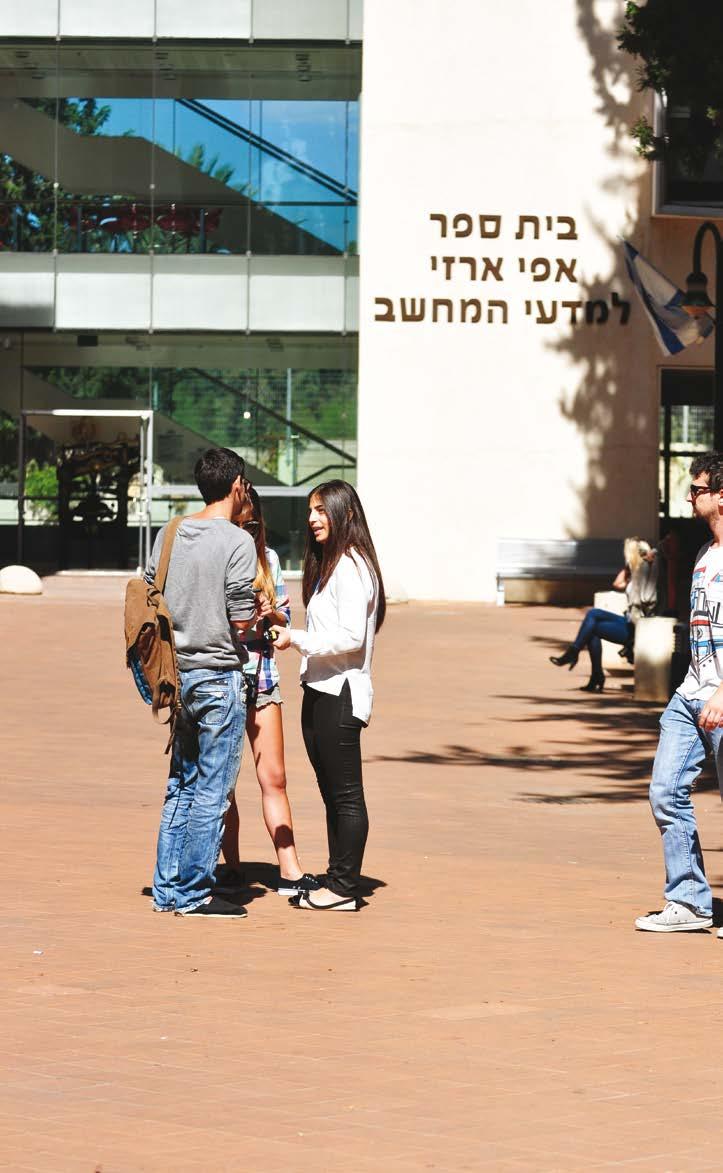
4 minute read
/// CREATING ACCESSIBLE SOLUTIONS TO REALLIFE PROBLEMS WITH MACHINE LEARNING
A part of Artificial Intelligence (AI), Machine Learning is where humans teach a computer or machine to perform an action by feeding it as much data as possible, and the machine learns from that “experience”. Examples of this include DALL·E 2, the system that enables us to create realistic images and art from text, and ChatGPT, the model that interacts in a conversational/dialog format, allowing follow-up questions and answers, amongst other features.
At the Efi Arazi School of Computer Science, Dr. Ohad Fried and his students are trying to solve reallife issues with Machine Learning, like making sign language more accessible in TV and movies, looking for solutions to prevent child abuse in daycares, and dealing with deep fake videos and photos.
“The latter brings up an important point,” says Dr. Fried. “While Machine Learning has many advantages, it also has its limitations, and it can be negatively used by bad players to create deep fake media. Since Machine Learning outputs can make a reliable impression of pictures that look accurate, these bad players can use them to hurt, incite and publish lies disguised as truth.” According to Dr. Fried, while the solution to deep fakes cannot be exclusively technological, we can use Machine Learning to identify and flag the “signatures” of the algorithm that created the fake media in the photos and videos.
One method they’ve used to identify deep fake videos combines Machine Learning and classical algorithms, and identifies specific parts of speech in videos as fake. Dr. Fried and his collaborators analyzed speech and letter pronunciation and concluded that while certain letters cannot be pronounced with the mouth open, deep fake algorithms often miss this behavior. They used this insight to create an algorithm that can identify and flag each part of the video where the speaker’s mouth is open when it should be closed. These videos are then labeled as fake.
Another problem Dr. Fried and his students are trying to solve is the lack of accessible media content for people with hearing disabilities. They aim to replace human sign-language translators with a video of a sign-language translator created by a machine. Toward this goal, they produced a “Stick Figure” that translates to human sign language. This method makes translations of media content more accessible. Responses from people with hearing disabilities were that the translator should look human, and this will fall within the scope of their follow-up project.
Another important project that Dr. Fried and his students have worked on is finding a solution to prevent child abuse at daycares. Since Israeli law prevents everyone, including the police, from watching recordings taped in daycares without a formal complaint being filed, the team suggested that a machine watch the videos and flag any child abuse by adults. However, they are facing several challenges and hope to upgrade and improve the machine’s human identification capabilities in future.
About the fast-growing field of Machine Learning, Dr. Fried says, “When I entered the field, there were already proven examples that Computer Science, and Machine Learning specifically, can solve real-life problems. I have witnessed a dramatic improvement in the performance of Machine Learning tools, which were theoretical and didn’t always work properly, and now solve many important problems at a very high level. One specific tool, called deep neural networks, has existed for dozens of years, yet only in 2012, when I began my doctorate studies, did it start working. And today, in 2023, full departments in universities and companies deal with this tool alone. The technology has massively developed in the last 10 years, and this is very exciting to see.”
Dr. Fried has collaborated with a number of people on his projects, including his MSc students Rotem Shalev and Bar Cohen, as well as PhD student Amit Moryossef from Bar-Ilan University, Daniel Arkushin, MSc and Prof. Shmuel Peleg, from the Hebrew University of Jerusalem.
Given an input text, the system generates stick figures that mimic the motion of a human sign language interpreter
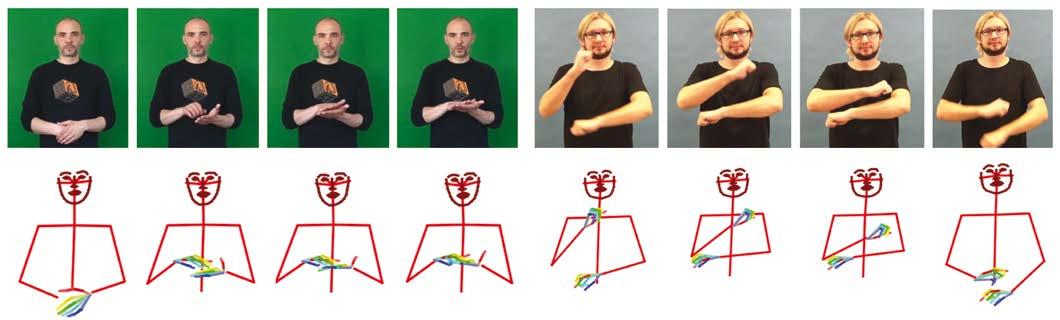
Given an input text, the system generates stick figures that mimic the motion of a human sign language interpreter