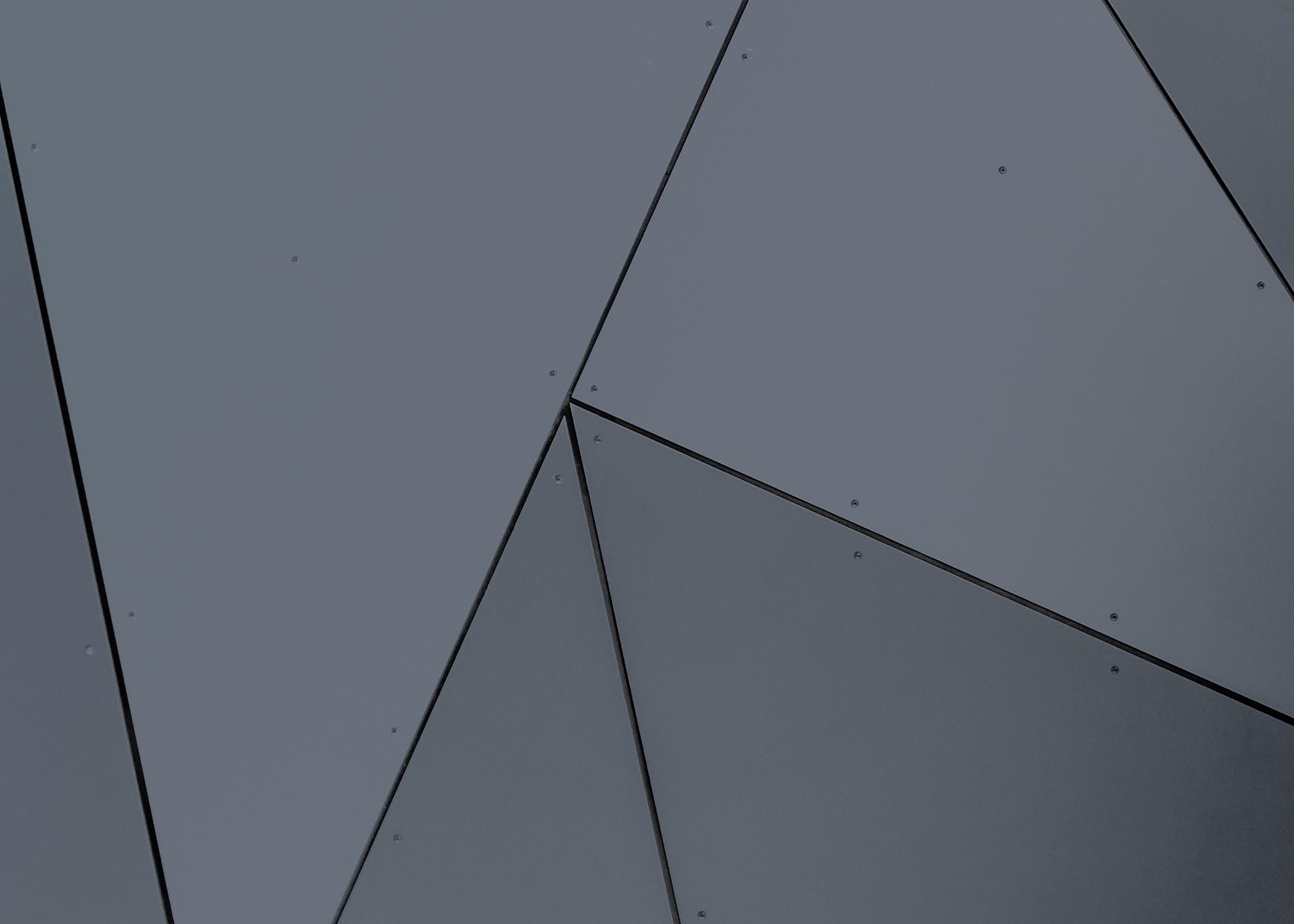
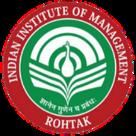
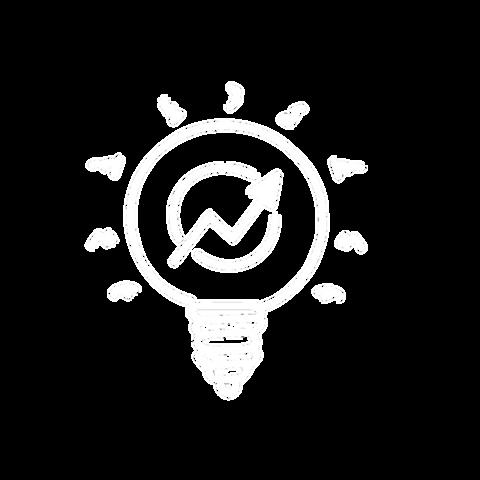
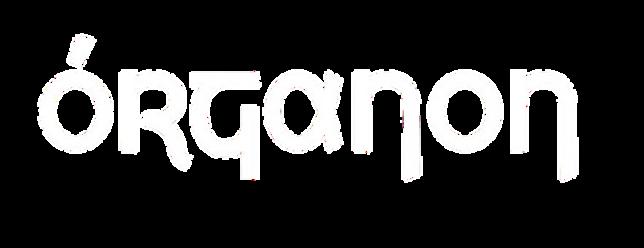
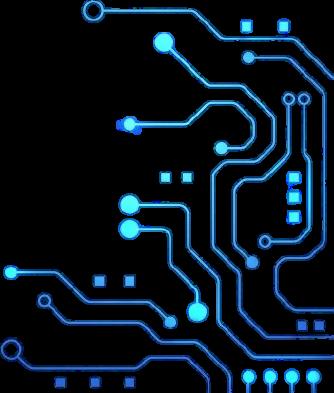
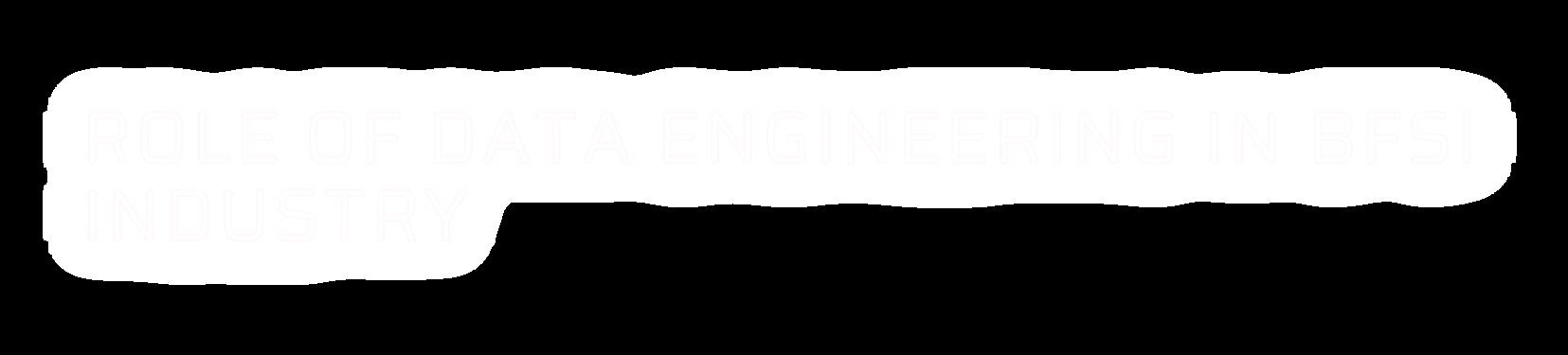
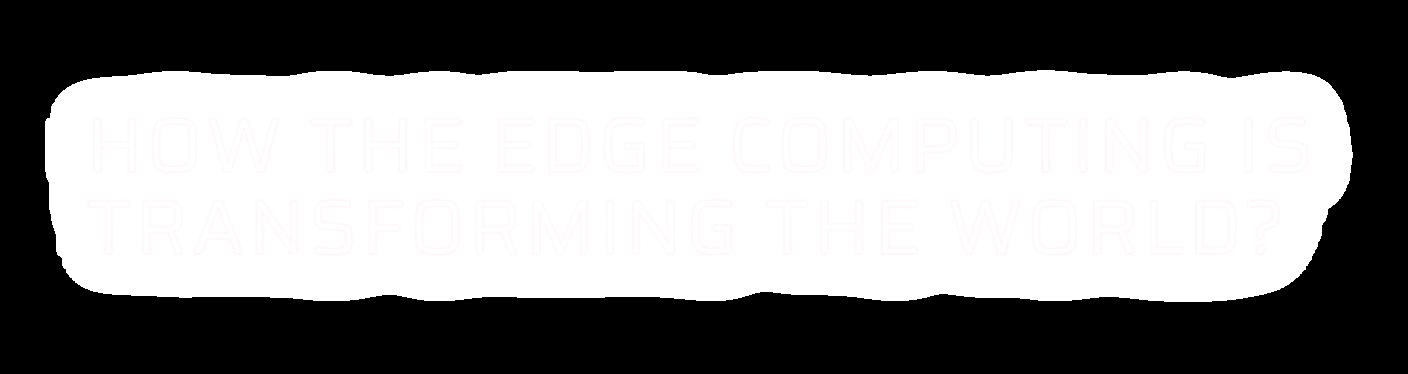
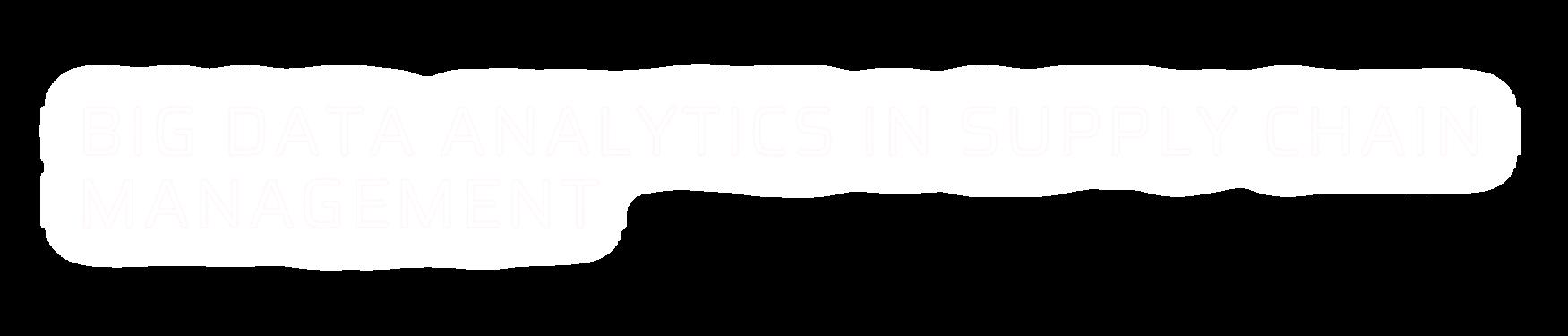
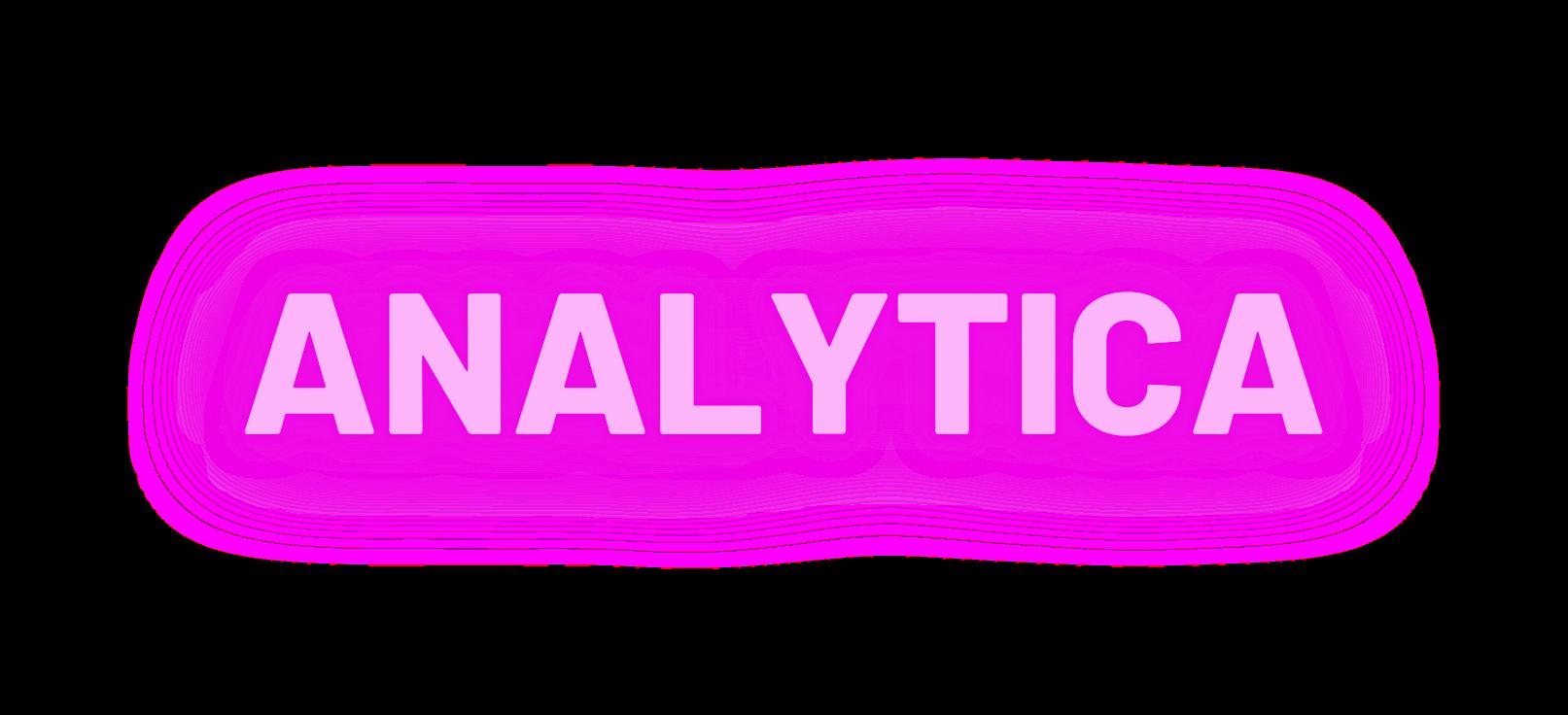
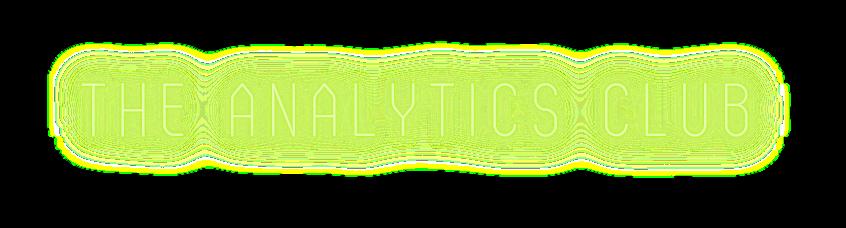

Organon, the Analytics Club of IIM Rohtak is a student-driven initiative whose basic idea is to generate and cultivate student interest in Analytics and technology. Our primary goals and responsibilities include conducting seminars and webinars covering on-demand topics, skills and tools that will help students of IIM Rohtak fraternity gain expertise in various analytical tools. These skills will help students apply analytical tools in their respective fields during placements. We conduct Analytics related events, competency builder events, Case Study Competitions, Quiz questions
$623 Billion is the forecasted capital expenditure amount that global banks and investment firms will spend in 2022 on IT products and services as per the Gartner report Recent developments in retail digital banking system and the ease of use of financial apps have helped the world shift from traditional banking ways to mobile-based payments. Gone are the days of standing in long queue for cash withdrawals, passbook fillings and loan processing. All these transactions can be done within few seconds in few mobile clicks Consumers, now, are willing to prefer banks that provide such solutions in a hassle-free way Hence, it becomes imperative
How much does Banking and Finance Industry spend on technology?
for the banks, in today’s world, to invest such large amount in IT systems to stay at forefront of technology. In India itself, UPI, debit and credit cards, mobile wallets, and prepaid cards collectively processed 23.06 billion transactions totalling INR 38 32 trillion in Q3 2022. By the end of Q3 2022, 1.01 billion credit and debit cards were in use in India
How is Data Science transforming the Banking and Finance Industry?
Today's banks and financial organisations are employing data analytics in a more strategic way, as opposed to the previous approach to analytics in finance and banking, which was to create reports and dashboards Banks have started leveraging technology in almost every operation ranging from market research, identifying right customers, knowing customer behaviour to predictive sales performance analysis. Data analytics come handy in such applications where one has to comb through a lot of data to identify the hidden trends and patterns. The Banking, Financial Services and Insurance (BFSI) industry utilizes data analytics primarily, in risk management, operations (supply side) and for consumer market analytics (demand side) Let’s see each in detail:
Each branch has daily number of customers either withdrawing or depositing cash. When we add daily internet transactions to the offline sales that people make, the total number of daily transactions rises dramatically. This increases the complexity of operational management for the banks as they have to consider, record and process how much money they have on hand as well as what is entering and leaving the channel after each transaction. For a glimpse: In 2021, India accounted for the most real-time transactions globally and this number is going to grow at CAGR of 33 5% reaching 206.2 billion transactions annually by 2026 Data science can help banks make sense of their data in real time. For example, it can help segment the branches as per daily visitor counts, transaction turnover ratio, customer retention ratio, etc. This data can be used by the management to allocate resources in an optimized way to the branches helping them achieve profitability and operational efficiency
Business performance analysis
Performance analysis allows one to track and measure results over several periods so one can understand what's occurring at each stage of organisation
It can help to find profitable as well as loss making business divisions and customer segments, reduce operating expenses and give management an overview of their business. The use of machine learning can help in providing insightful and valuable findings thus making the job of business analysts easier.
Automation of customer engagement channel
Traditional methods of resolving customer queries involved setting up information kiosks, complaint centres at local branches or 24x7 callcentres With the advent of AI, this process can be completely automated. Companies in BFSI sector have already started using AI-driven chatbots and virtual assistants. In addition to automating customer service work, these bots can help in learning about their clients' habits and giving more insightful investment advice by understanding the clients’ needs.
Customer market research: BFSI caters to a diverse set of consumers from both B2C and B2B channels. While a retail consumer can be graded based on his age, income and credit score, corporate clients are profiled based on their financial statements and other economic factors. In a dynamic and ever-changing world, these factors can keep on changing from time to time. Machine learning can help in such cases in offering fresh perspectives on consumer patterns and behaviour. Machine learning may assist sales professionals in finding new leads, developing focused sales efforts and forecasting which goods or services clients are most likely to buy.
CLV Ratio: The total amount of money a client is anticipated to spend with company or on products over the course of an average business relationship is known as customer lifetime value (CLV). It paints a picture of the company's longterm prospects and financial viability High CLV is a sign of product-market fit, customer loyalty and consistent cash flow in future
Calculations of client lifetime value can be complicated by variations in items, prices, purchase frequency and volume One can quickly determine client lifetime value using latest AI-driven tools. Banks and other financial institutions can use this data to inform their marketing and sales decisions, enhancing their business strategy. Customer acquisition: For businesses to increase their income, acquiring new customers is crucial. To do this, they use machine learning algorithms on their CRM systems to uncover novel customer acquisition strategies. Banks and other financial organisations focus on attracting new consumers as well as how their present clients use the products they provide These institutions are employing analytics to create prediction models that consider unique client attributes such as a credit score, income level, etc., as well as factors like geography, which can be used to analyse customer behaviour trends and create more individualised products and services based on the behaviour of various population groupings.
Credit Risk and scoring: The practise of analysing historical data to determine a borrower's creditworthiness or to determine the risk associated with approving a loan is known as credit risk analysis. The analysis' findings aid banks, investors and insurance firms in assessing both their own and their clients' risks For such analysis businesses use Machine Learning (ML) algorithms that make use of large datasets to identify trends and create insightful recommendations These algorithms gather information about the consumer, such as his income, credit worthiness, payment and default history, present assets and liabilities, run through its models and forecasts the risks
associated with lending amount to such customer. Lenders can anticipate the expected loss (EL) using these approaches by calculating the probability of default (PD), loss-given default (LGD), and exposure at default (EAD).
Indian digital payment volume has witnessed a CAGR of 45 4% in the period 2019-22 with total transaction count reaching to 7195 crores amounting to value of INR 1,744 lakh crores.
A substantial danger of security penetration by cybercriminals lurks due to such extremely high volume of financial payment transactions that take place every day. Therefore, maintaining cybersecurity remains a top priority for banks and other financial organisations. Analytics can be used to categorise and rank specific consumers who are at risk of fraud before applying various levels of account monitoring and verification. Organisations might prioritise their efforts to detect fraud by looking at the risk of such accounts. Many fraudulent acts can be identified and stopped using AI before they do serious harm. With the help of technology, banks can apply multiple controls and checks to prevent unauthorized access and if necessary, block the accounts that encounter irregular activities.
Portfolio valuation
Asset management firms perform risk modelling and analysis using AI-driven tools before buying any financial asset. These tools perform various tests such as regression, sentiment analysis, market and credit risk assessment, etc enabling investors to lower the overall risk of their portfolio and earn maximum profits
Due to advancements in computing power, data science and innovations in quantitative
finance and Machine Learning, algorithmic trading has seen a rise in popularity in the asset management industry According to the most recent research analysis by Spherical Insights & Consulting, the global algorithmic trading market size was estimated at USD 13.02 billion in 2021 and is projected to reach USD 31 30 billion by 2030, rising at a CAGR of 13.6% from 2021 to 2030.
As the number of digital enterprises and financial transactions rises, it becomes crucial for the BFSI industry to develop new competencies Leveraging technologies like machine learning and AI can help companies to automate and optimize their business operations, maintain cybersecurity and to forecast risk factors and make better future decisions Efficient data management will help companies save valuable employee time, increase visibility, reduce operational expense and deliver better customer experience. Companies that keep up with technology will forge a competitive advantage, stay profitable over the long term, and remain relevant In conclusion, smart business intelligence technologies and the strategic and intelligent use of data will have a major impact on the future of banking.
Global
Institute of Technology (GIT), Jaipur, RajasthanEdge computing is extremely important in healthcare because of multiple reasons and one of the main reasons is privacy. Edge computing is defined as one, the computing, the actual CPU, the actual modules, and the actual training of the models, and everything is done right at the edge right at the level of the device rather than the data leaving the edge device going all the way to cloud and then coming back rather than going to the cloud it is a big portion of that is being done directly on the edge device. Why is it important? So, if you look at the big i t th bi l d lik
streaming up all these videos to us and it’s connected through a core network and then there are edge servers and then there are edge devices. If we remove the latency and we bring down all that compute power to the level of the edge, we’d significantly remove the latency, the chances of decreasing the data breaches and then we improve privacy. So, all these are extremely important in health care as compared to many other places but healthcare is one of the best applications for edge devices. So, there's a huge difference in the i l ti f thi d i i the
within the router or the specific locations. So, the data in itself doesn't leave the facility. It is a single hop so therefore it’s a decreased latency and the jitter is very low which is the loss of data and the data that bounce between two different devices therefore it's extremely important, especially in devices like robotic surgery, so it’s extremely important to have low latency and low jitter for health care. Geolocation-wise is distributed so whenever things redistributive if there is a data breach, we’re not losing a lot of data and therefore it is extremely important and then secondly more and more healthcare
is distributed mobility there CT scans that are on ambulances, now there are portable MRIS. Right now, we're going to see more and more mobile healthcare being delivered and therefore edge computing is becoming more and more not important not just a reality but an essential part of providing care.
It was truly the main reason that edge computing became such a thing was because of the gaming industry, you have a cd you put it on your ps5 or Xbox whatever you have, and then it uploads 10 to 12 or 25 gigabytes of complete graphics and everything but in a
multiplayer game you don’t upload the whole graphics and back and forth you just simply give the location or an instrument or anything very limited amount of information to the cloud and game you don’t upload the whole graphics and back and forth you just simply give the location or an instrument or anything very limited amount of information to the cloud and backs, so, what happens is that that when you have a processing power the significant power that you have in an Xbox and or in a PlayStation that decreases the workload of the cloud itself, decreases the data transfer that is needed and still has a very good low latency gaming experience I’m not a gamer myself but just from a technology perspective we need to understand that the main benefit that this edge computing or the main industry that took such a big advantage of edge computing is the gaming industry.
NVidia which is actually what creates GPU have been slowly scaling up, as a matter of fact, more and more health IOT internet of things devices are going to be there including the apple watch or other Fitbit or whatever these are billions of sensors that they’re going to collect more and moderate want to crunch those numbers as on the
device, then on the edge server while decreasing the data throughout outside the local network, therefore, decreasing data breaches, increasing privacy and decreasing sort of overall environmental impact. So, therefore it is very important as a matter of fact.
A very recent paper decentralized confidential clinical machine learning, in this, they decided they exemplify in this paper that how a completely new model which is beyond federated learning called swarm learning in which not only that you have the data computing on the edge side you have models of artificial intelligence and machine learning being calibrated and then uploaded and then to the federated and then redistributed to all the edge devices and therefore this sort of swamp learning like multiple drones think about that and then each one of them doing an obstacle course and one of them learns something and all the whole swarm of drones getting updated, this is the same way that we can have a decentralized confidential learning environment only in which rather than the data being uploaded the knowledge being uploaded Therefore, it completely becomes private and therefore it's extremely important in this environment of ransomware and data breaches.
Breakthroughs from NVIDIA: NVidia has done a big push for it as a matter of fact if you can see that they have now hardware consoles that go all the way from 10000 teraflops to 0.5 teraflops and they are trying to do because for two reasons one is, of course, decreasing latency but more importantly if you ever want to get to real-time analytics that we need as physicians, especially in critical situations, we need realtime analytics and that real-time analytics will only be achieved by distributed computing from the edge to the cloud and therefore we need to have these devices more and more intelligent and that's also becoming reality because if we look at the architecture, the main semiconductors initially used to be scalar that was CPU and then vector which was GPU but, now we are having this matrix actual semiconductors The graph here shows the forecast market size of edge computing in the United States from 2017 to 2025 which is insane The rise of edge computing is really increasing and this is just about the USA, the other countries are also going for the Edge Computing specially in the Healthcare industry. Silicon Architecture Evolution :Chips are developed to do AI and these are already in the market if we look at the first M1 chip that was introduced by apple there’s a huge neural core engine with 16 cores nly dedicated to the neural engine to do artificial intelligence
otests and this is not just for apple it’s actually if we look at the arm version 9machine learning which is a big portion of the new brand new architecture introduced in the whole decades a big portion of arms version 9 on which QUALCOMM and apple chips are being designed for So, there's a huge push in the industry itself that the semiconductors are going to become artificial are trying to build in at the hardware level ai so they can perform better, and hence these chips are going to be better, the edge devices are going to better and therefore we're going to have more and more edge computing and more distributed decentralized edge computing.
Recent Innovations: This has direct results right, if we look at the latest innovations from google we can see that they did on device caption so we can click a button and the data doesn't go to the cloud and back it's nothing like that it’s just simply the device has enough power that it can do ON device caption or ON device speech recognition apple also introduces it more recently with Siri and it doesn't have to leave any data doesn’t have to leave your device but it’s going to be automatically done within the device itself because the device is much over powerfully has all the capabilities and the models have been also shrunk down in terms of memory usage that now we have better models better hardware to take care of these new realities
Economic globalisation, rising customer expectations, information technology advancements, and other competitive business priorities have forced businesses to adapt to the competitive environment of today Thus, competition between firms and their supply chains has taken on the role of commercial rivalry. At the same time, data is expected to grow by an exponential rate to a zettabyte annually. So, it has become necessary to design systems that can swiftly and intelligently evaluate huge amounts of data as there is a rapid rise in volume and variety of data types throughout the supply chain. These systems employ technologies that can process these massive amounts of data in order to boost operational effectiveness, reduce costs, and boost service levels while strengthening the supply chain capabilities of the companies.
Supply chain analytics (SCA) refers to the application of Big Data Analytics (BDA) methodologies to extract hidden valuable knowledge from supply chains.
These analytics can be divided into descriptive, predictive, and prescriptive analytics. By marrying data and quantitative approaches, big supply chain analytics improves decision-making for all supply chain activities. Particularly, it includes two new functionalities First, it expands the dataset for analysis beyond the typical internal data kept in ERP and SCM systems Second, it analyses both recent and historical data sources using sophisticated statistical techniques. By reducing the expenses of sourcing, transportation, storage, running out of stock, and disposal, this produces new insights that directly impact the bottom line
Big Supply Chain Analytics encompasses and empowers all applications of supply chain technology.52 distinct sources of big data that are produced in supply networks are summarised in the following graphic. Big Supply Chain Analytics also enables more intricate supplier networks that
emphasise communication and cooperation as a value-add over merely concluding transactions. The progression of supply chains from networks or webs, where knowledge sharing becomes a priority, is seen in the accompanying figure.
BDA helps in the improving the Traceability in the Supply Chain Process. For instance, by employing barcode scanners and adding radio frequency identification devices to certain products, supply chain managers can quickly trace a product. Tracking consumer behaviour and usage patterns is another benefit The information gathered from the analytics report can be used by businesses to keep their subscribers and significantly increase revenue. For instance, Jio makes effective use of big data analytics to estimate network growth and plan network expansions. Additionally, it helps businesses manage their inventories For instance, Amazon uses big data analytics to control its inventory It chooses warehouses depending on the proximity of its suppliers and clients in order to reduce distribution costs. Also, it helps with machine maintenance in production plants. For instance, sensors can be used to monitor production, foresee issues, and notify you when routine maintenance is necessary to keep your equipment in good working order.
Applications of Big Data Analytics in Supply chain Planning with Big Data: Statistical models and integrated data from the entire supply chain can help estimate demand more accurately. For instance, to guarantee consistency in its customers' shopping experiences, IKEA developed the revolutionary Demand Sensing, an AI-based solution that optimises stock levels Also, a recent study proposes a BDA model for forecasting the demand for air passenger demand, which calculates the demand for air passengers using big data. Only a 5.3% prediction error was found in the study's findings. Purchase decisions with Big Data: Using supply chain analysis to evaluate supplier performance and compliance in real-time on a quarterly or yearly can help us spot opportunities and address issues before us spot opportunities and address
issues before they become serious. For example, Ireland-based Keelvar is one business attempting to address the procurement issue head-on It has received $24 million to finance its "intelligent sourcing" platform. It has created sourcing bots that enable customers to look at information about direct and indirect procurement bids from suppliers and then analyse various awarding scenarios based on those standards and other constraints (4)
Performance with BDA: Big Data helps reorganise the supply chain's multiple flexible portions, optimise the use of resources and increase efficiency throughout implementation For instance, an Indian company called Reliance Games used big data analytics a few years ago to enhance product creation and boost sales (5)
Reverse Logistics with BDA: Analytics can help lower costs and provide the knowledge needed for trouble-free returns by combining data from inventory and sales systems, as well as from incoming and exiting flows. Surajit et al showed how big data analytics (BDA) has been useful in improving quality assessments of reverse logistics (6)
Supplier Relationship Management with BDA: Employing BDA methodologies can deliver precise data on corporate expenditure trends that support managing supplier relationships. Big data, for instance, can offer precise data on the return on investment (ROI) of every project and in-depth study of possible suppliers
Customized production with BDA: Through fast and accurate data analysis of each step of the production process and a thorough examination of the whole supply chain, BDA helps manufacturers to precisely determine each person's activities and tasks. Manufacturers can locate bottlenecks and expose underperforming components and processes thanks to this ability For instance, Zhong et al. used big data with RFID capabilities to enhance shop floor logistics
planning and scheduling. Then, he applied the Physical Internet concept to create an environment with RFID-enabled intelligent shop floors employing wireless technology, BDA, and the Internet of Things.
Product design and development with BDA: Making sure that the items match the desires of their clients is one of the main concerns of producers of adaptable products. Designers may create goods that satisfy customers' expectations if they keep an eye on consumer behaviour and have access to the most recent data about their preferences.
Sustainable Supply Chain using BDA: In order to gain a competitive advantage, businesses are becoming more and more interested in using BDA in their sustainable initiatives For instance, a Mckinsey survey found that businesses utilising BDA can forecast the 65% of customers who make repeat purchases through shop notifications,
and 75% of those customers said they are likely to use the service again.
Conclusion
There are significant advantages to incorporating big data technology into each stage of supply chain management BDA can aid in the creation and development of an adaptable, trustworthy, and/or sustainable supply chain. BDA is capable of integrating and managing massive collections of heterogeneous data in a convoluted global supply chain. Combining solid data sets with predictive analytics, IoT, and other technologies may now provide supply chain managers with the resources they need for strategic decision-making
Although making an initial big data investment can be scary, many businesses find that the rewards far surpass the expenses Businesses will undoubtedly continue to use and invest in big data technology for the foreseeable future.
The research team used their method to detect a hydrocarbon-saturated zone in the sandstone-based reservoir at 2.3 km underground in the Tipam formation of the Upper Assam basin.
Blockchain analytics unable to prevent FTX-level illicit schemes
Data transparency has been a focal point for the crypto industry, but the FTX fiasco has shown that centralized exchanges (CEX) are not transparent enough. So far, crypto analytics firms are apparently not capable of tracking transactions to prevent collapses like FTX All Bitcoin (BTC) transactions are available publicly on-chain, which enables tracking such transactions when sending crypto from one address to another. However, this is not the case when it comes to interacting with a centralized crypto exchange.
Amidst Rising GST Collections, Analytics Will Emerge as Critical Tool for MSME Lending in 2023, says Jocata
"The GST framework is a treasure trove of business transactions data and is now beginning to drive financial institutions' lending decisions, especially to MSMEs," said Prashant Muddu, CEO & MD, Jocata, a B2B digital lending and compliance fintech.
Musk’s New Twitter is planning to optimize unregretted user minutes that users spent on the platform: In recent days Elon Musk’s New Twitter, which is running under him is on the frontline of every news because of implementing various changes. Elon Musk has said that the new Twitter aims to optimize user minutes that a user spends on the microblogging platform, and not regret it. Presently busy making Twitter faster, Elon Musk has brought back the company from going bankrupt and now is in search of a way to make the company profitable.
AI & Analytics Service Companies Sees 4–5% Increase in M&A
AI and analytics always have something interesting brewing be it mergers and acquisitions, fundraising or strategic partnerships. One way to look at this is that larger traditional players are acquiring small to midsize companies and expanding their service offerings, alongside tapping into the talent pool of the organisation. “There has been at least 4–5% increase in the kind of mergers and acquisitions that have happened across AI and analytics service companies,” said UnearthInsights’ founder Gaurav Vasu, in an interaction with Analytics India Magazine.
What does SAAS stand for?
System Aerosurface Actuator Simulation Systems as a Service Software acting as Service Software as a Service
6. A free, Java-based programming framework that supports the processing of large data sets in a distributed computing environment.
Hadoop Python R
Apache Groovy
Relational Databases
Hadoop HDFS
Hadoop HDFS MongoDB
1. 2. According to a very recent Jaspersoft Survey, what is the most popular big data store? 3. Which of the following best describes the requirement-gathering process in data analytics?
Collect and analyze data, decide the problem to solve
Find a need and then collect data
Find a need and then analyze data to support it
7. The branch of data mining concerned with the prediction of future probabilities and trends.
4 A system for managing a single source of truth is called as?
8. The science of examining raw data with the purpose of drawing conclusions about that information
Find a need, ask questions, define and analyze data, and find connections Data model Database Data Lake Master data management Facebook Apple Twitter None of the above
5 The company having the biggest Hadoop cluster is?
9. A method of storing data within a system that facilitates the collocation of data in various schemata and structural forms.
10 Where did Hadoop get its name from?
In-memory Analytics Predictive Analytics Behavioral Analytics Big Data Analytics Data Analytics In-memory Analytics Descriptive Analytics Predictive Analytics Data Visualisation Deep Analytics Data Lake Big data management Splunk Storm Splunk Cloud Hunk MongoDB