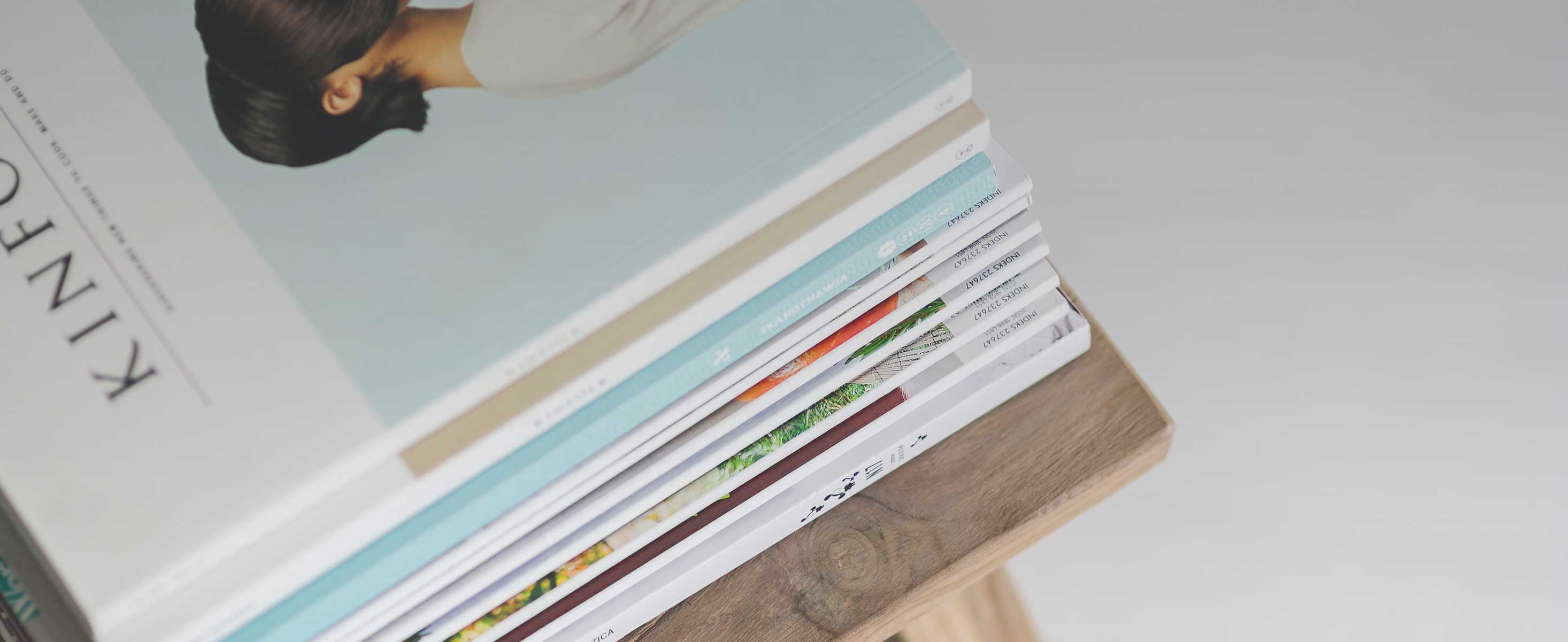
13 minute read
digging into project risks aimed at climate change
by Ganesh Melatur
Several organisations are presently in the process of initiating and planning significant projects for addressing climate and energy risks, for reduction of their carbon emissions and water consumption, or for switching partly or fully to renewable energy sources. However, a common failing observed in the preliminary evaluation of such projects relates to the confused identification and assessment of the different kinds of risks impacting project success.
Advertisement
It is important that risk identification and assessment for this type of project, that usually requires large initial investments for feeble returns but bears high chances of failure, be carried out by paying attention to the various stages at which these risks arise. Such a stage-wise differentiation enables a better understanding of the risks themselves, permitting the definition of appropriate risk responses so that threats can be effectively eliminated within each stage for the overall chances of project success to be enhanced.
Introduction Categories Of Project Risk
It is possible to obtain a holistic and exhaustive view of all potential project risks during preliminary project evaluation by proceeding in a sequential manner to identify and categorise such risks as pertaining to one of: modelling, financing, or schedule realisation of investments and returns. This categorisation is reflective of the project evaluation and selection process that is performed sequentially, with project modelling being the first step, choices for project financing considered second, followed by the finalisation of dates when investments are to be made and returns programmed. Though these three risk types are separate and distinct from each other, their simultaneous impacts on each other (e.g. financing risks can impact both the developed model and its realisation), cause confusion that can be resolved only by individual identification and assessment of risks for evolving appropriate responses to them at each stage.
This article refers as example to projects that are currently being planned by businesses in non-EU countries for exporting carbon-intensive products (such as iron and steel, cement, fertilisers, etc.) to the EU whilst adhering to the EU CBAM (Carbon Border Adjustment Mechanism).
Modelling risks relate to the project idea, which is the asset being created, in the form of benefits that will be realised less their costs for realisation. The project idea is securely established and realised when project planning, control and execution are appropriately exercised. At the modelling stage however, it is only the value of the asset that is being evaluated, independent of either how the asset construction is financed or when value flows, in and out, are scheduled.
Projects that do not provide intrinsic value do not merit further evaluation, but of those projects that do, the risks that can diminish their intrinsic value need to be fully identified and assessed at this stage for mitigation by suitable means. Thus, the quanta of value flows, their certainty and controllability, are the important conditions to be captured in the model at this stage, whilst guarding against excessive optimism or pessimism and retaining mindfulness that the value flow rendered is because of the asset itself and not due to any conditions surrounding it.
For example, lower taxes or grants from the government accruing to a climate risk addressing asset are relevant value inflows for its model, but the financing assistance that is available in the form of a lower cost debt instrument for constructing the above asset, belongs rightfully to the next stage. Apart from building a realistic model, sufficient attention has to be paid to embedding control points within the project for safeguarding the value created by the project. Furthermore, the opportunities provided by the modelling stage for programming proper risk response actions to protect appraised value should be fully utilised.
In the case of non-EU businesses exporting carbon-intensive products to the EU, modelling for CBAM involves appropriately capturing a carbon charge linked to the EU’s carbon market price that is currently around €90/tonne, as against the global average of $2/tonne. On the other hand, modelling for exporting to the US market may mean factoring in tax credits for supporting the development of green technologies. Moreover, as such tax credits are conditioned on local content requirements and may conflict with WTO rules, they are likely to be replaced, due to which the latter project model may have to be re-built sometime in the future.
Financing risks are linked to how the appraised, intrinsic project value is expected to be conveyed to the financiers of the project. This is the financing model for building the asset, and a project that is financed using incorrect instruments or employing inappropriate proportions of equity and debt is certain to reduce the project’s intrinsic value delivered to shareholders.
Assessing a project’s delivered value on the sole basis of a low-cost (green) debt instrument that is used to partly finance it may satisfy the returns demanded by the debt financiers but it will be destructive of overall shareholder value, as the benefits delivered are deemed lower than overall project costs. Whilst it is conceivable that such projects are deemed strategic and are hence undertaken despite their negative net present value (NPV), the project’s negative value add should be closely controlled during project execution. Further, and more importantly, the cost of capital used in evaluating a project is usually different from the actual achieved cost, and hence risk responses should be geared towards their equalisation.
Financing risks are unique and hence not controllable as part of project management techniques of planning, execution, and monitoring. For developing optimal responses to fluctuations in the cost of capital, detailed sensitivity analysis should be carried out by performing simulations such that in each iteration, the proportions of equity and debt are varied, in combination with their individual component costs. The resulting optimal ranges both for component costs and financing proportions should be adhered to in financing the project, and suitable financial risk responses should be programmed before project initiation for ensuring control over financing costs.
For non-EU businesses exporting carbon-intensive products to the EU, a favourable interest rate environment and high investor demand are currently permitting the issue of sustainable debt. Such debt was mainly in the form of green bonds earlier, but of late, so-called “brown” or “transition” bonds that encourage transiting to net-zero emissions, are becoming increasingly popular. Whilst green bonds provide the lowest cost financing for a compliant business, brown bonds start costlier but feature a step-down mechanism that lowers their cost to the level of green bonds until the time that the business becomes net-zero. More important however, brown bonds may include “step-ups”, which are key performance indicator (KPI) targets that are penalties for failure of the business to achieve the pre-agreed emission targets. It is necessary to adequately and realistically capture these targets and their associated costs in the financing model within the context of a risk response structure, for use during the subsequent and ultimate stage of the project.
Schedule realisation risks are linked both to project financing and to the project model and its execution. They fall into an entirely different category because the planned time schedule of investments and returns provides an estimate of the intrinsic value, whereas the actually realised time schedule underlines the delivered value of the project to its stakeholders.
Given that a discounted cash flow (DCF) model, based on a well-controlled cost of capital provides the most appropriate value for the project, any uncontrolled variances between planned and actual, inflows and outflows, will cause project values to fluctuate from the plan. The use of project management techniques to control both flow and timing variances is necessary at this time. This ultimate stage is therefore different from the two preceding it. Control over risks arising at this stage is essential to ensure full planned value realisation on the project.
The stage-wise identification and categorisation of risks on climate change and renewable energy projects, as pertaining to one of modelling, financing, or schedule realisation of investments and returns, helps in improved risk assessments and also permits the evolution of distinctive responses to the risks at their very origin.
At the modelling stage, risks to intrinsic value should be identified for mitigation. During the financing stage, risks that are linked to how the intrinsic project value is delivered to the financiers of the project should be identified for mitigation. Schedule realisation of a project leads to actual value delivery to stakeholders and control over risks at this stage is essential to ensure full planned value realisation from the project. As the risks at each of the three stages are separate and distinct, a stage-wise differentiation enables better understanding and allows the definition of targeted risk responses.
Carl Densem
Author
Ganesh Melatur
Ganesh Melatur is a Chartered Manager and an experienced strategic management professional. He is Fellow member of the Chartered Institute of Management Accountants (CIMA) and Fellow member of the Association of Corporate Treasurers (ACT). Possessing considerable experience in the planning, controlling and execution of projects as a Systems Engineer, he is a Project Management Professional (PMP) from the Project Management Institute (PMI) and Senior Member of the Institute of Electrical and Electronics Engineers (IEEE). Ganesh completed his MBA from the Alliance Manchester Business School, University of Manchester.
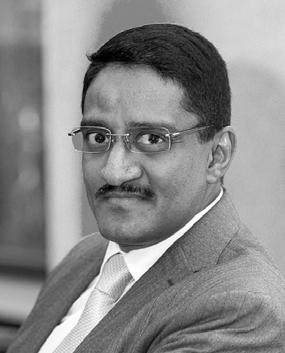
Synopsis
Rapid adoption of APIs is making application endpoints an increasingly attractive target for cyber criminals. API calls from within an app can provide a blueprint for critical backend infrastructure making it vulnerable to malicious attacks. This calls for a security roadmap beyond the traditional focus on the network layer.
mitigating cybersecurity risk through effective management of application programing interfaces (APIs)
by Preet Makkar
International Data Corporation (IDC) projects global digital transformation spending will reach $3.4 trillion in 2026. Post-pandemic, many businesses are focused on enhancing customer experiences and personalized customer journeys through newer and improved digital engagements, products, and services. Implementing technology solutions that leverage robotic process automation (RPA), artificial intelligence and machine learning (AI/ML), real-time data processing and the power of cloud computing can increase the operational resiliency of businesses against market disruptions.
A key capability enabling such solutions is the development of microservices based API-first modular application architectural patterns. With businesses recognizing APIs as a critical component to drive digital transformation use cases, adoption of APIs has increased exponentially in recent years. See Figures 1 and 2:
To what extent do you agree or disagree with the following statement? “APIs are an essential part of an organization’s digital transformation”
Figure 1: 98% of Enterprise leaders agree
Source: 2021 RapidAPI State of APIs
“Do you expect to rely on APIs more, less, or about the same in 2022?”
Figure 2
Source: 2021 RapidAPI State of APIs
While APIs have become integral to the cloud native application design patterns and for interoperability in a microservices architecture, managing API-related security risks has become paramount. According to a report by Imperva, unsecure APIs can significantly increase the cost of business operations attributed to cyber losses.4 See Figure 3:
Unsecure APIs can provide threat actors an attack route for backend systems and underlying infrastructure leading to breaches involving sensitive data. Increasing usage of cloud-based services and applications can inherently extend the attack surface through the use of managed infrastructure (virtual machines, storage, cryptographic systems etc.) as well as the availability of descriptive API documentation that can provide hackers with a better understanding of the functional layers of a web application. While cloud service providers build controls such as metering and tracking of API usage to address API security for managed services, organizations must understand the lifecycle of APIs they implement across their distributed hybrid cloud and multi-cloud IT environments in order to effectively manage risks and better govern their digital footprint.
API risk scenarios
Some common risk scenarios involving APIs include:
Incomplete API visibility: Time-to-market pressure and evolving application architecture and DevOps processes can render even well-designed API development, deployment and documentation practices inefficient. Incomplete visibility into the API inventory can persist the growth of shadow and zombie APIs which can impact security and monitoring practices leading to increased risk of cyberattacks. Hidden APIs can be a breeding ground for SQL injection attacks which use malicious SQL code to access backend systems. A recent survey5 by Noname Security indicates that 74% of companies do not have a full API inventory, impeding their ability to manage API related risks.
Unsecure API Endpoints: Threat actors can exploit API endpoints to retrieve Personal Identifying Information (PII) data, e.g. name, address, social security numbers, credit card information etc. by invoking API calls using basic parameters such as a mobile number us or enumeration of user IDs.
Broken user and object level authentication should be addressed through robust implementation of authentication and authorization protocols as well as testing of the business logic layer of the APIs. A recent data breach at Optus 6 appears to have involved an unauthenticated publicly accessible API endpoint which resulted in exposure of PII information of over 2 million customers.
Insufficient API Testing: Lack of sufficient testing of the API codebase and user interface (UI) integration layer during development can trigger significant remediation activities later in the production environment, thus delaying the overall API release timelines. In 2019, Centers for Medicare and Medicaid Services (CMS) experienced a coding error in their Blue Button 2.0 API7 used for providing access to beneficiary Medicare claims data. The issue persisted in production environment and was determined to have been caused by poor code quality review and validation process. The impact of the coding error could have led to inadvertent sharing of Personal Health Information (PHI) data with unintended recipients. Mock API endpoints can be used to accelerate the application development lifecycle by enabling front-end developers to build UI features and integrate testing against the mock endpoints in order to resolve bugs and defects earlier in the API lifecycle.
actions to manage APIs and mitigate risk
The following considerations are crucial for managing risk across the API lifecycle:
Automate API footprint discovery process to facilitate cataloging and risk classification of APIs, including internal and external APIs. Integrate the discovery process with security monitoring to manage unexpected API activity by leveraging log and traffic data from API gateways, cloud provider logs, content delivery networks, and other orchestration tools.
Deploy real-time threat detection and prevention countermeasures that continuously scan incoming data for vulnerabilities and business logic abuse, paired with real-time blocking and alerting. Integrate security monitoring with API testing during the development phase to conduct in-depth codebase reviews pre-production.
Safeguard secrets (e.g., API keys, tokens, passwords, certificates, etc.) from code leaks by implementing source code hygiene training for relevant stakeholders (developers, testers, system administrators, etc.). Implement a peer review process that incorporates automated review of sensitive data in addition to bugs and styling issues before committing any proposed code changes.
Leverage API schemas to identify and mitigate unexpected API usage patterns related to client interactions with API endpoints. Deploy API discovery tools with schema ingestion capabilities to enable API traffic pattern analysis by understanding methods and data components. The analysis can feed the implementation of business logic rule-based workflows for accessing API endpoints (e.g., blocking unused HTTP methods, implementing rate limiting to mitigate DDoS attacks, etc.) that can mitigate malicious attacks.
Conclusion
Strategic management of APIs can scale and effectively monetize APIs while mitigating the risks associated with API security and implementation deficiencies. Implementing a well-designed API governance model can enable uniformity and compliance with standards covering the API lifecycle. In addition, it can provide clarity on roles and responsibilities to key stakeholders (e.g., product owners, developers, testers, and security teams) that can supplement data privacy and information security compliance across the organization’s API sprawl. Further, by automating key API-related processes, such as API documentation, testing, and security assessments, an organization can gain a more timely view of its API inventory and identify potential security vulnerabilities and business logic flaws. This allows for quicker triage and remediation of events such as account takeovers, fake account creation, or scraping by malicious actors.
Disclaimer: All views expressed in this article are author’s own based on his practical client side and consulting experience working in the data analytics field. These views do not represent the opinion of any entity author has been or is associated with
Carl Densem
Preet Makkar
Preet Makkar is a Data Analytics Subject Matter Professional at U.S. Bank with a broad experience in data governance, data management, analytics and technology domains. As a Principal, Data Analytics at Cuda Data Analytics, he has authored several thought leadership articles covering topics such as Cloud Data Governance, Data Privacy and Open Source Technologies. He previously worked at KPMG, where he oversaw the delivery of large-scale transformation projects aimed at leveraging data and analytical solutions to improve the strategic execution of risk-driven processes for his clients. Over his consulting career, he has served more than a dozen global and US domestic financial institutions in managing core data analytics initiatives, covering strategy, design, implementation and risk management. Preet holds an MBA in Business Analytics from Bentley University and a B.S. in Business from University of Technology, Sydney.
Fraud costs organizations dearly and, unlike some other risks, is present across countries and industries. The repercussions of ignoring it are well-documented in case studies. Still, better and worse approaches make a difference to the magnitude of fraud losses, as well as reassure management and the Board that everything is being done to identify and address fraud wherever it may pop-up next.
fraud risk management: an assurance approach
by Dami Osunro
Alan Greenspan, Former Chair of the Federal Reserve of the United States
Introduction
Given the reality that all companies face the risk of fraud, this article seeks to make a case for an assurance approach to fraud risk management rather than a siloed approach. A siloed approach is where just one assurance unit takes responsibility for fraud risk management, while an assurance approach suggests an active coalition of various assurance units in the management of fraud risk.
The Reality Of Fraud
Agnes has been solely running the fraud risk program of her company for the last 5 years. She is an Internal Control officer, so the management thought it best to have her manage the fraud risk program since she would be able to initiate appropriate controls head on based on whatever she finds.
The problem, however, is that the company has had at least one major fraud incident in the last 3 years, the last one almost taking the company out. Agnes is feeling the heat, and everyone is fearing when the next one might hit.
While the story above is an adapted version of a true situation, the reality of it stares many companies in the face. The Association of Certified Examiners (ACFE) Report to the Nations for the year 2022 (The ACFE, 2022) estimates that organizations lose 5% of revenue to fraud each year. It can’t be said that Agnes is not trying all she can, but she can only see as far as she knows. When interviewed, it was discovered that Agnes had been running the fraud program independently just as the company policy had stipulated. Herein lies the pitfall though.
how do you gauge the susceptibility of your company to fraud?
Many companies are in the same situation as Agnes’ company while some are even in worse situations. A Director at Agnes’ Company began asking some questions to determine the company’s fraud risk management maturity. These questions included: when was the last time the company carried out a fraud risk assessment? Does the company have a fraud risk program? If the company has a fraud risk program, how robust is it when compared to best practices? Then comes the question, is the fraud program operating a standalone approach or an assurance approach? This was found to be a major issue with Agnes’ company’s fraud risk management program. They were operating a standalone approach where only the internal control team was involved in managing the fraud risk program.