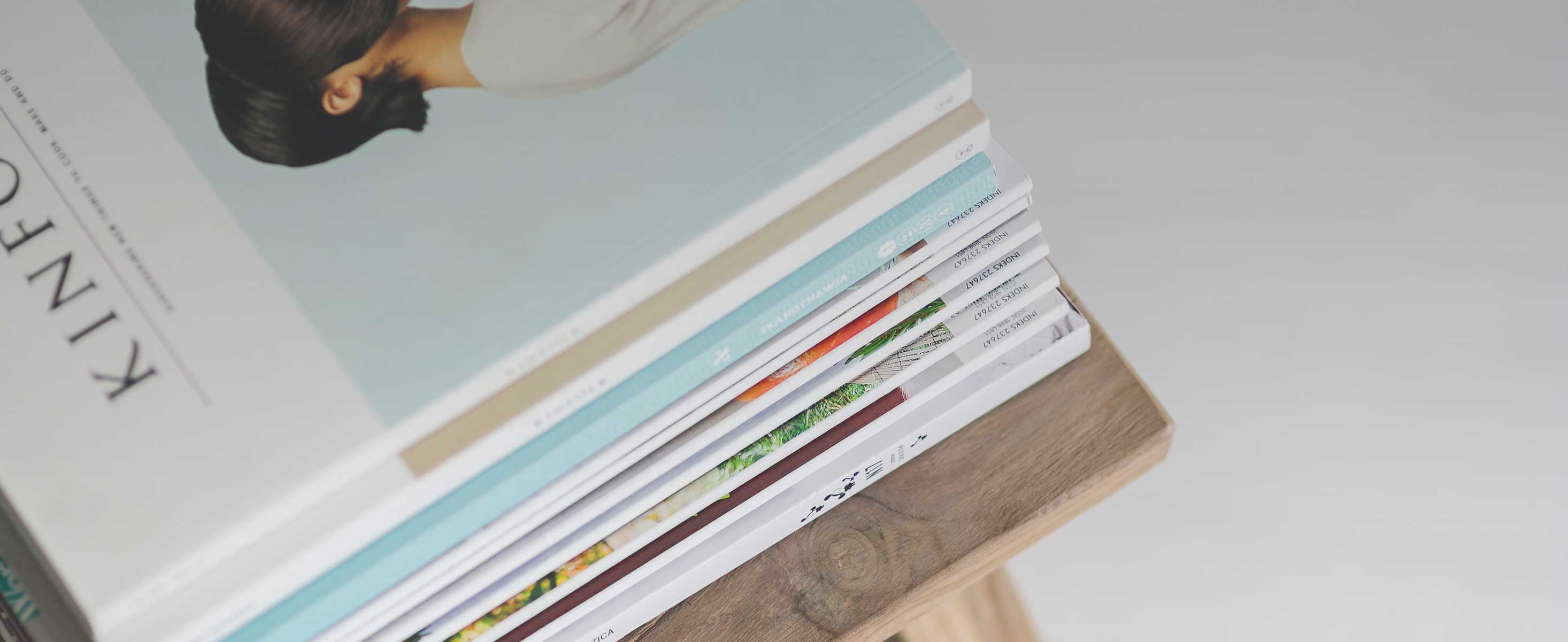
4 minute read
Automated pattern recognition in wholecardiac cycle echocardiographic data: Capturing functional phenotypes with the machine learning
Automated pattern recognition in wholecardiac cycle echocardiographic data: Capturing functional phenotypes with the machine learning
REVIEWED BY | Anthony Wald ASA SIG: Cardiac
REFERENCE | Authors: Filip Loncaric, MD, Pablo-Miki Marti Castellote, MSc, Sergio Sanchez- Martinez, PhD, Dora Fabijanovic, MD, Loredana Nunno, MD, Maria Mimbrero, MD, Laura Sanchis, MD, PhD, Adelina Doltra, MD, PhD, Silvia Montserrat, MD, PhD, Maja Cikes, MD, PhD, Fatima Crispi, PhD, Gema Piella, PhD, Marta Sitges, MD, PhD, and Bart Bijnens, PhD, Barcelona and Madrid, Spain; and Zagreb, Croatia Journal: Journal of the American Society of Echocardiography Open Access: No
WHY THE STUDY WAS PERFORMED
This study aimed to demonstrate the feasibility of applying machine learning (ML) tointerrogate echocardiographic data from a whole cardiac cycle automatically. Primarily it wasto recognise patterns in velocity profiles and deformation curves allowing the identification offunctional phenotypes.
Pattern recognition in echocardiography is vital; tissue and blood flow Doppler reflect important patterns directly related to systolic and diastolic function. Each velocity profile can contain certain patterns specific to an underlying impairment. When these specific profiles are analysed, they can represent a ‘larger’ pattern that describes a patient phenotype.
Machine learning is being incorporated into echocardiography on an ongoing basis. Most approaches are centred around automated measurements of singular parameters such as left ventricular outflow tract velocity time integral or peak velocities across a valve. Whole cardiac cycle echocardiography data contains much information about cardiac function; by analysing the whole cardiac cycle, machine learning can capture patient similarities without being conditioned to a diagnostic label or clinical outcome.
The authors hypothesise that a machine learning workflow can automate data integration and pattern recognition from whole cardiac cycle echocardiography.
HOW THE STUDY WAS PERFORMED
Two hundred and eighty-six participants were enrolled in the study, 189 with hypertension and 97 without. All patients in the hypertensive cohort were asymptomatic and clinically managed.
Echocardiography was performed on the E9 or E95 echocardiography machines (GE Vingmed Ultrasound, Horton, Norway). Post imaging analysis was performed on EchoPAC, looking at longitudinal strain curves of the left ventricle. These were performed in the apical four-chamber view using an average of all six segments.
Deformation analysis of the left atrium was performed from the four-chamber and twochamber views. Left atrial reservoir, conduit and contractile function were evaluated by measuring the total systolic, early diastolic and late diastolic strain. The left atrial strain values were averaged across the four and two-chamber windows.
Deformation analysis, blood pool, Doppler tissue imaging velocity and all associated measurement profiles were semiautomatically analysed and acquired. In total, 1413 data points for every whole cardiac cycle of each participant were generated.
Multiple kernel unsupervised machine learning was used to integrate each data point per participant and position the patient in a virtual space representation of the echocardiographic characteristics. Regression and clustering analyses were performed to identify echocardiographic patterns present within the virtual space.
Automated pattern recognition in whole-cardiac cycle echocardiographic data: Capturing functional phenotypes with the machine learning continued
WHAT THE STUDY FOUND
This study showed the feasibility of using machine learning to automate the integration of tissue and blood flow Doppler obtained during a cardiac cycle to compose a comprehensive description of cardiac function. At the same time, it was automating the pattern recognition capability to recognise disease-related patient phenotypes.
RELEVANCE TO CLINICAL PRACTICE
Further research needs to be done to establish the prognostic value of this type of whole cardiac cycle assessment. This study did show the capability of machine learning to classify patients into a phenotype; however, the prognostic value of this practice needs to be studied further.