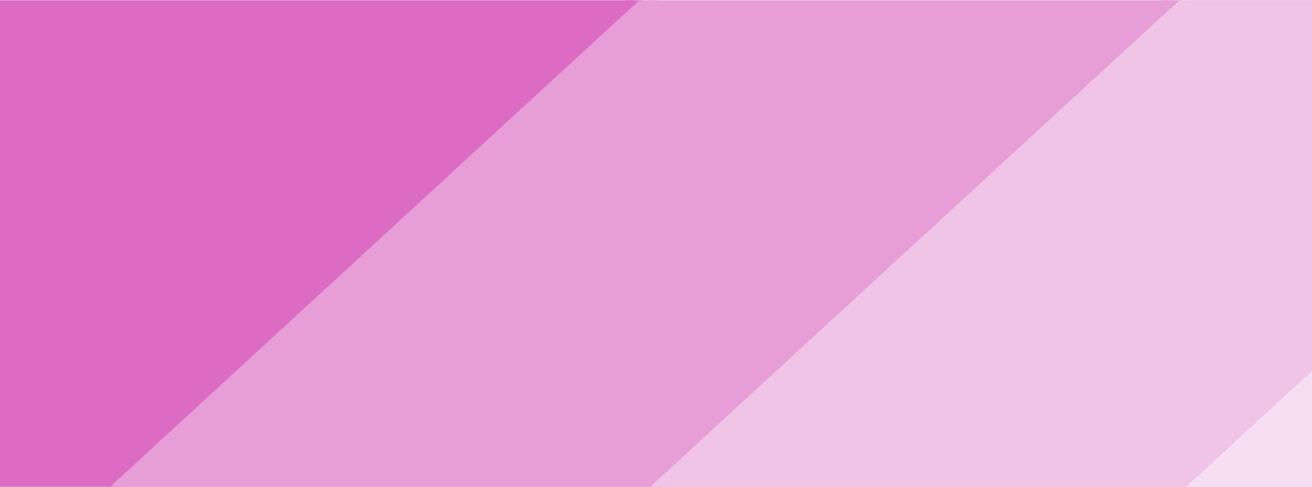
5 minute read
How Should We Incorporate AI into Clinical Judgement?
By Sipan Haikazian
The impact of artificial intelligence (AI) on the healthcare system is becoming increasingly hard to overlook. The global market for AI technology is predicted to increase at a compound annual growth rate of 37% by 2030.1 With its ability to analyze and make predictions from a large amount of data, leading experts believe that AI can help healthcare professionals prevent disease, make accurate diagnoses, and suggest treatments tailored to specific patients.2
Advertisement
Although relevant in many aspects of healthcare, AI is set to play an extremely significant role in clinical judgement, an aspect of healthcare that is often the most relevant to patients. Clinical judgement refers to the application of knowledge and skills about best medical practices over time, gained through analysis and synthesis of patient data. Clinical judgement can be a complex task for healthcare providers, and AI technology has the potential to greatly aid healthcare providers in the important decisions they make involving patient treatment. However, there are concerns that AI may lead to a loss of critical thinking in physicians, eventually fully replacing human judgment in decisionmaking.3 While it is hard to believe that AI will ever autonomously make diagnoses and treatment recommendations for patients, it is important for us to understand where AI stands in the clinical decision-making process. And it is crucial that AI is viewed as merely a supportive tool.
But what exactly is AI referring to, and why is there so much hype about its use in healthcare? The term “AI” has been around for decades now. AI in itself is a catchall term for a multidisciplinary field that focuses on creating computers which perform tasks normally associated with human intelligence. Within AI, there is a branch called machine learning, where computers use patterns from structured data (also known as the training data) to construct algorithms: a set of rules that computers follow to carry out pre-specified operations. These operations are carried out on data that is significant to a particular goal (also known as the testing data). Technology that uses machine learning differs from earlier forms of AI that relied on pre-programmed rules to perform tasks.
However, a further subset of machine learning called deep learning is what is driving the hype behind AI in recent years.4 One 2017 study published in Nature found that a deep learning system was capable of classifying skin cancer, both common and uncommon types, at a level of competence comparable to certified dermatologists.5 And that study was published six years ago. Recently, one study found that a deep learning algorithm could predict whether patients who suffer from less severe forms of acute kidney injury (AKI) would progress to a more fatal form given their current symptom characteristics.6
In deep learning, computers recognize patterns from unstructured data and use these patterns to make algorithms, ultimately leading to predictions. This “new” AI finds patterns and constructs algorithms from training data with lesser human intervention required.
Some advantages of deep learning are apparent. These algorithms can identify patterns and correlations in health data that may be missed by humans, leading to more accurate diagnoses and treatment strategies. 7 This is especially useful as a preventative measure. AI has the potential to predict patient outcomes based on their medical history, potentially leading to preventative treatment strategies. An example of this was presented earlier with the deep learning system that classified cancer. Furthermore, AI can be trained to recognize differences in disease characteristics between patients, and then suggest interventions that are tailored to the patient. 6 This feature is relevant as healthcare is increasingly adopting the precision medicine model of personalized and tailored diagnoses and interventions.
Other advantages of AI-based technologies in healthcare include an objective assessment of patient data. All healthcare professionals may harbour some amount of bias in the clinical setting based on patients’ race, age, and socioeconomic status, but since AI is only trained on raw data (in the absence of any external manipulation), it produces more objective predictions.
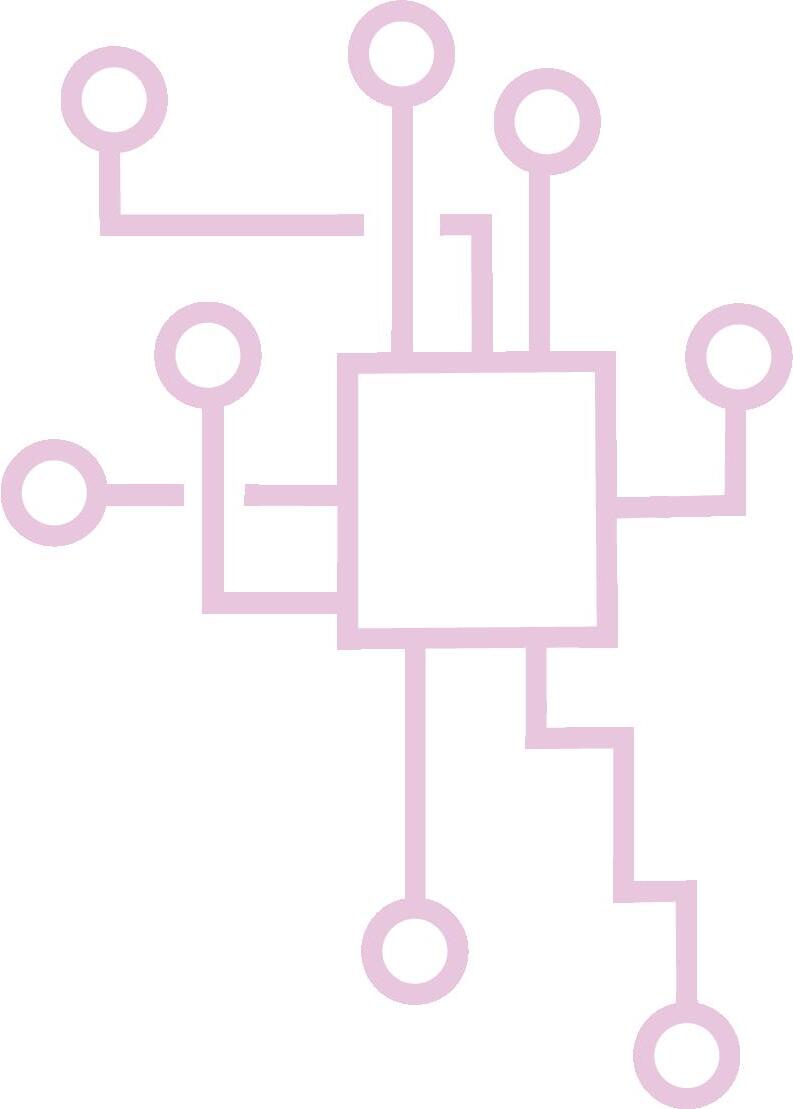
However, because AI systems are only as good as the data on which they are trained, AI-generated predictions may have their own faults. If the training data is incomplete or biased in some way, the predictions that AI makes can be inaccurate. Furthermore, AI may not take into account the patients’ social and cultural contexts. For example, if the data that AI uses to make its predictions is trained primarily on white, middleclass males, predictions would be less accurate for individuals belonging to other demographic groups. If an over-reliance is placed on treatment recommendations generated from AI, these important factors will be omitted. AI may also reinforce biases and discrimination through its data-driven predictions, strengthening inequalities in healthcare.
While developers of AI may address some of these aspects as advancements are made, human oversight is still required. AI systems should be continually monitored and evaluated to test for accuracy, especially as the technology becomes increasingly autonomous. AI algorithms should be transparent, and the data used to train them should be representative of diverse populations. Moreover, healthcare professionals need to ensure that AI follows ethical principles and that the predictions that are made consider factors not captured by patient data (e.g., the aforementioned sociocultural context). And no matter how advanced AI becomes, there is something comforting about having a human physician make the final call for diagnosis and treatment.
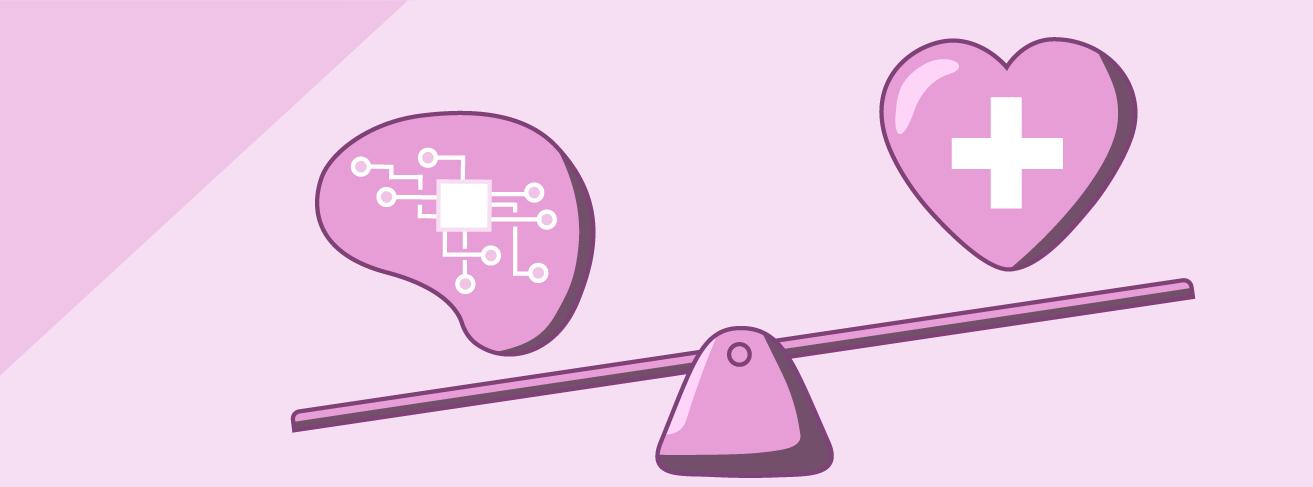
If AI is used solely as a supportive tool alongside human judgement, healthcare will only benefit. AI can help healthcare professionals come to conclusions from a large amount of data, implement preventative interventions, and support patient monitoring and follow-up. However, it is important to recognize the flaws inherent to AI, which highlight the importance of cautionary integration into healthcare. For healthcare providers, it should only be used as a supportive tool rather than a complete substitute for clinical judgment. Moreover, AI should be implemented ethically and appropriately, requiring oversight from all stakeholders in healthcare. Ultimately, AI presents opportunities for massive improvements in how we deliver healthcare, but if we wish to see this success, it is imperative that healthcare providers follow a model of patient-centered and evidence-informed care where AI plays a supportive role in clinical decision-making.
References
1. Stewart C. Artificial Intelligence (AI) in healthcare market size worldwide from 2021 to 2030 [Internet]. 2023 Mar [cited 2023 Apr 17]. Available from: https://www.statista.com/statistics/1334826/ai-in-healthcare-market-size-worldwide/#:~:text=In%202021%2C%20the%20artificial%20 intelligence,percent%20from%202022%20to%202030
2. Bohr A, Memarzadeh K. The rise of artificial intelligence in healthcare applications. In: Artificial Intelligence in Healthcare [Internet]. Elsevier; 2020 [cited 2023 Apr 16]. p. 25–60. Available from: https://linkinghub. elsevier.com/retrieve/pii/B9780128184387000022
3. Froomkin AM, Kerr IR, Pineau J. When AIs Outperform Doctors: The Dangers of a Tort-Induced Over-Reliance on Machine Learning and What (Not) to Do About it. SSRN Journal [Internet]. 2018 [cited 2023 Apr 16]; Available from: https://www.ssrn.com/abstract=3114347
4. Pettit RW, Fullem R, Cheng C, Amos CI. Artificial intelligence, machine learning, and deep learning for clinical outcome prediction. Emerging Topics in Life Sciences. 2021 Dec 21;5(6):729–45.
5. Esteva A, Kuprel B, Novoa RA, Ko J, Swetter SM, Blau HM, et al. Dermatologist-level classification of skin cancer with deep neural networks. Nature. 2017 Feb 2;542(7639):115–8.
6. Wei C, Zhang L, Feng Y, Ma A, Kang Y. Machine Learning model for predicting acute kidney injury progression in critically ill patients. BMC Med Inform Decis Mak. 2022 Jan 19;22: 17. Available from: 10.1186/ s12911-021-01740-2
7. Johnson KB, Wei W, Weeraratne D, Frisse ME, Misulis K, Rhee K, et al. Precision Medicine, AI, and the Future of Personalized Health Care. Clin Transl Sci. 2021 Jan;14(1):86–93.