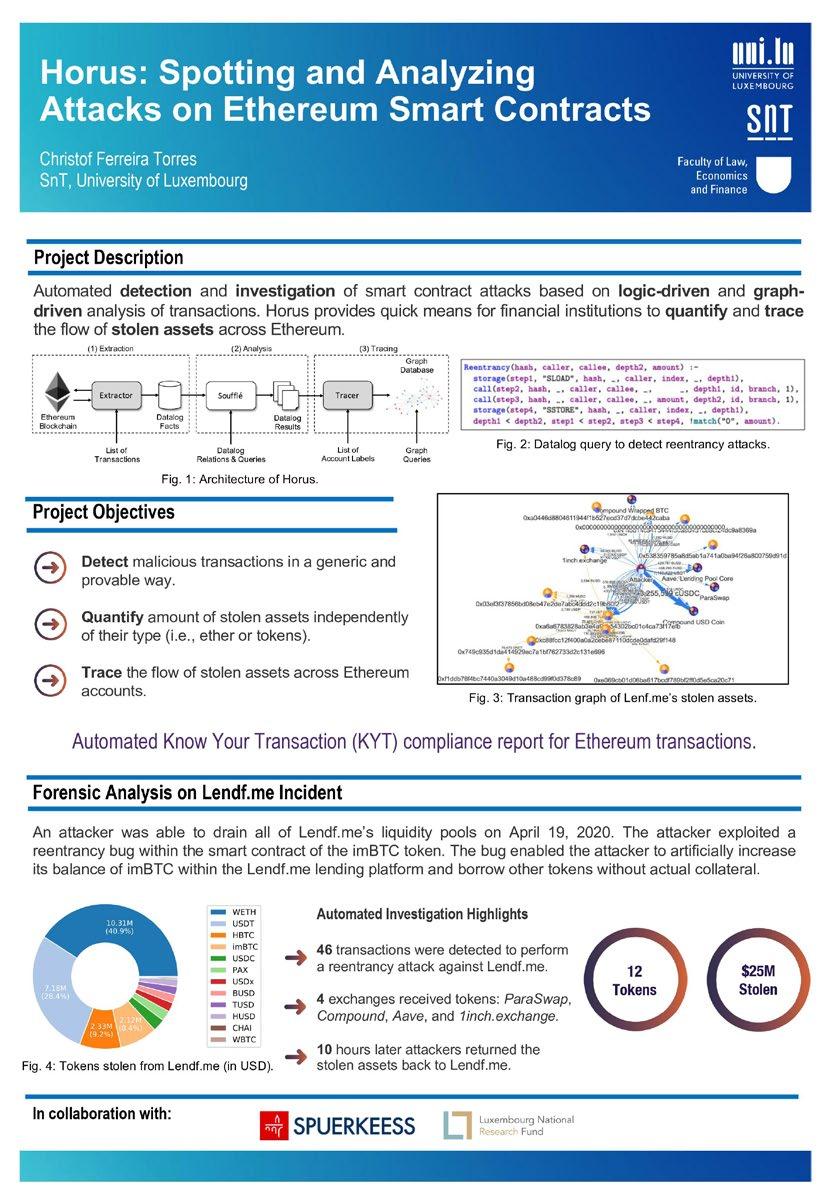
2 minute read
Robust Financial Machine Learning
Simonetto, S. Dyrmishi S. Ghamizi, M. Cordy, Y. Le Traon SnT, University of Luxembourg
A. Boytsov, C. Lefebvre, A. Goujon, BGL BNP Paribas
Financial machine learning systems
can be fooled!
Adversarial examples are inputs carefully designed to cause erroneous predictions in machine learning systems Our contribution is to generate realistic adversarial examples and use them to improve the models
Model-Based Specification and Analysis of Requirements in the Financial Domain
A. Veizaga, A. Rizzi, S. Shin, L. Briand, SnT, University of Luxembourg
T. Henin E. Pitskhelauri, S. Rohackova, Deutsche Börse Group, Clearstream Service
B. Cardinael ESCENT
Context
Good requirements must be: ü Precise ü Consistent ü Complete Risks arise when requirements are: x Vague x Inconsistent x Inadequate
Our Solution: Drona
Challenges
Requirements analysis is crucial for the success of an IT project in the financial domain
Production of high-quality requirements and models
Generation of a full deliverable in a single tool
Automated generation of acceptance criteria
Robustness of real-world ML systems
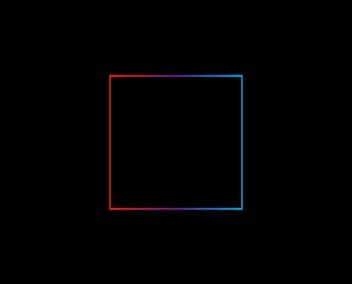
RQ1: How robust are financial ML systems to realistic adversarial examples?
97.5% of the transactions can be altered to trick the system
RQ2: How to make ML systems robust to adversarial examples?
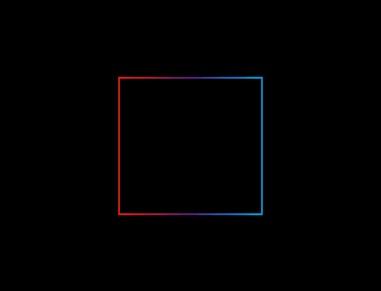
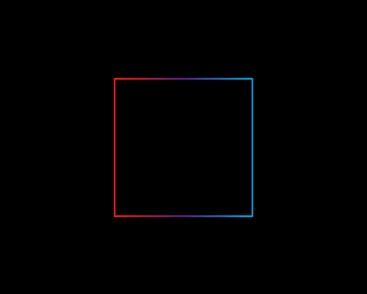
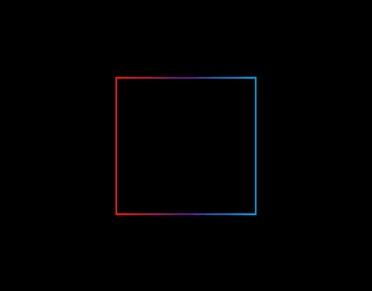
We need dedicated methods to fool real-world systems
1. Modeling support
2. Requirements authoring support
4. Full deliverable generation
5. Gherkin test scenarios generation
21% more robust with no additional training cost Real machine learning systems are vulnerable to realistic adversarial
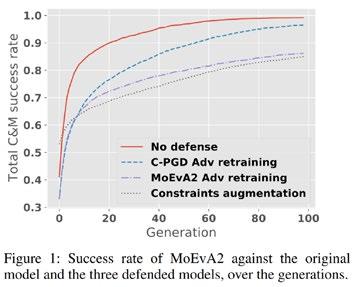
Effectiveness of our defenses
Ready for industrial applications
Applied on real-world systems at BGL BNP Paribas. We have demonstrated the benefits of our approach on the bank’s ML systems.
Cross domain, no tuning. Our approach can be applied to different domains and use cases without parameter tuning.
Also improve model generalization. Training a model using our generated examples also help it to correctly classify unforeseen patterns.
In collaboration with:
3. Requirements-to-model reconciliation support
Interdisciplinary Approach: Software Engineering, Linguistics, and Artificial Intelligence
1 Modelling Support
Full integration into the Enterprise Architect modeling platform including customized toolboxes, model patterns, and model templates
2 Requirements Authoring Support
RIMAY: A language for writing requirements Improve the quality of requirements
LEVERAGING Detect errors and assist in writing requirements in Rimay
3 Reconciliation Support
• Consistency checking between textual requirements and models
• Proposal of recommendations for model enrichment
4. Deliverable Generation
Capture of all deliverable sections in Enterprise Architect to generate a full deliverable
5 Gherkin Test Scenarios Generation
Automated generation of Acceptance Criteria in the Gherkin language based on the requirements (text and models)
Drona requirements authoring support, deliverables generation and automation of Acceptance Criteria will save significant time on your projects!
Conclusions
The NCER-FT will have a strong, sustainable impact on not only research, but also the economy and society. By supporting the development of Luxembourg’s deep-rooted financial tradition with leading technologies, we will create a more inclusive future for everyone who relies on the markets we will influence – and make Luxembourg a global leader in financial technology innovation.
Our ambitious, collaborative approach to research in financial technologies fosters a dynamic culture of technological innovation in Luxembourg’s strongest industry. We strengthen Luxembourg’s position as an innovative financial hub by attracting talent and providing world-class research and education.
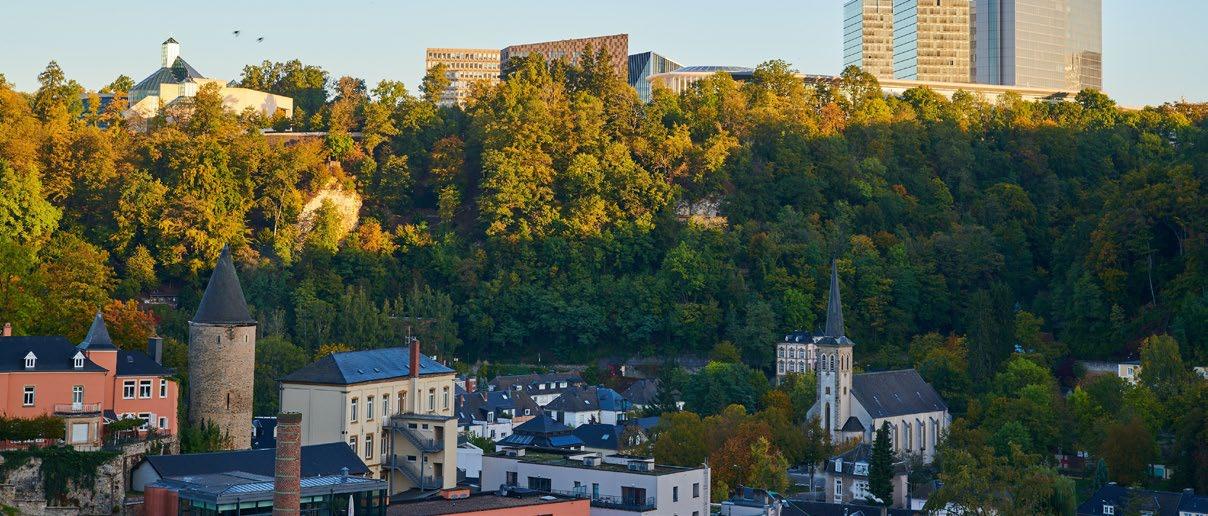