilcoxon’s Signed-ranks Test
When the dataset is quantifiable but the variables themselves are ordinal or not normally distributed, the non-parametric Wilcoxon Signed-ranks Test may be used to obtain a confidence interval for the difference in population medians.

Wilcoxon’s Signed-ranks Test Hypotheses
1. H0: The median difference in the population is equal to zero.
2. H1: The median difference in the population is not equal to zero.
Wilcoxon’s Signed-ranks Test Example
The table below contains the results of a case–control study on the dietary intake of people with schizophrenia in Scotland.
It shows the daily energy intake of two dietary substances for the cases and the controls.
Interpretation:
1. The Protein Intake Median Difference CI includes 0 and p-value/sig. > 0.05: NO Statistically Significant Difference between the cases and the controls is noticed.
2. The Alcohol Intake Median Difference CI does not include 0 and pvalue/sig. < 0.05: A Statistically Significant Difference between the cases and the controls is noticed.
Mann-Whitney U Test/Wilcoxon Rank Sum Test
Used for comparing the same variable in two different groups. This test is the non-parametric alternative to the Independent T-test. The main difference is that the Mann-Whitney U Test is based on mean ranks (or medians), rather than the mean of the original values.
Mann-Whitney U Test Assumptions
Like the Independent T-test, the data must be obtained from two independent random samples for which we assume the original populations had the same variability.
Only the central tendency of the two samples is allowed to be different.
Mann-Whitney U Test Hypotheses
1. H0: The two samples come from the same population (same median).
2. H1: The two samples come from two different populations (different median).
Mann-Whitney U Test Example
This table is from a randomized controlled trial in an emergency department to compare the cost effectiveness of two treatments (ketorolac versus morphine) in relieving pain after a blunt instrument injury.
The table shows the median times (in minutes) spent by the two groups of patients between receiving analgesia and leaving the emergency department. The CI in the last column was derived by Mann-Whitney U Test.
Interpretation:
The Median Difference CI does not include 0: A Statistically Significant Difference between the ketorolac and the morphine groups is noticed for the study outcome: Interval between receiving analgesia and leaving the emergency department.
Kruskal-Wallis Test
Used for comparing the same variable in more than two groups. This test is the non-parametric alternative to the ANOVA. And note, like the ANOVA, it only tells us whether there is a difference between the groups, without telling us which.
Kruskal-Wallis Test Hypotheses
1. H0: All groups come from the same population (same medians).
2. H1: At least one group comes from a different population (different medians).
Kruskal-Wallis Test Example
Three groups of different ethnicities (Black, White, and Others) were tested using the Kruskal-Wallis Test to find whether their “happiness of marriage” scores differ.
Significance of the Results: Sig. = 0.000
Interpretation:
The Median Difference’s p-value/sig. < 0.05: A Statistically Significant Difference between the ethnicities is noticed for the “happiness of marriage” scores.
Spearman’s Correlation Coefficient
Used to measure association. This test is the non-parametric alternative to Pearson’s Correlation Coefficient as a linear association measure. The difference is that any (or both) of the variables is allowed to be ordinal or not normally distributed.
Spearman’s Correlation Coefficient has the same characteristics of the Pearson’s Correlation Coefficient but is usually expressed as ρs in the population and rs in the sample.
Chi-squared Test
Used to test for an association between two Categorical Variables. It gives a p-value with no direct estimate or confidence interval for the estimates. It needs a large sample. In the case of having only two categories, the expected frequencies should not be less than 5.
In the case of having more than two categories, the expected frequencies should not be less than 1 in all categories and no more than 20% of the categories can fall below 5.
Chi-squared Test Hypotheses
1. H0: There is no association between the two variables.
2. H1: There is an association between the two variables.
Chi-squared Test Example
A large US study was done to assess the impact of time to defibrillation after inhospital cardiac arrests on the incidence of cardiac events over hours. A chi-squared test produced a p-value of 0.013 (< 0.05). We can then say that we have Statistical Significance for a link between the incidence of cardiac events over hours and delayed defibrillation time.
Fisher’s Exact Test
Used to test for an association between two categorical variables. It has the same assumptions as the Chi-squared Test. However, unlike the chisquare test, the Fisher’s Exact Test is valid for small samples (where a chi-squared test produces a p-value that is too small).
Fisher’s Exact Test Hypotheses
1. H0: There is no association between the two variables.
2. H1: There is an association between the two variables.
Fisher’s Exact Test Example
The proportions of extremely preterm infants still on home oxygen at age 2 were categorized according to the mode of ventilation at birth: the high frequency oscillation ventilation (HFOV) versus the conventional ventilation (CV).
A Fisher’s exact test was done to test for an association between the mode of ventilation at birth and whether the infants are still on home oxygen. The test produced a p-value of 0.69 (> 0.05). That means that the results of this study are Not Statistically Significant and we do not have enough evidence to reject the null hypothesis.