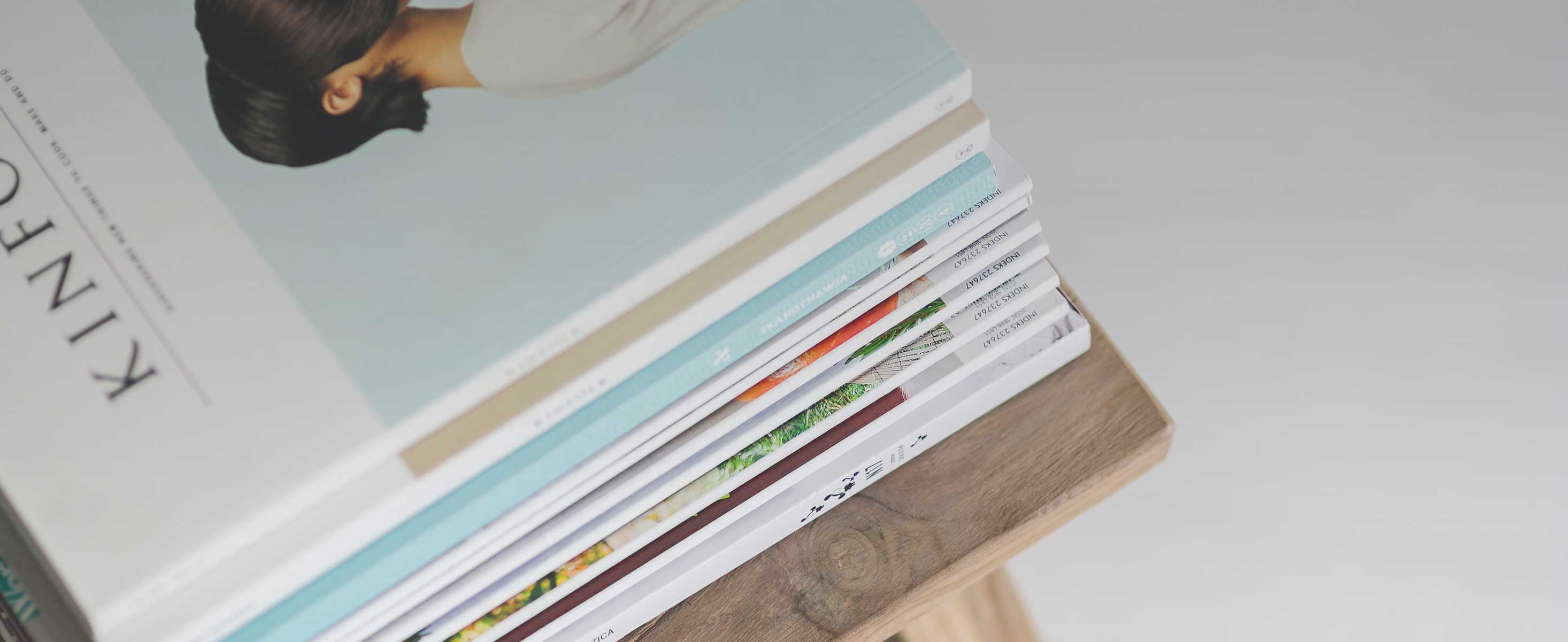
2 minute read
AWS offers fast-track to developing generative AI apps for healthcare
BY JERRY ZEIDENBERG
TORONTO – While Microsoft and Open AI have recently been the focus of media attention when it comes to generative AI – thanks to the massive uptake of the ChatGPT app –Amazon Web Services (AWS) is no slouch, either, on the artificial intelligence front.
At the e-Health 2023 conference, held in May, AWS’s Fred Azar asserted that “100,000 customers have already used AWS AI and ML capabilities.”
Dr. Azar leads AI and ML business development as part of AWS’s worldwide public sector team. He and colleague Dr. Rowland Illing, chief medical officer at AWS, together outlined the resources that AWS brings to healthcare organizations using AI and machine learning in the cloud. Dr. Illing claimed that AWS is also the most broadly adopted cloud provider, with millions of active customers around the world.
Running AI in the cloud means that customers can develop and use advanced applications without buying or maintaining their own infrastructure. It can all be done remotely and scaled up or down as needed.
Dr. Illing said Canadian customers using a range of AWS cloud services include AlayaCare, BC’s Provincial Health Services Authority (PHSA), the Canadian Institute for Health Information (CIHI), Ontario’s Central East Hospital Cluster (CEHC) and WELL Health Technologies.
These centres are using the cloud to better manage their fast-growing data.
Dr. Illing noted that in addition to the current sources of data, such as diagnostic imaging centres, there are new ones just on the horizon. In the future, consumer data from wearables, implantables and ingestibles will be connected to patient records, creating a sizeable jump in the volume of data collected by hospitals.
In the last year alone, said Dr. Azar, AWS produced 250 new AI products and services. Customers using AWS’s AI services include Pfizer and Moderna, who deployed the AWS cloud and machine learning assets to help develop the COVID-19 vaccines in record time.
He mentioned that Grey Bruce Health Services, a 400-bed organization in Ontario, wanted to digitize its large base of paper-based, legacy health records. This was done to help clinicians to obtain the historical records they needed more quickly and to reduce the amount of physical space needed for storage.
To accomplish the task, Grey Bruce partnered with Iron Mountain and made use of the AWS cloud and AI to clean the data and convert it into a digital format. It was accomplished with the help of Iron Mountain’s InSight application us- and corrected by the physician afterwards, to ensure accuracy while reducing the time needed for paperwork and allowing him or her to focus on the patient during the encounter. ing large-scale scanning and optical character recognition.
Dr. Azar discussed the emergence of generative AI, based on large-language models. These systems have abilities far beyond earlier AI frameworks, as they are able to carry out tasks in plain English – and many other languages. One doesn’t need to be a data scientist or programmer to use them, anyone can instruct them and use them.
On another front, AWS is involved in a project to reduce the charting load on physicians at Houston Methodist Hospital, with an application that uses AI for natural language processing and ambient listening during the patient/clinician encounter.
The application has been trained to capture the medical problems, diagnosis and treatment being discussed, and to automatically chart it. It can be reviewed
The large-language models need to be trained for certain tasks before they can be deployed effectively. For its part, AWS has its own trained family of foundation models, called Titan. It has also partnered with other companies offering different foundation models, including AI21 Labs, Anthropic and Stability.ai.
Customers can experiment with these foundation models online to see which one would suit their needs most closely.
A major benefit, said Azar, is that the healthcare organization’s data is not needed to initially train the model – it has already been trained, saving time and
CONTINUED ON