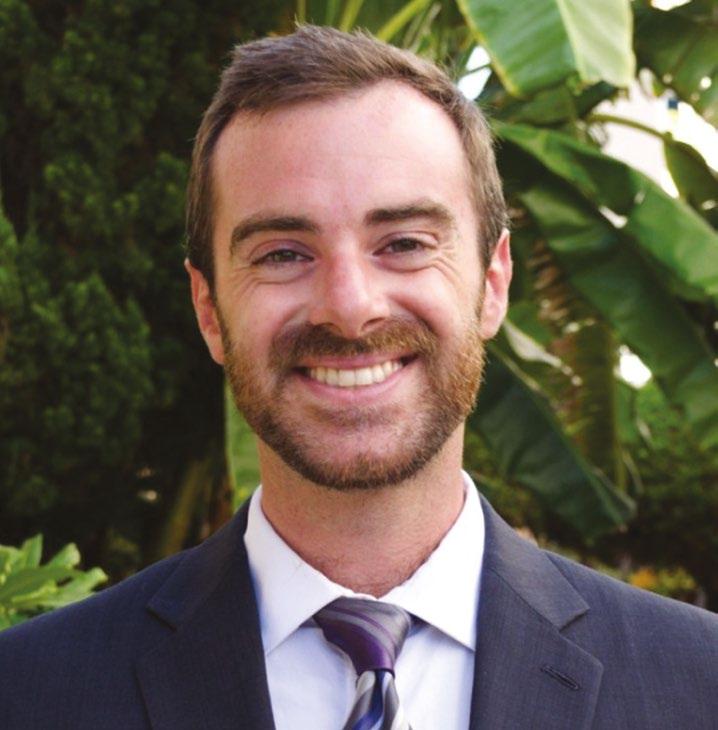
19 minute read
THE BIOLOGICAL MODEL
THE BIOLOGICAL MODEL
By PATRICK MCQUILLAN
PATRICK MCQUILLAN has a successful history leading data-driven business transformation and strategy on a global scale and has held data executive roles in both Fortune 500 companies as well as various strategy consulting firms.
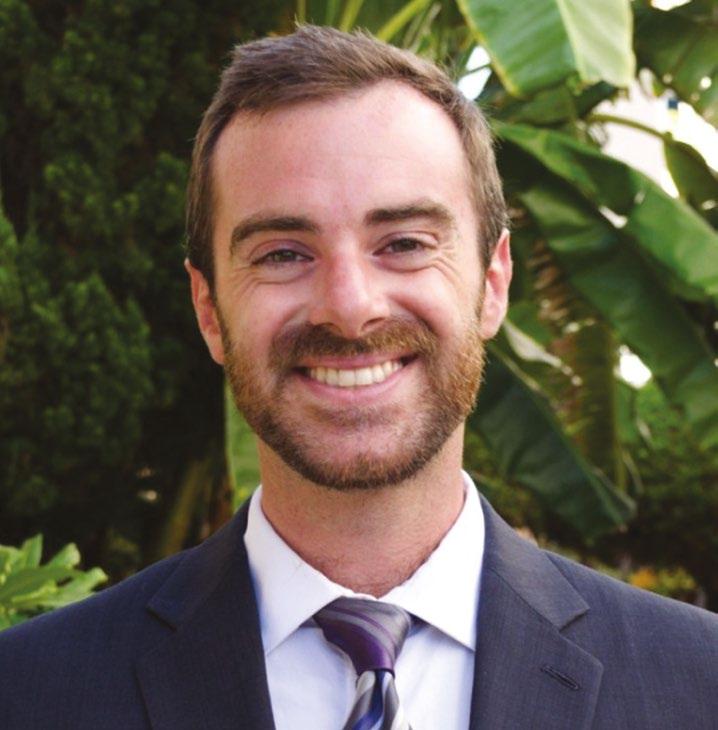
He is the Founder of Jericho Consulting and a Professor at Northeastern University and University of Chicago, where he teaches graduate programmes in Analytics and Business Intelligence.
How would you describe the concept of a ‘biological model’ that you developed in the context of data strategy and organisations?
It’s essentially the idea that all data, decision-making power, and resources should be consolidated into a single epicentre that’s connected throughout an entire business or organisation. It becomes a self-feeding system that can quickly react to, or predict, any anticipated challenges or bottlenecks based on what it’s historically encountered.
Crucially, the model continues to learn and provide an adjacency between access to data and the immediate access of that data by key decision-makers in
the organisation, rather than disseminating it to individual managers on different teams, and having decentralised analytics hubs or centres of excellence that might exist across different verticals that don’t communicate with each other.
What’s the difference between your biological model that functions like a nervous system, and centralised data structures that currently exist? For example, small or medium-sized companies tend to be centralised already, as they don’t have the capacity to decentralise everything.
Excellent question. The biological model is so named because it simulates the central
nervous system. The nerves collect information on what’s felt in the fingertips and internal organs, and automated processes like blinking and breathing; they’re similar to AI.
But the brain is the epicentre: the key decision-making component which processes the non-automated, conscious decisions like grabbing things, and influencing the world.
So when using the biological model, it’s crucial to have a robust data foundation. With this in place, the model rectifies two common issues encountered when using traditional models:
The first issue is that large companies with decentralised data centres can’t communicate with each other effectively, making it difficult to get the full picture
PATRICK MCQUILLAN
THE CONCEPT OF A BIOLOGICAL MODEL
quickly at the decision-making level.
Small and medium-sized businesses encounter a different problem. Although, as you pointed out, their data tends to be centralised, the nervous system may not actually be healthy because it isn’t collecting the right data, or the data it does collect isn’t a sufficient volume to make meaningful decisions. This can really affect the business’s success if, for example, they need to gain the edge over their competitors. Do they rely on faulty data to make that decision, or use non-data sources to help fill
those gaps?
That’s usually what happens when the system is technically working, but not necessarily flowing in the way it needs to: it’s not fluid. There may not be as many neurons or not enough information collected.
In terms of practical application, this could mean there aren’t enough data sources, or there’s not enough testing. If you’re trying to go to market, it might be you’ve rolled out a new marketing plan without testing in the right markets first, or without finding statistical significance in your results before branching out
CENTRALISATION VS DECENTRALISATION
What would be the advantages of centralising the data model for large organisations that are currently decentralised?
Typically, decentralised large organisations need to scale quickly, so they create different ‘centres of excellence.’ But these end up no centre of excellence at all. Supply Chain has its own vertical; Marketing its own vertical, Customer Service its own vertical. Consequently, the organisation ends up with all these different decentralised data centres. While this does operate to a certain degree of functionality within those verticals, in my experience I’ve yet to see a system like that work in the long run.
Individual leaders of those verticals might testify to their vertical’s effectiveness, but the people who report laterally to those leaders, or the people who they report up to, will always mention the knowledge gap. That’s because each leader is focused only on their lane, without understanding the wider context; how other silos may be impacted.
Centralisation is particularly important at the C-suite and board level, where leaders must report performance to shareholders and
make key decisions on a quarterly basis, or even on a monthly basis if something critical is happening across the organisation. Under the decentralised model, it takes the leaders about a month (and costs lots of money) to get all the FTEs on the ground to pull those reports, which are usually substandard to what they would be if centralised. The reports tend to be contextualised within the avenue of each particular vertical.
The benefit of having a fully centralised system is that, instead of having eight little brains each connected to different body parts, we have one brain collecting all our data, and making informed decisions from that data. Both the data and the decisionmakers are in one place - ready to send reports to the head of the organisation, and to loop all existing lines of data collection and communication into that central base.
and scaling that strategy.
Similar to supply chain optimisation (and anything happening on the op side), these companies may be getting ahead of themselves with a less-thanpreferred level of maturity for their data infrastructure: data’s going to the brain and being collected, but it’s incomplete. They may have too much information on the arm, but not enough on the leg. This creates a system that overcompensates in some areas and undercompensates in others, which becomes a difficult habit to break as you scale.
horizontal translation layer where their input is levelled out and presented easily to senior leadership. That person can also be a partner to senior leadership and the other vertical heads.
It’s a simple fix that costs as little as one additional leader and a small team of two or three. The team can develop a diplomatic relationship with each of these verticals, and set up a simple framework of data collection and reporting which keeps folks accountable, increases transparency, and significantly boosts the organisation’s efficiency, all with minimal disruption.
is that, instead of having eight little brains each connected to different body parts, we have one brain collecting all our data, and making informed decisions from that data.
You can still have the silos, but you need a leader in place: a CTO, a CIO, or some sort of VP of efficacy. The leader needs to take each of those verticals and create a
In your opinion, what’s a good way to convince leaders in verticals to share - to give up some of the ownership or insights that come from their data?
That’s a pain point many teams encounter, particularly in large
The benefit of having a fully centralised system
organisations. Often, they don’t want to relinquish control - not for selfish reasons, but because they’re concerned losing full control will impair performance of their vertical.
But in reality, there’s no actual relinquishing of control. It’s more a partnership with these different vertical heads, or with translators at the leadership level who say: your name is going to be on this report one way or the other, and it’s costing you guys $40,000 a month to chase down and pull these metrics together. That amounts to almost $500,000 a year per vertical.
Instead, why don’t we save ourselves a few million dollars, get a small team of three or four folks to stand this up, and present it as a partnership? You’ll have more time to innovate, more time to chase down projects, and fewer bottlenecks in your work stream if you let us assist with reporting. And again, your name is going to be on this, so we’ll be able to share this up to C-suite.
And C-suite won’t have to ask questions like: what is this and what’s happening? There won’t be any more unpleasant surprises. Instead, it’s going to be something wonderful happening with our name on it. Or if something suboptimal is happening, but we’ve already got ahead of it and we can speak in greater detail about the problem, maybe issue a report before this large meeting, or before these big reports go out to the C-suite.
So sharing verticals increases efficiency and creates more confidence. And whether something’s going well - or not as well - they always come out looking better, because if that thing was going to happen one way or the other, this partnership grants the capacity to anticipate the issue. There’s an external partner who understands what they’re working with on the reporting side, and how it will affect different verticals in the organisation.
For example, if it’s a supply chain issue and software engineering
should be included, they need someone who can help manage that relationship and both their work streams, so that Supply Chain doesn’t have to worry about Software’s work stream, and vice versa. There’s a third party to help them collaborate, and eventually roll up the same solution as normal, but better. It would be reported more quickly and with greater agility. The organisation would be saving money instead of chasing down reports and having people on the ground hopping off projects to issue ad hoc reports for senior leadership.
Ultimately, this impact for the organisation can be sustained over time, and can be scaled quite easily.
In the biological model, are there ways of sharing power, or keeping decision-making fluid and flexible at the same time while centralising?
I’d argue there’s no sacrificing of power or metrics whatsoever. It’s purely a partnership. To give a perfect example, an organisation I worked with in the past had about eight key verticals, and issues with decentralised reporting: no transparency, little accountability, and inconsistent and inaccurate reporting. So these verticals were doing their jobs, but they weren’t doing their jobs within the context of each other. They weren’t melding as well as they could have.
When I first joined and built up my team, the concern was that we would be taking the data away from them; that we would be sacrificing power or influence.
But that wasn’t the case. What we actually did was equip them with a framework to make their work look better. So they would be reporting the same metrics: we would not be taking over the calculation of those metrics. We wouldn’t be chasing down those metrics each day, because there might be 1300 KPIs at the organisation, and one team can’t know the narrative for each of those KPIs every single day.
Instead, we cleaned up how the KPIs were reported (you’d be surprised how many times in an organisation something as simple as ROI or cost per’s, are actually calculated differently across different verticals, but reported as the same thing upward). We called a meeting and basically said: what’s going to be our ultimate indicator here, what’s the definition we’re going to agree on? And we created an alignment.
From there, we created data dictionaries that tracked down the individual owners on each team of each KPI. So it’s clear who to speak to if there’s going to be an issue, if there’s a breakage, or an interesting trend happening. Who can we bring into a meeting with the higher-ups? Who in local teams might be working cross-functionally?
So we didn’t actually take over reporting. We imposed a governance structure to help secure their data and make their performance reporting more confident, more consistent, and keep the across verticals more effective.
So the way leaders presented their findings changed: my team would steer those meetings and we would have the KPIs categorised by division. We would report a simple trend, and incorporate the insights their team wanted us to incorporate, but we would hold standing business reviews weekly, monthly, or quarterly, depending on the audience. Usually weekly for each vertical, monthly for C-suite, and quarterly occasionally with the board or CEO.
Each of these VPs or SVPs would be sitting on that call, and we would let them speak to the narrative and share the story. But the VPs became more effective decision-makers because now they had another team, my team, that would be helping contextualise those metrics with their team, so they no longer had to constantly chase things down. We tell the story that they want to tell, and we put it in the context of
other folks’ stories. So it becomes a complete view of the business.
And most importantly, no data’s being sacrificed. It’s still owned by those teams. It’s just being filtered into a master document that our team is managing. And we’re not changing the values. The only values that could be readjusted are some KPIs, to ensure every team is calculating them, and reporting them, and speaking on them, in the same way with the same understanding - which ultimately makes everyone look good, and gives C-suite and board a lot more confidence to report outward to shareholders and make internal decisions.
centralised lakes on top of those existing lakes. So, you have this foundational layer where each vertical has its data sources already being compiled into, whether it be the cloud or a combination of cloud and manual spreadsheets. But above that foundational layer, we have a curated layer where nothing is being recalculated; it’s just we’re filtering away the metrics that we’re not concerned about at an executive level.
This might already cut 80, or 85% if we’re talking 1300 metrics or 2000 metrics. Maybe we want to whittle it down to the metrics that only C-suite or vice presidents - the senior leadership or above - care about. With this approach, the
or so to contextualise some of those. Maybe if you have a cost per customer service engagement when someone contacts a customer service centre, for example. But then you want to break that out into contextual metrics such as cost per call, cost per email, or cost per chat.
So we roll up those primary and secondary or contextual KPIs into a curated layer, which essentially sits at the base of the brain. This curated layer ideally exists on the cloud, but usually it’s on a combination of cloud, querying the individual cloud lakes they have in their own verticals, and pulling manually (or through email load-up) on a synchronised frequency from those partner teams any additional CSVs, or department-wide reporting documents that are useful to source additional metrics that might not be loaded into the cloud.
That makes a lot of sense. How does the biological model work from the technical side? Do you centralise physical data in one big data centre somewhere? Or keep the data physically decentralised, and just establish this virtual layer on top to funnel into insights and inform decision-making?
Data would be collected from different points, as you mentioned, and we don’t want to separate the hand from the rest of the body or mix it with another hand. So we want to make sure the collection epicentres - the data lakes that each vertical is managing - are as undisrupted as possible.
It’s best to avoid getting involved too deeply in having each individual team manage their backend data engineering, because usually that engineering has been inherited from many years of certain builds, and has certain rules in place. It’s a lot for one small team to manage, and it wouldn’t be valuable to fully transform that.
Instead, we create one or two
individual teams can focus more on the smaller metrics that matter in their day-to-day. It will filter those out, and then it’s just an extra layer of collection.
And again, to use our biological analogy, that’s to get it into the base of the brain by saying: okay, we can’t over-process a lot of information, so let’s just focus on what needs to be understood. Let’s focus on breathing. Let’s focus on blinking. Let’s focus on vital organ health. So what are the metrics that help us measure that? And what are the metrics that are going to drive the health of the body at a high level, at the key decisionmaking level? Rather than focussing on the health of a joint in the finger for that vertical, we want to make sure the body can breathe, absorb oxygen into the lungs, and maintain healthy functioning at a universal scale. Usually, those will be primary metrics and then what I call secondary or contextual metrics. So maybe you have, say 10 to 30 metrics, that will tell the performance of the entire business. You might add an additional 50
The result is a comparatively simple foundation of clean data that’s querying already clean data. But it’s rolling it up into a safe place that can be managed at an executive level without disruption, and some additional CSV polls for other data that might not necessarily be coming in. And then those get rolled up into overall reports and infrastructure discussions. So the technical side isn’t too complicated when compared with individual verticals.
In the curated layer, how would you establish having one source of truth in data that comes from different verticals? So, as a classic example, client or CRM data that lives in different parts of the organisations and is often conflicted?
One of the highest value aspects this function delivers is a single source of truth. And that comes into a strong data governance infrastructure. Most folks are focused on the model, or the outcome, or the narrative which is driving those decisions they’re going to be making. But (and this applies to all organisations at all levels) more attention needs to be
We imposed a governance structure to help secure their data and make their performance reporting more confident, more consistent, and keep the across verticals more effective.
paid to creating a sound and strong foundation of data.
What that means is imposing universal standards that are simple to put into place. They don’t require a lot of coding, engineering, or changing at the foundational layer level. These standards ensure consistent reporting at all levels.
The best solution I found is creating a data dictionary and an end-to-end management process for any sort of changes that happen in the way a KPI is calculated, or the way data’s being sourced, to ensure that the backend all the way to the front end can manage and adapt to those changes.
So the data dictionary side, for example, is saying: over time we can focus on cleaning up these 2000 KPIs, but for the main ones that we’re trying to report at an executive level - these 100 KPIs that are most important for different levels of reports - for those KPI’s, the most important thing is to have conversations with leaders and say: alright, we have eight verticals. All eight verticals rely on different variants of ROI, but they get rolled up into a large ROI number. So let’s sit together and walk through the calculations, and let’s bring the folks who make those calculations into the conversation.
That can be a standing meeting twice a month for two months - very simple, half an hour of everyone’s time. And in these meetings, we say: okay, how are we calculating this? Let’s all calculate it the same way. This is obviously easier said than done; sometimes you might have to break it up into two KPIs or three KPIs, but it’s better than having seven or eight different versions, and you can rename them and contextualise them.
So it’s getting that alignment on what you’re trying to report upward on. It comes back to that partnership agreement; trying to horizontalise the
conversation where you get that buyin from leadership and say: this is going to help all our collective bosses. It’s a quick adjustment that’s going to help everybody, and it can be done without disrupting previous reporting.
But it’s better than just creating more KPIs, which, frankly, I think is more disruptive. Instead, we retire ones we don’t need, creating a consolidated list of fully aligned KPIs on their definition from a layman’s term, their calculation and their individual owners. So, if there’s a KPI being managed by say, the North American team and there’s a team in the EU that has it from a different data lake or a different centre, they
and paste; and the owner.
In this way, the dictionary creates a one-stop shop. It’s something that takes a little while to buildmaybe three or four months. But if it’s being maintained, it lives in the organisation forever. And it’s extremely good for performance reporting, and a great reference when folks are using self-service dashboards to understand metrics more fluidly and with less translational error.
Also, the end-to-end process is about connecting those individual KPI owners. Whenever there’s an issue such as a data blackout, or a change to a KPI because some reporting rule has been shifted or the data that’s being collected has changed, there are recurring meetings from a small subset of those groups.
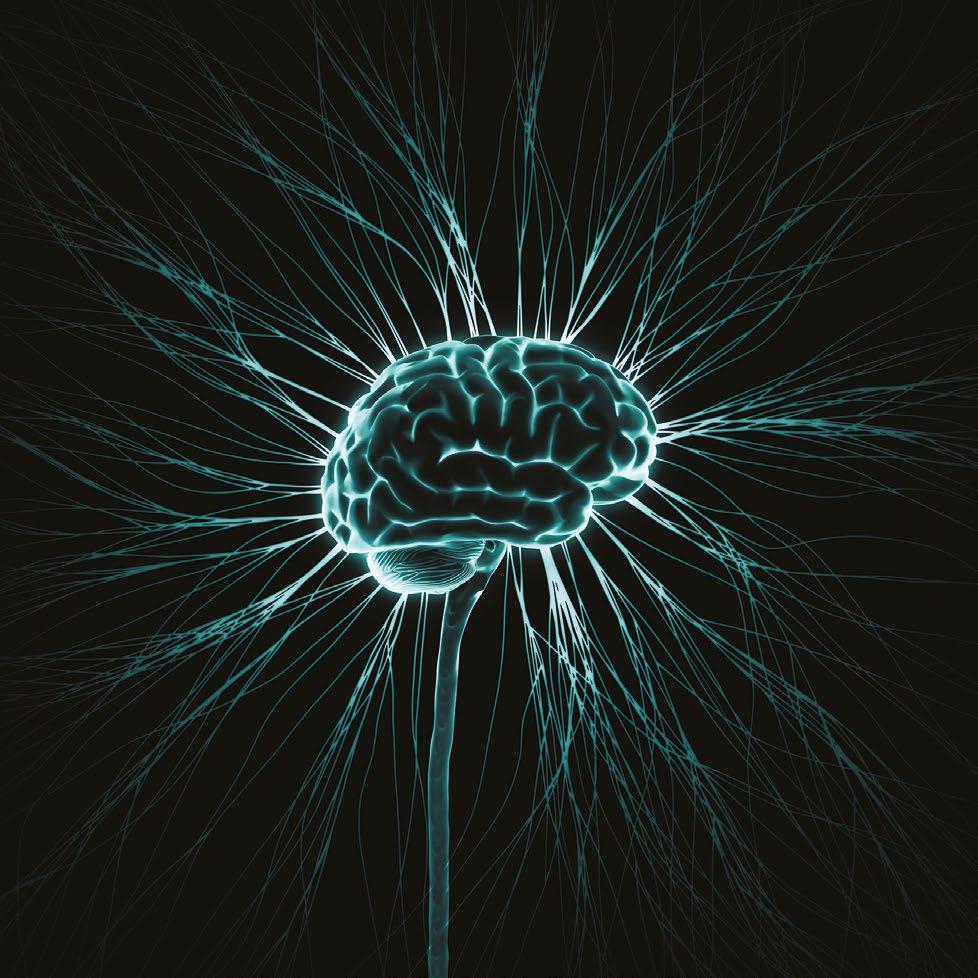
try to approximate that calculation as best they can. That way, we can have a breakout that’s as close to one-to-one as possible, and have a leader in the EU team, and the leader in the North American team, who can speak to that. And we have that in place for each KPI.
So this dictionary would consist of: the name of the KPI; the team or teams that manage it from an actual calculation perspective; the location of where it can be found from a cloud or data lake standpoint; the layman’s definition; a technical definition; the SQL code or similar code that’s used to calculate it which folks can copy
So in the past, I’ve led those meetings with just the managers or the analysts: a handful of people who actually pull those metrics. They’ll have recurring meetings, and they’ll mention if something’s changing. And all we have to do is update the dictionary, and then we connect with whoever’s being affected by the issue. So if there’s going to be a 24-hour data blackout that will affect Marketing, for example, and they’re not going to have access to their complete marketing data, we can inform them ahead of time.
And while the engineering team is updating that, our team can partner with them on how to mitigate problems while we’re waiting for the new solution. So there’s no loss of efficiency, money, or time from the backend update through to the front-end team that’s actually working with that information. It’s basically about having a responsible hand in the management of that information from end to end, top to bottom, and horizontally.