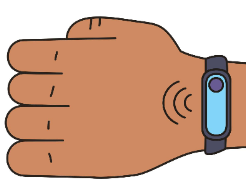
6 minute read
CAN WEARABLE TECH AND AI IMPROVE TREATMENT FOR BIPOLAR?
Early Detection, Early Intervention: Can Wearables and Artificial Intelligence Improve Treatment for Bipolar?
By Dr Filippo Corponi
In this month’s Research Corner, Dr Filippo Corponi, a psychiatrist and PhD student at University of Edinburgh, explores how combining wearable devices and AI for bipolar management is a growing area of interest, and the challenges to this becoming a part of the bipolar treatment pathway.
Wearable devices (‘wearables’), such as smartwatches and fitness trackers, have become increasingly commonplace over the past decade. They come equipped with sensors to capture physiological data including activity levels, sleep patterns, heart rate variability, and sweat response of the wearer. Thanks to their unobtrusive and continuous data collection capabilities, wearables are emerging as powerful tools for monitoring various aspects of health and well-being, and are extending healthcare beyond the doctor’s office. The collection of daily data from wearable devices for this purpose is known as ‘personal sensing’. Blood glucose management in diabetes and remote monitoring of Parkinson’s disease are the first applications of wearables recommended by the National Institute for Health and Care Excellence (NICE) in the UK.
HOW MIGHT WEARABLES IMPROVE BIPOLAR MANAGEMENT AND TREATMENT?
Episodes of bipolar feature disturbances in mood, sleep, and energy levels, which are reflected by changes in physiological data. Wearables may therefore be particularly useful in this condition, complimenting current approaches which rely on intermittent inperson appointments and self-reported symptoms. For a person with bipolar, this could mean detecting early signs of a developing mood episode, such as changes in sleep, heart rate and the amount a person is moving. As patients sometimes may not be aware that an episode is underway, early, objective, detection enables timely access to treatment, thus minimizing disruption to a person’s life and improving outcomes. This new type of monitoring could enhance ordinary clinical observation, especially at a time when the mental health workforce is shrinking in the face of rising demand, in the UK and globally, and access to specialized care has reduced.
WHAT ARE THE TECHNICAL CHALLENGES WITH WEARABLES AND ARTIFICIAL INTELLIGENCE FOR BIPOLAR?
One issue with using wearables in healthcare is the fact that processing and analysing large amount of data is very time-consuming and complicated. One solution to this is Artificial Intelligence (AI) - a a set of methods that enable computers to extract patterns and learn from large volumes of data. On the back of recent advances in AI techniques to analyse data from wearables, enthusiasm around healthcare personal sensing has grown. However, researchers have found that training an AI to detect the occurrence of bipolar episodes from physiological data is difficult. Some scepticism therefore crept in: will wearables eventually be counted among the undelivered promises in mental health care, as with psychiatric genomics and neuroimaging? Indeed, in both cases, decades of research have, as of today, failed to deliver any clinical decision-support tools, and wearables are yet to be implemented in the daily psychiatric practice. So what are the main challenges when it comes to combining wearables and AI detection of bipolar episodes?
CHALLENGE 1: HOW TO ‘LABEL’ LARGE AMOUNTS OF WEARABLE DATA
Traditionally, AI learns from massive amounts of ‘labelled’ data. Simply put, this means data is presented to the AI with an explanation already attached –this data was collected from someone in a manic episode, but that data was collected from someone in a depressed episode. The AI can then learn what ‘manic episode’ data looks like compared to ‘euthymic’ or ‘depressed episode’ data and identify them in new data. Attaching labels to data from wearable devices is resource-intensive and requires people with clinical experience such as doctors to do the labelling. This limits the amount of data available to train the AI, and ultimately the accuracy of the predictions that the AI can make.
A newer approach called “self-supervised learning” gets around this. It uses unlabeled data, which means the wearable data doesn’t need to be ‘explained’ before giving it to the AI. Here’s the trick: the AI is given incomplete data (like a missing puzzle piece) and has to guess the missing part. This method could help to solve the issue of limited amount of labelled wearable data from people with bipolar.
CHALLENGE 2: DETECTING PHYSIOLOGICAL CHANGES DUE TO A MOOD EPISODE IS DIFFICULT BETWEEN PEOPLE
A second challenge is that our physiological functions are constantly changing dayto-day, and any changes from a bipolar mood episode might be relatively small and hard to detect. This ‘bipolar-related signal’ in wearable data is also very different between people, because bipolar episodes can manifest differently across patients and even within the same patient over time. Developing an AI that reliably detects different bipolar episodes across different people is difficult, and will need to include ‘tunable’ features to personalize the episode detection.
CHALLENGE 3: WE NEED TO FIGURE OUT THE BEST WAY TO COMBINE DIFFERENT TYPES OF WEARABLE DATA
Lastly, wearables record multiple types of physiological data, each having its own dynamics. For example, heart rate variability is unreliable when someone is moving around a lot, so it is better to look at heart rate data when someone is asleep and moving less. Careful consideration needs to be given as to how to combine the different types of data in the best way to detect bipolar mood episodes.
WHERE DO WE GO FROM HERE?
While the use of wearables for enhanced monitoring in bipolar is promising and the argument for it compelling, some technical challenges need to be overcome before this technology is rolled out. Preliminary research shows a good level of acceptability for wearables in people with mental health conditions, but we need more engagement with people with bipolar to make sure wearables are a feasible, and helpful, approach.
A CALL TO ACTION - WHAT DO YOU THINK?
As a psychiatrist for the NHS and a PhD student at the University of Edinburgh, I appreciate the potential that personal sensing has to revolutionize how bipolar is managed, but I also identified areas which need further research. I am passionate about making a difference for people living with bipolar, and believe personal sensing is one way to do this. That is why I am seeking your insights! If you have lived experience with bipolar, I would be grateful to hear your feedback, comments, or questions, about AI, wearables and personal sensing so that your views are reflected in my work. You can contact me via email: filippo.corponi@ed.ac.uk
Research Corner is coordinated by researchers from the University of Edinburgh. If there is a topic you would like to find out more about, or if you have suggestions for this section, please get in touch! You can contact Amber Roguski at:amber.roguski@ed.ac.uk