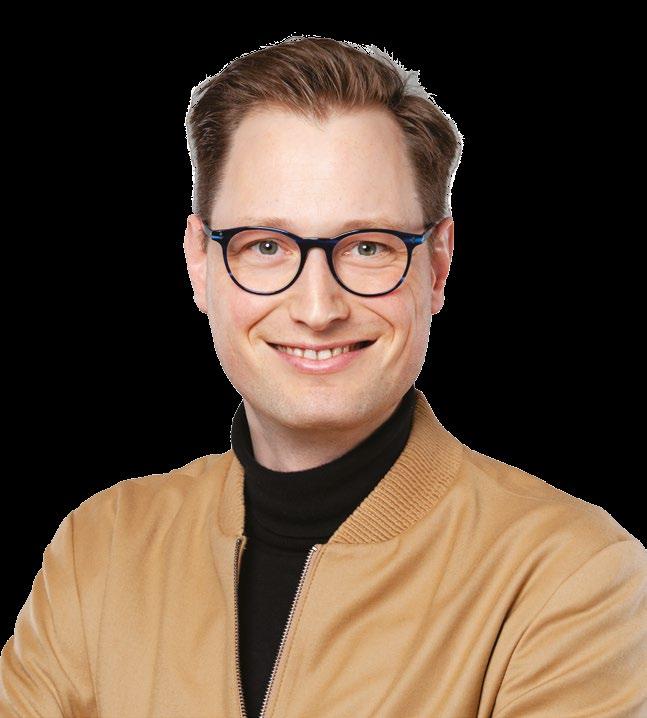
17 minute read
FROM INTUITION TO INTELL IGEN CE: CRAFTING AN EMPOWERED DEMAND FORECAST
FROM INTUITION TO INTELL IGEN CE: CRAFTING AN EMPOWERED DEMAND FORECAST
A JOURNEY INTO THE WORLD OF DATA-DRIVEN PLANNING: NAVIGATING THE PATH TO PRECISE FORECASTS
DR ALEC SPROTEN is a renowned data science expert and the Head of Data Science at E. Breuninger GmbH & Co., the leading premium and luxury department store chain in the DACH region. With a diverse background in psychology and a Ph.D. in economics, Alec brings a unique perspective to the field of data science. Previously, he served as an Assistant Professor for Economic Theory, conducting research on social norm compliance. Alec has also held the role of A.I. Evangelist for STAR cooperation and has extensive experience in strategy and management consulting, specialising in after-sales pricing. Currently, he manages a large Data Science Centre of Excellence, overseeing a team of talented Data Scientists and product managers. Alec’s expertise lies in leveraging data-driven insights to drive business strategy, innovation, and customer-centric solutions.
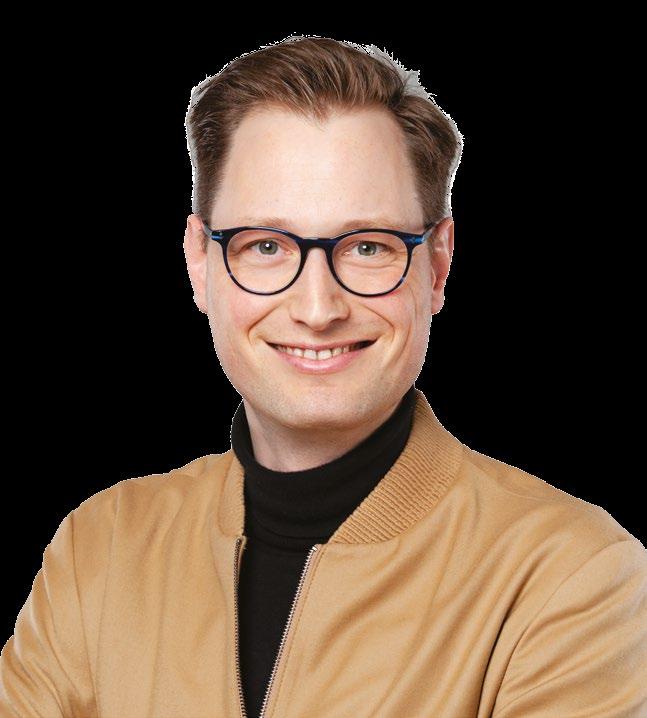
IRIS HOLLICK , a Data Science Product Owner, is on a mission to empower Breuninger with data-driven excellence by spearheading transformative Data Science projects. With a strong foundation in demand forecasting and strategic marketing, coupled with her adeptness in project management, she excels in orchestrating international teams by having an extensive global experience.
Iris’s expertise lies in orchestrating data-driven initiatives across diverse technical landscapes, and maximising the value of data science implementations. She is a distinguished academic, holding a Master’s Degree in Technical Mathematics with a specialisation in Economics from Vienna University of Technology, complemented by an MBA in Project Management.
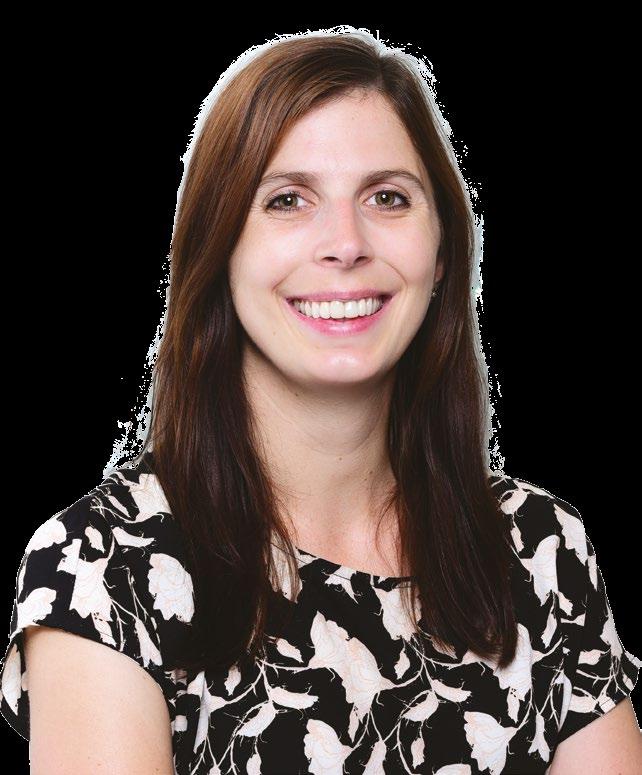
EMBRACING OUR ORIGINS
As the leading luxury department store chain in the DACH region and beyond, E. Breuninger GmbH & Co. faces a range of challenges demanding data-driven solutions. To meet these challenges head-on, the Data Platform Services (DPS) unit, a part of the IT department, brings together expertise in data engineering, data modelling, data science, and data product management. Established four years ago, DPS emerged to bring a structured, data-first approach to Breuninger’s operations. Since its inception, the unit has continuously grown and now manages a data lake brimming with up-to-date raw data from vital source systems like ERP, logistics, and the online store.
In conjunction, a business pool has been curated, housing business objects that empower self-sufficient utilisation, as described in Tarush Aggarwal’s article in Issue 3 of The Data Scientist This reservoir of insights serves various purposes, with one notable application being the creation of an integrated buying and planning solution. This solution acts as a guiding light for
the merchandise department, illuminating the path to informed decisions that align seamlessly with customer preferences.
At the core of this approach lies a meticulously detailed customer demand forecast. This forecast serves as the foundation for merchandise management, encompassing stock modelling, order management, assortment curation, and financial planning for both new product introductions (NPI) and perennial stock items (NOS, neverout-of-stock). With a vast catalogue of several hundred thousand stockkeeping units (SKUs), this intricate orchestration is adjusted on a weekly or even daily basis, covering an extensive 18-month time horizon.
Driving the technical part of the implementation is a supply chain management service provider. This collaborator is tasked with the technical realisation of the project: crafting a user platform, developing the forecast and its plug-ins in the software, and addressing the nuanced, Breuningerspecific requirements integral to the project’s success. Guided by an agile project management approach, the process unfolds in iterative stages. The initial emphasis revolves around refining the forecast and optimising software performance, all centred around a prototype product group - a strategic launching point for an innovative journey.
ERECTING THE FOUNDATION
Now, let’s delve into the fundamental requisites of the customer demand forecast. It’s a dynamic landscape, encompassing a multitude of critical factors, including sales transactions, prices, promotions, opening hours, customer data, and extending all the way to macroeconomic and market data. The demand forecast we’re crafting operates at a high level of granularity, working with SKUs, demand locations, and week granularity, all spanning a substantial 18-month window. With each weekly forecast run, our
aim is steadfast: to furnish reliable outcomes, backed by the latest insights and employing precise algorithms and methodologies.
As we navigate the complexities of forecasting for a distinguished upmarket department store, two primary challenges come to the forefront: intermittent sales patterns and fleeting product lifecycles. Our inventory thrives on fashion items, which enjoy brief moments in the spotlight, heavily influenced by pricing and promotions. But the journey of product management commences even earlier, with crucial decisions around granularity and master data structure. These foundational choices ripple through every subsequent development, shaping the trajectory of the entire venture.
The first milestone on this path was carving out our demand location structure. Considering the inventory’s lens, Breuninger’s warehouse configuration mirrors distinct stores and an e-shop arm for sellable wares. In light of this, we opted to define demand locations pertinent to forecasting by blending warehouse and channel considerations. This initial structural choice is a reminder that the granularity of your demand location directly impacts forecast quality. Yet, this granularity must harmonise seamlessly with the customer demand structure, ensuring its efficacy across diverse use cases.
The subsequent key definition, one wielding substantial influence over forecast accuracy, centres on the product master. Intermittent sales patterns and the prevalence of NPIs with their transient lifecycles present the main challenge. To address this, we’re driven by the pursuit of delivering forecasts with elevated granularity. Employing a progressive profiling technique, generating forecasts at a higher
product level is poised to yield superior outcomes. To facilitate profiling and comprehensive analysis, our product master data
furnishes a spectrum of levels and hierarchies, ranging from the nittygritty SKU specifics up to broader product groupings (such as shoes or jackets) and even further to encompass department hierarchies (like womenswear).
In the face of NPI challenges, our focus shifted to enriching the product master with data attributes tailored for forecasting. Quality data pertaining to standard department store attributes such as colour, size, and customer demographics, alongside product-specific fashion dimensions like heel height and scarf length, stand as sentinels of precision within the realm of retail business. By imbuing the forecast with these rich attributes, we ensure our accuracy is resolute, even amidst the nuanced landscape of fashion’s ephemerality.
UNVEILING THE TIME SERIES: A JOURNEY INTO UNIVARIATE DEMAND FORECASTING
With demand locations and a refined product master in place, our focus turned to the bedrock of our endeavour: constructing a comprehensive time series. Envisioning the diverse applications of the customer demand forecast and embracing the existing demand location and product structure, we embarked on a journey to create a time series based on weekly sales transactions, quantified in units. The time series mirrors the desired output granularity, encapsulating SKUs, demand locations, and weeks or days.
This canvas was adorned with every form of transaction that captures customer demand - a rich
PHILIPP KOEHNBREUNINGER
[An integrated buying and planning solution] acts as a guiding light for the merchandise department, illuminating the path to informed decisions that align seamlessly with customer preferences.
tapestry that transcends traditional sales. Notably, for online sales, we considered the order creation date rather than the invoice date, while for product trials (a Breuninger customer service benefit), we tracked the moment of withdrawal. A pivotal choice was the omission of customer returns: retailers, especially in the online realm, grapple with a considerable return rate, one that should remain excluded from the “pure customer demand forecast” aimed at merchandise management or financial planning. However, for use cases involving stock modelling, returns could not be ignored. Thus, a parallel return forecast
materialised, rooted in historical returns and correlating with the customer demand forecast - more on this shortly.
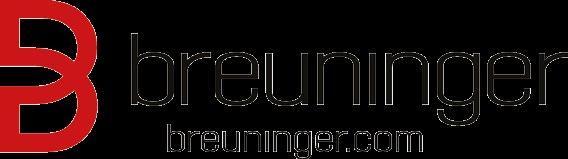
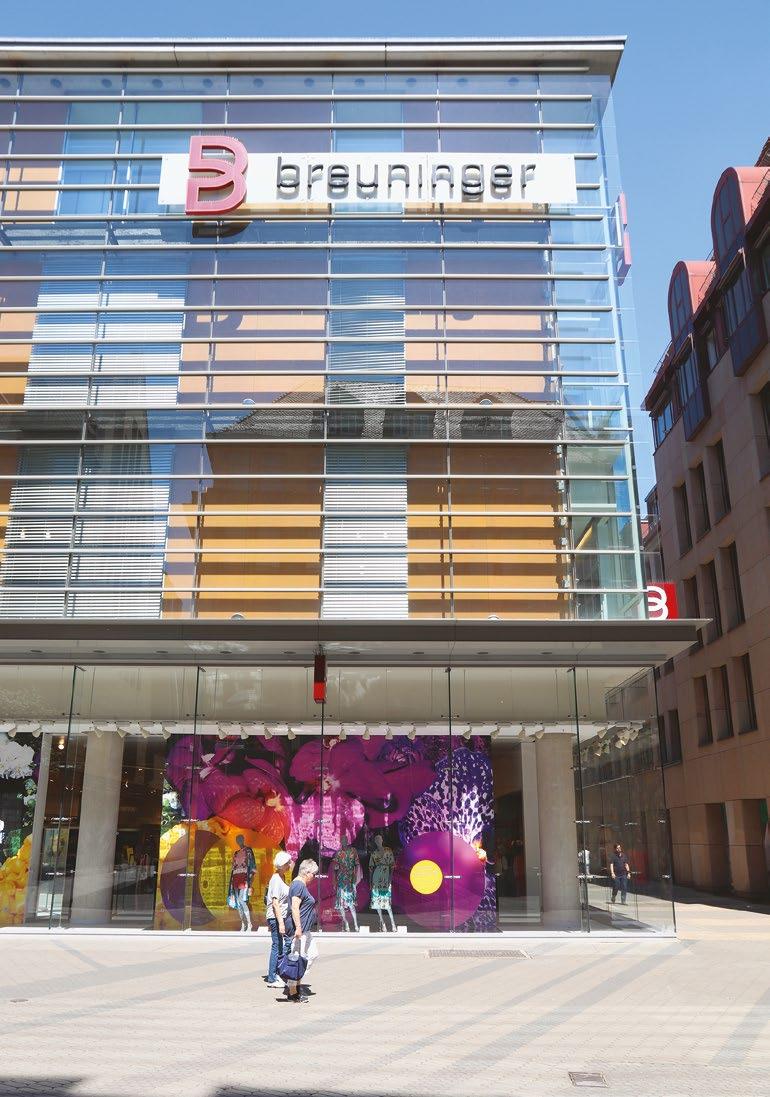
Having forged our time series, the next cornerstone was assortment information. This repository essentially outlines the items earmarked for forecasting, their horizons, and designated demand locations. This data isn’t solely about forecasting; it casts a long shadow on future forecast accuracy. The assortment must harmonise with the overarching business strategy, accounting for launch dates and avoiding confusion with the purchase or production planning periods, which factor in lead times. The goal, always, is to forecast customer needs, understanding that sales are the linchpin. Granularity depends on nuances like simultaneous product launches across all stores or location-dependent launches, the sequence of product group releases, and the timing of alterations. We prioritised a robust level of granularity to ensure flexibility. With our time series in hand and guided by assortment information, refining our results in the
realm of retail meant rectifying outliers and mitigating stock-outs. Understandably, when products aren’t available, sales naturally taper off - this phenomenon affects both in-store and online scenarios. We took a savvy approach by employing sellable stock in each demand location as a reference for stock-out rectification. Naturally, the lowest granularity, in our case, SKU/store level, is pivotal here. Yet, luxury department store dynamics introduce challenges due to high intermittency, heightening the risk of redundant data cleansing. As a starting point, we employed a stock-out cleansing strategy that focused on filtering out very low sellers and identifying zero stocks, which we then corrected using diverse interpolation techniques. To address general time series outliers, we employed seasonal interquartile ranges and sigma methodologies. Finally, with these foundational steps in place, we advanced to construct our maiden forecast - a univariate forecast. During exploratory data analysis (EDA) and rigorous testing, key performers emerged, including tbats, croston, prophet, simple moving average, arima, alongside rule-based inductions. Further enhancing the decision-making around algorithm application, we incorporated a segmentation logic based on sales volume and variability. For our initial release, we seamlessly integrated these models into our weekly batch run, employing best-fit logic by comparing WMAPE scores. Nevertheless, these model selections bear a strong dependency on article types and granularity levels. Recognising this, we initiated a product profiling method based on insights garnered from EDA. Yet, the landscape remains fluid; we’re acutely aware that as time progresses, fresh evaluations will steer our course. When addressing granularity, considering the sporadic
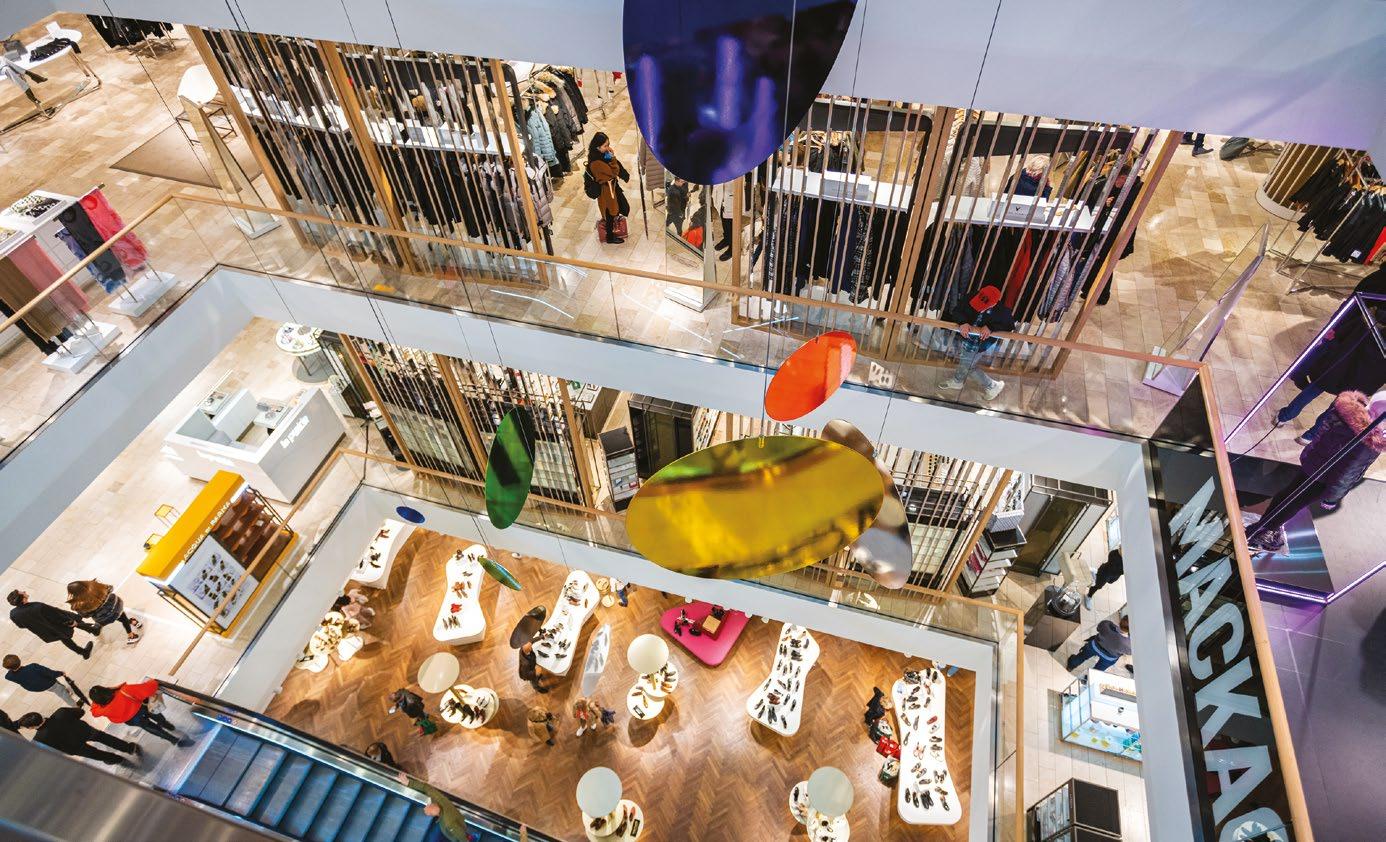
nature of demand, we recognised that forecast level and output level might diverge. Thus, we embraced a higher forecast level based on optimal results per product family and deployed profiling techniques centred around product, location, and time granularity requirements. For instance, to perform stock modelling on a daily level, we channelled past daily data for proportions, factoring in operational hours, promotions, and other variables.
HARNESSING THE MACHINE: Crafting the machine learningdriven tomorrow
Until now, our journey was paved with the creation of a robust univariate statistical forecast - a reliable companion for NOS articles. Yet, recognising that the realm of fashion predominantly thrives on NPIs, we ventured into the heart of our endeavour: constructing potent multivariate and machine learning (ML) models, a vital step to satiate the demands of NPI forecasting.
As the ensemble of these models unfurled, we first fortified them with the bedrock of driver data. Echoing back to our earlier discussions, we infused these models with the richness of product-dependent attributes and fashion dimensions. Steering forward, we ventured into the realm of prices and promotions.
Undoubtedly, the dominion of prices, especially regular retail and reduced prices, holds profound sway over retail enterprises. Markdowns and sales periods wield unparalleled influence over overall demand, thus rightfully claiming their place within the forecast fold. To distil these drivers, we considered historical price and markdown data, blending it seamlessly with future projections. Notably, markdown strategies planned over extended horizons can yield significantly refined results, encompassing both the immediate and distant future.
A similar narrative plays out with the inclusion of promotion
data. The efficacy of your forecast hinges on the availability and quality of your promotions data. In our scenario, we delineated between article-based, customer-based, and location-specific promotions. These categories might at times overlap (as in the case of brand events), necessitating meticulous structuring to prevent duplication. Just as with prices, a comprehensive promotions dataset includes both historical and future data.
Holiday and special events data also found a place in our pantheon of drivers. While sales during these periods naturally manifest in the demand time series, delivering explicit event information remains pivotal, particularly for weekly forecasts where weekly structures might diverge. Depending on the product and customer groups, macroeconomic and sociodemographic data could also augment forecast accuracy. Even weather data, offering insights into store performance during distinct weather conditions, was factored in. And in a retail setting, the inclusion of customer data, centred around behaviour patterns, added another layer of depth. This data encompassed a spectrum of aspects, from evaluating sales across diverse target groups and customer profiles to tracking online interactions.
Armed with quality driver data, product attributes, and fashion dimensions, we charted onward to construct the multivariate and machine learning forecasts. Given fashion’s inherent dynamic of NPI prevalence, ML forecasts emerged as the stalwarts of our strategy. Guided by insights drawn from EDA and the outcomes of previous data tests within the initial project scope, we forged ahead with LightGBM and Catboost - champions that exhibited the most promising results. Similar to our approach with the univariate forecast, our compass guided us towards what worked best for a given product family at a particular juncture in time. Adaptability
remained our watchword.
With the univariate and ML forecasts in hand, the final lap encompassed the application of best-fit logic, diligently seeking the pinnacle of results for our weekly outputs. This orchestration, the culmination of our arduous journey, was more than a mere computational endeavour; it encapsulated our need for precision, customer insights, and the ever-evolving landscape of retail dynamics.
BEYOND THE HORIZON: Envisioning beyond demand
The journey towards an allencompassing planning tool transcends the creation of a sole demand forecast - it’s a symphony orchestrated by numerous considerations. In the realm of online shops, a formidable challenge emerges in the form of returns. To cater to this complexity, fashioning a return forecast becomes imperative for use cases like stock modelling and financial planning. Pioneering a nuanced approach, we birthed a distinct time series rooted in returned transaction data, capturing the original sales juncture. By integrating past sales, we paved the way for return probabilities to surface across various granularities - ranging from transaction specifics to broader product family categories, grounded in statistical significance. In practice, these probabilities intertwine with actual sales and the demand forecast, forging a projection that spans the entire forecast horizon. To fortify this approach, machine learning models were harnessed to analyse attributes that exert influence on customer returns - attributes such as material type or patterns that unfurl diverse facets of return dynamics.
Venturing further, the landscape widens with a kaleidoscope of potential forecasts. For instance, catering to aged stock consumption enters the fray. As time elapses, old stock necessitates calculated consumption trajectories, rooted
in comprehensive insights to avert wastage and optimise resource allocation. Additionally, projecting into the future, we contemplated estimations on forthcoming prices, spanning a lengthier horizon. This realm demands an intimate understanding of market dynamics, the intricate dance between supply, demand, and the economic currents that shape them.
data’s integrity.
In the grand tapestry of crafting an all-powerful planning tool, these supplementary forecasts and enrichments emerge as keystones. Each forecast dances in harmony with others, their outcomes intertwined with the symphony of customer needs, industry trends, and the ebb and flow of retail’s everevolving tide.
ILLUMINATION THROUGH INSIGHT: Monitoring Progress, Measuring Success
The bedrock of data-driven planning lies in the bedrock of stabilityrendering a forecast that’s both unwavering and accurate. In our pursuit of this essence, vigilant monitoring and continuous measurement of progress emerged as indispensable components, woven into the very fabric of our project. In this journey, the lifeblood of stability resides in actual sales data and assortment data. Recognising their pivotal role, we embarked on a dual-tiered monitoring approach. The first layer, a technical vigil, stands as a sentinel, safeguarding the daily transmission of data. Beyond the technical dimension, our scrutiny deepened to the very content being transmitted, guided by a litmus test of time series quality and the count of active items - a preliminary checkpoint ensuring the
Yet, it’s the performance of our forecasts that takes centre stage in this narrative. A mosaic of key metrics - WMAPE, Bias, R², and MSE - paint a comprehensive picture of forecast efficacy. In our iterative pursuit of forecast enhancement, these metrics unfurl in tandem with the development of the project. As we weave new drivers into our equation and experiment with diverse models, this ongoing evolution provides a dynamic canvas for comparison. Our goal: to etch a clear demarcation between forecast errors before and after introducing new drivers, or venturing into uncharted models.
As these metrics oscillate and trends emerge, they form the basis for informed decisions, steering our journey towards refined forecast models. This perpetual dialogue between insights and outcomes, driven by vigilant monitoring and meticulous measurement, crystallises our commitment to not only delivering a stable and accurate forecast but also perfecting it over time.
A DESTINATION, A BEGINNING
In the intricate tapestry of retail, where dynamic demands and ever-evolving trends interlace, our expedition from best guesses to an all-powerful demand forecast has unveiled a roadmap of innovation. Anchored in the ethos of data-driven precision, our journey has been an exploration of art and science, where meticulous methodology marries the artistry of fashion’s ephemerality.
From the inception of the Data Platform Services (DPS) unit to the meticulous construction of our univariate and multivariate forecasts, our narrative has unfolded in layers. The first steps, framed by the need for refined granularity, led us to erect a robust foundation -
the demand location and product master. Through these pillars, we harnessed the potency of time series construction, nurturing it with sales data, assortment insights, and an unyielding resolve to rectify outliers and stock-outs.
With our gaze fixed on the fashion horizon, we ventured into the realm of multivariate and machine learning models, where prices, promotions, and even weather danced as drivers. This strategic dance was underpinned by a profound understanding that each attribute, each thread, wove together the tapestry of forecast accuracy.
But our voyage was not merely about crafting forecasts; it was about weaving a comprehensive tool for planning. Encompassing return forecasts, exploring aged stock consumption, and even peering into the crystal ball of future prices, our endeavour transcended singular predictions. It embraced the entire spectrum of retail dynamics, manifesting a comprehensive toolkit for precision decision-making.
As we navigated these waters, the vigilant pulse of monitoring and measurement echoed, providing a metronome to the rhythm of our progress. Key metrics like WMAPE, Bias, R², and MSE stood sentinel, guarding the threshold between forecast iterations and allowing us to meticulously mould our predictions into refined instruments.
Through these layers, we emerged with an unshakable truth - our journey is not an endpoint, but a stepping stone. The evolution of retail is ceaseless, and so too must be our commitment to innovation. As we unveil our unified demand forecast, a beacon of precision and insight, we do so with the awareness that it is a testament to our dedication, adaptability, and the indomitable spirit of data-driven transformation. Our voyage is a testament that, while retail’s horizon may shift, our resolve to chart its course stands unswerving.
Anchored in the ethos of data-driven precision, our journey has been an exploration of art and science, where meticulous methodology marries the artistry of fashion’s ephemerality.