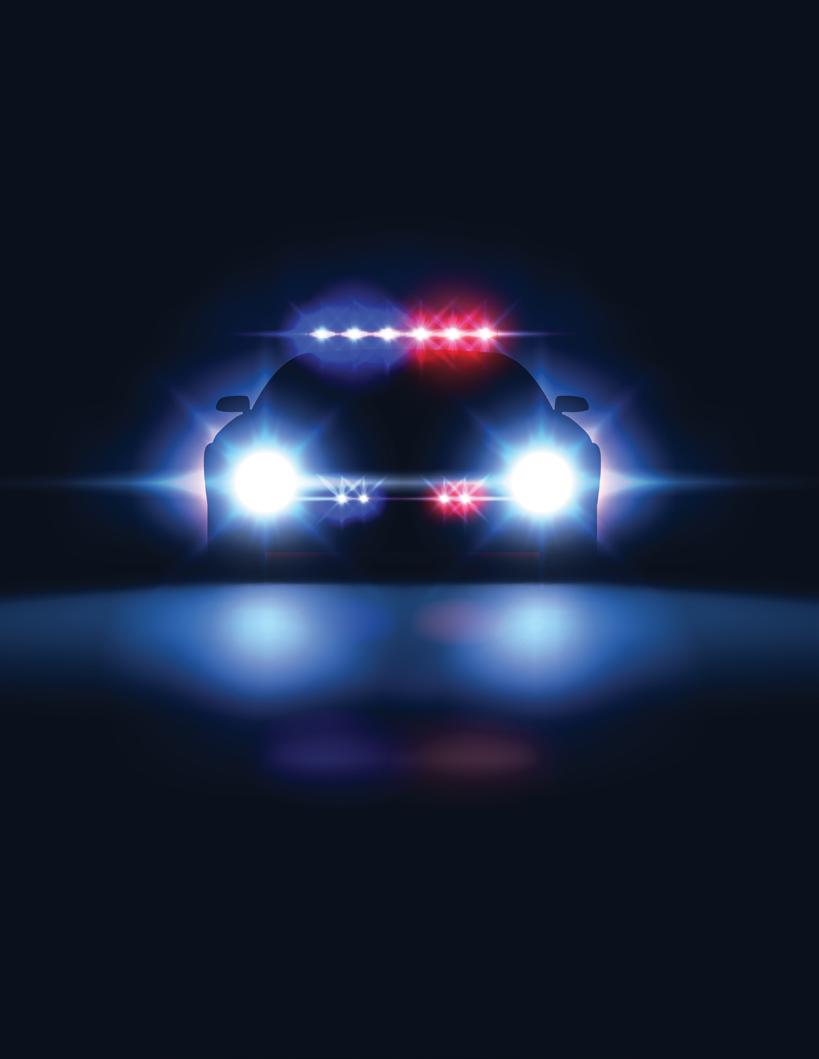
6 minute read
CAN WE PREVENT THE NEXT CRITICAL INCIDENT?
Case Study on Improving Law Enforcement Outcomes with Evidence-Based Risk Management
BY STEVE BREWER
Advertisement
WHY DO SOME LAW ENFORCEMENT AGENCIES have up to 60% fewer critical incidents, officer injuries or accidents than other agencies of similar size, serving similar communities?
This question and a mission to help its member agencies reduce officer injuries and improve community outcomes prompted a large risk pool in the Midwest to embark on a partnership with Benchmark Analytics. The pool administers property/casualty and workers compensation coverage for cities throughout their state and further supports members with a robust loss control program.
However, due to continuing trends of increasing frequency and severity of law enforcement related claims, the pool and its board sought new ways to harness data and analytics to offset these trends. Benchmark designed a data-driven, continuous improve- ment program based on proven Statistical Process Control (SPC) methods. The program was configured to support unique aspects of municipal government and risk pool needs.
In any industry, the practitioners must implement changes to improve outcomes. Results show strong engagement from agency command staff who find program analytic insights actionable, relevant, and timely to help them prioritize time and resources to act. This is an important leading indicator to measure program success.
This case study provides ideas applicable to any public sector or insurance risk management program. Through my work with Benchmark supporting municipalities and risk pools across the country, I see incorporating capabilities of SPC into risk management as one of the biggest opportunities to improve the practice of municipal risk management today.
The Loss Control Paradox
If a risk management program mitigates risk and prevents a claim, did the risk ever exist in the first place? How can we know if a loss control investment is working?
The inability to measure loss control programs is the biggest blocker to risk management success today. When we can’t measure the benefit program initiatives become hamstrung by limited adoption, funding constraints, changing priorities and resistance from the practitioners we need to implement the risk management into their everyday work. Rarely do risk managers incorporate data capture and analysis design into new program business plans which results in stakeholders wondering if a program worked and filling in their own perspective on the value of the investment.
State Of Practice For Municipal Risk Management
Most public sector risk management programs are Initiative-Driven or Individual-Driven Approaches as illustrated in Figure 1.
In the Law Enforcement world, risk managers offering an Initiative-Driven Approach deploy one or more programs such as a virtual training simulators, policy manual subscriptions, training courses, or equipment grants. Typically, the same programs are offered to all members with the assumption that every member should participate and would get equal benefit from doing so. Agency command staff (the practitioners from an SPC perspective) usually see these initiatives as extra work and only engage them if the grants align with a priority the Chief of Sheriff has already identified. Feedback from risk pool and municipal risk managers is that these programs suffer from low adoption.
Risk Managers offering an Individual-Driven Approach typically deploy a former law
Initiative-Driven Approach
Individual-Driven Approach
enforcement or corrections leader as a loss control consultant who meets with each Agency to discuss operations and recommend changes command staff can implement to reduce risk. This approach can feel personalized and deepen critical relationships between and agency and its risk management partner. However, recommendations are typically vague and/or not aligned with command staff priorities. In the end, Agency leaders usually see these recommendations as extra work and out of reach for agency budget and competing priorities unless they tie directly back to a significant loss or regulatory requirement. Feedback from risk pool and municipal risk managers is that recommendations don’t often get implemented due to resource constraints and the lack of a mandate.
The result? Both approaches fail to gain traction and achieve sustained qualitative or quantitative success.
APPLYING A PROVEN APPROACH TO PUBLIC SECTOR RISK MANAGEMENT What can we learn from successful methodologies in other industries?
Statistical process control (SPC) is a wellknown, data-driven approach to quality control that uses statistical methods to monitor and control a process. SPC can be used in risk management to improve outcomes by identifying and addressing potential problems before they become significant issues. SPC can help identify potential risks by monitoring data from processes over time. By measuring the variation in the process output, SPC can identify when the process is not operating as expected.
For example, in a manufacturing process, SPC can be applied via Six Sigma or similar frameworks to monitor the quality of the product being produced. By tracking the variation in product quality over time, the process can be monitored to ensure that it is operating within the desired parameters. In a healthcare setting, SPC can be applied via Quality Improvement (QI) frameworks and can be used to monitor patient safety. By tracking the number of adverse events over time, healthcare providers can determine if the safety of patients is being maintained.
Applying SPC to public sector risk management programs is a powerful addition. Most loss control staff utilize claims runs to inform their Loss Control Approach. Prior claims are informative but alone are insufficient. Without statistical context we can’t know if prior claims patterns are within expected ranges. For small Agencies, few claims certainly doesn’t mean low risk. This results in loss control recommendations aimed to prevent recurrence of a prior event but unlikely to address key risk factors which haven’t yet resulted in claims. This is
Figure 2: Frequency Thresholds for Law Enforcement Liability, by Agency Size
learn and replicate the factors that make top performing agencies successful.
EVIDENCE-BASED PRACTICES
Can law enforcement agencies really reduce officer injuries and critical incidents, or are these risks inherent to the communities they serve?
Through our work in this case study, we observed that community factors showed little to no correlation with a law enforcement Agency’s predicted claims outcomes. That is, the crime rate, median income, education levels, demographic and socioeconomic factors had very little impact on an Agency’s expected claims. Rather, an agency’s operational practices were far more predictive of expected claims thresholds. With this insight, we set out to systematically capture key aspects of agency operations and statistically measure which factors had the strongest correlation to reduced claims outcomes. We call these “EvidenceBased Practices” which are actual policies, operations, programs, training or equipment whose implementation shows statistical correlation to reducing claim frequency or severity.
akin to driving a car by only looking in the rear-view mirror. We’re destined to frequently run off the road at each turn and crash into obstacles we can’t see until we are past them.
Case Study Results
This brings us back to our case study with a large risk pool in the Midwest. In this program, we integrated SPC into their loss control program in two ways:
• Predictive Risk Profiles
• Evidence-Based Practices
Predictive Risk Profiles
By analyzing time series data of payments such as claims, we established expected frequency and severity thresholds for member police departments, by peer group. Peer groups included a multitude of factors about the agency and community they served. Figure 2 shows a time series of typical distribution of property/casualty claims for agencies serving different size communities. Size of agency or population served is a critical variable to consider when establishing SPC thresholds — especially for smaller agencies where a single claim could be considered an outlying event.
We then deployed predictive analytics to identify expected frequency (number of claims) and severity (cost of claims) expected for each agency by line of coverage. These models also identify risk attributes that most contributed to the threshold prediction.
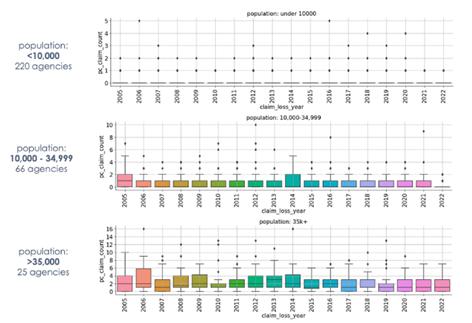
These insights significantly advanced the pool’s public safety loss control expert’s understanding of risk levels and contributing factors for each member Agency. These insights also elevate a loss control reps’ status as trusted advisor to agency command staff and municipal leaders. Agencies outperforming their peers can be recognized and engaged as a reference point and source of insight as to what practices have made them successful. Agencies under-performing their peers can receive specific recommendations based on their unique risk factors. Agency command staff and municipal leaders tell us they appreciate data-driven insights and the opportunity to
Like all good research and analytics, the more we discover, the more we want to know. We have a long list of new loss control practices to continue researching and analyzing. Recent results yield a critical insight: Law Enforcement command staff are eager to learn about Evidence-Based Practices and deploy them within their agency. Research and statistical evidence supporting recommendations help command staff prioritize changes and justify investment from their municipal councils.
Leading metrics of success are the number of agencies and municipalities adopting new Evidence-Based Practices. Lagging metrics are lower claims frequency and severity. With each iteration of the SPC-driven loss control cycle we learn more, better prioritize resources and empower practitioners to make data-driven decisions as part of their everyday agency operations.
Steve Brewer, Partner, Benchmark Analytics