6 minute read
Coffee Needs Research
Next Article
The Algorithm Will See You Now:
Chen-Nee Chuah and Xin Liu on Big Data, AI and Machine Learning in Medicine
Dr. Chen-Nee Chuah, UC Davis (Dr. Chen-Nee Chuah/UC Davis) Dr. Xin Liu, UC Davis (Dr. Xin Liu/UC Davis)
By Cristina Deptula
MACHINE-LEARNING ALGORITHMS, while not intended to replace human doctors, can be powerful tools to help process and make sense of patient data. Electrical and computer engineering professor Chen-Nee Chuah and computer science professor Xin Liu are preparing sensors and algorithms to work alongside doctors to treat patients.
Chuah explains deep learning algorithms in medicine are intended to identify patterns from data that a human might miss or take over labor-intensive tasks, such as monitoring a hospitalized patient’s every breath or heartbeat. The algorithms will digest a large amount of real-time, raw data to help doctors diagnose disorders or figure out a patient’s prognosis.
Liu says the time is right for big data in healthcare. This is because of technical advances in AI, including computer vision, deep learning algorithms, natural language processing, and an increased use of mobile devices that can democratize healthcare and enable personalized medicine. Chuah points to greater data storage, computation power and edge computing’s potential to enable intelligent learning tasks on computer networks.
Chuah had always wanted to apply data science and intelligent learning techniques to benefit society. As a patient at UC Davis’ medical center, she noticed a ‘digital gap’ between how people practiced healthcare and the advanced data analytics and AI/ machine learning techniques she used as a professor in computer networks. During her sabbatical she reached out to the UC Davis Health School of Medicine’s health informatics group and began working with Dr. Jason Adams, an ICU physician in pulmonary and critical care medicine.
Liu said she’s motivated by the potential advances in AI technology to help people live better and healthier lives. “My parents are aging and technology can help them stay healthier and more independent and better enjoy their golden years.”
She says big data in medicine could greatly benefit the elderly. “The potential for [geriatric therapy] is multifold, from monitoring cognitive functions to observing mobility to chronic disease monitoring and intervention.” For example, observing gait changes and warning that a patient is at risk of falling.
Both Chuah and Liu are addressing the COVID-19 pandemic. Chuah has collaborated with Adams to design AI-driven diagnostic and prognosis tests to predict who among newly infected patients under mechanical ventilators will develop serious lung injuries. They have also developed COVID surge prediction models using multivariate time analysis. Finally, she recently started working with mechanical and aerospace engineering professor Cristina Davis on her National Institutes of Health-funded project to develop a breath-based COVID test. Liu has worked with Dr. Katherine Kim to develop a COVID symptom tracking app relying on big data.
Big data, artificial intelligence and machine learning can protect patients on ventilators. “Any patient-ventilator asynchrony can cause severe lung damage and it’s difficult to have a physician or a respiratory expert at each patient’s bedside 24/7,” said Chuah. “Automatic alarms have a high false positive rate, which can lead to physicians not responding as quickly as they should. A more intelligent algorithm can provide real-time critical alerts to providers without overwhelming them.”
Big data and machine learning algorithms can also prove especially good for monitoring the health of patients with chronic conditions such as diabetes. The technologies let clinicians observe patients’ personal data with finer granularity, learning over time what’s normal for each person’s body. “Ten percent of the US population has diabetes and one in three has prediabetes. Integrating continuous glucose monitoring devices, wearable sensors such as the FitBit and dietary apps on our phones can provide comprehensive information to help doctors personalize medication and prescribe more effective lifestyle interventions,” says Chuah.
She’s also working on a Department of Defense-funded project, Endovascular Perfusion Augmentation for Critical Care, that will optimize critical care management, such as resuscitation after trauma, in real-time. In another NIH-funded project, she’s developing methods to analyze video screenings of young children for signs of autism to enable earlier intervention.
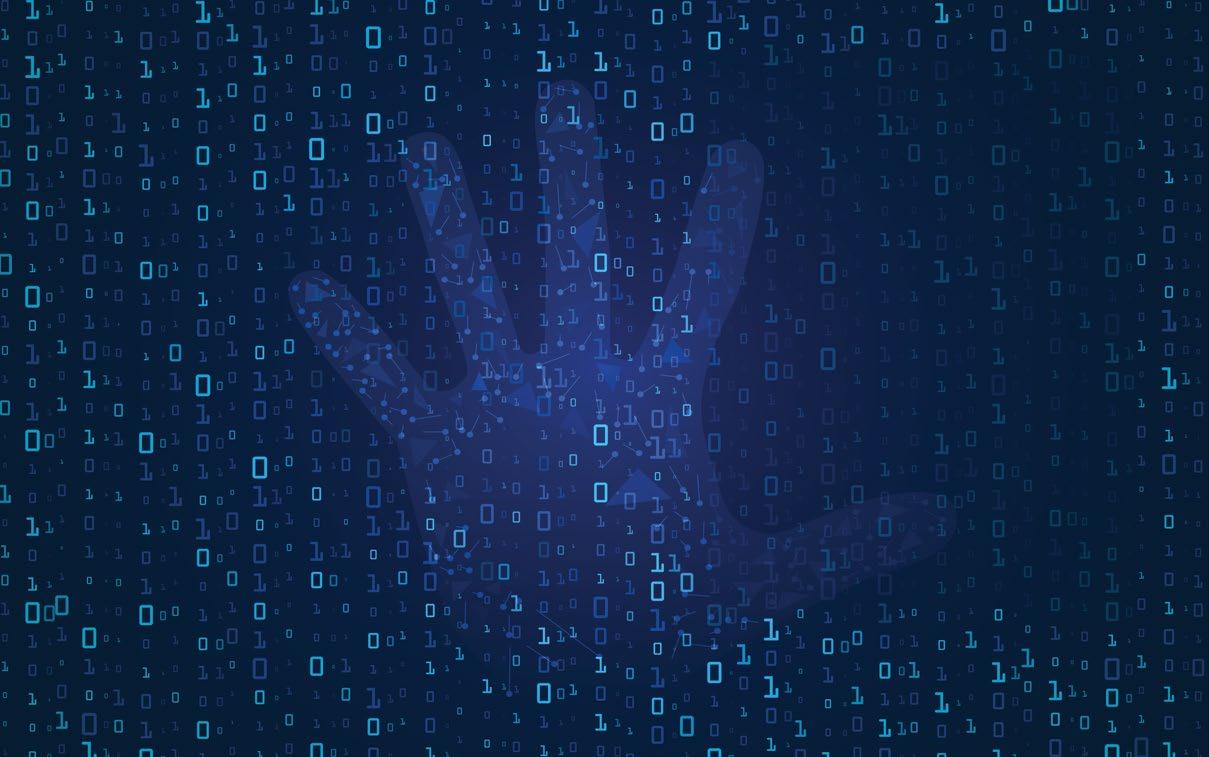
Liu is creating machine learning algorithms for digital sensing in healthcare applications. “Digital sensing involves non-invasive monitoring of vital signs and behaviors in both temporal and spatial overlays at various scales and granularities. Sensors we use include accelerometers, gyroscopes, visible light and infrared cameras, audio sensors, ECGs, glucose monitors, walking plates in motion labs, medical imaging devices, neuron sensors and electrophysiology devices.” She’s creating AI algorithms to analyze data from those sources to provide relevant insights for healthcare.
Liu and her lab also developed an AI-driven sensing system with an iPhone app collecting data with the phone’s accelerometer sensor (currently used by UC Davis clinical researchers), a web-based tool to aggregate and store data and a suite of AI algorithms to analyze sensory data. Their work could help predict recovery of mobility in ICU patients, identify strokes earlier and better predict prognosis for patients with brain injuries. These projects use AI algorithms for time-series sensory data from potentially multiple sources.
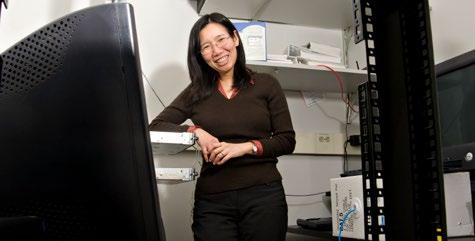
Chuah says researchers have made various advances towards fairness and accuracy of medical machine-learning algorithms for diverse sub-populations, including statistical methods of quantifying prediction uncertainty and incorporating fairness in the loss function of deep learning models. Liu advocates addressing diverse groups’ needs in the entire big data pipeline, including algorithm development, testing, deployment and redeployment, and having a diverse big data workforce.
Liu explains that patient privacy and data security are concerns in most big data research, and especially vital for healthcare. There are technical as well as regulatory ways to address this. “We are working on a type of algorithm called federated learning that allows data to stay with the person instead of being gathered by a central entity,” she said. This will streamline pre-screening for health conditions through people’s smartphones with data remaining safely on the patient’s phone.
Chuah and Liu both emphasize that their work will not eliminate human physicians. “We aren’t replacing human doctors,” says Chuah. “We envision an AI-assisted clinical decision-making support system with humans in the loop.”
Professor Izzat “Ed” Idriss (third from right) with geotechnical engineering faculty at UC Davis.
Dr. Chen-Nee Chuah. (Jason Spyres/UC Davis)
Mentor Collective
at UC Davis College of Engineering
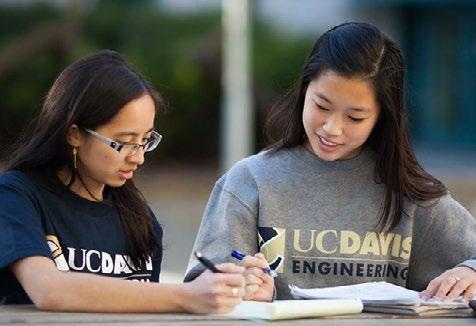
The Mentor Collective program connects first-year engineering students (freshmen and transfers) with a recent UC Davis engineering graduate. These alumni mentors are ready to listen, inspire and help students navigate challenges and recognize opportunities. Students will learn what to expect in classes and how to approach new experiences; they will also gain career advice and valuable insights from alumni in the field.
If you are an engineering alumni interested in this program, visit http://bit.ly/MentorCollectiveCOE
STUDENT ORGANIZATIONS
• American Indian Science and Engineering
Society • Black Engineers Association • Chicano and Latino Engineers and Scientists
Society • Pilipinx Americans in Science and
Engineering • Out in Science, Technology, Engineering, and Mathematics • Society of Women Engineers
#3 #11
Biological and Agricultural Engineering (#3) and Civil Engineering (#11) among the nation’s top graduate engineering programs
– U.S. News and World Report 2022
220
Total Faculty
13
Members of the National Academies
$93 million
in research expenditures (2019-20) (USNWR)
4,538
Undergraduate Students
30.98% women 22.9% underrepresented groups 4.1 years—mean time to degree LEADR Student Support Center Engineering Design & Startup Centers 1,017
Graduate Students
330 M.S. 687 Ph.D.
Departments
• Biological & Agricultural • Biomedical • Chemical • Civil & Environmental • Computer Science • Electrical & Computer • Materials Science • Mechanical & Aerospace
UNIVERSITY OF CALIFORNIA, DAVIS
#2
Best Public University for Social Mobility
– Washington Monthly
#1
Campus Sustainability (U.S.)
– UI GreenMetric World Ranking
#4
Best Colleges for Your Money
– Money Magazine
UC Davis technologies produced 115 startups in the last 10 years
#5
Best Public U.S. University
– Times Higher Education
#1
University in the nation for Inclusiveness, Diversity and Internationalization